- 1Department of International Affairs, College of Arts and Sciences, Qatar University, Doha, Qatar
- 2Civil Engineering and Environmental Technology, Technical University of Cologne, Köln, Germany
Building energy consumption can be enhanced to a certain level, but human-oriented dimensions can open the doors to new opportunities. This article presents an assessment on the interactions between human-driven factors and energy consumption patterns in office buildings based on empirical evidence and a co-simulation. The objective of this work is to evaluate a range for saving capacities as a result of readjusting occupants’ prior habits and engaging them in building operation by determining internal and external factors associated with human-building drivers, needs, actions, and systems. The study is based on a real office building, and according to real behavioral data and subjective measurement to analyze human attitudes and interactions with the building end-uses such as cooling, lighting, and equipment along with factors that directly impact energy use such as fenestrations and window blinds. The survey results are extensively investigated and the correlations between demographic/socioeconomic traits and behavioral factors are examined. The survey results are incorporated into a co-simulation testbed to represent occupant attitudes and behaviors and model human-building interactions accordingly. Different scenarios are designed to perform an assessment on the role of human factors such as attitude, awareness of consequences, habits, ascription of responsibility and personal/social norms on building energy end-uses. The outcomes of this study demonstrate that by adjusting behavioral factors in an office building, an energy saving between 9 and 18% can be achieved without sophisticated technology interface or building retrofit/upgrade. The results are elaborated, and recommendations are explained in detail.
Introduction
Energy demand in residential and commercial building sectors accounts for about 20% of the total global energy consumption (U. Energy Information Administration, 2019). This value is estimated to increase about 100% by 2050, which demonstrates the importance of this sector in the power and energy industry and, in turn, future environmental, economic, and social sustainability challenges (I. Renewable Energy Agency, 2018). This necessitates the need to recognize novel multidisciplinary perspectives to curb the building sector’s demand and leverage any interventions and strategies to pave the road to achieve sustainability targets. For the past few decades, the state-of-the-art technologies concerning building energy efficiency, automation, and ICT have enabled opportunities that are feasible and within reach, but efficacy gaps in the field are still acute and cannot be denied (Holzmann and Schmid, 2018). To ensure closing the performance gaps, it is suggested that a proper understating and characterization of elements associated with human dimensions can offer new a new set of alternatives and ease of flexibility (Deuble and de Dear, 2012). This emanates from the dominant influence of human–building interactions (HBI) in built environments through active and passive activities along with diverse and stochastic behavioral drivers among building users (Jia et al., 2017). The integration of human and social dimensions in built environments is reflected in a wide range of applications in pure and applied social science energy research (SSER) (Stern, 2017).
Human behavioral drivers vary significantly by social and demographic factors. Delineating these factors requires a solid definition of behavioral models through applied social science investigations (Stern et al., 2016), empirical evidence, and subjective measurements to acquire proper insight to incorporate the role of human in the system (Rinaldi et al., 2018). In general, the sole understanding of human factors would not suffice and must be accompanied by proactive interventions for human inclusion. From one perspective, such interventions can be categorized based on Behavioral Change Wheels (BCW) as a system with three main interacting layers, namely sources of behavior, intervention functions, and policy categories (Michie et al., 2011). Sources of behavior remains at the core of this architecture and consists of three main categories of 1) capability, which is defined as the need, physical and psychological, of the individual to have the necessary knowledge and set of skills to get engaged in activities, 2) motivation, which is in accordance with cognitive processes that trigger certain actions, and 3) opportunity, which is all the surrounding factors that influence behavior by the means of social or physical factors. The outer layer of the wheel, concerning intervention functions, consists of nine main elements of education, persuasion, incentivization, coercion, training, restriction, environmental restructuring, modeling, and enablement.
It is well-established that education and awareness can positively influence pro-environmental awareness (Allen et al., 2013) and create a baseline for social norms in building energy conservation and beliefs among different communities and generations (Cialdini, 2003). Awareness regarding environmental concerns along with social values, norms, human attitudes, and intentions should be reflected in the behavior of energy consumers through long- and short-term policies (Frederiks et al., 2015). The crucial factor to changing energy consumption patterns associated with human factors is to provide opportunities for the consumer to acquire awareness through education about energy conservation and environmental issues (Cotton et al., 2016). For instance, social awareness regarding environmental consequences and health issues associated with fossil fuels were evaluated in Qatar, and education and information were determined as an effective factor in improving human attitudes and social norms (Al-Marri et al., 2018). In another study by Pothitou et al., the association between domestic energy conservation and environmental knowledge was assessed, and a positive correlation between environmental values, environmental knowledge, and energy conservation was observed (Pothitou et al., 2016). Based on self-determination theory, Al-Marri et al. demonstrated that once an individual is aware of the environmental risk associated with energy consumption, they are more likely to be cautious about environmental values and, consequently, energy conservation (Al-Marri et al., 2018). Similar outcomes were reported by Mohamed et al. in a survey study in Libya. The effects of domestic energy use and householders’ energy behavior on overall energy consumption were evaluated among 429 households (Mohamed et al., 2015). The results reported a lack of knowledge, awareness, or interest in relation to energy-saving measures and energy efficiency among the sample. However, it was demonstrated that minor changes in behavioral factors, social awareness, and social norms could result in an energy consumption reduction of about 2 gigawatts (GW). Empirical assessments show that curriculum-based learning can also act as a low-cost intervention to achieve energy savings and enhance energy behaviors in private and public environments (Craig and Allen, 2015).
Moreover, although having overlaps with other interventions, persuasion has also been recognized as a major practice to convey positive perceptions and emotions to individuals towards energy conservation in workplaces (Staddon et al., 2016). A proper and purposeful representation of information will improve the human’s motivations, attitudes, and actions (Simcock et al., 2014), and can help to achieve considerable amounts of savings through the use of technological and non-technological persuasion interventions (Schelly et al., 2010).
Further, incentives can be regarded as an intervention with the goal of establishing new behavioral norms with respect to building energy conservation and can be allocated based on monetary or social rewards. Such strategies can potentially engage building occupants in the operation of the buildings to enhance energy efficiency, waste reduction, and participation (Gustafson and Longland, 2008a). Although the efficacy of incentive models is disputed; but in some studies, social rewards outperform financial rewards (Handgraaf et al., 2013). Among all the intervention strategies, coercive actions seem to be more ambiguous due to negative connotations arising from factors such as sense of punishment or mortification (Wright et al., 2007), legal consequences, or possible adverse impacts on building users’ productivity and attitude (Staddon et al., 2016). Training interventions can also be implemented in public built environments and workplaces, especially in distributed and decentralized systems, where the users have more access to override the system and require instructions to better interact with devices and environment.
As another BCW element, environmental restructuring is referred as the process of changing the physical or social contexts to promote a particular behavior by leveraging interventions such as signs and posters, electronic feedback devices and online dashboards to provide real-time energy feedback, retrofitting technology and technology automation on lights and other electronic devices (Michie et al., 2014). For instance, significant energy savings are observed when real-time feedback gadgets are installed in office spaces (Murtagh et al., 2013), or when online dashboards are utilized to provide energy feedbacks (Yun, 2014) and integrated with automation systems (Yun et al., 2015). Besides, modeling interventions and inspiring building occupants have demonstrated noticeable influence on building energy consumption with the use of public recognition (Owen and Energy, 2010) or competition as a way to motivate occupants to improve their prior energy use habits (Dixon et al., 2015). Finally, enablement intervention is defined as offering energy saving opportunities by eliminating or reducing possible barriers, such as (Yun, 2014), where about 30% energy saving was achieved by enabling remote control of electronic devices, or (Gustafson and Longland, 2008b) with 12% savings through skilled staffs’ assistance to interact with the environment. All the aforementioned interventions can be a basis for a comprehensive definition of human-building interactions and relevant solutions to improve the energy performance of buildings.
Turner et al. developed an analytical framework to model the occupant-building interaction energy usage (Turner et al., 2014). The framework consisted of four main elements based on drivers, needs, actions, and systems, and each element was further categorized into two components: “outside world” (i.e., the building environment) and “inside word” (i.e., the cognitive processes of human being). In this framework, drivers are associated with external factors (i.e., environmental factors) that force the occupant to take an action or perform interactions with the indoor environment with possible impacts on building energy consumption patterns. Needs represent that the criteria or expectations of the occupant’s personal perception be met in order to ensure their satisfaction and comfort with their environment (physical and non-physical). Actions are the activities and interactions with systems that the occupant follows to restore their personal comfort in relation to the outside world. Systems refers to anything inside the building that the occupant can interact with to condemn environmental comfort. In order to achieve consistent human–building interactions, opportunity and ability should be established within the built environment. Most of the discrepancy issues in human–building interactions can be avoided by keeping an eye on factors like oversimplifying or ignoring human behavior in the building design and operation process, defected interfaces between human behavior and the building system, and unreliable technology and system controls (Oca and William, 2015). However, research studies are raising skepticism in behavioral factor modeling and human–building interaction analysis due to the stochastic nature of human perceptions and decisions (Tabak and de Vries, 2010). Thus, the human behavior models should go beyond social and psychological analysis and be supported by the proper amount of data to resolve the uncertainty issues in the analysis. Although understanding the human drivers in building environment interactions and consumption patterns is important, the well-being of occupants, including thermal and visual comfort, cannot be ignored.
The goal of every building operation and control strategy is to maintain an optimal balance between the user’s indoor comfort level and energy consumption. This operation can be called a success when at least 80% of the occupants feel within their perceived comfort zone (Ansi/Ashrae, 2004). But any discomfort can cause interruptions in the building operation. Humphreys’s principle says: “if a change occurs such as to produce discomfort, people react in ways which tend to restore their comfort” (Nicol and Humphreys, 2002). Any discomfort can cause adjustment and adaptive actions that can be categorized into behavioral adjustments, psychological expectations and prior habituations, and acclimatization and adaptation (Brager and de Dear, 1998). Based on the occupants’ needs, they demonstrate adaptive behaviors to reconcile or achieve their comfort expectations, such as changing their surroundings (e.g., opening/closing windows, lowering blinds, adjusting thermostats, and interacting with the lights) or changing themselves (different clothing or moving to another space to find comfort) to get adapted to indoor environment variations (de Dear and Brager, 1998). The aforementioned interactions can dramatically change energy consumption profile of a building.
To this end, technology-driven solutions and smart operation scheduling of building environments must be accompanied by human interaction considerations. Human interactions and human-oriented processes can be integrated into building operation scheduling and as a part of the decision-making framework, such as occupancy patterns, productivity levels, and indoor environment comfort. Additionally, human interactions, such as control override, can adversely interrupt desirable building operation. Human dimensions vary by regional and demographic factors and need detailed investigations. Such human factors are not only crucial in operation planning but also important in policy planning at higher levels. Since human and social dimensions are complex phenomenon, they have to be fully scrutinized for different regions and demographics. To the best of our knowledge, there is still inadequate literature to investigate a technical analysis of human–building interactions reflecting factors such as awareness, norms, prior habits, responsibility factors, demographic, and socioeconomic to measure the influence of occupancy drivers on the energy demand profile of commercial buildings and potential savings as a result of behavioral adjustments on saving energy (Nazemi et al., 2021). Besides, knowing the fact that experimental assessments and onsite measurements are prone to error and biased as well as flexibility issues with regard to sensitivity analysis of preferable end-uses and indoor environment adjustments, there is still a lack in terms of adequate simulation analysis on this matter.
In this work, we present a comprehensive, human–building investigation based on authentic psychological/social factors and validated building simulations to demonstrate energy-saving opportunities through improving and establishing new personal attitudes and consumption habits. The goal is to determine the associations between human-oriented dimensions, such as demographic and socioeconomic factors, with human-building interactions and habits and to understand the extent to which that non-technological aspects and human behavior adjustments can reduce building energy consumption. The investigation starts with a survey study to understand habitual behaviors of the occupants and human-building interactions in an office building in Doha, Qatar. The survey results are comprehensively presented, and the variable dependencies, such as demographics, socioeconomic, and psychological factors, are determined. Important factors such as human awareness of environmental consequences, perceived responsibility, personal norms, social norms, habits, behavioral control, and attitude are investigated and the intercorrelations are identified. Concerns such as the main drivers for building operation override is demonstrated. A building simulation is developed, occupancy patterns are simulated via Non-homogenous Markov Chain models, and human dimensions, including habits, preferences, and interactions, are modeled and incorporated into the building simulation through a co-simulation testbed. Five different case scenarios are designed and compared with the actual base scenario and extreme consumption cases to demonstrate the influence of engagement of occupants in building operation and energy consumption with respect to thermostat override, windows and blinds interactions, and lighting/equipment system usage. The case studies exhibit potential savings ranging from 9% (the exiting condition of the building and human behaviors) to 18% (at an extreme point, where all occupants demonstrate adverse habits) when building occupants adjust their consumption habits in interacting with building energy end-uses. Finally, a set of recommendations to improve behavioral norms in office buildings is presented and solutions are discussed.
Problem Statement and Methodology
Qatar is one of the fastest growing economies in the world with a high GDP per capita. Qatar has been seeking futuristic plans and new strategies to support its economy through aggressive targets in modernization and industrialization plans. However, achieving such targets presents a variety of challenges in sustainability and infrastructure preparedness, especially in energy transition. The growing demand of energy and environmental concerns has become a priority for policymakers in Qatar to ensure a reliable and effective master plan to achieve its long- and short-term (Zaidan et al., 2019; Zaidan et al., 2021) targets. Among all sectors, built environments are of paramount importance in energy policy due to their uncertain and growing trends in energy demand as a result of the boosting economy, demographic factors, and arid climate conditions. Energy-saving alternatives and solutions can curb the growing demand of energy and mitigate emissions and adverse environmental impacts in this regard. Energy efficiency practices and technology-driven alternatives such as advanced automation systems, ICT infrastructures, and smart solutions can help enhance future energy demand in the building sector; however, human factor and social dimensions must not be neglected in planning and decision-making in energy policy. Overlooking the insight that social sciences and human dimensions can add to energy policy and planning has been criticized in the literature (Labanca and Bertoldi, 2018).
There are always questions concerning the role of human behavior in building energy consumption. Intuitively, human interaction with buildings changes consumption patterns. However, the extent of this impact and the major factors in this regard are most of the time unknown. In this study, we implement a subjective measurement analysis and a framework (Figure 1) to determine the main human factors impacting daily building electricity consumption profiles and incorporate those factors in a simulation platform. This framework will assess the magnitude of each factor on building total electricity demand and identify solutions to mitigate the adverse effect of human override or interruption on a desirable building operation.
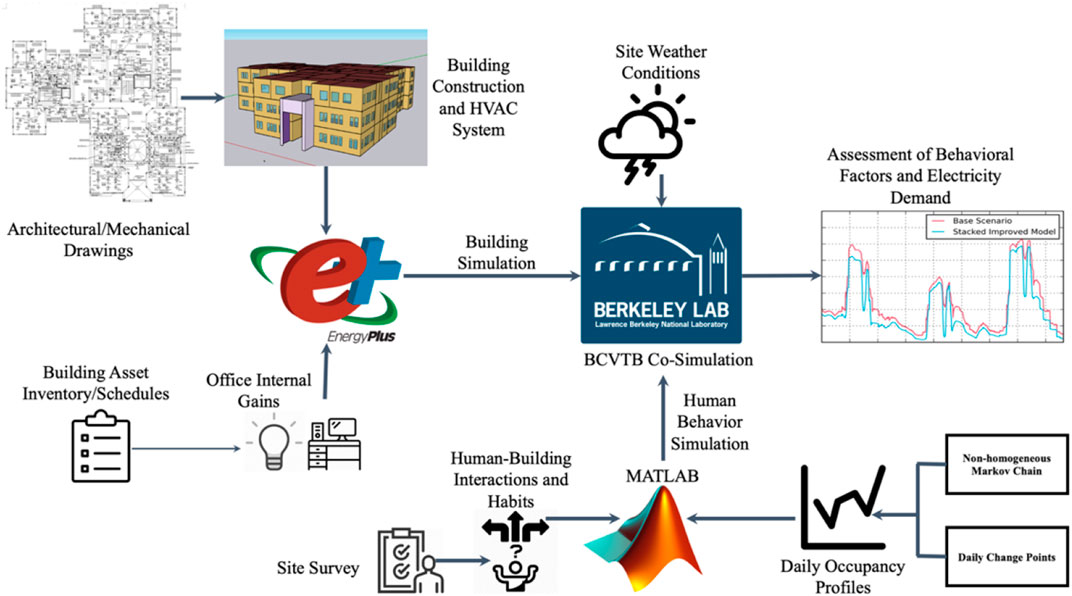
FIGURE 1. The framework that was implemented to assess human–building interactions and building electricity consumption under different case scenarios.
In this study, we target an office space in Doha, Qatar to evaluate the interactions between human factors and building operation based on real data to determine the extent to which building performance is influenced by occupants and how improving adverse behavioral norms and prior habits can enhance the energy efficiency of a building without any major structural and technological changes. An EnergyPlus (EnergyPlus Energy Simulation Software, 2014) building simulation is constructed with the use of the actual building’s mechanical and architectural drawings. The internal gains of the building, including lighting and equipment loads, are incorporated in the building simulation based on the actual schedules and equipment of the building. Besides, a survey study is conducted among 81 building users, and their self-perceived habits with regard to building interactions are enquired. Five main elements in building end-uses are considered that can be impacted by human override, and the survey acquires the information with this regard:
• Room thermostat
• Lighting system
• Fenestrations
• Window blinds and curtains
• Office equipment
The survey was designed to accurately reflect such behavioral attributes and conducted among all occupants in the office spaces. The self-perceived human habits are then used to construct discrete probability distribution functions to simulate human interactions with the above elements at individual office rooms and at different times of the day or week. Non-homogenous Markov Chain models are implemented to model binary occupancy profiles for each zone and combined with human-building interactions accordingly. The combined behavioral factors are simulated via MATLAB at each timestep of the simulation. Occupancy behavior and override simulation, building simulation, and weather conditions are linked to a co-simulation environment [Building Control Virtual Testbed (Wetter, 2012)] to create scenarios that represent the current state of the building operation and human behaviors (base scenario) along with sensitivity analysis to determine the crucial behavioral factors and how to establish new habitual norms to improve human–building interactions and building operation performance. Aside from the subjective measurement to determine the occupants’ habits and building interactions and incorporate their influence in the simulation analysis, any statistically significant associations between demographics and socioeconomic dimensions with personal traits such as human energy attitude, pro-environmental knowledge, and habits are analyzed based on empirical evidence and via Cramér’s phi (Bergsma, 2013) statistical association method.
Building Characteristics and Simulation Procedure
The target building in this study was a three-story administrative office space located in Doha municipality in Qatar. The total building area was 2,844 m2 with a net conditioned area of 2,786 m2. The building consisted of 145 zones with functionalities such as breakrooms, kitchenette, conference room, lobby, archive rooms, server rooms, mechanical/electrical room, storage space, cafeteria, and shared offices along with 74 closed office spaces. The building was constructed in the early 2000s and equipped with three air handler units operating with chilled water coils for cooling, and electric coils for possible heating. The air distribution system was a variable air volume system with controllable and non-reheat air terminals. The building construction followed ASHRAE 189.1 and 90.1 standards and ASHRAE climate zone 1B assumptions (Staff, 2018). The building geometry created by Openstudio software is shown in Figure 2.
In order to reflect the impact of an arid climate and the actual impact of weather conditions on the operation of the building simulation, TMY2 historical data (Typical Meteorological Year 2 [Typical Meteorological Year, 2007)] was implemented in the building model. The distribution of some of the weather parameters based on the historical data is shown in Figure 3.
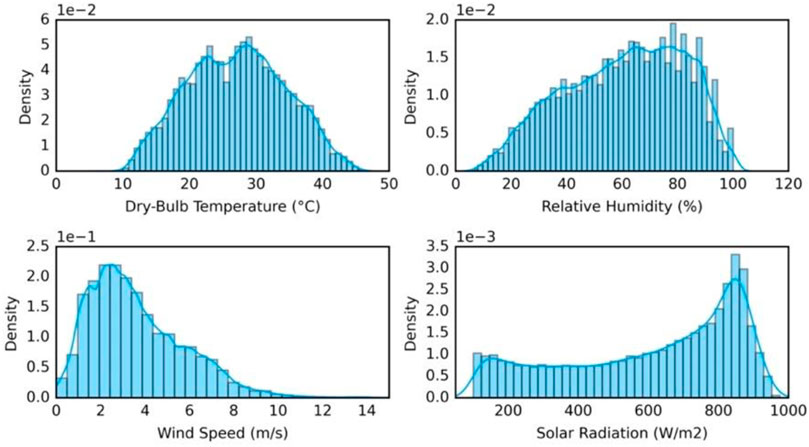
FIGURE 3. Frequency density of historical weather data representing dry-bulb temperature, relative humidity, wind speed, and solar radiation used in the building simulation.
The building simulation was developed using EnergyPlus, a tool developed by the US Department of Energy (2015). The internal loads associated with the lighting and office equipment and appliances represented the actual building loads and based on data acquisition of each individual office space. The simulation was based on the actual characteristics of the building and carried out for an 8,760-h time horizon with 5-min time resolution. The internal lighting and equipment information was collected through drawings, an asset inventory of the building, and equipment as reported by the occupants. The occupancy variation for each office space followed a binary form (occupied or unoccupied), and the number of occupants was neglected (Figure 4). The binary occupancy patterns (X) at time k were modeled via an Non-homogeneous Markov Chain (NHMC) according to the below equation (Li and Dong, 2018):
where S = {0,1} as a binary set of the occupancy states and
Due to the inhomogeneous behavior of the Markov Chain model, three different transition probability sets were considered for time intervals between arrival and lunch break, during lunch break, and between lunch break and departure, which were separated by daily change points. The office building operation in this study started at 7:30 AM and ended at 2:30 PM. It was assumed that besides short breaks, the users take a lunch break from 12 PM to 1 PM. The deterministic occupancy patterns for arrival time, lunch break start, lunch break end, and departure were adjusted based on a Gaussian distribution with a mean value based on the deterministic arrival, departure, and break times and a standard deviation of 10 min in order to add stochasticity in human–building interactions. The pattern follows,
where
In order to add stochasticity to the model input variables, a co-simulation of MATLAB and EnergyPlus, using Berkley sockets, an inter-process communication, was implemented to adjust the simulation inputs at each timestep. In this co-simulation, a MATLAB code generated the probabilistic inputs at each 5-min time interval, and the inputs were transferred to EnergyPlus environment to simulate the building thermal dynamics and human interactions. The building annual electricity consumption was 811 MWh and calibrated based on the building historical meter data. The annual electricity demand is shown in Figure 5. Figure 6 presents the main building end-uses and their contribution in building total energy consumption. As shown in Figure 6, about 54.4% of the total energy consumption is dedicated to the HVAC system, including chillers, cooling tower, pumps, and fans. The current simulation platform enables the impact assessment of human–building interactions under different circumstances in the later sections of the paper.
Survey Study and Discussion
A survey study was conducted among 81 office users of the target building to evaluate any possible strong association between human–building interactions and psychological/social factors with the perceived habitual norms associated with energy end-uses. In this survey, all the examined variables were categorical or qualitative, thus; due to the relatively small size of the sample, a bias-corrected Cramér’s V method (Bergsma, 2013) was implemented among all the survey elements to determine any noticeable statistical correlations between the categorical variables within the sample and based on a
where
The distribution of the demographic and socioeconomic factors, including users’ gender, nationality, level of education, and income level, is shown in Figure 7. As shown in Figure 7, the sample consists of a comparable number of male and female users and mainly Qataris, along with Arab and Asian nationalities. Level of education was one of the selected factors with possible impacts on human–building interactions and other energy-related subjects. Most users held a BS or MS degree while 13 users held high school diplomas. The user income level was categorized into three different levels—low, medium, and high—to identify possible associations. Due to privacy issues, other factors such as exact income and age were not evaluated in this survey. Such information was crucial to enable the authors to determine possible correlations between demographic and socioeconomic dimensions with HBIs and other behavioral factors with respect to the building environment.
Besides demographic and socioeconomic information, users were asked about energy-related concerns to evaluate their attitude toward subjects that are directly associated with psychological factors developing human’s ascription of responsibility in public environments, awareness of environmental consequences, and attitude towards energy efficiency. The respondents’ feedback was assessed, afterwards, to find intercorrelations with HBI factors. The test results demonstrate the following observations based on Likert scale method in Figure 8. As shown in Figure 8, there is a clear gender-based discrepancy (V score ranging from 0.31 to 0.42), where female respondents demonstrate positive inclination towards the enquired topics. This discrepancy is more significant in response to the importance of energy efficiency ratings and efficient appliance use, where a considerable proportion of the females are in favor and more than half of the male respondents do not consider it as an important factor. No other significant correlation was observed with respect to nationality, education, and income level.
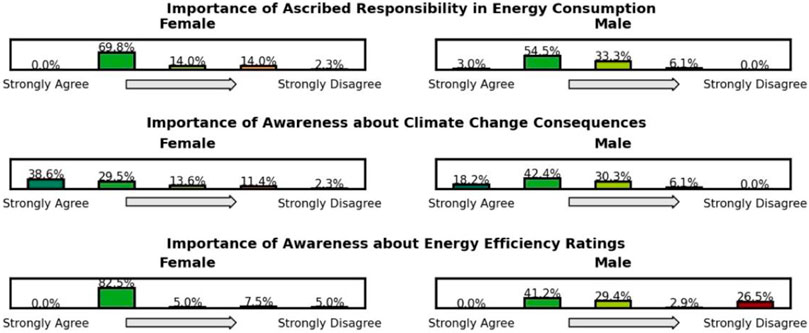
FIGURE 8. Sample’s response to general psychological topics regarding awareness of consequences and ascription of responsibility in energy.
Users were also asked about the frequency at which environmental concerns are brought up in their family and friend gatherings. A Cramér’s V test shows only a difference between two gender groups, which is shown in Figure 9. As seen in this figure, female users bring up environmental discussions more often compared to the male respondents who show an insignificant concern about these topics. No other significant correlations were observed with respect to education, income, and nationality, meaning that these factors do not influence human attitude towards environmental concerns.
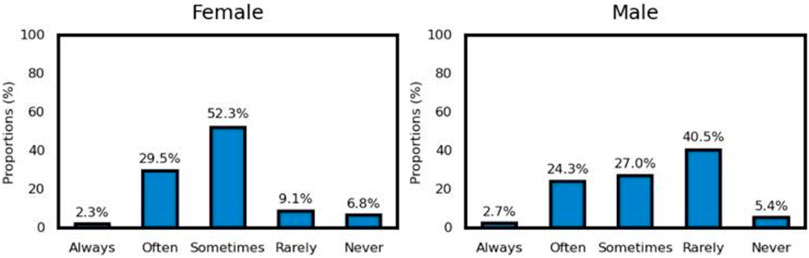
FIGURE 9. Users’ response to the frequency of environmental discussions in gatherings based on their gender.
The users were asked about their individual perceptions and habits regarding interactions with the building in the areas of lighting, equipment, fenestrations, window blinds, and thermostat upon arrival to the office, departure from the office, and during breaks. Figure 10 and Figure 11 demonstrate the users’ responses to the following questions:
1 Do you turn off the office lights/equipment when going on a break?
2 Do you turn off the office lights/equipment when you leave for the day?
3 Do you open the windows in your office?
4 Do you open the curtains/blinds in your office?
5 Do you override your office thermostat?
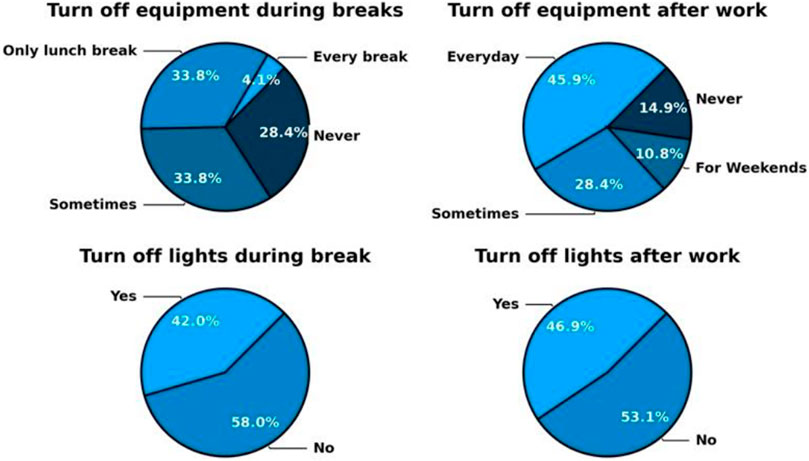
FIGURE 10. Human interaction with office lighting and equipment during break times and after leaving office.
Based on Cramér’s test, no meaningful correlations were observed between HBI factors and demographic/socioeconomic dimensions except the nationality of the respondents, where Asian nationals show more adverse interactions with respect to lighting and equipment during the breaks and after leaving office. The results also demonstrate that those with less concern about energy efficiency show more inclination toward irresponsible attitude and perceived behavioral control in human-building interactions. This negative attitude is also found in the users with less awareness regarding environmental challenges.
In addition, the preferred indoor temperature for each individual was incorporated to the survey (Figure 12). According to the results, intercorrelations were observed between different genders and nationalities, where the male respondents show more inclination to extreme hot or cold indoor temperatures compared to the females whose preference distribution is more concentrated about 22°C, Qatari nationals have more inclination towards colder environments, and Asian nationals are more inclined towards hotter indoor temperatures. It can also be seen that those with colder temperature preferences are more tend to thermostat override and open office windows that is indicative of a sign of thermal discomfort forcing the occupant to have adverse building interactions. No other noticeable interdependencies were identified in the survey data.
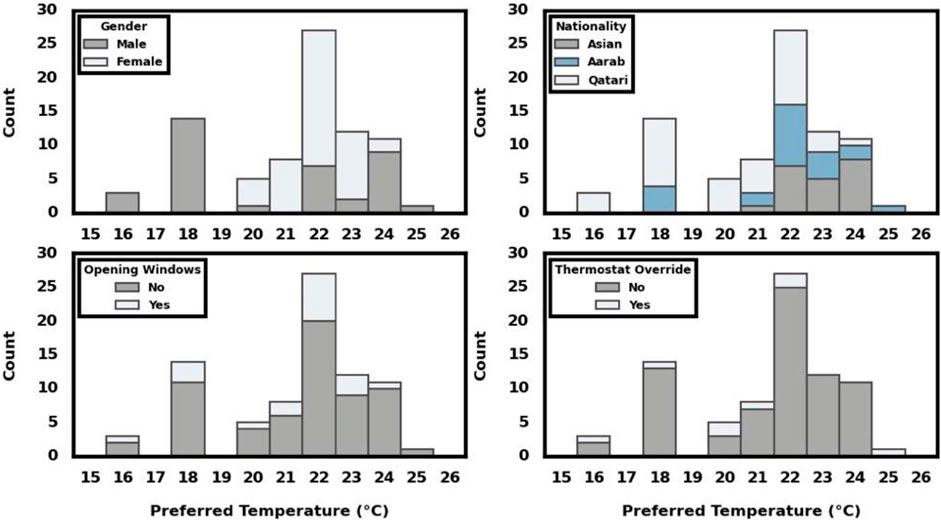
FIGURE 12. Distribution of user indoor temperature preference based on demographic and human building interactions.
Case Scenario Analysis and Discussion
Regardless of the fact that energy efficient equipment, smart control, and technology-driven solutions can eventually result in better building operation performance at the same time, human-driven factors can become an impediment toward a desirable building operation; conversely, human interactions, perceived responsibility, and normative goals can result in considerable energy-saving opportunities. As a result, human dimension factors should not be neglected in the operation and control of buildings. However, as mentioned previously, the extent of this adverse or positive impact is associated with uncertainties and dependent on factors such as building envelope, building mechanical/electrical assets, control capacities, data manipulation capabilities, and climate, demographic factors, and social factors. In this section, the objective was to reflect the impact of corrected adverse human–building interactions on building energy consumption patterns. Cognitive models were derived and incorporated in the building simulation to construct different decision probability distributions for human–building interactions. The analysis was categorized into seven different scenarios:
1 Base scenario, in which the building simulation and consumption patterns reflect the reported self-perceived interactions in Survey Study and Discussion section. In this scenario, the occupants can constantly override the thermostats and interact with building fenestrations, internal lighting and equipment, and window curtains/blinds. This scenario will reflect the saving potentials by improving the actual behavioral factors.
2 In this scenario, thermostat override is restricted, and temperature setpoint schedules follow the occupancy patterns.
3 In this scenario, the lighting system is actuated by motion sensors and follows the occupancy patterns.
4 In this scenario, opening office windows is restricted and windows are constantly closed.
5 In this scenario, the window curtains and blinds are constantly closed.
6 In this scenario, the office equipment is controlled when the zone is unoccupied.
7 In this scenario, the stacked impact of all previous scenarios (3–7) is demonstrated.
8 Extreme scenario, in which it is assumed that all the occupants, regardless of their self-perceived behavior, present the worst interactions with the office environment. This scenario will determine the maximum possible consumption discrepancies due to extremely adverse human building interactions.
Result Analysis
A comparison between the second scenario and the base scenario is demonstrated in Figure 13. As shown in this figure, no considerable improvement in electricity peak demand is created by adopting this new control policy. However, the total electricity consumption is reduced by 0.58%, which is equivalent to 4.15 MWh annual savings. It should be noted that this saving is a result of restricting possible human override in only 74 zones out of the total 145 conditioned zones.
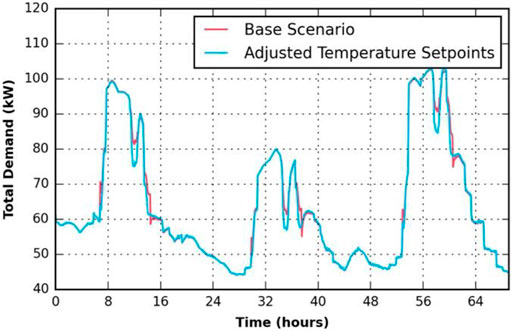
FIGURE 13. Improving building electricity demand by adjusting temperature setpoints following office occupancy.
The third scenario demonstrates more noticeable saving opportunities when the lighting system follows occupancy patterns by motion sensors in the office zones. The results demonstrate a 3.31% total electricity consumption saving, equivalent to 23.68 MWh annual energy consumption reduction. A comparison between scenario 2 and the base case scenario is shown in Figure 14. The improvement is the result of adjusting the interactions of the occupants without perceived responsibility.
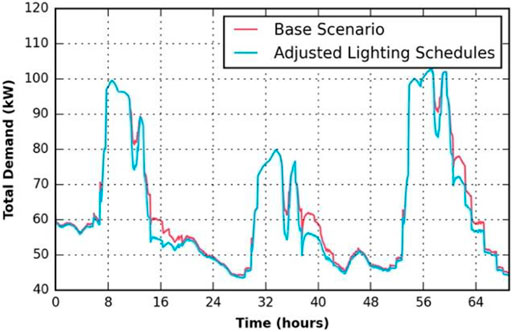
FIGURE 14. Improving building electricity consumption by adjusting lighting system operation following office occupancy.
The fourth scenario demonstrates a 1.06% annual electricity consumption reduction (about 7.54 MWh annual consumption) only as a result of partially disabling opening building fenestrations. A comparison between this scenario and the base scenario is shown in Figure 15. Changing human interactions with office curtains/blinds did not show noticeable improvement in energy savings (about 0.14% saving).
The simulation outcome of the sixth scenario exhibits an 4.24% electricity consumption savings as a result of occupancy-oriented control of office equipment. This is equivalent to 30.25 MWh annual savings. A comparison is shown in Figure 16.
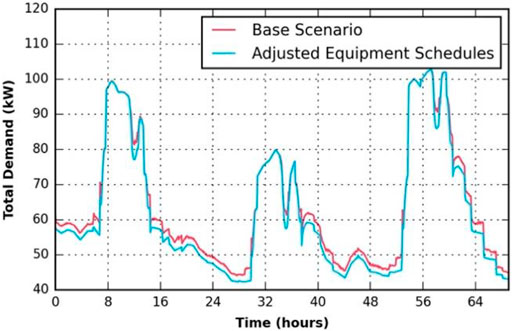
FIGURE 16. Improving building electricity consumption by controllable office equipment following occupancy patterns.
In general, building dynamics are complex systems with non-linear characteristics. Consequently, the savings in scenarios 2 through 6 cannot be superimposed to evaluate the stacked impact in scenario 7. When all human–building interactions are adjusted as described in scenario 7, annual electricity consumption reduction achieves 8.99%—or 64.15 MWh electricity savings. The stacked result is shown in Figure 17. Figure 18 shows a comparison of all the scenarios.
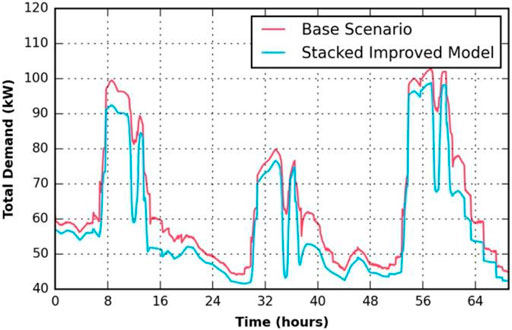
FIGURE 17. Adjusting building consumption patterns as a result of adopting and stacking scenarios 2 through 6.
Conclusion
This study emphasizes the associations between human dimensions and building interactions and the impact of factors such as demographics, socioeconomics, and behavioral dimensions on building electricity consumption patterns. An office space was targeted, and a survey study was conducted among the building occupants, with various demographic and economic traits, regarding their self-perceived attitude and preference toward building interactions. Concerns associated with awareness of energy and environmental consequences, ascribed responsibility, and knowledge about energy efficiency were addressed in the survey to determine possible associations between behavioral factors. The survey outcome demonstrated that about women are more likely to be either aware or concerned about energy/environmental topics, with no considerable association with education, ethnicity, and income level. Association was observed between gender/nationality and indoor temperature preference, while user nationality demonstrated intercorrelation between user and building end-use interactions, probably as a result of cultural and gain goals. It was found out that users with colder indoor temperature preferences were more likely to override office thermostats. The survey analysis also provided insight to the building occupants’ behavioral factors interacting with the building end-uses. The behavioral factors for each occupant were incorporated in a building simulation and modeled under multiple scenarios. The results indicated that human behavioral factors noticeably change the building load profile for the subject building. Thermostat adjustment based on occupancy patterns for closed office spaces only (74 zones out of 145 total zones) resulted in 0.58% annual savings, and adjusted lighting use behavior (either human-oriented or actuated by occupancy sensors) resulted in 3.3% annual savings. Adjusted human behavior associated with interactions with office fenestrations and office equipment resulted in 1.05 and 4.2% annual savings, respectively. The aggregate implementation of the adjusted human behavior also resulted in total annual savings of 8.99%. The scenarios were conducted again based on extreme adverse human-building interactions and the stacked total annual savings increased to 18.3%.
Such saving opportunities are only achievable through establishing correct habitual norms and creating motivation, opportunity, limitations, and ability. Most improved human interactions can be effectively enabled by communication technologies and automation systems, generally requiring retrofit, upgrade, and refurbishment. Thus, the building operator or decision-makers must conduct a proper economic analysis to evaluate viable alternatives in engaging humans in building operations. Building operation enhancement and improved human–building engagement could also involve energy policies and high-level targets to point society segments toward more awareness. Such energy policies need comprehensive social studies due to the fact that human–building interaction and the extent of the impact are correlated with demographics, socioeconomic factors, and externalities that are independent from social factors.
Data Availability Statement
The original contributions presented in the study are included in the article/Supplementary Material, further inquiries can be directed to the corresponding author.
Author Contributions
EZ and AG: Research idea, writing, supervision ED: Literature Review.
Conflict of Interest
The authors declare that the research was conducted in the absence of any commercial or financial relationships that could be construed as a potential conflict of interest.
Publisher’s Note
All claims expressed in this article are solely those of the authors and do not necessarily represent those of their affiliated organizations, or those of the publisher, the editors and the reviewers. Any product that may be evaluated in this article, or claim that may be made by its manufacturer, is not guaranteed or endorsed by the publisher.
Acknowledgments
This publication was made possible by an NPRP award (NPRP11S-1228-170142) from the Qatar National Research Fund (a member of Qatar Foundation). The statements made herein are solely the responsibility of the authors. The publication of this article was funded by the Qatar National Library.
References
Allen, M., Wicks, R. H., and Schulte, S. (2013). Online Environmental Engagement Among Youth: Influence of Parents, Attitudes and Demographics. Mass Commun. Soc. 16 (5), 661–686. doi:10.1080/15205436.2013.770032
Al-Marri, W., Al-Habaibeh, A., and Watkins, M. (2018). An Investigation into Domestic Energy Consumption Behaviour and Public Awareness of Renewable Energy in Qatar. Sustainable Cities Soc. 41, 639–646. doi:10.1016/j.scs.2018.06.024
Ansi/Ashrae (2004). “ANSI/ASHRAE 55:2004 Thermal Environmental Conditions for Human Occupancy,” Ashrae. 2004, 30. doi:10.1007/s11926-011-0203-9
Bergsma, W. (2013). A Bias-Correction for Cramér's and Tschuprow's. J. Korean Stat. Soc. 42 (3), 323–328. doi:10.1016/j.jkss.2012.10.002
Brager, G. S., and de Dear, R. J. (1998). Thermal Adaptation in the Built Environment: a Literature Review. Energy and Buildings. 27 (1), 83–96. doi:10.1016/S0378-7788(97)00053-4
Cialdini, R. B. (2003). Crafting Normative Messages to Protect the Environment. Curr. Dir. Psychol. Sci. 12 (4), 105–109. doi:10.1111/1467-8721.01242
Cotton, D., Miller, W., Winter, J., Bailey, I., and Sterling, S. (2016). Knowledge, Agency and Collective Action as Barriers to Energy-Saving Behaviour. Local Environ. 21 (7), 883–897. doi:10.1080/13549839.2015.1038986
Craig, C. A., and Allen, M. W. (2015). The Impact of Curriculum-Based Learning on Environmental Literacy and Energy Consumption With Implications for Policy. Utilities Policy. 35, 41–49. doi:10.1016/j.jup.2015.06.011
de Dear, R. J., and Brager, G. S. (1998). Developing an Adaptive Model of Thermal Comfort and Preference. ASHRAE Trans. 104 (1), 1–18.
Deuble, M. P., and de Dear, R. J. (2012). Green Occupants for Green Buildings: The Missing Link? Building Environ. 56, 21–27. doi:10.1016/j.buildenv.2012.02.029
Dixon, G. N., Deline, M. B., Mccomas, K., Chambliss, L., and Hoffmann, M. (2015). Using Comparative Feedback to Influence Workplace Energy Conservation. Environ. Behav. 47, 667–693. doi:10.1177/0013916513520417
EnergyPlus Energy Simulation Software (2014). U.S. Department of Energy. [Online].Available at: http://apps1.eere.energy.gov/buildings/energyplus/(Accessed September 1, 2012).
Erickson, V. L., and Cerpa, A. E. (2010). Occupancy Based Demand Response HVAC Control Strategy. Buildsys’10 - Proc. 2nd ACM Work. Embed. Sens. Syst. Energy-efficiency Build., 7–12. doi:10.1145/1878431.1878434
Frederiks, E. R., Stenner, K., and Hobman, E. V. (2015). Household Energy Use: Applying Behavioural Economics to Understand Consumer Decision-Making and Behaviour. Renew. Sustainable Energ. Rev. 41, 1385–1394. doi:10.1016/j.rser.2014.09.026
Gustafson, C., and Longland, M. (2008a). “Engaging Employees in Conservation Leadership,” ACEEE Summer Study Energy Effic. Washington, DC: Build., 134–147.
Gustafson, C., and Longland, M. (2008b). Engaging Employees in Conservation Leadership, Washington, DC, 134–147.
Handgraaf, M. J. J., Van Lidth de Jeude, M. A., and Appelt, K. C. (2013). Public Praise vs. Private Pay: Effects of Rewards on Energy Conservation in the Workplace. Ecol. Econ. 86, 86–92. doi:10.1016/j.ecolecon.2012.11.008
Holzmann, A., and Schmid, E. (2018). Consumer Behaviour in the Residential Heating Sector in Austria: Findings from a Bottom-Up Modelling Approach. Energy and Buildings. 158, 486–493. doi:10.1016/j.enbuild.2017.10.036
Jia, M., Srinivasan, R. S., and Raheem, A. A. (2017). From Occupancy to Occupant Behavior: An Analytical Survey of Data Acquisition Technologies, Modeling Methodologies and Simulation Coupling Mechanisms for Building Energy Efficiency. Renew. Sustainable Energ. Rev. 68 (2017), 525–540. doi:10.1016/j.rser.2016.10.011
Labanca, N., and Bertoldi, P. (2018). Beyond Energy Efficiency and Individual Behaviours: Policy Insights from Social Practice Theories. Energy Policy. 115, 494–502. doi:10.1016/j.enpol.2018.01.027
Li, Z., and Dong, B. (2018). Short Term Predictions of Occupancy in Commercial Buildings-Performance Analysis for Stochastic Models and Machine Learning Approaches. Energy and Buildings. 158, 268–281. doi:10.1016/j.enbuild.2017.09.052
Michie, S., Atkins, L., and West, R. (2014). The Behaviour Change Wheel: A Guide to Designing Interventions Sutton, UK: Silverback Publishing.
Michie, S., van Stralen, M. M., and West, R. (2011). The Behaviour Change Wheel: A New Method for Characterising and Designing Behaviour Change Interventions. Implementation Sci. 6 (1), 42. doi:10.1186/1748-5908-6-42
Mohamed, A. M. A., Al-Habaibeh, A., Abdo, H., and Elabar, S. (2015). Towards Exporting Renewable Energy From MENA Region to Europe: An Investigation into Domestic Energy Use and Householders' Energy Behaviour in Libya. Appl. Energ. 146, 247–262. doi:10.1016/j.apenergy.2015.02.008
Murtagh, N., Nati, M., Headley, W. R., Gatersleben, B., Gluhak, A., Imran, M. A., et al. (2013). Individual Energy Use and Feedback in an Office Setting: A Field Trial. Energy Policy. 62, 717–728. doi:10.1016/j.enpol.2013.07.090
Nazemi, N. D., Zaidan, E., and Jafari, M. A. (2021). The Impact of Occupancy-Driven Models on Cooling Systems in Commercial Buildings. Energies 14 (6), 1722.
Nicol, J. F., and Humphreys, M. A. (2002). Adaptive Thermal comfort and Sustainable Thermal Standards for Buildings. Energy and Buildings. 34 (6), 563–572. doi:10.1016/S0378-7788(02)00006-3
Oca, D., and William, J. N. (2015). An Ontology to Represent Energy- Related Occupant Behavior in Buildings Part I: Introduction to the DNAs Framework Elsevier.
Owen, T., and Energy, P. (2010). Employee Engagement and Energy Information Software Supporting Carbon Neutrality Introduction – Employee Engagement for Energy Conservation. American Council for Energy-Efficient Economy, 233–244.
Pothitou, M., Hanna, R. F., and Chalvatzis, K. J. (2016). Environmental Knowledge, Pro-Environmental Behaviour and Energy Savings in Households: An Empirical Study. Appl. Energ. 184, 1217–1229. doi:10.1016/j.apenergy.2016.06.017
Rinaldi, A., Schweiker, M., and Iannone, F. (2018). On Uses of Energy in Buildings: Extracting Influencing Factors of Occupant Behaviour by Means of a Questionnaire Survey. Energy and Buildings. 168, 298–308. doi:10.1016/j.enbuild.2018.03.045
Schelly, C., Cross, J. E., Franzen, W. S., Hall, P., and Reeve, S. (2010). Reducing Energy Consumption and Creating a Conservation Culture in Organizations: A Case Study of One Public School District. Environ. Behav. 43 (3), 316–343. doi:10.1177/0013916510371754
Simcock, N., MacGregor, S., Catney, P., Dobson, A., Ormerod, M., Robinson, Z., et al. (2014). Factors Influencing Perceptions of Domestic Energy Information: Content, Source and Process. Energy Policy. 65, 455–464. doi:10.1016/j.enpol.2013.10.038
Staddon, S. C., Cycil, C., Goulden, M., Leygue, C., and Spence, A. (2016). Intervening to Change Behaviour and Save Energy in the Workplace: A Systematic Review of Available Evidence. Energ. Res. Soc. Sci. 17, 30–51. doi:10.1016/j.erss.2016.03.027
Staff, I. C. C. (2018). IgCC International Green Construction Code: A Comprehensive Solution for High-Performance Buildings. International Code Council, Incorporated, 2018.
Stern, P. C. (2017). How Can Social Science Research Become More Influential in Energy Transitions? Energ. Res. Soc. Sci. 26, 91–95. doi:10.1016/j.erss.2017.01.010
Stern, P. C., Sovacool, B. K., and Dietz, T. (2016). Towards a Science of Climate and Energy Choices. Nat. Clim Change. 6, 547–555. doi:10.1038/nclimate3027
Tabak, V., and de Vries, B. (2010). Methods for the Prediction of Intermediate Activities by Office Occupants. Building Environ. 45 (6), 1366–1372. doi:10.1016/j.buildenv.2009.11.018
Turner, W., Hong, T., and Lawrence, E. O. (2014). A Technical Framework to Describe Occupant Behavior for Building Energy Simulations.
Typical Meteorological Year (2007). Nsrdb.Nrel.Gov. https://ec.europa.eu/jrc/en/PVGIS/tools/tmy
US Department of Energy (2015). EnergyPlus Engineering Reference https://energyplus.net/sites/all/modules/custom/nrel_custom/pdfs/pdfs_v9.2.0/EngineeringReference.pdf
Wetter, M. (2012). Co-Simulation of Building Energy and Control Systems With the Building Controls Virtual Test Bed. [Online]. Available at: http://www.escholarship.org/uc/item/7cp597jf.
Wright, T. A., Cropanzano, R., and Bonett, D. G. (2007). The Moderating Role of Employee Positive Well Being on the Relation Between Job Satisfaction and Job Performance. J. Occup. Health Psychol. 12 (2), 93–104. doi:10.1037/1076-8998.12.2.93
Yun, R. (2014). Persistent Workplace Plug-Load Energy Savings and Awareness through Energy Dashboards: Feedback , Control , and Automation. New York: ACM, 331–334.
Yun, R., Aziz, A., Scupelli, P., Lasternas, B., Zhang, C., and Loftness, V. (2015). “Beyond Eco-Feedback,” in Conference on Human Factors in Computing Systems - Proceedings. 2015, 1989–1992. doi:10.1145/2702123.2702268
Keywords: building energy, human-building interactions, behavior adjustment, human attitude, behavioral norms
Citation: Zaidan E, Ghofrani A and Dokaj E (2021) Analysis of Human-Building Interactions in Office Environments: to What Extent Energy Saving Boundaries can be Displaced?. Front. Energy Res. 9:715478. doi: 10.3389/fenrg.2021.715478
Received: 27 May 2021; Accepted: 02 August 2021;
Published: 12 August 2021.
Edited by:
Bassem Kahouli, University of Sousse, TunisiaReviewed by:
Elina Husini, Islamic Science University of Malaysia, MalaysiaKamel Bel Hadj Miled, Shaqra University, Saudi Arabia
Copyright © 2021 Zaidan, Ghofrani and Dokaj. This is an open-access article distributed under the terms of the Creative Commons Attribution License (CC BY). The use, distribution or reproduction in other forums is permitted, provided the original author(s) and the copyright owner(s) are credited and that the original publication in this journal is cited, in accordance with accepted academic practice. No use, distribution or reproduction is permitted which does not comply with these terms.
*Correspondence: Esmat Zaidan, ezaidan@qu.edu.qa