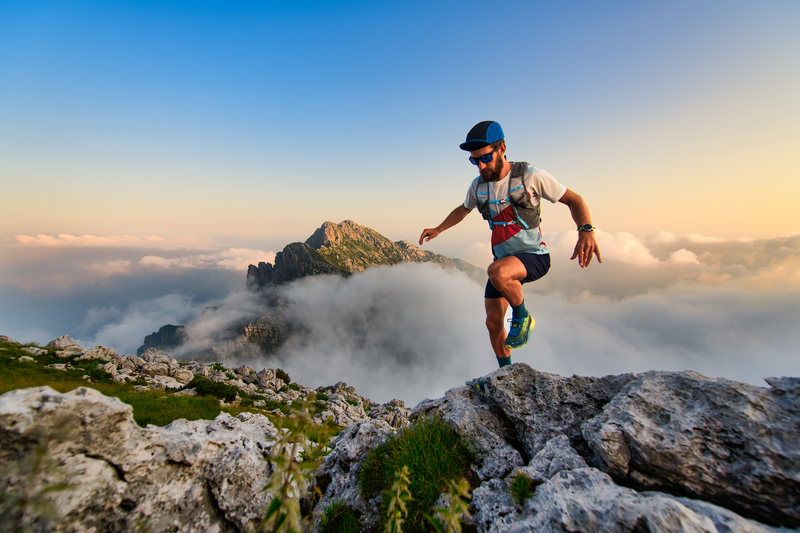
95% of researchers rate our articles as excellent or good
Learn more about the work of our research integrity team to safeguard the quality of each article we publish.
Find out more
EDITORIAL article
Front. Energy Res. , 12 July 2021
Sec. Nuclear Energy
Volume 9 - 2021 | https://doi.org/10.3389/fenrg.2021.719245
This article is part of the Research Topic Nuclear Power Plant Equipment Prognostics and Health Management Based on Data-driven methods View all 12 articles
Editorial on the Research Topic
Nuclear Power Plant Equipment Prognostics and Health Management Based on Data-Driven Methods
In response to the fierce competition in the energy market, nuclear power companies are considering operating nuclear power plants in a more economical, efficient, and safe manner. Besides, with the upgrading of nuclear power plants, systems and equipment are becoming more sophisticated and expensive (Kwon et al., 2018), which poses challenges to the timeliness, accuracy, and forward-looking of operation and maintenance (O&M) practices (Al Rashdan and St Germain, 2018; Liu and Wang, 2019). Traditional O&M practices with periodic maintenance as the core need to be further upgraded to meet these requirements. As a novel O&M strategy, data-driven health management of nuclear power plant equipment is gaining more and more attention (Patel and Shah, 2018). On the one hand, the digitization of nuclear power plants provides a rich source of data. On the other hand, the development of data science and technology, especially the development of big data technology and artificial intelligence technology represented by machine learning and deep learning, provides technical means for efficiently mining and learning laws and knowledge from data. This Research Topic explore's the application of the latest technical means such as big data, artificial intelligence, deep learning, etc. for the prognostics and health management (PHM) of crucial equipment of nuclear power plants. We include the advanced sensor technology. For example, Chu’s work Study on Measure Approach of Void Fraction in Narrow Channel Based on Fully Convolutional Neural Network (Chu et al.). Besides, we have three articles about the data-driven approach in condition monitoring, which include Xu’s work Research on Time-Dependent Component Importance Measures Considering State Duration and Common Cause Failure (Xu et al.), Huang’s work Data-Driven-Based Forecasting of Two-Phase Flow Parameters in Rectangular Channel (Huang et al.), and Wang’s work A Method of Containment Leakage Rate Estimation Based on Convolution Neural Network (Wang et al.).
For the data-drive approach in fault diagnosis, we collect four articles, they are Wu’s A Framework for Monitoring and Fault Diagnosis in Nuclear Power Plants Based on Signed Directed Graph Methods (Wu et al.), Hu’s Data-Driven Machine Learning for Fault Detection and Diagnosis in Nuclear Power Plants: A Review (Hu et al.), She’s Diagnosis and Prediction for Loss of Coolant Accidents in Nuclear Power Plants Using Deep Learning Methods (She et al.), and Wu’s A Framework of Distributed Fault Diagnosis for Nuclear Power Plant (Wu et al.). We also have one article for the data-driven approach in prognostics, it is Wang’s Remaining Useful Life Prediction Based on Improved Temporal Convolutional Network for Nuclear Power Plant Valves (Wang et al.). Zhao’s Prognostics and health management in nuclear power plants: an updated method-centric review with special focus on data-driven methods (Zhao et al.) provides a systematic overview of the full PHM spectrum and an in-depth survey of its modeling approaches, placing a strong emphasis on the state of the art of data-driven methods for PHM. Finally, Sun’s Development and Validation of Multiscale Coupled Thermal-Hydraulic Code Combining RELAP5 and Fluent Code (Sun et al.) contributes to the simulation capability of computational tools for nuclear systems.
All authors listed have made a substantial, direct, and intellectual contribution to the work and approved it for publication.
The authors declare that the research was conducted in the absence of any commercial or financial relationships that could be construed as a potential conflict of interest.
Al Rashdan, A. Y., and St Germain, S. W. (2018). Automation of Data Collection Methods for Online Monitoring of Nuclear Power Plants. Idaho Falls, ID (United States): Idaho National Lab.(INL). doi:10.2172/1475451
Kwon, S., Kim, B., An, K., Ryu, D., Mun, D., and Han, S. (2018). Standardized Exchange of Plant Equipment and Materials Data Based on ISO 15926 Methodology in Nuclear Power Plants. Ann. Nucl. Energ. 118, 185–198. doi:10.1016/j.anucene.2018.04.001
Liu, B.-Y., and Wang, M.-M. (2019). Research and Application of Reliability Management Process in Nuclear Power Plants. 2019 International Conference On Quality, Reliability, Risk, Maintenance, and Safety Engineering. QR2MSE, 224–229.
Keywords: advanced sensor, fault diagnosis, data-driven approach, condition monitoring, prognostics
Citation: Wang J, Zhong X, Zhao X, Yurko JP and Revankar ST (2021) Editorial: Nuclear Power Plant Equipment Prognostics and Health Management Based on Data-Driven Methods. Front. Energy Res. 9:719245. doi: 10.3389/fenrg.2021.719245
Received: 02 June 2021; Accepted: 28 June 2021;
Published: 12 July 2021.
Edited and reviewed by:
Uwe Schröder, Technische Universitat Braunschweig, GermanyCopyright © 2021 Wang, Zhong, Zhao, Yurko and Revankar. This is an open-access article distributed under the terms of the Creative Commons Attribution License (CC BY). The use, distribution or reproduction in other forums is permitted, provided the original author(s) and the copyright owner(s) are credited and that the original publication in this journal is cited, in accordance with accepted academic practice. No use, distribution or reproduction is permitted which does not comply with these terms.
*Correspondence: Xianping Zhong, eGlhbnBpbmcuemhvbmdAcGl0dC5lZHU=
Disclaimer: All claims expressed in this article are solely those of the authors and do not necessarily represent those of their affiliated organizations, or those of the publisher, the editors and the reviewers. Any product that may be evaluated in this article or claim that may be made by its manufacturer is not guaranteed or endorsed by the publisher.
Research integrity at Frontiers
Learn more about the work of our research integrity team to safeguard the quality of each article we publish.