- College of Economics and Management, Taiyuan University of Technology, Taiyuan, China
There is no choice for energy businesses with ongoing epidemic prevention and control but to adapt themselves to the epidemic. Analyzing the decisions of energy enterprises on adaptive behaviors amid the Corona Virus disease 2019 (COVID-19) pandemic goes a long way toward economic and social stability and successful containment of the pandemic. With a phone-based or online (Tencent QQ and WeChat) survey on 500 energy companies in 17 prefecture-level cities and autonomous prefectures in Central China’s Hubei Province, we examined the types of adaptive behavior these businesses had chosen to respond to the virus. We utilized the entropy weight method (EWM) and identified key factors that impacted how these companies made decisions on adaptive behavior. On that basis, we compared the samples with state-owned enterprises and private businesses in terms of the criticality of factors affecting their decisions on adaptive behavior. The significant findings are as follows. First, the adaptive behaviors of Hubei-based energy companies were for philanthropic, economic, or technical purposes. Besides, concerning the confidence level, the central government’s general requirement for epidemic prevention and control, current economic realities, cost-benefit analysis of adaptive behavior, the awareness of corporate social responsibility, and energy policy support from governments were key factors that affect decisions of Hubei’s energy enterprises on adaptive behavior. Their criticality rates 0.999 6, 0.999 5, 0.999 0, 0.997 1, and 0.995 8, respectively. Moreover, the key affecting factors of these samples differed from those of energy enterprises of a different nature. The nature of energy businesses holds sway over the identification of those key factors. The criticality of which also varies with the distinctive nature of the enterprises. Finally, we presented the theoretical implications of the present work and policy-making recommendations.
Introduction
As of November 17th, 2020, more than 55.3 million Corona Virus disease 2019 (COVID-19) cases were confirmed worldwide. Not only has the raging pandemic threatened the wellbeing of humankind, but it led to an uncertain global economic outlook. “Globally, the pandemic is actually speeding up,” said Tedros Adhanom Ghebreyesus, Director-General of the World Health Organization. “The critical question that all countries will face in the coming months is how to live with this virus. That is the new normal.” To reduce the impacts of the pandemic on the global economy, such international organizations as WTO, UNDP, IMF, and G20 have called on the international community to take every necessary measure to curb the spread of the virus. It is widely accepted in the global community to adapt ourselves to new COVID-19 realities. How we live with the virus will be an inevitable part of all people’s work and life for a long time to come, Dr. Barry R. Bloom, former Dean of Faculty at Harvard T.H. Chan School of Public Health stressed at the “Global Front Against COVID-19” symposium. Adaptation has been high on the global policy agendas on public health and economic recovery, and research efforts on adaptability brook no delay as the virus continues to spread, while pandemic prevention and control becomes part of the “new normal”. Such studies were carried out by a great number of scholars across the world, and they focused on how local governments, along with urban and rural communities, took measures to adapt and respond to pandemics or public health emergencies of international concern, such as influenza (Nigmatulina and Larson, 2009; Kim et al., 2014; Reissman et al., 2011), malaria (Shretta et al., 2017; Obol et al., 2018), SARS (Leung and Won, 2005), Ebola (Abayomiet al. 2019; Carter et al., 2017), and COVID-19 (Angel and Mudrazija, 2020). Beyond that, some examined changes to trade policies given the spread of a pandemic and global economic recession (Pak et al., 2020; Brenton and Chemutai, 2020), while others studied how small and medium-sized businesses broke through, survived, and reenergized in a pandemic crisis (Zhang and Han, 2020). Most research based its analysis on the sustainable livelihoods framework, cognitive-behavioral theory, and the theory on the adaptability of social-ecological systems, and concentrated on exploring the stress response, social mentality, behavioral regulation, and coping strategy of organizations or individuals facing a pandemic (Gatiso et al., 2018; Main et al., 2011; Li et al., 2020b; Samaraweera, 2018). In a nutshell, the emphasis of previous studies was on how residents and such organizations as government agencies, urban and rural communities, and enterprises acted and took measures to adapt to a pandemic crisis and its aftermaths, and on the results that they achieved in this process. Nonetheless, little attention has been paid to the adaptability of energy enterprises to the COVID-19 pandemic, and the factors that affect decision making on their adaptive behaviors remain to be expounded.
Enterprises producing or marketing energy products are crucial for the availability of energy sources. These energy companies can ensure economic and social stability by supplying such strategic resources tied up with residents as electricity, natural gas, coal, and petroleum. As devoted formidable forces against COVID-19, they offered protective materials and daily necessities to hospitals, disease control centers, producers of medical supplies, high-speed rail stations, airports, and other facilities essential to people’s wellbeing. Meantime, the supply and transportation of products of energy companies were hit hard by the epidemic, and the resumption of services, industry and commerce was slow and restricted, which resulted in a decrease in energy consumption. The epidemic posed a grave challenge to the survival and development of energy enterprises, and a heavy defeat was written in the stars as China’s short-term energy consumption, as data showed, shrank by 15% (Li et al., 2020a). With ongoing epidemic prevention and control, there is no choice for energy businesses but to adapt themselves to the epidemic. The move concerns China’s economic stability and security, as well as the quality of daily life among the general public.
Corporate nature is one of factors affecting decision making of energy businesses on adaptive behavior. Companies of a different nature result in the difference in the adoption of adaptive behaviors. Further analysis of the difference in the criticality of the impact that key affecting factors have on companies of varied nature is of practical significance and theoretical value to unveil the relationship between different types of energy enterprises and the factors affecting their decision making on adaptive behavior.
On that basis and with a survey of 500 energy enterprises based in 17 prefecture-level cities and autonomous prefecture in Hubei, the paper examined the types of adaptive behavior that these companies had chosen to respond to COVID-19 and by adopting the EWM, identified key factors that affect how these companies made decisions on adaptive behavior. Subsequently, the paper compared these samples with state-owned enterprises and with private businesses in terms of the criticality of factors affecting their decisions on adaptive behavior, with an aim to offer Chinese energy enterprises policy suggestions and a guideline on their sustainability under pressure from the epidemic.
Overview of the Study Area and Data Sources
Overview of the Study Area
Hubei was the hardest-hit area by COVID-19, and its capital city Wuhan had been locked down starting from 10 a.m. on January 23, 2020. The 24 h closed-off management was put in place in villages, communities, and residential communities and areas across the province in a way never seen before. It was such a determined response that stopped the virus from spreading nationwide.
Epidemic prevention and control forced Hubei’s energy enterprises to suspend their all or partial operations, and as a result, their revenue took a heavy toll. In 2020, among five Wuhan-registered companies listed in China A stock exchange, only Hubei Energy Group saw its first-quarter revenue flat with the level seen during the same period of the previous year due to an increase in power generation. The remaining four, namely SINOPEC Oilfield Equipment Corporation, Bestsun Energy, Kaidi Ecological and Environmental Technology, and Guodian Changyuan Electric Power, posted a year-on-year decrease in first-quarter revenue of 4.72, 23.24, 27.85, and 40.58%, respectively. That, compounded by sharp declines in business performance, threw them into a crisis of delisting from the stock market. While being active in responding to the changing epidemic by launching contingency plans, Hubei’s energy companies worked to prevent and control the spread of the disease and maintained production and other operations. All these efforts provided a solid foundation in the province’s ultimate victory over the fight against COVID-19 and in rapid economic recovery and social stability.
At the outset of the epidemic, Hubei-based energy enterprises owned by the Central Government promptly mobilized personnel and material resources to support the building of such large hospitals as Huoshenshan and Leishenshan, donated a large portion of funds to frontline virus control, and prepared emergency medical supplies, including protective suits, isolation gowns, goggles, and medical N95 masks. Some even leveraged their edges to turn to produce medical consumables, such as rubbing alcohol and raw materials for masks, in a way to meet the demand of the public for anti-epidemic supplies and contribute to Hubei’s battle against the virus.
Data Sources
Sample data came from a large-scale questionnaire survey in Hubei that was carried out by a research group at Taiyuan University of Technology on “Advancing Energy Production and Consumption Revolution (2035)” between February and May 2020. Up to 500 energy companies in Hubei’s 17 prefecture-level cities and autonomous prefecture were surveyed online (Tencent QQ and WeChat) or via phone. The focus of the questionnaire was four-fold. First, energy businesses’ perception of the trends and impacts of epidemic developments. Wherein the former involved how they perceived the possibility of epidemic recurrences in the province, the coming of coronavirus variants, and the global trend of COVID-19 developments; the latter included their perception of the energy production and supply, consumer demand, and survival of industrial chains in Hubei, as well as of global economic recession and vaccine development progress. Second, adaptive behaviors of energy companies. The survey used open-ended and closed-end questions to document the adaptive behaviors of respondents, and in so doing, prepared a summary of how these companies acted to adapt to the epidemic. Third, factors that affect the decision making of energy companies on adaptive behavior. Respondents were asked to evaluate the criticality of affecting factors from the perspectives of economic benefits, social benefits, maneuver decision making, the efficiency and stability of decision making. Fourth, the nature of enterprises, by which companies can be either state-run or private and engage in operations of conventional or new energy sources.
The survey was conducted in two stages. In Stage One, which lasted from late February to early March, leaders of 230 energy enterprises were interviewed by phone and tape-recorded, and the answers respondents gave, upon the recording and replay debugging, made for the Survey on Adaptive Behaviors of Energy Enterprises amid COVID-19. The questionnaire is available at Supplementary File 1. Stage Two started in late april and ended in early May, during which 270 energy businesses were surveyed online. Among the 500 companies, state-owned enterprises and private businesses accounted for 67.3 and 32.7%. Moreover, conventional-energy and new-energy enterprises represented 71.6 and 28.4% of all respondents, respectively. The types of companies surveyed were consistent with those of all energy companies in Hubei, and that, therefore, met the stratified sampling requirement.
The study excluded the personal information of leaders of energy enterprises surveyed when they answered questions or filled out the questionnaire, which included the respondents’ basic information, the extent to which they perceived epidemic developments, the actions taken during the epidemic, and how they evaluated the criticality of affecting factors. Having examined the validity of survey questions, we removed 14 questionnaires that were illogically answered or short of data, and the rest 486, or 97.2%, were considered valid. Besides, having used the SPSS 24.0 software to test the reliability and validity of these 486 questionnaires, we found the Cronbach’s α coefficient of each factor ranged from 0.85 to 0.89, and the Kaiser-Meyer-Olkin (KMO) value was 0.879. That those values were greater than 0.7 suggested acceptable reliability and validity of the survey and satisfied the requirement of the study.
Adaptive Behavior Types of Energy Enterprises
Generally, the business of energy enterprises was disrupted by the raging epidemic. That means adapting to the epidemic and working to put operations back on track were a sure way for these companies to sustain. According to descriptive statistics from the questionnaires, we identified the types of adaptive behaviors adopted by Hubei-based energy enterprises (Figure 1). The data are available at Supplementary Data 1.
Philanthropic Behavior
When things were at their most serious, energy companies in Hubei were courageous to shoulder corporate social responsibility and showed support for the hard-hit areas by carrying out charitable activities. To adapt themselves to epidemic prevention and control, some donated funds and materials, some ensured the supply of energy sources, and others helped build hospitals for emergency and even turned to produce medical raw materials. Companies giving funds, donating materials, and guaranteeing energy supply accounted for 87.6, 83.4, and 82.3% of all respondents, respectively. Donation of funds helped close the capital gap that authorities concerned confronted in sourcing protective materials. Purchasing medical masks, protective suits, and infrared thermometers before giving them to hospitals relieved the huge pressure from shortages of protective materials. The resumption of work, beyond the lowering of energy prices, ensured the massive support for virus control and economic recovery. Moreover, 46.7% of businesses surveyed mobilized a huge amount of people and materials to build such emergency hospitals as Leishenshan, Huoshenshan, and temporary treatment centers; 41.5% of respondents were dedicated to the production of medical raw materials in ways that ensured the supply of nonwoven fabrics, disinfectants, and medical oxygen. All these measures essentially strengthened companies’ sense of social responsibility and made them all the more resilient in the face of the disease.
Overall, Hubei-based energy companies would take the initiative to respond to the abrupt epidemic challenges as a way to carry social responsibility. Compared to the private sector, state-owned companies, particularly those run by the Central Government, were more likely to adopt philanthropic behavior and engage in charitable endeavors.
Economic Behavior
It was a wise, realistic option for energy companies to make self-help efforts through a behavioral change economically, such as lowering the human cost, suspending partial operations, applying for tax cuts and exemptions, and turning to producing protective materials. Among all answerers, 93.8% opted to control their human cost, and 86.7% suspended part of their business. This way they could minimize losses so as to keep the essential business in normal, orderly operation. To lower the human cost, companies and their employees, through consultations, agreed on a pay adjustment and the schedule of rotating shifts. For unprofitable operations that require long-term investment, energy companies temporarily closed down some related plants or suspended the acceptance of orders, with an aim to respond to the epidemic while lightening the burden on capital. Moreover, China announced a host of tax reduction policies and exemption policies to compensate for those companies’ economic losses from COVID-19. There are 74.3% of energy companies saw their financial difficulties eased by applying for the lawful postponement of tax payments and for low-interest loans. With an edge over raw materials, few energy businesses turned to producing much-needed medical products, all of which were purchased by governments for storage according to the system and measures for purchasing and storage during an epidemic. This lowered the costs of those producers and increased business income, ensuring their economic benefits.
Technical Behavior
Those opting for remote work, digitalizing their equipment, and automation in manufacturing accounted for 94.3, 37.6, and 31.6%, respectively, of the respondents. Among them, 94.3% would hold video conferences, coordinate management, and process business online through such online office software as DingTalk, Tencent Meeting, and WeChat Work. While allowing these companies to better connect to their staff, such behavior maximized the application of enterprise resources and considerably drove work efficiency. Meantime, boosting the pace of equipment to go digital by various technical means was a science-based decision that energy businesses made to adapt themselves to the coronavirus control. With that, 37.6% of them integrated their operations with such technologies as 5G and blockchain, upgraded online monitoring systems, and made contactless inspections on energy production and transportation through the video surveillance system. Given that some conventional energy companies came to realize the necessity of improving manufacturing processes, 31.6% of those surveyed rebuilt their assembly workshops by adding automated production lines. This was how they reduced dependence on labor while enjoying a surge in quality control, productivity and stability.
Identification of Key Factors Affecting Decision Making on the Adaptive Behavior of Energy Enterprises
Framework Building of Affecting Factors
Neoclassical economists believe that profit maximization is a necessary behavioral assumption that dictates how firms make output and pricing decisions. As always, enterprises would measure and examine the cost and benefit of adaptive behavior in a precise manner before developing a science-based evaluation system for comparison. On that basis, they can make an informed decision on whether they should have a behavioral change or not and which aspects they should adopt new behavior (Ahmad, 2001; Moller, 2006). Within ownership determinism theory, enterprise behavior is subject to the structure of the ownership system, which also has an extensive impact on decision making of enterprise behavior, and for firms of a different nature, their behavioral pattern and orientation vary (Yu and Jiang, 2004). The more resources a company has, including labor, natural endowment, materials, financial strengths, and social capital, the more likely it is to adopt adaptive behavior (Rastogi, 2000; Ko et al., 2018). As a key indicator of core competitiveness, innovative capacity would make a company significantly resilient (Smit, 2015; Li et al., 2019), whereas leadership holds key to whether a decision on some adaptive behavior is appropriate or not (Ready and Peebles, 2015). Meantime, firms must be keenly aware that commitment to social responsibility enhances the legitimacy of their establishment in society and the adaptability of behavioral decision making, and ultimately gains respect and recognition from the general public (Wu et al., 2021; Málovics et al., 2006). During COVID-19, the perception of epidemic developments represents the barometer of how firms should make behavioral decisions to meet market changes (Jin, 2020). Current economic realities present businesses with an opportunity and also a challenge. Not only does the new landscape make an extensive and far-reaching impact on the way firms produce and operate, but changes the previous pattern of how they make decisions on adaptive behavior (Jbilou et al., 2007). For energy companies, favorable energy policies are a matter of critical concern because the prospect of the energy sector is linked to the planning for enterprise development and the industrial layout, both of which pave the way for decision making on the adaptive behavior of these firms (Nicolli and Vona, 2012; Rosenkranz et al., 2017) Given that uncertain coronavirus developments disrupted global energy demand, energy businesses faced increased stress in production and operation and were forced to make a choice about which adaptive behavior they should adopt (Fu and Shen, 2020; Ghiani et al., 2020). Against the backdrop, the CPC Central Committee put forth a general requirement on nationwide epidemic prevention and control, which involves ordering enterprises to adapt themselves to the health crisis (Hu, 2020). Governments at all levels provided policy support for businesses to better adapt to a new reality (Huang, 2020).
Building on neoclassical economics, ownership determinism theory, and corporate capital theory, scholars have explored the factors that affect the decision making on the adaptive behavior of enterprises by means of quantitative analysis, case study, and empirical research. Factors that have been identified include the cost-benefit comparison of adaptive behaviors, the nature, capital, innovative capacity, leadership, social responsibility, and epidemic developments perception of enterprises, the current economic landscape, energy policy orientation, the outlook of the energy industry, uncertainties presented by COVID-19, China’s general requirement on virus control, and support from governments. The 13 factors above constituted the framework of factors that have an impact on how energy firms make decisions on a behavioral change.
Calculation of Entropy Weight in Evaluation Criteria
Setting of Variables
The set of factors affecting decision making of Hubei-based energy companies on adaptive behavior
Selection of Evaluation Criteria
The factor set
Setting of the Reviews Set
A typical five-level Likert item was employed to scale responses and set up the reviews set V = { v1, v2, v3, v4, v5 } = {Severe Impact, Major Impact, Moderate Impact, Minor Impact, Insignificant Impact}, and its corresponding assignments set
Establishment of the Fuzzy Evaluation Matrix
To avoid distractions of uncertain elements in decision making and make measurement results and quantitative interpretation more reliable and science-based, for an evaluation matter that contains
wherein the assignment value of element
In this study, the final assignment value of element
Standardization (Nondimensionalization) of the Fuzzy Evaluation Matrix
The standardized treatment can eliminate the deviations among various characteristic variables to ensure the reliability of data. Hence, upon the treatment of raw data in the fuzzy evaluation matrix
wherein
Calculation of the Fuzzy Entropy Value
The entropy value suggests the dispersion of an indicator. For a fuzzy evaluation matter that contains
to calculate the fuzzy entropy (Han and Wang, 1998) according to evaluation criterion
In our case, where
Calculation of Entropy Weight
For the calculation of the entropy weight in evaluation criterion
On the basis of Eq. 2, we calculated the assignment value of entropy weight according to the set evaluation criteria, which are shown below.
Analysis of Affecting Factors in the Entropy Weight-Based Decision-Making Model
Solution to the Normalized Weighted Matrix
The weighted operation of the assignment value of entropy weight on standardized matrix
Establishment of Ideal Points and Negative Ideal Points
The ideal point and negative ideal point of the normalized weighted matrix
where
Hence, the ideal point
Calculation of Fuzzy Nearness
The column vector
to calculate the relative nearness between each evaluation object/affecting factor
where
Setting of the Membership Function.
As a key indicator and method for measuring the extent to which two fuzzy sets are similar to or near each other, nearness represents a fuzzy value that, more often than not, is used to optimize the index weight in the overall evaluation. According to the description of the significance and character of the double-base method, the criticality of evaluation object/affecting factor
Equation 5 was then used to describe criticality
Calculation of Key Impact
We assigned nearness
Upon the sequencing of criticality
Obtainment of the Key Affecting Factor Set
For the key affecting factor set
In
In this paper, the assignment value of
That suggests on the condition that
Comparative Analysis of Key Affecting Factors
As one of the factors affecting decision-making of energy businesses on adaptive behavior, corporate nature hinges upon their position in the social economy. Companies of a different nature have a varied preference for the business environment and policies, which results in the difference in adopting adaptive behaviors, and the perception of and attitude toward key affecting factors.
In light of the nature of respondents, the sample data was divided into data provided by state-owned companies and those by private businesses. The data are available at Supplementary Data 2. Upon analyzing the entropy weight-based decision-making model, the results suggested that when the confidence level
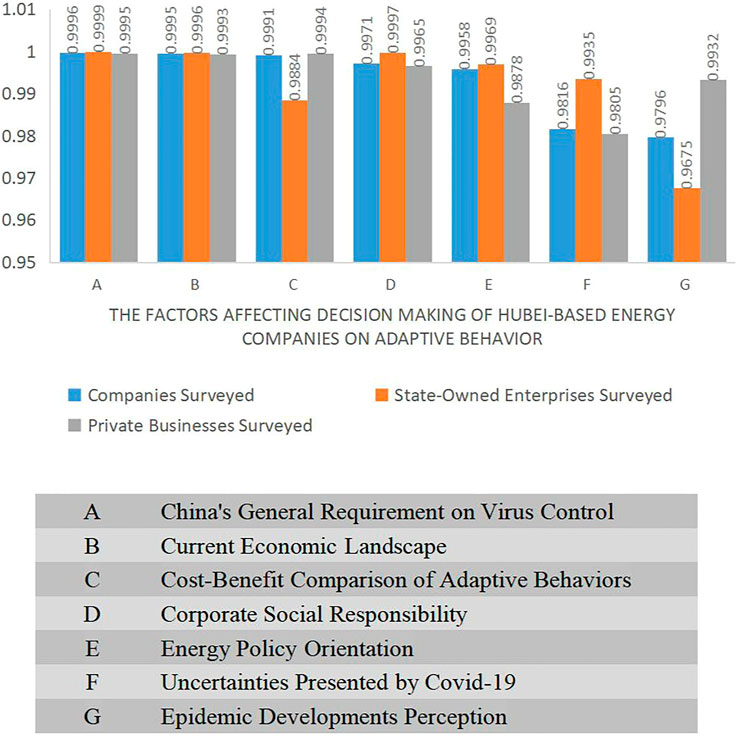
FIGURE 2. The comparison diagram on the criticality of key factors affecting decision making on the adaptive behaviors of all respondents, state-owned companies, and private businesses.
The distribution of criticality of key affecting factors in Figure 2 has two main features.
1) For all surveyed, including those of a different nature, China’s general requirement on virus control, current economic landscape, and corporate social responsibility are shared key affecting factors. Whatever type of companies is, China’s general requirement on virus control is regarded as the most critical factor that affects decision making of energy companies on adaptive behavior as it overtakes other affecting factors in criticality. For all respondents and private firms, the criticality of current economic realities is greater than that of corporate social responsibility, while the case with state-run companies is on the contrary.
2) The criticality of the cost-benefit comparison of adaptive behaviors ranks second among other key factors that have an impact on decision making of private companies on adaptive behavior, but still cannot constitute the key factor set of state-owned enterprises. On the contrary, energy policy orientation is not part of the private sector’s key factor set despite its fourth place in terms of the criticality of its impact on the behavioral decision-making of state-owned companies. Uncertainties presented by COVID-19 for state-run enterprises and epidemic developments perception by private firms are two critical factors excluded from the key factor set of all samples.
Policy-Making Recommendations
1) To encourage energy enterprises to make a behavioral change, local governments should work to understand and implement the general requirement on nationwide corona virus control put forth by the CPC Central Committee and the State Council. That means they need to stay committed to epidemic prevention and control by shoring up the weak spots thereof and improving and carrying out anti-epidemic measures.
2) Governments should pick up the pace of the development of economic recovery plans and issue a large-scale package of economic stimulus. In so doing, they need to restart the economy in a quick manner by stimulating domestic consumption through a host of measures, such as the issuance of shopping coupons. Moreover, greater efforts should be made to optimize the structure of energy sources, step up investment in renewable energy, and accelerate the pace of energy infrastructure building. This is how governments improve the quality of China’s economic and social development.
3) Governments should provide energy companies that were hit hard by COVID-19 with necessary financial support or assistance, such as concessionary financing, tax reduction and exemption, postponement of bank loans, and granting of allowances. Such measures allow businesses to lower the cost of having behavioral change while increasing earnings thereof.
4) Government functions need to be transformed. Specifically, governments should improve the legal system for responding to emergencies of major concern, rebuild the model for administering the society, and step up publicity and supervision. Beyond that, Chinese energy companies should be motivated to participate in the timely response and handling of public health emergencies as a way to strengthen their sense of social responsibility.
5) While concentrating on current coronavirus control realities and global economic developments, governments should continue to give priority to energy security and be oriented to green development in formulating policies. And more efforts need to be made to carve out a novel development model and optimize the structure of energy supply. On top of reducing the excess capacity of coal and coal power, governments should build a diverse energy supply system by launching various programs on renewable energy sources, including solar energy, wind power, biomass energy, and geothermal power. When it comes to policy development, the difference in regions, industries, and technological and economic conditions should be taken into account, so as to render policy resilient and effective.
6) On state-owned energy enterprises, governments should adopt proactive fiscal policy and moderately loosened monetary policy to give certainties to and share risks with companies as they pursue sustainable development. On top of that, holistic efforts should be made to drive economic growth and consolidate virus control by businesses. This way uncertainties brought by COVID-19 can be coped with by targeted policies, and businesses will thus be more confident about the economic rebound plan. As to private energy firms, governments need to put in place online platforms for government-enterprize interaction, on which businesses can be kept informed about updates on COVID-19 so as to help them better perceive epidemic developments.
Conclusion
With a phone-based or online (Tencent QQ and WeChat) survey of 500 energy enterprises based in 17 prefecture-level cities and autonomous prefecture in Hubei Province, the paper examined the types of adaptive behavior these companies had chosen to respond to COVID-19 and by using the EWM, identified key factors that have an impact on how these businesses made decisions on adaptive behavior. Building on that, it compared the samples with state-owned enterprises and with private businesses in terms of the criticality of factors affecting their decisions on adaptive behavior. The major findings are as follows.
1) The types of adaptive behavior that Hubei-based energy companies chose consisted of philanthropic behavior (including the donation of anti-epidemic funds and materials, guarantee of energy supply, support for the building of emergency hospitals, and production of medical raw materials), economic behavior (including human cost control, suspension of partial business, application for tax reductions and exemptions, and production of protective materials), and technical behavior (including remote work, equipment going digital, and automation in manufacturing).
2) On the condition that, the critical factors that affect the decision making of Hubei’s energy enterprises on adaptive behavior were China’s general requirement on virus control, the current economic landscape, the cost-benefit comparison of adaptive behaviors, corporate social responsibility, and energy policy orientation, with the first two taking the lead among others. Moreover, profit maximization is what companies are eager to achieve in business production and operation, and an essential criterion for profitability is the cost-benefit comparison of businesses’ adaptive behavior, which also drives their decision making on such behavior. Meantime, energy businesses followed government orders to contain the virus by quickly resuming production and ensuring the supply of protective materials as a way to show their social responsibility. Fundamentally, all these drove their decision making on adaptive behavior. Energy policy orientation, however, has a primary, guiding, or even decisive role to play. It can either drive the growth of energy businesses forward or slow it down, while exerting a direct, significant impact on decision making on their adaptive behavior.
3) When, the key factors affecting the decision making of all respondents and companies of a different nature on adaptive behavior varied. Indeed, the nature of energy companies held sway over their understanding of critical affecting factors, whose criticality would change accordingly.
Analyzing the decisions of energy enterprises on adaptive behaviors amid the COVID-19 pandemic goes a long way toward economic and social stability and successful containment of the pandemic. Thus, this study probed into the decision-making of energy enterprises on adaptive behaviors amid the pressure of the COVID-19 pandemic and achieved conclusions of both theoretical and practical significance. However, as the impacts of the COVID-19 pandemic are manifold, the adaptive-behavior decisions of energy enterprises are diverse, and the authors have limited expertize in this regard, the research shows some limitations, and some questions remain to be further explored. ①The contributing factors to the decision-making of energy enterprises on adaptive behaviors are manifold. The framework of contributing factors established in the present work is not complete. Many factors concerning the spread of the COVID-19 virus have not been incorporated into the framework, limiting the universality of the research on the identification of the key contributing factors. ②Though the criticality of contributing factors has been identified, the influencing mechanism of the key contributing factors to the adaptive behavior decision-making of energy enterprises has not been revealed, and the specific impact of the COVID-19 pandemic on the enterprises’ decision-making has not been analyzed in detail. Future studies will probe into the influencing mechanism of the COVID-19 pandemic on the decision-making of energy enterprises on their adaptive behaviors and the impact of the spreading characteristics of the COVID-19 virus on differentiated choices of enterprises.
Data Availability Statement
The original contributions presented in the study are included in the article/Supplementary Material, further inquiries can be directed to the corresponding author.
Author Contributions
XY: methodology; investigation; data curation; writing—original draft preparation; writing—review and editing. JL: project administration; funding acquisition. All authors approved the final version to be published.
Funding
This research was funded by the Major Consulting Project of Chinese Academy of Engineering “Promoting the Revolution of Energy Production and Consumption (2035) -- Strategic Research on Energy Revolution Promoting Economic and Social Development and Ecological Environment Protection” (2018-ZD-11), the 2018 Shanxi Philosophy and Social Science Planning Project (Project No: 2019B085).
Conflict of Interest
The authors declare that the research was conducted in the absence of any commercial or financial relationships that could be construed as a potential conflict of interest.
Supplementary Material
The Supplementary Material for this article can be found online at: https://www.frontiersin.org/articles/10.3389/fenrg.2021.716171/full#supplementary-material
Supplementary File 1 | The survey on adaptive behaviors of energy enterprises amid COVID-19.Supplementary Data 1 | The types of adaptive behaviors adopted by Hubei-based energy enterprises.Supplementary Data 2 | The evaluation assignment value (mean value) of the criticality of affecting factors by energy enterprises.References
Abayomi, A., Katz, R., and Spence, S. (2019). Managing Dangerous Pathogens: Challenges in the Wake of the Recent West African Ebola Outbreak[J]. Glob. security Health Sci. Pol. 1 (1), 51–57. doi:10.1080/23779497.2016.1228431
Ahmad, R., and Buttle, F. (2001). Customer Retention: a Potentially Potent Marketing Management Strategy. J. Strateg. marketing 9 (1), 29–45. doi:10.1080/713775729
Angel, J. L., and Mudrazija, S. (2020). Local Government Efforts to Mitigate the Novel Coronavirus Pandemic Among Older Adults. J. Aging Soc. Pol. 32 (4-5), 439–449. doi:10.1080/08959420.2020.1771240
Brenton, P., and Chemutai, V. (2020). Trade Responses to the COVID-19 Crisis in Africa[M]. Washington, DC: World Bank.
Carter, S. E., O’Reilly, M., and Frith-Powell, J. (2017). Treatment Seeking and Ebola Community Care Centers in Sierra Leone: A Qualitative Study[J]. J. Health Commun. 22 (1), 66–71. doi:10.1080/10810730.2016.1216204
Chen, L., and Qiu, W. (2003). The Fuzzy Analysis in View of Entropy Coefficient for Influence Factor of Large Scale Project Decision Making[J]. Forecasting 22 (3), 65–67.
Fu, M., and Shen, H. (2020). COVID-19 and Corporate Performance in the Energy Industry[J]. Energ. Res. Lett. 1 (1), 12967. doi:10.46557/001c.12967
Gatiso, T. T., Ordaz-Németh, I., Grimes, T., Lormie, M., Clement, T., Kühl, H. S., et al. (2018). The Impact of the Ebola Virus Disease (EVD) Epidemic on Agricultural Production and Livelihoods in Liberia[J]. PLOS Negl. Trop. Dis. 12 (8). doi:10.1371/journal.pntd.0006580
Ghiani, E., Galici, M., Mureddu, M., and Pilo, F. (2020). Impact on Electricity Consumption and Market Pricing of Energy and Ancillary Services during Pandemic of COVID-19 in Italy. Energies 13, 3357. doi:10.3390/en13133357
Guo, Y., and Jia, J. (1990). Comprehensive Evaluation and Order[J]. Syst. Eng. Theor. Pract. 10 (2), 26–30.
Han, L., and Wang, P. (1998). Application of Fuzzy Mathematics[M]. Beijing, China: Capital University of Economics and Business Press, 11–25.
Hu, Y., Wang, J., and Dong, Z. (2020). Analysis on Decision for Enterprises to Resume Work during COVID-19 Epidemic Prevention and Control: Based on Behavioral Economics[J]. Stat. Decis. 36 (5), 157–160. doi:10.13546/j.cnki.tjyjc.2020.05.035
Huang, S., Lv, P., and Fan, X. (2020). How Does the Epidemic Affect Corporate Development Expectations? — Empirical Research Based on Pressure Transmission Mechanism[J]. Public Finance Res. (4), 44–57+65. doi:10.19477/j.cnki.11-1077/f.2020.04.006
Jbilou, J., Amara, N., and Landry, R. (2007). Research-Based-Decision-Making in Canadian Health Organizations: A Behavioural Approach. J. Med. Syst. 31 (3), 185–196. doi:10.1007/s10916-007-9054-3
Jin, B. (2020). On Promises of a Paradigm for Economics of Economic Subject Behavior — Thinking about the COVID-19 Epidemic[J] (02), 1–8+198.
Kim, S. J., Han, J. A., Lee, T.-Y., Hwang, T.-Y., Kwon, K.-S., Park, K. S., et al. (2014). Community-based Risk Communication Survey: Risk Prevention Behaviors in Communities during the H1N1 Crisis, 2010. Osong Public Health Res. Perspect. 5 (1), 9–19. doi:10.1016/j.phrp.2013.12.001
Ko, S.-H., Choi, Y., Rhee, S.-Y., and Moon, T. (2018). Social Capital and Organizational Citizenship Behavior: Double-Mediation of Emotional Regulation and Job Engagement. Sustainability 10, 3600. doi:10.3390/su10103600
Leung, T. T. F., and WonG, H. (2005). Community Reactions to the SARS Crisis in Hong Kong. J. Hum. Behav. Soc. Environ. 12 (1), 1–22. doi:10.1300/j137v12n01_01
Li, G., Wang, X., Su, S., and Su, Y. (2019). How green Technological Innovation Ability Influences enterprise Competitiveness[J]. Technology in Society. 2019, 59. doi:10.1016/j.techsoc.2019.04.012
Li, J., Zhang, C., and Weng, Y. (2020a). Countries Should Make Great Efforts to Develop Renewable Energy amid COVID-19[J]. People’s Weekly (11), 54–55.
Li, S., Wang, Y., Jia, X., Zhao, N., and Zhu, T. (2020b). The Impact of COVID-19 Epidemic Declaration on Psychological Consequences: A Study on Active Weibo Users[J]. Int. J. Environ. Res. Public Health 17 (6), 2032. doi:10.3390/ijerph17062032
Liu, S., and Qiu, W. (1998). Generalization for the Double Base Points Ordering Method for MADM[J]. Syst. Eng. — Theor. Pract. 18 (2), 22–25.
Main, A., Zhou, Q., Ma, Y., Luecken, L. J., and Liu, X. (2011). Relations of SARS-Related Stressors and Coping to Chinese College Students' Psychological Adjustment during the 2003 Beijing SARS Epidemic. J. Couns. Psychol. 58 (3), 410–423. doi:10.1037/a0023632
Málovics, G., Noémi Nagypál, C., and Kraus, S. (2006). The Role of Corporate Social Responsibility in strong Sustainability[J]. J. Socio-Economics 37 (3). doi:10.1016/j.socec.2006.12.061
Möller, K. (2006). Role of Competences in Creating Customer Value: A Value-Creation Logic Approach. Ind. marketing Manag. 35 (8), 913–924. doi:10.1016/j.indmarman.2006.04.005
Nicolli, F., and Vona, F. (2012). The Evolution of Renewable Energy Policy in OECD Countries: Aggregate Indicators and Determinants[J]. SSRN Electron. J. 234 (6), 120–141.
Nigmatulina, K. R., and Larson, R. C. (2009). Living with Influenza: Impacts of Government Imposed and Voluntarily Selected Interventions. Eur. J. Oper. Res. 195 (2), 613–627. doi:10.1016/j.ejor.2008.02.016
Obol, J. H., Akera, P., and Ochola, P. A. (2018). Community-based Training of Medical Students Is Associated with Malaria Prevention and Treatment Seeking Behaviour for Children under 5years in Uganda: a Study of MESAU-MEPI COBERS in Uganda[J]. BMC Med. Educ. 18 (1), 131. doi:10.1186/s12909-018-1250-y
Pak, A., Adegboye, O. A., Adekunle, A. I., Rahman, K. M., McBryde, E. S., and Eisen, D. P. (2020). Economic Consequences of the COVID-19 Outbreak: the Need for Epidemic Preparedness. Front. Public Health 8, 241. doi:10.3389/fpubh.2020.00241
Rastogi, P. N. (2000). Sustaining enterprise Competitiveness - Is Human Capital the Answer? Hsm 19 (3), 193–203. doi:10.3233/hsm-2000-19306
Ready, D., and Peebles, M. E. (2015). Developing the Next Generation of enterprise Leaders[J]. MIT Sloan Manag. Rev. 57 (1), 43.
Reissman, D. B., Watson, P. J., Klomp, R. W., Tanielian, T. L., and Prior, S. D. (2011). Pandemic Influenza Preparedness: Adaptive Responses to an Evolving Challenge[J]. J. Homeland Security Emerg. Manag. 3 (2). doi:10.2202/1547-7355.1233
Rosenkranz, S., Vringer, K., Dirkmaat, T., van den Broek, E., Abeelen, C., and Travaille, A. (2017). Using Behavioral Insights to Make Firms More Energy Efficient: A Field experiment on the Effects of Improved Communication. Energy policy 108 (9), 184–193. doi:10.1016/j.enpol.2017.05.056
Samaraweera, H. U. S. (2018). Coping Strategies Identified and Used by Victims of Flood Disaster in Kolonnawa area:An Analysis from a Social Work Perspective [J]. Proced. Eng. 212, 675–682. doi:10.1016/j.proeng.2018.01.087
Shretta, R., Zelman, B., and Birger, M. L. (2017). Tracking Development Assistance and Government Health Expenditures for 35 Malaria-Eliminating countries:1990–2017[J]. Malar. J. 16 (1), 251. doi:10.1186/s12936-017-1890-0
Smit, J. (2015). The Innovation Value Chain and Adaptability of Organizations[J]. J. Int. Tech. Inf. Manag. 24 (3), 57–73.
Wu, X., Dluhošová, D., and Zmeškal, Z. (2021). Corporate Social Responsibility and Profitability: The Moderating Role of Firm Type in Chinese Appliance Listed Companies. Energies 14, 227. doi:10.3390/en14010227
Yu, L., and Jiang, C. (2004). A Comparison of Business Behavior and Social Welfare under Different Proprietorships [J]. Res. Econ. Manag. (6), 11–15.
Zhang, X., and Han, Y. (2020). Impact of COVID-19 on Small and Medium Sized Cross-Border E-Commerce Enterprises and Suggestions[J]. Int. J. Front. Eng. Tech. 2 (2), 71–80. doi:10.25236/IJFET.2020.020207
Zhang, Y., Zou, S., and Su, F. (1992). Fuzzy Mathematics Method and its Application[M]. Beijing, China: China Coal Industry Publishing House, 55-85. The following formatting styles are meant as a guide, as long as the full citation is complete and clear, Frontiers referencing style will be applied during typesetting
Keywords: COVID-19, epidemic, adaptive behavior, decision making, entropy weight method (EWM)
Citation: Yang X and Li J (2021) Analysis of Decision Making of Energy Enterprises on Adaptive Behavior Amid COVID-19. Front. Energy Res. 9:716171. doi: 10.3389/fenrg.2021.716171
Received: 28 May 2021; Accepted: 29 June 2021;
Published: 15 July 2021.
Edited by:
Xingxing Zhang, Dalarna University, SwedenReviewed by:
Zhanyou Sa, Qingdao University of Technology, ChinaXiangchun Li, China University of Mining and Technology, China
Copyright © 2021 Yang and Li. This is an open-access article distributed under the terms of the Creative Commons Attribution License (CC BY). The use, distribution or reproduction in other forums is permitted, provided the original author(s) and the copyright owner(s) are credited and that the original publication in this journal is cited, in accordance with accepted academic practice. No use, distribution or reproduction is permitted which does not comply with these terms.
*Correspondence: Jizu Li, bGlqaXp1QHR5dXQuZWR1LmNu