- 1Nuclear Energy and Fuel Cycle Division, Oak Ridge National Laboratory, Oak Ridge, TN, United States
- 2Department of Mechanical, Aerospace and Nuclear Engineering, Rensselaer Polytechnic Institute, Troy, NY, United States
- 3Department of Nuclear Science and Engineering, Massachusetts Institute of Technology, Cambridge, MA, United States
In a carbon-constrained world, future uses of nuclear power technologies can contribute to climate change mitigation as the installed electricity generating capacity and range of applications could be much greater and more diverse than with the current plants. To preserve the nuclear industry competitiveness in the global energy market, prognostics and health management (PHM) of plant assets is expected to be important for supporting and sustaining improvements in the economics associated with operating nuclear power plants (NPPs) while maintaining their high availability. Of interest are long-term operation of the legacy fleet to 80 years through subsequent license renewals and economic operation of new builds of either light water reactors or advanced reactor designs. Recent advances in data-driven analysis methods—largely represented by those in artificial intelligence and machine learning—have enhanced applications ranging from robust anomaly detection to automated control and autonomous operation of complex systems. The NPP equipment PHM is one area where the application of these algorithmic advances can significantly improve the ability to perform asset management. This paper provides an updated method-centric review of the full PHM suite in NPPs focusing on data-driven methods and advances since the last major survey article was published in 2015. The main approaches and the state of practice are described, including those for the tasks of data acquisition, condition monitoring, diagnostics, prognostics, and planning and decision-making. Research advances in non-nuclear power applications are also included to assess findings that may be applicable to the nuclear industry, along with the opportunities and challenges when adapting these developments to NPPs. Finally, this paper identifies key research needs in regard to data availability and quality, verification and validation, and uncertainty quantification.
Introduction
Reducing anthropogenic greenhouse gas (GHG) emissions for climate change mitigation while expanding energy access to billions of people is a central global challenge of this century. As the world’s second-largest low-carbon power source (second only to hydropower), nuclear power makes up more than one-quarter of annual low-carbon electricity supply worldwide and has avoided about 60 gigatons of GHG emissions over the past 50 years (IEA, 2019). At present, approximately 10% of global electricity generation is produced by nuclear power each year (IAEA, 2021). To achieve deep decarbonization targets, including the one limiting average global warming to 2°C in 2050 (Gao et al., 2017), it is imperative to maintain the existing nuclear share of electricity production (MIT, 2018).
Despite its important role in energy transitions to meet climate goals, the nuclear industry is facing an uncertain future in many countries, not only due to the March 2011 Fukushima accident in Japan but also more fundamentally for economic reasons. In advanced economies such as the United States, unfavorable market conditions—including weak growth in electricity demand, low natural gas prices, and increasing competition from renewables-based power supply—are putting pressure on the financial performance of existing nuclear power plants (NPPs), which may lead to their early retirements. One of the first thrusts being pursued to support economical nuclear power has been focused on life extensions of the legacy fleet, from the initial license period of 40 years (in most cases) to 50–60 years and possibly beyond. Life extensions are considerably cheaper than new construction and will be cost-competitive with any other electricity generation technology, as illustrated in Figure 1 for the projected US levelized cost of electricity associated with different technologies in 2040. A new joint report (IEA and NEA, 2020) by the International Energy Agency (IEA) and the Nuclear Energy Agency (NEA) also concludes that prolonging the operation of existing nuclear assets, known as long-term operation (LTO), is the most affordable low-carbon solution. Unfortunately, the LTO of current NPPs alone—mostly light water reactors (LWRs)—can only provide temporary support for the transition to clean energy systems. New builds are necessary, and near-term interests are rising in LWR-based small modular reactors (SMRs) and mature Generation-IV concepts.
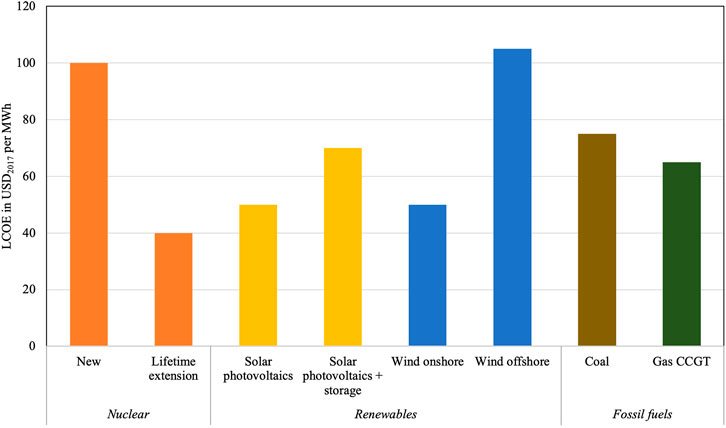
FIGURE 1. Projected US LCOE by technology in 2040 [modified from IEA (2019)]. Note for Figure 1: LCOE = levelized cost of electricity, average cost to build and operate a power plant over its lifetime divided by the total electricity output of the plant over the same period; it represents the break-even price of electricity generation at a power production facility. CCGT = combined-cycle gas turbines. Estimates of nuclear lifetime extension are based on a 500 million USD investment to extend operations for 20 years. Other cost assumptions can be found in IEA (2019).
To achieve safe, reliable, and economical operation of NPPs, attention is turning to enhanced plant asset management methods within the activities of both legacy fleet LTO and new construction. Decades of global operational experience have shown that greater situational awareness of the condition of key structures, systems, and components (SSCs) is essential for managing and mitigating plant equipment degradation, particularly the aging-related degradation due to exposure to a harsh operating environment. While the traditional approaches to maintenance and aging management complied with the defense-in-depth policy (IAEA, 1996) and proved to be adequate for maintaining safety margins in the past, they were not optimized in terms of effort, time, or cost (Coble et al., 2012). Historically, corrective find-and-fix maintenance policies prevailed in the early days of the nuclear industry, which would incur overly long facility downtime and excessively high cost (Ayo-Imoru and Cilliers, 2018). The time-based periodic maintenance scheduling became widely employed since the 1970s. However, this strategy is generally conservative and often yields unnecessary planned inspection and maintenance that challenge the economics of nuclear generation. Meanwhile, it does not prevent plant downtime caused by unanticipated equipment failure, which leads to a significant amount of lost revenue: at least $1.2 million per day of plant shutdown for an average NPP in the United States and France (NEI, 2011; Power Engineering International, 2017). Therefore, it is necessary to move from uneconomic find-and-fix or periodic maintenance strategies to the more cost-effective just-in-time repair policies.
The just-in-time repair is a predictive maintenance strategy that relies on continuous monitoring and full awareness of the equipment health condition throughout its life cycle, or in other words, the use of prognostics and health management (PHM) principles. The full PHM suite includes five modules: data acquisition, monitoring and anomaly detection, fault diagnostics, prognostics, and planning and decision-making. Through appropriate detection, diagnosis and prognosis, and mitigation actions, a robust PHM system will allow early warning of degradation in NPPs and will potentially preclude serious consequences due to faults and failures while helping alleviate the burden of unnecessary maintenance activities.
This paper provides an updated method-centric review of the full PHM suite in NPPs since the last major survey article by Coble et al. (2015) was published in 2015. The results of that survey are augmented with new progress made in the intervening years. In particular, recent advances in data-driven analysis methods—largely represented by those in artificial intelligence (AI) and machine learning (ML)—have enhanced applications ranging from robust anomaly detection to automated control and autonomous operation of complex systems. PHM in NPPs is one area where the application of those algorithmic advances can significantly improve the ability to perform enhanced asset management. Therefore, special attention is dedicated to the advances in data-driven diagnostic and prognostic methods. PHM technologies in non-nuclear power applications are also included to assess findings that may be applicable to the nuclear industry, along with the opportunities and challenges when adapting these developments to NPPs. “US NPP Monitoring and Maintenance: Historical Approach and Motivations for Prognostics and Health Management” Section summarizes the historical approach to monitoring and maintenance in US NPPs and outlines the PHM needs for improving the safety and economy of both LTO and new builds. “Prognostics and Health Management Framework and Modeling Approaches” Section describes the PHM framework, followed by the state of practice for each of its five modules with a focus on data-driven methods. “Research Needs for Deployment of Prognostics and Health Management in Nuclear Power Plants” Section identifies the overarching research needs to support the development and deployment of PHM in NPPs. “Summary” Section summarizes the key findings of this paper.
US NPP Monitoring and Maintenance: Historical Approach and Motivations for Prognostics and Health Management
The United States has the largest number of commercial nuclear reactors in the world. Its operating fleet [94 LWRs in 56 NPPs (IAEA, 2021) as of January 2021] has steadily generated about 20% of the nation’s electricity since the mid-1990s (NEI, 2021a) at by far the highest capacity factor [93.4% in 2019 (US DOE, 2019)] of any energy source. Despite this performance and the fact that nuclear makes up more than half of the nation’s clean energy (NEI, 2021b), nine reactors have been shut down in the United States before their licenses expired since 2012 due to unfavorable market conditions, and an additional five units are scheduled to retire in 2021 (US DOE, 2020a; US EIA, 2021a). The average age of US operating reactors is almost 40 years. The youngest unit, Tennessee’s Watts Bar Nuclear Plant Unit 2, began operation in 2016 and was the nation’s first new reactor in 20 years (US EIA, 2021b). Meanwhile, only two commercial reactors—2 AP1000 units at Georgia’s Vogtle plant—are currently under construction (IAEA, 2021) in the country. To keep America’s nuclear capacity from sharply declining and to enable clean energy transition, the current LWR fleet is undergoing 20-years life extensions from the original 40-years licenses; 85 reactors1 have been approved by the US Nuclear Regulatory Commission (NRC) to operate 60 years through the initial license renewal applications (NEI, 2021c). To date, 53 reactors have already entered extended operation or LTO (US NRC, 2021a). Additionally, utilities are intending to operate up to 80 years through second 20-years extensions or subsequent license renewals; four reactors have been issued a second renewed license for extended LTO and six additional applications are under review (US NRC, 2021b).
To comply with the NRC’s license renewal rule [Title 10, Part 54 of the Code of Federal Regulations, or 10 CFR 54 (US NRC, 1995)] and to continue to provide secure nuclear power generation, it is imperative to understand and manage the effects of SSC aging in NPPs. As described in Coble et al. (2012), the NRC monitoring and maintenance programs usually draw a distinction between active and passive SSCs.2 The active SSCs—such as control rod drives, generators, sensors, motors, pumps, and valves—must move to perform their intended functions. Their performance monitoring and aging management have been historically covered by the Maintenance Rule (10 CFR 50.65) (US NRC, 2021c). The Maintenance Rule provides a performance-based approach to monitoring and improving the overall effectiveness of active component maintenance. However, it does not directly improve the economics of performing maintenance (Coble et al., 2012). Under the Maintenance Rule, a large majority of maintenance activities remain periodically scheduled. The passive SSCs—such as reactor pressure vessels (RPVs), heat exchangers, transformers, cables, support structures, and piping—do not move during normal functions. Their degradation and maintenance are managed through periodic in-service inspection as dictated by the plant’s aging management program. As codified in 10 CFR 50.55a (US NRC, 2021d), nondestructive inspection requirements are specified for the in-service inspection of passive components.
As plants enter LTO, aging becomes a more challenging problem. Because it is of paramount importance to be warned of impending SSC faults and failures, the frequency of periodic inspection and maintenance will need to increase to compensate for potentially growing failure rates over time due to wear-out failures in active SSCs and for reduced safety margins toward the lowest allowable level due to degraded material characteristics in passive SSCs. The increased inspection frequency would cause extended (and sometimes unnecessary) downtime of plant safety-critical systems and eventually affect the industry’s competitiveness. Transitioning from periodic maintenance scheduling to a more continuous, just-in-time health management approach is essential to ensure that the intended functions of NPP assets are maintained for the period of extended operation. Advanced monitoring techniques will provide the necessary support to this transition, along with advances in diagnostic and prognostic methods.
Currently, there is a growing interest in applying condition-based (rather than time-based) maintenance for active components and automated online monitoring (instead of periodic inspection) for passive components through the use of PHM. In fact, well-applied PHM technologies will benefit not only aging LWRs but also new builds, especially LWR-based SMRs [such as the pressurized water NuScale720 (US DOE, 2020b) and the boiling water GEH BWRX-300 (US DOE, 2020c)] and mature advanced reactor designs as part of the Generation-IV initiative [such as TerraPower’s Natrium and X-energy’s Xe-100 under the US Department of Energy [DOE]’s Advanced Reactor Demonstration Program (US DOE, 2020d)], into which inherent and passive safety features are extensively incorporated. These reactors have additional monitoring and surveillance needs over currently operating LWRs due to extended fuel cycles, exposure to harsher operating environments, use of innovative materials, and remote siting with reduced maintenance staffing levels (Coble et al., 2015). Traditional inspection techniques and maintenance policies will not meet such needs.
In addition to improving plant safety and reliability, PHM is also economically attractive for reducing operations & maintenance (O&M) costs compared to time-based and even traditional condition-based (i.e., without the use of PHM)3 policies. The O&M costs represent a crucial disadvantage for the nuclear industry and comprise about two thirds of total generating costs in NPPs (Coble et al., 2015; Al Rashdan et al., 2018). As discussed in “Introduction” Section, periodic inspection and maintenance could lead to unnecessary and unanticipated repair or replacement of SSCs, incurring significant additional downtime and costs. Besides, compared to the traditional concept of condition-based maintenance (CBM), PHM-enabled CBM provides capabilities to achieve more proactivity in O&M, places stronger emphasis on the operation stage than the design stage, and rely on condition-based, facility-specific status identification rather than population statistics. While detailed cost-benefit analyses of using PHM in specific NPPs are yet to be conducted, Bond et al. (2011) suggest that applying PHM technologies to all key SSCs in the nation’s legacy plants could result in an annual fleet-wide savings of more than $1 billion.4 Furthermore, proper application of the complete PHM suite—especially with automated planning and decision-making capabilities—can effectively reduce labor reliance and frequency of O&M activities because labor costs account for approximately 80% of O&M costs in US plants.
Prognostics and Health Management Framework and Modeling Approaches
This paper reviews the full PHM suite, which utilizes sensor technologies and data analytics to monitor health conditions, detect anomalies, diagnose faults, predict the remaining useful life (RUL), and proactively manage failures (Droguett, 2020) in complex engineering systems such as NPP assets. The five modules/steps of a full PHM system are depicted in Figure 2, and each module will be elaborated on in the following subsections. To date, there has been no universally well-defined categorization of PHM systems partly due to lack of unifying PHM standards, which are needed for harmonized terminology, consistency of PHM methods, and compatibility/interoperability of PHM technology. A number of disparate industrial standards—mostly developed by the International Organization for Standardization (ISO) and the Institute of Electrical and Electronics Engineers (IEEE)—exist which pertain to different modules of a PHM system, such as ISO 13374 series for condition monitoring (CM) of industrial machines, ISO 13379 for diagnostics, ISO 13381 for prognostics, and IEEE P1856 for PHM of electronic systems. Vogl et al. (2014) surveyed existing PHM-related standards and identified areas for development of future standards.

FIGURE 2. Five modules/steps of PHM [adapted and extended from Coble et al., 2015)].
In a PHM system, sensory data collected from a target SSC are continuously monitored for deviations from normal behavior, which are indicators of incipient faults or anomalies.5 Once an anomaly is detected, it is important to diagnose the fault, or in other words, to locate the fault to a specific component or area of a structure (i.e., fault isolation) and to determine the root cause of the fault (i.e., fault identification). Depending on how the SSC will degrade, an appropriate prognostic model is then applied to estimate its RUL. Finally, O&M planning is informed by integrating prognostic calculations and risk assessment of proposed mitigation actions based on the current and postulated future health states of the target SSC to achieve optimal (and ultimately autonomous) control and decision-making.
Besides traditional modeling tools, the recent advancements in AI and ML technologies provide opportunities for leveraging emerging data-driven algorithms to effectively address PHM problems, especially those of diagnostics and prognostics. Details will be provided in the corresponding subsections. Figure 3 illustrates the growing research interest in the application of one such algorithmic example for PHM: deep learning (DL), a quickly developing subfield of ML. Through a systematic review of state-of-the-art DL-based PHM frameworks, Rezaeianjouybari and Shang (2020) recently presented the benefits and potentials of DL technologies in the PHM paradigm, especially in the presence of high-volume and multidimensional data streams that contain real-time information about the degradation and health condition of the system of interest.
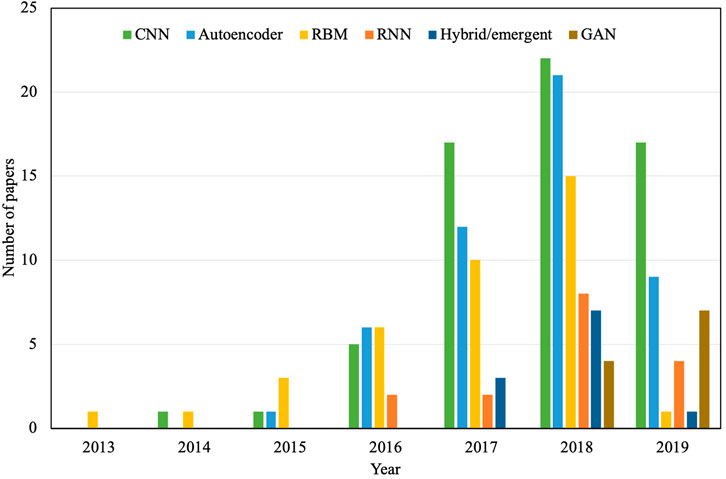
FIGURE 3. Breakdown of published papers on DL in PHM between 2013 and September 2019 (modified from Rezaeianjouybari and Shang (2020)) Note for Figure 3: DL architecture–share of total publications as follows: CNN (convolutional neural network)—32.1%; autoencoder—26.8%; RBM (restricted Boltzmann machine)—19.6%; RNN (recurrent neural network)—9.2%; hybrid/emergent—6.7%; GAN (generative adversarial network)—5.6%.
Data Acquisition: Emerging Sensor Technologies
Traditional reliability analyses rely on population statistics rather than condition-based status identification. Thus, they do not provide any useful insight regarding a specific SSC’s current or future state. The process of data acquisition from the target equipment is necessary to make an accurate, reliable prediction of individual SSC health. Collected data can be either event or sensory data (Atamuradov et al., 2017). Event data are O&M logs containing actions taken by the operator or maintenance staff in response to events that occurred to the physical asset and are not the focus of this paper. Sensory data are measurements tracked via sensors installed on the target equipment. Various types of sensors are needed to monitor key health related parameters in nuclear SSCs. Examples of such parameters include vibration, electrical signatures (current/voltage) and position measures in active SSCs, localized change in material properties (mechanical, magnetic, optical, thermal, or electrical) in passive SSCs, as well as general measurands of process conditions (such as temperature, flow, pressure) that may be associated with equipment degradation.
Due to the harsh operating environments (such as radiation, high pressure, high temperature) encountered in some parts of the NPP systems, many of the existing sensors that are widely used in other industries for abovementioned measurands may not survive. This section surveys recent research efforts to improve sensor survivability and measurement sensitivity for nuclear instrumentation. Some of the emerging sensing techniques, including those in use for nuclear applications and those which are deemed useful in the near future for CM inside NPPs, are briefly described in the following six subsections and compared in Table 1.
Magnetic Anisotropy
Magnetic properties of ferromagnetic materials depend on the direction in which they are measured. This phenomenon is known as magnetic anisotropy (Stefanita, 2008). Magnetic Barkhausen noise (MBN)—the electromagnetic waves emitted during a ferromagnetic material’s magnetization process—allows one to characterize magnetic anisotropy without regard to its origin (Spasojevic et al., 1996). Several studies have utilized MBN to continuously or periodically monitor material structure degradation, such as in structural steels of nuclear reactors (McCloy et al., 2013). Another effect produced by the movement of magnetic domain walls is the magneto acoustic emission (MAE). Acoustic signals are generated by the sudden and discontinuous changes in magnetization, which involve localized deformations (Stefanita, 2008). Simply put, the magnetic signal from a sensing coil corresponds to MBN (Deng et al., 2018), whereas the acoustic signal from a piezoelectric (PZT) sensor corresponds to MAE (Makowska et al., 2017). Li et al. (2015) investigated magnetic anisotropy of α-iron containing nonmagnetic particles for checking integrity of a nuclear RPV and suggested the possibility of using magnetic technologies for nondestructive evaluation of RPV embrittlement.
Piezoelectricity
Progress in sensor technology development has enabled the use of PZT transducers, which can be mounted on the surface or embedded inside host structural materials. Once they are integrated with the host structure, PZT elements are utilized as sensors to deliver signals in real-time. Simultaneously, they can also serve as actuators to generate diagnostic stress waves into the structure to detect, localize, and quantify damage in the materials. Various studies have proposed techniques using piezoelectric thin films attached to a material surface (Komagome and Matsumoto, 2002; Takahashi and Matsumoto, 2009; Sharma et al., 2012). By measuring the electric potential distribution on the piezoelectric film, the location, the aperture shape, and the defect’s depth can be estimated. The piezoelectric wafer active sensor (PWAS), another type of PZT sensor, has emerged as one of the major sensing techniques. PWASs were developed as convenient enablers for generating and receiving Lamb waves—a type of ultrasonic guided waves propagating between two parallel surfaces without much energy loss—for structural health monitoring in space applications (Cuc et al., 2007). Radiation influence on their sensing capability and survivability has been investigated to determine the reliability of PWAS-based methods for PHM in extreme nuclear environments (Haider et al., 2017). Additionally, research of PZT sensors using Lamb waves has been ongoing, and their capabilities for impact localization (Si and Baier, 2015; Park et al., 2017; Qiu et al., 2018), acoustic emission detection (Bhuiyan et al., 2018; Bhuiyan and Giurgiutiu, 2018), and damage detection in isotropic and composite plates (Dziendzikowski et al., 2016; Ebrahimkhanlou et al., 2016; Hong et al., 2018) have been explored. In-pile instrumentation development activities using PZT sensors have also been conducted recently—such as under several DOE Nuclear Energy programs investigating the use of new fuels and materials for advanced and existing reactors—to address crosscutting needs for irradiation testing by providing higher-fidelity, real-time data with increased accuracy and resolution from smaller, compact sensors that are less intrusive (Daw et al., 2014; Reinhardt et al., 2018).
Optical Fiber
Measurement techniques based on optical fibers have demonstrated the capability to provide multi-sensing (measuring different operational parameters within a single sensor configuration, such as temperature, pressure, and strain) and multiplexing (communicating data collected at multiple locations through the single line) instrumentation. They are intrinsically immune to electromagnetic interference, electrically passive, and widely available at a reasonable cost. Beyond the use as a light guide, several optical sensors and related measurement techniques have been considered for nuclear applications. Fiber Bragg grating (FBG) sensors have been the focus of many research efforts due to their demonstrated potential for high-temperature operation in a radioactive environment and their multiplexing capability. An FBG is achieved by creating a periodic modulation of the refractive index of the fiber core, which generates a distributed reflector characterized by its period and modulation depth. Several recent studies (Morana et al., 2016; Chen, 2018; Calderoni et al., 2019) have shown the effectiveness of certain radiation-resistant FBG sensor types—such as femtosecond-etched FBGs and germanosilicate singlemode FBGs—in monitoring diverse physical parameters for in-reactor instrumentation.
Piezoelectric-Fiber Hybrid Sensor System
A hybrid PZT/FBG system offers the best decoupling of actuator and sensor signals because the two devices apply different mechanisms for signal transmission. The PZT transducers rely on electrical channels to actuate or detect dynamic responses, whereas the FBG sensors rely on optical means to measure quasi-static or relatively low frequency responses (Wu et al., 2009). In other words, such a hybrid system uses piezoelectric actuators to input a controlled excitation to the structure and uses fiber optic sensors to capture the corresponding structural response (Qing et al., 2005). More generally, the accuracy and stability of SSC health monitoring can be potentially improved by constructing a hybrid sensor network and integrating multi-source sensor information (Wang et al., 2020a). Such hybrid sensor systems have not seen applications in NPPs but should not face hurdles given the respective success of PZT and FBG sensors.
Visual Vibrometry
Visual testing has played a prominent role in inspecting civil infrastructures. Recently, researchers have been able to use computer vision techniques to analyze small motions in videos. Those techniques amplify imperceptibly small motions in specified frequency bands, effectively producing a visualization of an object’s operational deflection shapes (Wadhwa et al., 2013). Video cameras provide the benefit of long-range measurements and enable the collection of a large amount of data at once since each pixel can be considered as a sensor. Objects tend to vibrate in a set of preferred modes, and the shapes and frequencies of the modes depend on the structure and material properties of an object. Focusing on the case where geometry is known or fixed, information about an object’s vibration modes can be extracted from video and used to make inferences about that object’s material properties (Davis et al., 2017). A camera-based vibration measurement methodology was also recently demonstrated for civil infrastructure by measuring an antenna tower’s ambient vibration response (Chen et al., 2017). Future research is needed to investigate the application of visual vibrometry inside NPPs with radiation exposure, such as for nuclear containment systems.
Electrical Impedance
Electrical impedance–based sensing is a relatively mature measurement field with broad nuclear applications, including passive structure, standby component, and in-pile monitoring. Lee et al. (2014) proposed laser-based mechanical impedance (LMI) measurement, utilizing a fiber-guided laser ultrasound system to generate and measure LMI response for damage detection in NPP pipes. Shin et al. (2016) suggested an online monitoring technique for standstill motors based on an impedance analysis method. More recently, Fleming et al. (2019) developed an impedance-based diameter gauge consisting of an electrically conductive concentric ring around fuel cladding, such that the electrical impedance between the ring and cladding could be measured.
Condition Monitoring and Fault Detection
Condition monitoring describes a suite of activities for providing state estimation and early warning of anomalous behavior. It is a crucial step of the PHM framework, and the effectiveness of PHM largely depends on the accuracy of the CM process (Ayo-Imoru and Cilliers, 2018). The process of fault detection attempts to recognize incipient faults and failures6 from CM data and quantification of the inconsistencies between the actual and the expected behavior of the monitored SSC in nominal conditions (Atamuradov et al., 2017).
The instrumentation and control (I&C) systems in NPPs receive large amounts of sensory data from various components to enable and support safe and reliable power generation by controlling the system variables. However, most raw data collected by sensors are not ready to be used directly, and appropriate data manipulation is required. The multidimensionality of high-volume data and redundancy among data attributes are examples of challenges faced by CM and fault detection. Therefore, the feature selection process—including choosing high-quality attributes, removing collinear features, and selecting an optimal subset from the original data set—is usually needed (Chandrashekar and Sahin, 2014). The objective of feature selection is to find a subset of variables from the full array of raw sensor data that can efficiently describe the input data stream while reducing effects from error/noise or irrelevant information (Guyon and Elisseeff, 2003).
Feature Selection Methods
Feature selection methods can be divided into three categories: filters, wrappers, and embedded methods. Filter methods pick up the intrinsic properties of the features measured by univariate statistics. In general, filter methods use variable ranking techniques as the principal criteria for variable selection. A suitable ranking criterion is used to score the input variables, and thresholds are applied to filter out the less relevant features. Several studies (John et al., 1994; Blum and Langley, 1997; Kohavi and John, 1997) have presented various definitions and measurements for the relevance of a variable. The widely used metrics such as mutual information, Fisher score, relief, separability, and correlation are all under the umbrella of the filter methods. The primary advantage of filter methods is their speed and ability to scale to large data sets. They are computationally light and are not prone to overfitting (Lazar et al., 2012). They also do not rely on the learning algorithm. One of the drawbacks of filter methods is that the selected subset might not be optimal because a redundant subset might be obtained. Besides, essential features that are less informative on their own but are informative when combined with other features could be discarded in error (Xu et al., 2010). Bommert et al. (2020) recently published a comprehensive survey analyzing 22 filter methods concerning runtime and accuracy in high-dimensional classification data.
Wrapper methods use the predictor as a black box and the predictor performance as the objective function to evaluate the variable subset. They search through the space of feature subsets using a learning algorithm and calculate the estimated accuracy of the learning algorithm for each feature that can be added to or removed from the feature subset. Also, they depend on a classification algorithm used to evaluate the candidate solutions (i.e., subsets of features) generated by a search algorithm and thus are more computationally expensive. Wrapper methods often provide more accurate results than filter methods (Pudil and Somol, 2008), although one needs to take extra care to prevent overfitting and wrappers usually scale poorly to large data sets (Das, 2001). The selection process is based on a specific learning algorithm trying to fit on a given data set. In general, it follows a greedy search approach by evaluating all the possible combinations of features against the evaluation criterion. For instance, the branch and bound algorithm (Narendra and Fukunaga, 1977), genetic algorithm (Goldberg, 1989), particle swarm optimization (Kennedy and Eberhart, 1995), adaptive floating search (Somol et al., 1999), recursive feature elimination (Guyon et al., 2002), and similarity measure (Chen and Chen, 2015) are all under the category of the wrapper methods.
Embedded methods complete the feature selection process within the construction of the ML algorithm itself. This method category combines the qualities of both filters and wrappers. The search for an optimal subset of features is embedded into the classifier construction and can be seen as a search in the combined space of feature subsets and hypotheses. The embedded methods use an independent measure to decide the best subsets for a given cardinality and use the learning algorithm to select the optimal subset among the best subsets across different cardinalities. Therefore, they are specific to a given learning algorithm and have the advantage of taking into consideration the interaction of features with the classification model (like wrapper methods) while being far less computationally intensive (like filter methods) (Saeys et al., 2007). Regularization and tree-based models are some common methods that use embedded feature selection. The weights of a classifier can also be used to rank the features for their removal, and the features can be selected by conducting sensitivity analysis on the corresponding weights. Several methods (Archibald and Fann, 2007; Mundra and Rajapakse, 2010; Zhang et al., 2015a) used support vector machines (SVMs)7 as classifiers, optimizing the SVM equation and assigning weights to each feature. In some other studies (Setiono and Liu, 1997; Verikas and Bacauskiene, 2002; Romero and Sopena, 2008; Yang and Ong, 2011), an artificial neural network (ANN) was applied for the same purpose.
As examples in the nuclear field, Deleplace et al. (2020) recently used a separability-based feature selection metric (i.e., filter method) to enhance accuracy of fault detection in NPP water screen cleaners; Peng et al. (2018a) applied correlation analysis (i.e., filter method) for dimensionality reduction of NPP transient data simulated from their personal computer transient analyzer; Zio et al. (2006) selected features for early transient detection by means of genetic algorithms (i.e., wrapper method); Moshkbar-Bakhshayesh (2021) investigated six different feature selection techniques for parameter estimation in an NPP, among which the ANN with Bayesian regularization (i.e., embedded method) gave the most accurate results.
Anomaly Detection Methods
One can attempt to derive first principles–based analytical models to describe the expected nominal or faulty SSC behavior if its underlying physical mechanisms/relationships are well understood. In an engineering system, physics-based models are attractive for three reasons: first, they consider mechanical, material, and operational characteristics explicitly; second, they can be developed and evaluated even before the system has been built and operated; and third, they can be used to understand behavior over a broad range of operational and material conditions (Coble et al., 2012). Unfortunately, it is challenging, time-consuming, and often impossible to model a complex, nonlinear system with first principles and mathematical functions alone (Mirnaghi and Haghighat, 2020). Furthermore, the physical foundation in such models is inevitably diluted by the use of simplifying (sometimes unjustified) assumptions to make up for runtime performance or incomplete domain knowledge (Coble et al., 2012; Zhao et al., 2020a). In contrast, data-driven approaches have shown the potential to characterize system operations and develop system models due to their independence in modeling and sole reliance on system data (Yang and Rizzoni, 2016).
With the development of sensor technologies—which enable routine collection of online data for numerous system variables—various anomaly detection approaches based on multivariate statistics have gained attention. Principal component analysis (PCA) and partial least squares (PLS) are two basic multivariate statistical techniques (Severson et al., 2016), and many applications based on those techniques have been considered for detecting faults (Harrou et al., 2013; Liu et al., 2013; Rato and Reis, 2013; Mnassri et al., 2015; Jia and Zhang, 2016; Li et al., 2016; Jiao et al., 2017; Jiang and Yan, 2018). Once the detection method is selected, a metric for identifying faults is needed. In PCA- and PLS-based methods, Hotelling’s t-squared statistic (Hotelling, 1933)—a generalization of Student’s t-statistic in multivariate hypothesis testing—is widely used to detect anomalies with specific thresholds. The sum of squared prediction error (Box, 1954), also known as the Q statistic, is another metric that denotes the change of the events that are not explained by the model of principal components (Mujica et al., 2011). In the nuclear field, Li et al. (2019a) recently applied an improved PCA method using data pre-processing and false alarm reducing techniques for NPP sensor fault detection, which reduced the false alarms of both t-squared and Q statistics.
Traditional multivariate statistical-based methods have inherent limitations. Calculating monitoring statistics and thresholds of the PCA- or PLS-based methods is made under the assumptions that data from sensors are Gaussian-distributed and linearly correlated and that the process is operated under a single stationary condition (Ge et al., 2013). In practice, most of these assumptions may be violated. Various research efforts using data-driven methods have been developed to relax assumptions in the traditional statistical-based methods. Independent component analysis (ICA), finding both statistically independent and non-Gaussian components, is a reliable alternative for fault detection (Li and Wang, 2002). Stefatos and Ben Hamza (2010) further introduced the dynamic ICA technique, extending the advantages behind ICA to detect faults in a time-correlated environment. Cai and Tian (2014) developed a non-Gaussian process based on robust ICA to alleviate the effect of outliers. Ajami and Daneshvar (2012) showed the validity and effectiveness of ICA for fault detection of a typical turbine system, which are found in an NPP.
The Gaussian mixture model (GMM) is another commonly used technique for non-Gaussian data processing. Yu (2012) proposed a nonlinear kernel GMM-based inferential monitoring approach for fault detection, which projected data from a raw measurement space into a high-dimensional kernel space so that the GMM could be estimated in the feature space satisfying multivariate Gaussianity. Karami and Wang (2018) proposed an adaptive GMM for automatic fault detection in nonlinear systems. Ma et al. (2019) presented a nuclear application by using a GMM-based early fault detection method on 30 sets of real data from reciprocating compressors containing three fault types.
More recently, SVM variants—which do not require the data to be Gaussian—have emerged. Liu and Zio (2018) developed a k-nearest neighbors–based fuzzy SVM to reduce the computational burden and tackle the issue of data imbalance and outliers. Several applications exist for fault detection in NPP assets using SVM-based models (Jamil et al., 2018; Lin and Wu, 2019; Meng et al., 2020).
Fault Diagnostics
Within the overarching area of PHM, fault diagnostics begins after a fault has been detected during the CM process. Diagnostics is further divided into fault isolation, which seeks to identify the piece of equipment or component from which the fault originates, and fault identification, which determines the cause of the fault. Logically, these two subtasks of fault diagnostics are often performed as a single analysis. The analysis is based upon fault symptoms, which primarily take the form of available features or signatures of the fault, obtained in the form of sensed data and measurements. A common classification scheme for diagnostics problems is by modeling method, in which the problem is approached using either a model-based or a data-driven method. This is not a completely clear distinction, though, as some overlap can exist between the two approaches, and various hybrid approaches can be developed. One specific area of overlap is in the use of rule-based expert systems for fault diagnosis. These expert systems rely on “if-then” rules to diagnose a system’s state given its fault symptoms. It will be seen that the development of “if-then” rules can be done by either model-based or data-driven methods.
This review places emphasis on developments in data-driven methods for PHM. However, a brief review of advances in model-based methods is still deemed beneficial to the reader interested in fault diagnostics or PHM in general. As such, the following subsections will survey model-based methods first; then rule-based expert systems, namely those which rely on fuzzy rule bases; and finally, data-driven methods. Additionally, the interested reader can refer to Li et al. (2020) for a second review of diagnostic methods.
Model-Based Methods
According to Yang (2004), who presented a review of both model-based and data-driven methods, common model-based methods include the use of observers or statistical filters, checks of the parity between plant models and sensor outputs, generation of residuals in the frequency domain, use of causal graphical models (such as signed directed graphs and fault trees), and approaches based on qualitative physics (such as qualitative simulation and qualitative process theory). A common classification scheme for these methods is that filtering, parity, and frequency approaches are grouped as quantitative methods, and that graphical models and qualitative physics are considered qualitative methods.
In the area of filtering-based methods, Gautam et al. (2019) used an extended Kalman filter for fault identification and performed fault isolation for single and simultaneous multiple-sensor faults in an NPP with a recursive least squares estimate. An advancement to the parity space method was performed by Cho and Jiang (2018) for nuclear applications, in which Fisher discriminant analysis (FDA) was used to address the issue where the number of fault classes exceeds the total independent residual signatures. Lee and Shin (2018) proposed a method using time-frequency domain reflectometry and k-means clustering to determine the fault location and faulty line in a multi-core I&C cable system to assure the safety and reliability of NPP operation. Advances in qualitative causal graphs for NPP asset fault diagnosis include those on signed directed graphs (Liu et al., 2016) and dynamic uncertain causality graphs (Zhao et al., 2017a).
Rule-Based Methods
Rule-based methods operate by firing specific “if-then” rules to determine the consequence associated with a measured/detected fault symptom. Rule bases have traditionally been developed using expert judgment and prior knowledge about the system. Although a system based on engineering knowledge may be attractive, issues in classical rule-based systems can include rule bases growing to unmanageable size to describe an increasing number of scenarios and the potential for a rule-based system to fail when it encounters a situation for which there is currently no rule (Coble et al., 2012). Another large difficulty encountered by the standard rule-based method is how to operate when there is not complete certainty as to which rule should be activated given the measured symptoms. This situation typically arises when the symptom cannot be simply classified into a single qualitative category, such as “low” or “high.” The most common means of handling this uncertainty is by using a fuzzy rule–based system. Similar methods have also received some attention, such as the use of confidence degrees (Deng et al., 2017) or the development of a belief rule-based expert system (Xu et al., 2017). However, fuzzy rule–based fault diagnostic tools are still the most prominently used method in the literature to deal with uncertainty.
Fuzzy rule bases, and the fuzzy logic in general on which they operate, act as a nonlinear mapping between inputs and outputs by means of determining the degree of membership to which “crisp” inputs belong to “fuzzy” qualitative states and using the fuzzy states to determine the consequence of the given inputs. Fuzzy rule bases have found application for fault diagnosis in various disciplines and numerous components—many of which are found in NPPs—including induction motors (Shetgaonkar, 2017), other standard rotating machinery (Da Silva et al., 2017), spur gears (Krishnakumari et al., 2017), bearings (Berredjem and Benidir, 2018), power transformers (Husain, 2018), diesel generators (Nain and Varde, 2013), distributed sensor networks (Bhajantri, 2018), and high-power lithium-ion batteries (Wu et al., 2017).
Despite their advantages over traditional rule-based fault diagnosis, fuzzy rule–based systems are the subject of ongoing research to improve their performance. Work by Yan et al. (2019) and Rodríguez Ramos et al. (2019) both addressed identifying multiple faults using fuzzy rule–based systems. Du et al. (2020) proposed a self-organizing fuzzy logic classifier based on the harmonic mean difference for application in bearing fault diagnosis. That approach in particular is an example of a rule-based method also potentially being classed as a data-driven method because measured fault features were used to train a fuzzy classifier. As a means of further characterizing the uncertainty present in signals and measurements, Wang et al. (2019a) introduced an interval-valued fuzzy spiking neural P system,8 also demonstrated on an example case with the presence of multiple faults.
Data-Driven Methods
Data-driven methods generally rely on a large amount of process data, typically historical, to develop models and reasoning methods (Yang, 2004). Methods traditionally classed as data-driven methods comprise ANNs–discriminative methods (for traditional neural networks), models based on Bayesian statistics or utilizing Bayesian networks (BNs)—generative methods, SVMs–discriminative, and PCA–generative (if unsupervised) or discriminative (supervised). Often, combinations of these methods are used. In addition, many more methods considered as data-driven exist, which have seen less applications in the nuclear field than those presented in this section.
The ANNs constitute a large subject area in data-driven methods for fault diagnostics, and research of ANNs is an extensive field unto itself due to the vast number of techniques and types of neural networks in use. Lin et al. (2021) developed a nearly autonomous management and control (NAMAC) system for advanced reactors and proposed to apply a feed-forward neural network (FFNN) model for NAMAC’s diagnostic digital twin (DT) layer; Gomes and Canedo Medeiros (2015) used a network of Gaussian radial basis functions (RBFs) to identify accidents in an NPP; Banerjee et al. (2020) demonstrated use of an ANN to identify nuclear reactor sensor and actuator faults in the presence of a proportional–integral–derivative controller; Ayo-Imoru and Cilliers (2018) implemented an ANN while using a plant simulator as a dynamic reference. A common theme in the literature sees ANNs working in tandem with some other technique to transform sensory data into a form usable by the ANN. As examples, Messai et al. (2015) and Tagaris et al. (2019) both used data from wavelet transformations; Lee et al. (2021) transformed the number of plant state variables into a 2D image and used a convolutional neural network (CNN) to process the image as a means of diagnosing abnormal states; Saeed et al. (2020) implemented a long short-term memory (LSTM) network and CNN after performing PCA; and Ayodeji et al. (2018) tested the effectiveness of an RBF network and an Elman neural network (ENN) after using PCA to perform noise filtering for NPP fault diagnosis. Another common trend, both in the literature surrounding ANNs for diagnostics and AI as a whole, is the increased interest in utilizing DL methods. In application to NPP fault diagnostics, DL architectures have been applied by Ahmed et al. (2017), Mandal et al. (2017), Peng et al. (2018a), and Kim et al. (2019). Outside of the nuclear industry, Yu et al. (2018) applied DL for fault diagnosis in wind turbines, and Ren et al. (2019) developed a DL diagnoser in autonomous vehicles.
In the current state of the industry, the application of BNs to diagnostics is sparser than those of ANNs. Unlike black-box data-driven methods such as ANNs, the BN approach offers transparent model interpretability, reasoning under uncertainty, and graphical representation capability to emulate the target SSC’s physical behavior (Zhao and Golay, 2020). However, constructing the BN knowledge base is a cumbersome and time-consuming process, and problems using BNs can become intractable for complex scenarios. Wu et al. (2018a) developed a BN framework for fault diagnosis in NPPs with multi-source sensor nodes, and Zhao et al. (2020b) proposed a method to diagnose operational and on-demand failures using dynamic BNs (DBNs). A large body of work on using BNs as fault diagnosers also exists outside the nuclear field. Cai et al. (2017) provided a detailed review of BNs for application in fault diagnosis. Wang et al. (2018) proposed an improved BN method by determining the network structure with a hybrid technique of process knowledge and data-driven correlation, which was validated with the Tennessee Eastman Process open-source benchmark (Downs and Vogel, 1993). Areas where BNs have seen application, in addition to those discussed in Cai et al. (2017), include the general case of industrial processes (Yu and Zhao, 2019), hydroelectric generation systems (Xu et al., 2019), and ground-source heat pumps (Cai et al., 2014). Lastly, as a method used in combination with ANNs, Bayesian statistics was used in Tolo et al. (2019) as a means of connecting a set of neural network architectures for early accident detection in NPPs.
Research efforts directed toward SVMs have been primarily focused on improving the optimization of SVM parameters and then applying the SVM to the problem of fault diagnostics. In the nuclear field, Wang et al. (2019b) developed an improved particle swarm optimization, and Zhang et al. (2015b) used a hybrid of the bare bones particle swarm optimization and differential evolution. Beyond simply developing a better means of parameter optimization, Wang et al. (2021) introduced a hybrid least squares SVM method for fault diagnosis in NPPs. Another approach beyond optimization was to separately train an ensemble of SVMs and combine them after training (Ayodeji and Liu, 2018).
Developments involving PCA are mostly fault diagnosers that utilize PCA combined with another tool, as was observed during the discussion of ANNs. An example of using PCA for fault diagnosis without combination with another major method is that of Li et al. (2018a), which presented an optimized PCA method for fault identification and reconstruction of NPP sensors. An approach fundamental to PCA, however, was the development of statistical methods to reduce the number of false alarms raised by PCA (Li et al., 2018b). Approaches having seen fusion with PCA for fault diagnosis in NPPs include FDA (Jamil et al., 2016), conditional Gaussian networks (Atoui et al., 2015), multilevel flow modeling (Peng et al., 2018b), ENNs (Liu et al., 2017), and SVMs (Xin et al., 2019). Additionally, Wang et al. (2017) used a semiparametric PCA in combination with a BN.
Prognostics
Prognostics is one of the major tasks in PHM as its results are directly used to support proactive decision-making for maintenance practices. The prognostics module is typically defined as the process of predicting the remaining time before the equipment can no longer perform a particular function (i.e., RUL) (Atamuradov et al., 2017). Prognostic calculations cannot be done in isolation and depend largely on the stages of monitoring, detection, and diagnostics: the accuracy of these stages will all affect RUL estimation. It is desirable to develop generalizable prognostic methods that can accurately predict the future equipment state given a set of measurements correlated to the equipment’s current state (Ramuhalli et al., 2020). An appropriate estimate of the equipment’s RUL can improve overall plant performance and reduce costs by optimizing O&M activities. Therefore, prognostics is seen as one of the most beneficial aspects of PHM (Hess, 2002).
Paradoxically, prognostics is an underdeveloped element of PHM systems (Vogl et al., 2019), especially in the nuclear industry (Coble et al., 2015; Ayo-Imoru and Cilliers, 2018). Unlike fault detection and diagnostics, the prognostic technology is just emerging and often is deemed immature due to lack of uncertainty calculations, method verification and validation, and risk assessment for PHM system development (Saxena et al., 2010). Although many approaches to prognostics have been proposed in the literature, the state of practice is mainly at the research level and much of the published work has been exploratory. There is no universally accepted methodology for all prognostic problems (Lee et al., 2011; Coble et al., 2012). A variety of models have been developed for application to specific situations or specific classes of components. As such, prognostic algorithms can be categorized according to different criteria. Based on the recent publications (Atamuradov et al., 2017; Lei et al., 2018; Taheri et al., 2019; Vogl et al., 2019; Baur et al., 2020; Bektas et al., 2020; Ramuhalli et al., 2020) that contain a comprehensive review of prognostics, these algorithms can be loosely divided into four categories according to their basic techniques or methodologies: physics-based methods, knowledge-based methods, data-driven methods, and hybrid methods.
Physics-Based Methods
Physics-based prognostic methods attempt to describe the evolving SSC degradation process based on a comprehensive mathematical model—usually in the form of a series of ordinary or partial differential equations—that represents the underlying physics of failure and encodes the first-principles input-output relationship. The derived mathematical model is combined with CM data to identify model parameters, which are then used to predict the future evolution of SSC health state. A commonly illustrated physics-based method example in the literature is a crack growth model for which Paris’ law (also known as the Paris–Erdogan equation) (Paris and Erdogan, 1963) or the Forman equation (Forman, 1972) is used to relate the growth rate of a fatigue crack to the stress intensity factor and the number of fatigue cycles. Some other examples include prediction of bearing deterioration, turbine creep evolution, pipeline tube degradation, battery life, and gearbox failure (Qiu et al., 2002; Liao and Kottig, 2014; Hu et al., 2016).
When the failure mechanism is well known and correctly captured, a physics-based prognostic model should yield highly robust and accurate RUL prediction for a specific type of component and require less data for tuning (Baur et al., 2020). Unfortunately, the underlying physical processes leading to failure are often not completely understood or cannot be explicitly modeled. In this case, simplifying assumptions and estimations must be made to facilitate model development, raising skepticism about the model’s applicability to real-world engineering systems (An et al., 2015; Coble et al., 2015). Due to their nature of being component-specific, physics-based methods can hardly be reconfigured to fit alternative domains, and most of them are only applicable at the component or subsystem level (Baur et al., 2020; Bektas et al., 2020). When applied to system-level prognostic problems or when multiple failure modes need to be represented (which is the case for a typical SSC in a nuclear facility), the model complexity and associated computational cost may become prohibitive for online analysis and decision-making. For these reasons, Coble et al. (2015) concluded that physics-based methods would be preferable for high-cost, high-risk equipment, such as electronic components in which failure data needed to develop empirical methods might not exist.
Knowledge-Based Methods
Knowledge-based (also known as experience-based or rule-based) prognostic methods are solely built upon expert knowledge. Such methods do not rely on a physical model of the system. Their implementation is relatively simple; however, they are applicable only in cases where expert knowledge exists to mimic human-like representation and reasoning with algorithm families that employ expert systems or fuzzy logics.
Analogous to rule-based diagnostic methods (see “Rule-Based Methods” Section), expert systems for prognostics aim to translate explicit knowledge from experts into human-coded “if-then” rules that closely resemble the way a domain specialist solves the same problem (Liao and Kottig, 2014). They do not perform well when a huge number of rules are needed and cannot handle new situations that are not explicitly coded. Compared with expert systems, fuzzy logic–based prognostic methods are more robust and can handle the uncertainty intrinsic to expert knowledge (Jardine et al., 2006). For complex systems and in the presence of high-volume data, fuzzy logics are typically used in conjunction with data-driven approaches—such as ANN to create a hybrid neuro-fuzzy (NF) model (Lei et al., 2018)—for systematized dimensionality reduction and membership function optimization. The stand-alone knowledge-based methods have been much less studied or recommended by recent publications than the other method categories (Atamuradov et al., 2017) due to their inherent limitations.
The similarity-based prognostics is an alternative knowledge-based approach—such as the one proposed by Liu et al. (2019) for the RUL prediction of a gas turbine—that removes the requirements to model qualitative knowledge from domain experts. Although this approach is sometimes classified under data-driven methods, it actually follows the rule-based modeling philosophy of similarity evaluation between a monitored case and a library of previously known failures (Taheri et al., 2019; Bektas et al., 2020), which does not give enough insight into the current or future condition of the specific SSC in question.
Data-Driven Methods
Data-driven prognostic methods directly use CM data for the target SSC and do not incorporate first-principles information or expert knowledge. They rely on trends within the observed data to construct mathematical models to estimate future states of the monitored equipment. As will be further elaborated in this section, the mathematical approaches range from conventional statistical methods to advanced ML and DL techniques. In data-driven methods, no mechanism or input-output relationship needs to be known a priori to produce acceptable results, and the method development/implementation cost is relatively low (Diez-Olivan et al., 2019). Therefore, these methods are highly flexible and can be deployed at any level (component, subsystem, or system level) of the physical asset, which is of particular interest to large, complex systems (Ramuhalli et al., 2020; Sun et al., 2010). As shown in Figure 4, statistical-based and ML/DL-based data-driven methods have attracted most of the research attention in machinery prognostics. However, prognostic models that use a data-driven approach usually require large amounts of data covering a broad range of conditions, including run-to-failure data for degradation models. Availability of run-to-failure data for a particular SSC can be a key challenge (Sutharssan et al., 2015), which is the case of safety-critical systems in NPPs (Coble et al., 2015). The performance and confidence level of RUL predictions are bound to the quantity and quality of available data that are used to infer model parameters and to determine failure thresholds. Furthermore, data-driven methods cannot extrapolate beyond the domain spanned by the training data (Ramuhalli et al., 2020).
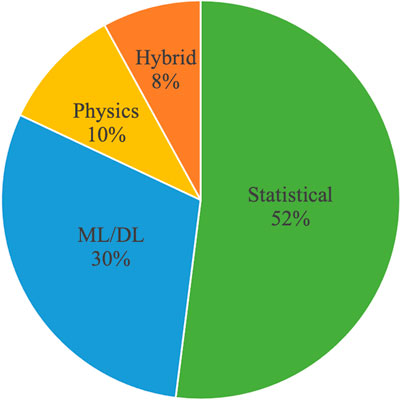
FIGURE 4. Distribution of method categories in 274 recently published papers on machinery health prognostics [modified from Lei et al. (2018)].
Statistical-Based Prognostics
Statistical-based prognostic methods, also known as empirical prognostic methods, are a grey-box approach that treats asset degradation as a stochastic process subject to different sources of variability and uncertainty (Baur et al., 2020). In statistical methods, RUL is a random variable whose probability density function is determined based on empirical data. Distinguished by its data-driven nature and ability to incorporate the uncertainty of the degradation process, this method category has been heavily focused upon in the literature, as illustrated in Figure 4 for the field of machinery prognostics. Multiple review papers (Si et al., 2011; Ye and Xie, 2015; Lei et al., 2018; Taheri et al., 2019; Baur et al., 2020; Bektas et al., 2020) have surveyed statistical-based models systematically and have included their advancements in recent years. To unify apparently confusing terminologies used by different authors while minimizing repetition, a summary is provided in this section.
Statistical-based prognostics can be generally classified into two subcategories. Models in the first subcategory are based upon time-series CM data that directly describe the underlying degradation process of the monitored SSC. Both regression- and Markovian-based models fall into this subcategory. In regression-based models, forecasting of time-series data is achieved by using auto-regressive moving average processes, which assume that the future state of the target SSC is linearly dependent of both past observations and normally distributed random noise. These models are easy to implement with low computational cost, but their performance is heavily affected by the trend information of historical observations, which may be unreliable during incipient failure stage and for long-term forecasts (Baur et al., 2020). Recent examples of using regression-based prognostic models include Qian et al. (2014) for bearing wear-out, Barraza-Barraza et al. (2017) for crack growth in aluminum plates, Nguyen et al. (2018) for NPP steam generator degradation, and Mei et al. (2020) for shear building structural damage. In Markovian-based models, the degradation process is assumed to transform within a finite state space that satisfies the Markov (or memoryless) property. With a well-established theoretical basis to support these models, the Markovian approach was first introduced into the field of prognostics by Kharoufeh (2003). It was later refined by Kharoufeh and Cox (2005) and Kharoufeh et al. (2010). This version of Markovian models was not widely adopted by the PHM community because all the health states would need to be observed directly. Moreover, the memoryless assumption may not be valid for some real degradation processes, and a large volume of data or empirical knowledge is typically required for constructing the state transition probability matrix.
Models in the second subcategory rely on partially observed state processes and indirect CM data (i.e., data that can only indirectly indicate the underlying health state of the monitored SSC, such as vibration data). Stochastic filtering-based methods, which are based on the Bayes’ theorem, fall into this subcategory. Built upon DBNs, Kalman filter and particle filter are two of the most common types of filtering algorithms. The basic Kalman filter algorithm is designed for linear Gaussian problems, and some of its enhanced versions have been proposed; the particle filter algorithm is a sequential Monte Carlo method and is a better choice in nonlinear, non-Gaussian systems. Due to their ability to characterize the future uncertainty of degradation processes by updating the probabilistic state estimation from online measurements, both filtering methods have seen many applications in machinery RUL prediction (Lei et al., 2018) and were introduced by Ramuhalli et al. (2010) for prognostics of NPP components. Similar to, yet simpler than the filtering-based models, hidden Markov models (Ghahramani, 2001) are extensions of the standard Markovian approach to incorporate unobservable health states. The hidden Markov models and their variants [e.g., hidden semi-Markov models (Yu, 2010)] have been applied to the PHM framework since the beginning of this century (Baur et al., 2020). However, their capabilities are still limited by the memoryless assumption.
Machine Learning–Based Prognostics
ML-based prognostic methods attempt to learn degradation patterns and predict RUL directly from available observations (or extracted features) using ML or DL techniques. Numerous opportunities have arisen from the continuously fast-growing trends of AI and ML to effectively address the problems of prognostics, especially those in complex multidimensional, nonlinear systems with large amounts of training data representative of true data range and variability. No prior physical understanding of the analyzed SSC is required in ML-based methods. However, as a black-box approach, the results are hard to interpret due to their lack of transparency. The ML-based methods generally provide point estimates of RUL instead of a probabilistic treatment unless additional uncertainty quantification—usually with Bayesian inference methods—is performed. A more fundamental comparison of statistical- and ML-based methods can be found in Bzdok et al. (2018). A variety of ML algorithms have been used for prognostics, which can be loosely grouped into variants of ANN, Gaussian process regression (GPR), and SVM.
The ANNs are the most common modeling techniques in data-driven methods for prognostics (Bektas et al., 2020), just like for fault diagnostics (see “Data-Driven Methods” Section). Comprehensive surveys of ANN architectures—in the context of DL—and their recent applications in machinery prognostics have been presented by Rezaeianjouybari and Shang (2020), Khan and Yairi (2018), and Wang et al. (2020b). Among the multiple types of ANNs in use, FFNNs and recurrent neural networks (RNNs) are the most popular. The FFNNs are the simplest form of ANN and have been mainly used to learn the relationship between the health index9 and RUL (Lei et al., 2018). Lin et al. (2021) recently implemented FFNNs into the prognostic DT of their NAMAC system for advanced reactors. The RNNs, descendants of FFNN, are distinguished by their ability to handle time-series data explicitly. Standard RNNs suffer from vanishing and exploding gradients when learning long-term temporal dependencies; the gated recurrent unit (GRU) and LSTM networks are RNN variants to remedy that problem. Generally, GRUs are computationally less expensive and better suited for smaller data sets, whereas LSTMs work better with large data sets (Rezaeianjouybari and Shang, 2020). A limited number of studies in the literature have applied GRU for prognostic tasks, such as Zhao et al. (2018) for milling machine cutter tool wear prediction, Li et al. (2019b) for rolling bearing RUL, and Chen et al. (2019) for a nonlinear degradation process using the US National Aeronautics and Space Administration’s commercial modular aero-propulsion system simulation (C-MAPSS) turbofan engine data. LSTM-based networks have gained greater attention in applications of RUL prediction. Some recent studies include Ramuhalli et al. (2020) using NPP asset data from the feedwater and condensate system (FWCS) of a boiling water reactor (BWR); Zhao et al. (2017b) using a convolutional bidirectional LSTM and raw sensory data from high-speed milling machine cutters for a real-life tool wear test; Zhang et al. (2018), Wu et al. (2018b), and Elsheikh et al. (2019) using different variants of LSTM on the C-MAPSS data set; Shi and Chehade (2021) using a novel dual-LSTM framework for both change point detection and RUL prediction on the same C-MAPSS data; and Bampoula et al. (2021) using LSTM autoencoders to estimate RUL in a cyber-physical production system. Besides the above two commonly used ANNs, several other variants—such as wavelet neural network (Javed et al., 2014), CNN variants (Wang et al., 2019c; Zhu et al., 2019), generative adversarial network (Khan et al., 2018), and reinforcement learning (Kozjek et al., 2020)—can be found in the literature of prognostics.
The GPR models build upon Gaussian processes—cumulative damage processes of random variables with joint multivariate Gaussian distributions—to predict future health states. In contrast to ANNs, this approach is adaptable to both small- and large-size data sets, although it often suffers from high complexity in terms of computation and storage (Rasmussen, 2004). As elaborated in the modules of fault detection (“Condition Monitoring and Fault Detection” Section) and diagnostics (“Data-Driven Methods” Section), SVMs are well-established supervised learning tools based on the core concept of support vectors. Different SVM variants have been applied to the machinery RUL prediction (Lei et al., 2018). In the nuclear domain, Liu et al. (2015) proposed a dynamic-weighted probabilistic SVM model to evaluate fault scenarios in the reactor coolant pump of a typical pressurized water reactor, and Ramuhalli et al. (2020) applied SVM with both a linear kernel and an optimized Gaussian kernel on a BWR FWCS data source. Compared with ANNs, SVM-based models usually perform better on small data sets and can guarantee a unique solution (i.e., global minimum) to a given problem. However, their performance is strongly correlated with the selected kernel functions.
Hybrid Methods
The physics-based, knowledge-based, and data-driven prognostic methods each have their own strengths and limitations. While appropriate method selection depends on knowledge of the system behavior and available data, a hybrid or fusion approach attempts to integrate the advantages of different method types for improved RUL prediction results. Additionally, in the real world, no single method is deemed adequate to account for all the possible faults and failure modes of an analyzed system (Baur et al., 2020; Venkatasubramanian, 2005). As shown in Figure 4, this area of research is still at its early development stage. The hybrid methods can consist of any combination of the previously described approaches. Of special interest is the ensemble of physics- and ML/DL-based techniques where both physics-of-failure knowledge and experimental data can be properly leveraged (Dourado and Viana, 2020). In this way, the combined approach fosters a physical interpretation of the input-output relationship instead of a black-box treatment while not requiring as accurate physical understanding or large-size data as stand-alone counterparts would do (Zhao et al., 2020a). Another popular direction is to develop hybrid prognostic tools under the Bayesian framework (e.g., Kalman filter and particle filter) because of their robustness and ability to reason under uncertainty. This direction has been the subject of several research studies and has been applied to various applications like rotating machinery, batteries, and electrolytic capacitors (Taheri et al., 2019).
Some hybrid models use one method to predict health state and another one to estimate RUL, while other models attempt to apply both method types to RUL forecasting (Ramuhalli et al., 2020). The selection of the actual model and method is usually driven by the problem and specific to the application. As an example in nuclear systems, Gurgen et al. (2020) recently proposed a physics-guided RNN (with LSTM blocks) prognostic model within the NAMAC system to predict the evolution of fuel centerline temperature in loss of flow transient conditions and demonstrated its superior performance over pure data-driven prognosis. In other fields, research related to the hybrid approach has been much more active (Liao and Kottig, 2014; Atamuradov et al., 2017). Goebel et al. (2006) combined a physics-based model of fault initiation and an empirical model of condition-based fault propagation rate to estimate RUL of avionic roller bearings. Liu et al. (2012) developed a hybrid method to improve the accuracy and transparency of long-horizon lithium-ion battery health state forecasting by leveraging particle filter and ANN predictors (FFNN, NF and recurrent NF). Eker et al. (2019) presented a unified approach integrating the short-term prediction of a physics-based model with the longer-term projection of a data-driven model and validated with run-to-failure observations for crack growth and filter clogging. Yucesan and Viana (2020) proposed a physics-informed neural network model that merged physics- and data-driven layers within a deep neural network to predict main bearing failure in wind turbines.
Decision-Making
Once the current and postulated future health states of a monitored SSC are determined based on CM data and diagnostic/prognostic modeling, it is of crucial importance to be able to act in a timely and correct fashion on possible (incipient) faults/failures before they progress to becoming emergencies. Therefore, decision-making is deemed an indispensable module in the full PHM suite. In this context, the process of decision-making refers to using outputs from the previous modules—failure analysis and probability of failure (POF) estimate from diagnostics, RUL prediction from prognostics—to inform O&M planning and the selection of optimal maintenance action among several alternative options to be executed for the most beneficial operational performance. This process can be conducted by human labors with different operator decision support levels, or ultimately through autonomy-enabled technologies. If properly implemented, this module will play an impactful and beneficial role in asset integrity management as well as planning for O&M activities and staffing levels.
While the study of decision theories has a rich history, autonomy—i.e., operation without relying on human intervention—is in large part an advancement that appeared with the invention of computers and programmable devices that could perform fairly complex computations. Significant technological advances in controls and autonomy have been demonstrated in robotics, aerospace, unmanned aerial vehicles, and self-driving automobiles. However, autonomous control has not been extensively studied for any operating NPP or any new reactor concept (Wood et al., 2017). The nuclear industry lags far behind some other industries (such as avionics and electronics) in transferring the current human-based roles and responsibilities to cutting-edge machines, systems, and controls. To date, NPP equipment surveillance, diagnostics, and prognostics have been mostly used for offline asset management and modest decision support, but those technologies are not being fully leveraged for intelligent, optimal O&M planning and control. To achieve the desired operational efficiency with a reduced staffing burden, autonomous decision-making capabilities must be developed and demonstrated in the nuclear power context.
Given the current status and apparent gaps for NPPs, this section first provides a summary of general approaches used in decision-making, all of which are driven by data to a certain extent. [See Cetiner et al. (2014) and Cetiner and Ramuhalli (2019) for more detailed surveys]. A pioneering study in the nuclear domain by Ramuhalli et al. (2017) is then briefly presented to showcase the ability to integrate diagnostics and prognostics results with supervisory control systems for making risk-informed autonomous decisions that utilize real-time information on component conditions.
Decision-Making Methods
Statistical Decision Theory
Statistical decision theory is concerned with making decisions based on statistical knowledge, which sheds light on the uncertainties involved in the decision problem. The field of classical statistics is directed toward using sample information arising from statistical investigation to make inferences about the use of the data; in contrast, statistical decision theory attempts to combine sample information with other aspects of the problem to make the best decision. In addition to sample information, two other types of information are typically relevant. The first is the knowledge of possible consequences of decisions. Often this knowledge can be quantified by determining the loss that would be incurred for each possible decision and for various possible values of uncertainties. The second type, prior information, generally comes from past experience about similar situations involving similar uncertainties. The approach to statistics that seeks to utilize prior information is called Bayesian analysis. The Bayesian approach is one of the most commonly referred mathematical methods that are exclusively used in decision-making processes in a wide range of applications. Recent examples of applying Bayesian analysis for decision support in nuclear systems are Cai and Golay (2021), who proposed a DBN-based framework capable of analyzing interactions between system status and human activities for the 2011 Fukushima accident scenarios; and Kim et al. (2021), who coupled functional modeling with DBN to study a station blackout scenario leading to a seal loss of coolant accident in an NPP.
Rule-Based Decision-Making
A rule-based model 1) identifies the system state, 2) associates the state with a task, and 3) accesses stored rules to perform the task. Plant operating procedures (OPs) are essentially rule-based decision modules executed by human operators. OPs are developed for normal operation to ensure that the plant is run within the operational limits and conditions and to provide instructions for the safe conduct of all modes of normal operation. For abnormal conditions and design-basis accidents, either event-based or symptom-based procedures are created. A means of automating the plant procedural system is to implement the rules through decision tables, which associate conditions with actions to perform. In a recent paper by Hanna et al. (2020), an answer set programming representation of an NPP was presented, which included rules encoding the plant behavior for fast procedure lookup.
Utility Theory
Economists developed utility theory to explain and predict human decision-making under risk and uncertainty. The fundamental assumption underlying utility theory is that of a rational decision maker who always chooses the alternative for which the expected value of the utility is maximized. Built into this assumption is a further supposition that a code of rationality is accepted and utilized by human decision makers, making it possible to construct a mathematical representation that allows the prediction of human behavior. Utility theory can serve as a foundational building block for a decision-making system intended for real-time autonomous control. Given a collection of seemingly viable alternative solutions, implementation risks determined for each alternative can be compared to find a minimum risk solution. Independent loss and gain functions related to plant OPs or other decision strategies can be formulated and represented as nonlinear relationships. An exemplary implementation of the utility theory for NPPs can be found in Yildiz (2003), where an influence diagram-based advisory model was proposed to offer decision support to the plant personnel.
Markov Decision Process
For sequential decision problems in stochastic environments, the same principle of maximum expected utility still applies, but optimal decision-making will require reasoning about future sequences of actions and observations. Markov decision processes (MDPs) provide a mathematical framework for modeling decision-making in situations where outcomes are partly random and partly under the control of a decision maker. MDPs have been used successfully in a wide range of autonomous control problems—for autonomous driving (Thrun et al., 2006; Brechtel et al., 2014) in particular—and typically solve an optimization problem using dynamic programming (DP) for selecting the right decision. A partially observable MDP (POMDP) is a generalization of an MDP that models a decision process in which it is assumed that an MDP represents the system dynamics but not all states are observable. Instead, the measurements received by the model are incomplete and usually noisy predictions. Therefore, the model must estimate a posterior distribution over a possible state space. POMDPs compute a value function over a belief space. A belief is a function of an entire probability distribution. An exact solution to a POMDP yields the optimal action for each possible belief over the state space, maximizing the value function. However, this maximization procedure requires an iterative algorithm that is far from practical. For any reasonable number of states, sensors, and actuators, the complexity of the value function is prohibitive. One recommended solution to that challenge is the use of differential DP—a DP-based optimal control algorithm of the trajectory optimization class—as it optimizes only over the unconstrained control space. A promising implementation of POMDP can be found in Kochenderfer (2015) for an automated airborne collision avoidance system, leading to significant improvements to safety and operational performance of aircraft.
Discrete Event Models
Many artificial devices and systems and some natural systems demonstrate only discrete values or outcomes. These types of systems are best described as discrete event systems (DESs). The opening and closing of valves or commencing a pump startup process are examples of discrete event processes in an NPP. The processes are typically tied to OPs, and plant operators handle their controls. DESs satisfy the properties that 1) the state-space is a discrete set and 2) the state-transition mechanism is event-driven. Time in such systems is not an appropriate independent variable, and conventional differential equation approaches such as modern control theory do not apply to them. The DESs are typically modeled by finite state automata or Petri nets. Those models use a defined state-transition structure to describe the possible events in each state of the system, and they differ in how they represent state information. A detailed comparison between a finite state automation and a Petri net approach can be found in Aubry et al. (2016). Decision-making models for DESs have an established industrial track record; applications range from robotics to self-driving cars (Costelha and Lima, 2012; Badue et al., 2021).
From Enhanced Risk Monitors to Supervisory Control: A Pioneering Study
In the nuclear industry, successful implementations of autonomous controls seek to address both the probabilistic and deterministic aspects of decision-making. In other words, a risk-informed decision-making framework is needed for the plant control systems to maintain system variables within prescribed operating ranges. As shown in Figure 5, the probabilistic portion of the decision-making engine identifies decision options and provides the likelihood of success for each option given the status of the plant/systems and component health. It captures the uncertainties associated with sensors and those that arise from modeling assumptions used in inferences. On the other hand, the deterministic portion further evaluates the identified alternative success paths and generates a single solution that represents the best operational strategy. The evaluation metrics determine the cost function for finding the optimal decision, and additional constraints—such as regulatory rules and operating guidelines—can be enforced in the deterministic assessment phase.
A study on risk-informed decision-making using real-time equipment condition information was recently performed by Ramuhalli et al., 2017 (Liu et al., 2012), in which the enhanced risk monitor (ERM) methodology (Ramuhalli et al., 2014) was integrated with a plant supervisory control system (SCS) framework (Cetiner et al., 2016). The ERM methodology interprets components of interest based on sensory data and streamlines condition assessment, diagnostics, prognostics, and risk monitors that expand on probabilistic risk assessment (PRA) by incorporating the dynamically changing plant configuration. The functionality of ERMs can be further augmented to include uncertainty bounds and O&M-based risk metrics (in contrast to the traditional safety-based metrics, such as core damage frequency). As a proactive asset management philosophy, the ERM methodology can offer greater situational awareness to plant supervisory control and O&M planning routines.
The SCS framework is specifically designed to address the need for plant coordination/control that accounts for component degradation in its decision-making. Focused upon non-safety systems, the SCS integrates information from multiple sources, utilizes predefined success criteria, and evaluates plant control actions to ensure that the plant operates within a defined operational envelope. Using RUL and POF information from ERMs as inputs, the decision-making module in the integrated ERM-SCS is invoked only if any of the RUL values is estimated to be larger than the time to the next outage. Decision-making involves two steps: the probabilistic assessment using data acquired from individual ERM terminals to update the event tree/fault tree models with real-time failure probability estimations, and the deterministic assessment based on the multi-attribute utility theory to rank action paths that will not trip the plant’s safety system and generate an optimal solution for the operational strategy of the plant. The solution is verified using a lower-order model of the plant to ensure that the recomputed RULs of all components fall within the prescribed criteria.
Research Needs for Deployment of Prognostics and Health Management in Nuclear Power Plants
Implementation of PHM technologies is a key need for improving the safety and economics of NPPs moving forward with both LTO and new builds. “Prognostics and Health Management Framework and Modeling Approaches” Section has provided a method-centric survey of research in PHM since the 2015 review (Coble et al., 2015), and it can be seen that most advances and corresponding modeling approaches have originated in non-nuclear applications. As such, those developments represent the current state of the art in PHM technologies and methods. Adapting those developments to the nuclear industry may face additional challenges due to the unique operational framework and licensing requirements of NPPs. Additionally, further investigation is needed in several general areas to bring PHM from the research arena to commercial deployment. This section attempts to identify the overarching research needs and technical gaps which still must be addressed to support the development and deployment of PHM in nuclear power generation, including challenges specific to NPPs, a unifying framework for connecting PHM and PRA, verification and validation of PHM models, as well as efforts to evaluate uncertainties and their propagation.
Prognostics and Health Management Feasibility and Challenges Specific to Nuclear Power Plants
Even though significant research has been undertaken to develop PHM—notably, fault detection and diagnostics—for nuclear applications, very limited pilot applications and implementations have resulted in success outside a laboratory setting (Hashemian, 2011; Ramuhalli et al., 2016). The vast majority of modeling approaches described in “Prognostics and Health Management Framework and Modeling Approaches” Section should be widely applicable to nuclear assets in theory; however, implementing PHM in NPPs is quite different from implementing it in other industries because the nuclear industry has been facing a series of specific challenges.
• Operational compatibility: As introduced in “US NPP Monitoring and Maintenance: Historical Approach and Motivations for Prognostics and Health Management” Section, PHM technologies in the nuclear industry typically differentiate between active and passive SSCs. PHM for active SSCs is necessary to support the day-to-day application of O&M planning and controls. The health conditions of those assets need to be closely integrated into real-time control decisions to manage in situ degradation of critical equipment that could challenge the overall system’s operation or safety. PHM for passive SSCs will inform longer-term decision-making. The evolving degradation of these assets under specified operational conditions informs long-term risk assessment, maintenance planning, and outage scheduling. For both SSC types, the deployment of PHM systems should not pose an unacceptable increase in risk to the existing components/structures of the plant in terms of instrumentation constraints (Pham et al., 2012). Typically, the number of installed sensors available is small in NPPs—particularly for passive SSCs (Coble et al., 2015)—because operational compatibility is limited. Novel inspection methods and advanced sensing techniques are needed to perform measurements without compromising the plant’s integrity. Optimizing sensor placement for both legacy and new reactors is also an actively pursued research area to provide adequate and minimally intrusive coverage at a reasonable cost. In the case of new reactors, such needs should be incorporated in the initial design phase to avoid retrofitting.
• Sensor reliability: In addition to the compatibility concern, the sensors are often considered to be a weak link in NPPs because they are sometimes less reliable than the assets they monitor (Pham et al., 2012). Advanced sensor validation and qualification will help overcome sensor reliability issues. Additionally, sensors that can withstand the harsh operating environments (such as radiation, high pressure, high temperature) encountered in some parts of the NPP systems are desired, and their reliability needs to be evaluated carefully.
• Regulatory scrutiny: Nuclear power generation is justifiably a heavily regulated industry owing to the risks associated with plant accidents and radiation exposure to the public. The introduction of any new technology or methodology that may impact safety and protection systems in an NPP is scrutinized to such an extent that many research advances are never implemented and deployed (Ayo-Imoru and Cilliers, 2018). This is a significant challenge that makes adopting PHM in the nuclear industry a very daunting task. The SSCs in NPPs are categorized as safety-related and non-safety-related (Pham et al., 2012). The safety-related SSCs are relied upon to remain functional during and following a design-basis event. For those SSCs, in many countries, including the United States, it is impossible to add any additional I&C or even change the maintenance practice without prior approval from the regulators. The implementation of PHM for such assets will certainly bear heavy burdens of justification for approval. Another hurdle for PHM of safety-related SSCs is that most of those assets are stand-by during the plant’s normal operation, and their CM can be challenging itself regardless of the regulatory burdens. The non-safety-related SSCs do not require regulatory treatment. However, their unavailability may still carry significant risk in terms of preventing safety-related SSCs from fulfilling their function, triggering transients, or causing actuation of a safety-related system. This fact makes non-safety-related SSCs—usually referred to as the balance of plant assets—ideal candidates for coverage under the PHM framework.
• Nuclear-applicable standards: As briefly mentioned in “Prognostics and Health Management Framework and Modeling Approaches” Section, industrial standards for CM, diagnostics, and prognostics exist; however, those standards are largely not specific to the nuclear industry and have not been reviewed by the NRC (in the United States) for application to NPPs. Additionally, a unifying PHM standard that is applicable to generic assets is still missing. Some of the existing and pending standards are potentially relevant to SSCs in NPPs though. As an example, the IEEE P1856 standard covering PHM for electronic systems has broad applicability in digital I&C systems of NPPs. Besides, this IEEE standard provides a framework for developing a general PHM standard for any complex engineering system, as well as specific standards for nuclear SSCs. Developing nuclear-applicable PHM standards is a desired next step. Further efforts will be needed to have those standards reviewed and endorsed by the regulators, without which PHM cannot be deployed for safety-related systems (as mentioned in the bullet point above).
• Data availability and quality: There is a growing realization that although the nuclear power enterprise is more than 50 years old, most of the recorded operational data are not publicly available or useful from the perspective of plant reliability and production improvement. The underlying cause is threefold: justifiable protection of intellectual property and security (for safety-related data), low availability of run-to-failure data in most SSCs, and lack of customer needs for relevant data (until very recently). With most modeling tools reviewed in this paper, the effectiveness of the proposed PHM system is unfortunately constrained by the quality of data that can be ultimately reflected in these models. The data-driven methods—particularly those powered by ML/DL—require access to large quantities of data from anomalies observed in the field to train and validate PHM models. To support PHM development and deployment in the nuclear industry, a long-term campaign for coupled model building and data collection is needed, and initiatives to set a road map for coordinated data sharing are highly recommended. In the interim, the artificial/synthetic data obtained from high-fidelity simulations may be used before moving to actual operating data as they become available.
• Physics-of-failure knowledge: The NPP consists of various complex multidimensional, nonlinear engineered systems, many being exposed to potentially severe thermal, chemical, and radiological stressors. The underlying physics of certain failure modes for some systems (and their subsystems/components) remains too poorly understood to develop physics-based diagnostic and prognostic models. Research efforts to enhance physics-of-failure knowledge will also help with accurate sensor placement, especially for passive SSCs (Coble et al., 2012). Furthermore, developing high-fidelity physical models can be expensive and time-consuming. It is deemed more realistic and appropriate to leverage both experimental data and physics-of-failure knowledge within a hybrid framework to fully describe the failure modes and degradation process of a monitored asset.
Intersection of Prognostics and Health Management and Probabilistic Risk Assessment
Opportunities emerge as the modern industry moves toward the vision of a data-driven “Industry 4.0” paradigm (Farsi and Zio, 2019). Advances in digital I&C systems, low-cost sensors, and high-performance computing architectures offer new promises and insights for not only PHM but also PRA as means to enhance the safety and reliability of complex engineering systems such as NPPs. So far, PHM has been primarily focused on developing and implementing algorithms for component-level (or simple subsystem-level) health assessment. Significant challenges remain to be solved to develop system-level PHM tools, including component interactions, environmental effects, system nonlinearity, uncertainty propagation, and scalability concern (Atamuradov et al., 2017). On the other hand, PRA is mainly involved with using risk and reliability engineering methods to provide a system-level perspective with emphasis on engineering knowledge and systems logic modeling. Although PRA is well-established in high-consequence industries (such as nuclear), it has largely been used as an offline, static methodology, and few studies have recently attempted to incorporate online CM data within the implementation of dynamic PRA (Moradi and Groth, 2020). Both PHM and PRA technologies bring unique advantages and disadvantages, and they appear to have complementary characteristics that can be synergized in the context of complex engineering systems.
To date, only a handful of research publications have explored the potential intersection of PHM and PRA for complex systems. As introduced in “From Enhanced Risk Monitors to Supervisory Control: A Pioneering Study” Section, Ramuhalli et al. (2014) developed an ERM framework that integrated component-specific time-dependent failure information from PHM models into PRA to provide a dynamic risk measure. Similarly, Yadav et al. (2018) proposed a dynamic PRA model incorporating plant component health conditions using a PHM model based on sensor-based degradation data. More recently, Zhou et al. (2020) proposed a time-dependent common cause failure model by integrating degradation states of components inferred from multi-sensor data and demonstrated using an experimental study of three identical centrifugal pumps. Moradi and Groth (2020) reviewed the limited literature on PHM–PRA intersection and introduced a modernized risk assessment approach to systematically integrate those two families of techniques, considering that complex systems can be modeled as a multilevel hierarchical structure with interactive components. The component/subsystem-level analysis is reflective of the model development aspects of PHM, and the system-level analysis is reflective of those of PRA. In that vein, further research needs include developing of a physically interpretable logic model for learning, inference, and information updating as well as finding suitable risk metrics for assessing the logic model performance.
Verification and Validation
As compared to the amount of research put toward methods and frameworks for PHM, the state of research and effort toward verification and validation (V&V) remains nearly unchanged from the previous review by Coble et al. (Coble et al., 2015). A common trend in the literature is the tendency to perform V&V as one segment of research into a full PHM system, but that task is typically focused on a specific application rather than being an investigation of rigorous and robust V&V methods or frameworks. One work that has addressed this matter (Sun et al., 2016) introduced four performance metrics and two quantitative evaluation methods to provide one unified procedure for determining the trustworthiness of prognostic systems. That work was focused upon prognostics in general; however, there are challenges specific to V&V in the nuclear realm, namely data availability and regulation. Statistically significant data are lacking for V&V of prognostic algorithms across all relevant NPP SSCs. A desired action item at the overall DOE–industry level is to develop benchmark test beds for common data generation and collaborative method V&V efforts. Additionally, in the United States, any V&V methodology proposed will need approval and endorsement from the NRC, which would require a review of all data and models proposed to be used from a regulatory standpoint.
Uncertainty Quantification and Propagation
As emphasized throughout “Prognostics and Health Management Framework and Modeling Approaches” Section, the need to quantify uncertainty in PHM model predictions is of paramount importance, especially in data-driven models. A systematic uncertainty analysis can help reveal both reducible and irreducible sources of variability to aid in managing the overall uncertainty in RUL estimates, which is the last step of PHM before integrating it with O&M planning and control (Coble et al., 2015). The field of research in quantifying model uncertainty is not specific to the nuclear industry and is still evolving. A variety of uncertainty sources are involved in PHM, all of which can be categorized as a subset of one of the following: model input uncertainty, model discretization uncertainty, and model form uncertainty (Ewing et al., 2018; Dewey et al., 2019).
• Model input uncertainty comes from the uncertainty of any input parameters, such as uncertainty of material properties (e.g., electric conductivity, elastic modulus), operating conditions, and sensor readings. The overall accuracy of sensors in PHM systems can be compromised by several sources of uncertainty. Firstly, a sensor experiences a natural variation (e.g., a temperature sensor might have a natural uncertainty of ±0.5°). The quantization error from the analog-to-digital converter (ADC) is another source. Most sensors capture analog data that is then converted into digital data through an ADC, and loss of information is unavoidable given an ADC’s inherently limited precision. Furthermore, as sensors age, their accuracy decreases, and their variability increases. The level of such degradation is generally not specified by the manufacturers and is hard to quantify, and so-called “uncertainty of uncertainties” will emerge over long lifespans. As a result, to accurately estimate the overall uncertainty of sensor measurements, all the sources of uncertainty should be acknowledged and taken into account.
• Model discretization uncertainty refers to the uncertainty of treating continuous parameters as discretized variables, such as modeling space as discretized meshes and time as time steps. Quantification of this source of uncertainty could be achieved by comparing analytical solutions or another numerical analysis with a different level of discretization.
• Model form uncertainty arises from the inconsistency between the implemented mathematical model and the real physical world, notably simplifications and approximations made in theories and model implementations. This type of uncertainty source can be estimated by validating with real-world observations or high-fidelity simulations.
Typically, Bayesian inference methods are adopted for quantifying the model prediction uncertainties (Atamuradov et al., 2017; Ramuhalli et al., 2020) because they naturally incorporate information about the target SSC with prior knowledge (e.g., past analysis results, expert opinion) (Coble et al., 2012). Several Bayesian uncertainty quantification approaches have been used in the literature, including BNs (see “Data-Driven Methods” Section), filtering algorithms (such as Kalman filter and particle filter, see “Statistical-Based Prognostics” Section), relevance vector machines (Saha and Goebel, 2008), Bayesian neural networks (Benker et al., 2020), and their variants. Some non-Bayesian approaches have also been proposed for use in the problem of uncertainty estimation, each of which is well suited to specific algorithms or applications. Examples of such approaches include closed-form equations, bootstrapping, and Monte Carlo methods (Coble et al., 2012; Ramuhalli et al., 2012).
In addition to evaluating uncertainty in each module of a PHM system, it is also important to understand how uncertainty in one module can propagate to later modules as well as how uncertainties for individual components will propagate through subsystems, systems, and the whole plant. The latter task can be addressed by prognostic-informed PRA analysis in the ERM framework (Ramuhalli et al., 2014) to integrate sources of uncertainty and their propagation through the ERM calculations. The resulting uncertainty bounds in the ERM output can then be used to perform a probabilistic assessment of the changes in plant O&M and safety risk metrics due to component degradation.
Summary
The contributions of this review paper are threefold: 1) it provides the nuclear industry community with a systematic overview of the full PHM spectrum and an updated in-depth survey of its modeling approaches; 2) it places a strong emphasis on the state of the art of data-driven methods for PHM, primarily driven by recent advances in AI and ML; and 3) it identifies the overarching gaps that still must be addressed by the nuclear industry and PHM communities to support the development and deployment of PHM in NPPs.
To achieve safe and economical operation of NPPs in a competitive energy market, attention is turning to enhanced methods for plant asset management and greater situational awareness of the health condition of key SSCs throughout their life cycles. Interest is growing in applying condition-based (rather than time-based) maintenance for active SSCs and automated online monitoring (instead of periodic inspection) for passive SSCs through the use of PHM principles. Through appropriate detection, diagnosis & prognosis, and mitigation actions, a robust PHM system will allow early warning of degradation in NPPs and will potentially preclude serious consequences due to faults and failures while helping alleviate the burden of unnecessary maintenance activities. Proper application of the full PHM suite will provide improvements to plant reliability and availability and effectively reduce O&M costs and labor reliance.
The full PHM suite utilizes sensor technologies to monitor health conditions, detect anomalies, diagnose faults, predict RUL, and proactively manage failures in complex engineering systems such as NPPs. A complete PHM system proceeds in five modules/steps.
1) Data acquisition: The process of data acquisition from the SSC of interest is necessary to make an accurate, reliable prediction of its health. Collected data can be either sensory or event data. Sensory data are measurements tracked via installed sensors from the target equipment and the focus of “Data Acquisition: Emerging Sensor Technologies” Section, which introduces some of the emerging sensing techniques that have been used for nuclear applications or which are deemed useful soon for CM inside NPPs.
2) Monitoring and detection: Data collected from a target SSC are continuously monitored for deviations from normal behavior, which are indicators of anomalies. As explained in “Condition Monitoring and Fault Detection” Section, the process of fault detection attempts to recognize incipient faults and failures. Multidimensional, high-volume raw data collected by sensors are not ready to be used directly, and appropriate feature selection is required. “Feature Selection Methods” Section describes the three categories of feature selection methods: filters, wrappers, and embedded methods. In “Anomaly Detection Methods” Section, research efforts using data-driven methods for detecting anomalies are highlighted. In particular, various fault detection approaches based on multivariate statistics have gained attention.
3) Fault diagnostics: Once an anomaly is detected, it is vital to diagnose the fault, or in other words, to locate the fault to a specific component or area of a structure (i.e., fault isolation) and to determine the root cause of the fault (i.e., fault identification). As detailed in “Fault Diagnostics” Section, diagnostics can be approached using either a model-based method (“Model-Based Methods” Section), a rule-based method (“Rule-Based Methods” Section), or a data-driven method (“Data-Driven Methods” Section). The distinctions are not completely clear, however, and various hybrid approaches can be developed.
4) Prognostics: Depending on how the SSC will degrade, an appropriate prognostic model is then applied to estimate its RUL. Viewed as one of PHM’s most beneficial aspects, prognostics is paradoxically an underdeveloped module, especially in the nuclear industry. There is no universally accepted methodology for all prognostic problems, and a variety of algorithms have been developed for application to specific situations or classes of components. Based on a collection of recent review papers, “Prognostics” Section divides these algorithms into four model categories: physics-based (“Physics-Based Methods” Section), knowledge-based (“Knowledge-Based Methods” Section), data-driven (“Data-Driven Methods” Section), and hybrid (“Hybrid Methods” Section) models. The data-driven prognostic models—from conventional statistical methods (“Statistical-Based Prognostics” Section) to advanced ML/DL techniques (“Machine Learning-Based Prognostics” Section)—are of particular interest to large complex systems, and they have been the topic of most research in the field of machinery prognostics. A hybrid approach further integrates the strengths of different model types for improved RUL prediction results.
5) Decision-making: O&M planning is informed by the integration of prognostic calculations and risk assessment of proposed mitigation actions based on the current and postulated future health states of the target SSC to achieve optimal (and ultimately autonomous) control and decision-making. As an indispensable step of the broader PHM philosophy, the module of autonomous decision-making has not been extensively studied in the nuclear realm. Given the current status and apparent gaps for NPPs, “Decision-Making” Section first summaries general methods used in decision-making (“Decision-Making Methods” Section). The study presented in “From Enhanced Risk Monitors to Supervisory Control: A Pioneering Study” Section showcases the ability to integrate diagnostics and prognostics results with supervisory control systems for making risk-informed autonomous decisions that utilize real-time information on component conditions.
Even though significant research has been undertaken and more extensive efforts are underway—such as current DOE projects around development of DTs and autonomous control capabilities—to develop PHM systems for nuclear applications, the nuclear industry still lags behind some other industries in bringing PHM from the research arena to commercial deployment. “Research Needs for Deployment of Prognostics and Health Management in Nuclear Power Plants” Section has identified the overarching research needs and technical gaps which still must be addressed to support the development and deployment of PHM in nuclear power generation, including PHM feasibility and challenges specific to NPPs due to the industry’s unique operational framework and licensing requirements; a unifying framework for connecting PHM and PRA to synergize their complementary characteristics in the context of complex systems; benchmark test beds for common data generation and collaborative method V&V efforts; and systematic uncertainty quantification and propagation, especially in the case of data-driven methods.
Author Contributions
XZ: conceptualization and organization; literature search, collection and review; writing (original draft and revisions). JK: literature search, collection and review; writing (original draft and revisions). KW and XW: literature search, collection and review; writing (original draft). PR and SC: literature search; writing (review & editing); supervision. HK and MG: writing (review & editing); supervision; funding acquisition.
Funding
The information presented in this paper was developed from materials collected for the US Department of Energy Nuclear Energy Enabling Technologies project under contract number DE-NE0008873.
Conflict of Interest
The authors declare that the research was conducted in the absence of any commercial or financial relationships that could be construed as a potential conflict of interest.
Acknowledgments
We would like to thank Walter Koncinski for his assistance in editing and formatting of the manuscript. We are also thankful to Birdy Phathanapirom and Justin Weinmeister for their technical comments and feedback.
Footnotes
1The NRC has approved initial license renewal applications for 93 reactors. Unfortunately, eight of them have since ceased operations prematurely.
2The distinction between active and passive SSCs can be complicated. For example, pumps are active components, but their casings and support structures are considered passive.
3It is important to notice that PHM is not a type of maintenance by itself but rather a set of tools that yield information which can be used as input to CBM. In other words, CBM can be adopted with or without the use of PHM. In fact, many traditional frameworks considered CBM but did not include the treatment of PHM techniques/methods. To mark the difference with the traditional concept of CBM (i.e., CBM without implementation of PHM), new terms for PHM-enabled CBM have been introduced in the literature, including CBM+ and CBM/PHM. A comprehensive review on the role of PHM in CBM systems, which is not the focus of this paper, can be found in Guillén et al. (2016).
4The annual fleet-wide O&M costs in the US are estimated to be around $12 billion in 2017 US dollars. This is calculated with an annual O&M cost of $120 per kW for an average US plant of 36 years old (in 2017) (SargentLundy, 2018) and a total capacity of 100 GW (NEI, 2021c).
5The terms “faults” and “anomalies” have been used interchangeably in the literature. Technically speaking, they have a subtle difference in meaning: anomalies refer to deviations indicated by sensor measurements, whereas faults refer to the actual physical manifestations of such deviations in a monitored SSC.
6As defined in Isermann and Ballé (1997), a fault is “an unpermitted deviation of at least one characteristic property or parameter of the SSC from the acceptable/usual/standard operating conditions;” in contrast, a failure is “a permanent interruption of the SSC’s ability to perform a required function under specified conditions.”
7SVMs have become the reference for many classification problems because of their flexibility, computational efficiency, and capacity to handle high-dimensional data.
8Spiking neural P systems are one of the recently developed spiking neural network (SNN) models inspired by the way neurons communicate (Wang et al., 2016). Known as the third generation of neural networks, SNNs use time to encode information and employ the concept of individual spikes. Those features make SNNs biologically more realistic (Fan et al., 2020).
9The health index, computed from diagnostics, is an indicator of the ability of the monitored SSC to meet its functional goals.
References
Ahmed, I., Heo, G., and Kassim, M. (2017). Fault Detection and Diagnosis of Nuclear Power Plant Using Deep Learning Architecture. Jeju, Korea: Trans Korean Nuclear Society.
Ajami, A., and Daneshvar, M. (2012). Data Driven Approach for Fault Detection and Diagnosis of Turbine in thermal Power Plant Using Independent Component Analysis (ICA). Int. J. Electr. Power Energ. Syst. 43, 728–735. doi:10.1016/j.ijepes.2012.06.022
Al Rashdan, A., Smith, J., Germain, St. S., Ritter, C., Agarwal, V., Boring, R., et al. (2018). Development of a Technology Roadmap for Online Monitoring of Nuclear Power Plants. Technical Report, INL/EXT-18-52206. Idaho Falls, ID: US Department of Energy, Office of Nuclear Energy. Light Water Reactor Sustainability Program. doi:10.2172/1492833
An, D., Kim, N. H., and Choi, J.-H. (2015). Practical Options for Selecting Data-Driven or Physics-Based Prognostics Algorithms with Reviews. Reliability Eng. Syst. Saf. 133, 223–236. doi:10.1016/j.ress.2014.09.014
Archibald, R., and Fann, G. (2007). Feature Selection and Classification of Hyperspectral Images With Support Vector Machines. IEEE Geosci. Remote Sensing Lett. 4, 674–677. doi:10.1109/LGRS.2007.905116
Atamuradov, V., Medjaher, K., Dersin, P., Lamoureux, B., and Zerhouni, N. (2017). Prognostics and Health Management for Maintenance Practitioners - Review, Implementation and Tools Evaluation. Int. J. Progn Heal Manag. 8, 1–31. doi:10.36001/ijphm.2017.v8i3.2667
Atoui, M. A., Verron, S., and Kobi, A. (2015). Fault Detection with Conditional Gaussian Network. Eng. Appl. Artif. Intelligence 45, 473–481. doi:10.1016/j.engappai.2015.07.020
Aubry, J.-F., Brinzei, N., and Mazouni, M.-H. (2016). “Comparison Petri Nets - Finite State Automaton,” in Syst. Dependability Assess. Benefits Petri Net Model. (Hoboken, NJ, USA: John Wiley & Sons), 25–33. doi:10.1002/9781119262114.ch3
Ayodeji, A., and Liu, Y.-k. (2018). Support Vector Ensemble for Incipient Fault Diagnosis in Nuclear Plant Components. Nucl. Eng. Technol. 50, 1306–1313. doi:10.1016/j.net.2018.07.013
Ayodeji, A., Liu, Y.-k., and Xia, H. (2018). Knowledge Base Operator Support System for Nuclear Power Plant Fault Diagnosis. Prog. Nucl. Energ. 105, 42–50. doi:10.1016/j.pnucene.2017.12.013
Ayo-Imoru, R. M., and Cilliers, A. C. (2018). A Survey of the State of Condition-Based Maintenance (CBM) in the Nuclear Power Industry. Ann. Nucl. Energ. 112, 177–188. doi:10.1016/j.anucene.2017.10.010
Badue, C., Guidolini, R., Carneiro, R. V., Azevedo, P., Cardoso, V. B., Forechi, A., et al. (2021). Self-driving Cars: A Survey. Expert Syst. Appl. 165, 113816. doi:10.1016/j.eswa.2020.113816
Bampoula, X., Siaterlis, G., Nikolakis, N., and Alexopoulos, K. (2021). A Deep Learning Model for Predictive Maintenance in Cyber-Physical Production Systems Using LSTM Autoencoders. Sensors 21, 972. doi:10.3390/s21030972
Banerjee, S., Deng, J., Gorse, C., Vajpayee, V., Becerra, V., and Shimjith, S. R. (2020). ANN Based Sensor and Actuator Fault Detection in Nuclear Reactors. 2020 8th International Conference on Control, Mechatronics and Automation (ICCMA), November 6-8, 2020, Moscow, Russia, 88–94. doi:10.1109/ICCMA51325.2020.9301579
Barraza-Barraza, D., Tercero-Gómez, V. G., Beruvides, M. G., and Limón-Robles, J. (2017). An Adaptive ARX Model to Estimate the RUL of Aluminum Plates Based on its Crack Growth. Mech. Syst. Signal Process. 82, 519–536. doi:10.1016/j.ymssp.2016.05.041
Baur, M., Albertelli, P., and Monno, M. (2020). A Review of Prognostics and Health Management of Machine Tools. Int. J. Adv. Manuf Technol. 107, 2843–2863. doi:10.1007/s00170-020-05202-3
Bektas, O., Marshall, J., and Jones, J. A. (2020). Comparison of Computational Prognostic Methods for Complex Systems Under Dynamic Regimes: A Review of Perspectives. Arch. Computat Methods Eng. 27, 999–1011. doi:10.1007/s11831-019-09339-7
Benker, M., Furtner, L., Semm, T., and Zaeh, M. F. (2020). Utilizing Uncertainty Information in Remaining Useful Life Estimation via Bayesian Neural Networks and Hamiltonian Monte Carlo. J. Manufacturing Syst. doi:10.1016/j.jmsy.2020.11.005
Berredjem, T., and Benidir, M. (2018). Bearing Faults Diagnosis Using Fuzzy Expert System Relying on an Improved Range Overlaps and Similarity Method. Expert Syst. Appl. 108, 134–142. doi:10.1016/j.eswa.2018.04.025
Bhajantri, L. B. (2018). Fuzzy Logic Based Fault Detection in Distributed Sensor Networks. Int. J. Sci. Res. Comput. Sci. Eng. 6, 27–32. doi:10.26438/ijsrcse/v6i2.2732
Bhuiyan, M. Y., and Giurgiutiu, V. (2018). The Signatures of Acoustic Emission Waveforms from Fatigue Crack Advancing in Thin Metallic Plates. Smart Mater. Struct. 27, 015019. doi:10.1088/1361-665X/aa9bc2
Bhuiyan, M. Y., Bao, J., Poddar, B., and Giurgiutiu, V. (2018). Toward Identifying Crack-Length-Related Resonances in Acoustic Emission Waveforms for Structural Health Monitoring Applications. Struct. Health Monit. 17, 577–585. doi:10.1177/1475921717707356
Blum, A. L., and Langley, P. (1997). Selection of Relevant Features and Examples in Machine Learning. Artif. Intelligence 97, 245–271. doi:10.1016/S0004-3702(97)00063-5
Bommert, A., Sun, X., Bischl, B., Rahnenführer, J., and Lang, M. (2020). Benchmark for Filter Methods for Feature Selection in High-Dimensional Classification Data. Comput. Stat. Data Anal. 143, 106839. doi:10.1016/j.csda.2019.106839
Bond, L. J., Ramuhalli, P., Tawfik, M. S., and Lybeck, N. J. (2011). Prognostics and Life beyond 60 Years for Nuclear Power Plants. 2011 IEEE Conference on Prognostics and Health Management, June 20-23, 2011, Denver, CO. doi:10.1109/ICPHM.2011.6024316
Box, G. E. P. (1954). Some Theorems on Quadratic Forms Applied in the Study of Analysis of Variance Problems, I. Effect of Inequality of Variance in the One-Way Classification. Ann. Math. Statist. 25, 290–302. doi:10.1214/aoms/1177728786
Brechtel, S., Gindele, T., and Dillmann, R. (2014). Probabilistic Decision-Making under Uncertainty for Autonomous Driving Using Continuous POMDPs. 17th International IEEE Conference on Intelligent Transportation Systems (ITSC). Qingdao, China, 392–399. doi:10.1109/ITSC.2014.6957722
Bzdok, D., Altman, N., and Krzywinski, M. (2018). Statistics versus Machine Learning. Nat. Methods 15, 233–234. doi:10.1038/nmeth.4642
Cai, Y., and Golay, M. W. (2021). A Framework Analyzing System Status and Human Activities: Illustrated Using 2011 Fukushima Nuclear Power Plant Accident Scenarios. Nucl. Eng. Des. 373, 111025. doi:10.1016/j.nucengdes.2020.111025
Cai, L., and Tian, X. (2014). A New Fault Detection Method for Non-Gaussian Process Based on Robust Independent Component Analysis. Process Saf. Environ. Prot. 92, 645–658. doi:10.1016/j.psep.2013.11.003
Cai, B., Liu, Y., Fan, Q., Zhang, Y., Liu, Z., Yu, S., et al. (2014). Multi-source Information Fusion Based Fault Diagnosis of Ground-Source Heat Pump Using Bayesian Network. Appl. Energ. 114, 1–9. doi:10.1016/j.apenergy.2013.09.043
Cai, B., Huang, L., and Xie, M. (2017). Bayesian Networks in Fault Diagnosis. IEEE Trans. Ind. Inf. 13, 2227–2240. doi:10.1109/TII.2017.2695583
Calderoni, P., Hurley, D., Daw, J., Fleming, A., and McCary, K. (2019). “Innovative Sensing Technologies for Nuclear Instrumentation,” in 2019 IEEE International Instrumentation and Measurement Technology Conference (I2MTC), Auckland, New Zealand. doi:10.1109/I2MTC.2019.8827129
Cetiner, S., and Ramuhalli, P. (2019). Transformational Challenge Reactor Autonomous Control System Framework and Key Enabling Technologies. Technical Report, ORNL/SPR-2019/1178. Oak Ridge, TN: Oak Ridge National Laboratory. doi:10.2172/1530084
Cetiner, S., Muhlheim, M. D., Flanagan, G. F., Fugate, D. L., and Kisner, R. A. (2014). Development of an Automated Decision-Making Tool for Supervisory Control System. Technical Report, ORNL/TM-2014/363. Oak Ridge, TN: Oak Ridge National Laboratory. doi:10.2172/1252136
Cetiner, S., Muhlheim, M. D., Guler-Yigitoglu, A., Belles, R. J., Greenwood, M. S., Harrison, T. J., et al. (2016). Supervisory Control System for Multi-Modular Advanced Reactors. Technical Report, ORNL/TM-2016/693. Oak Ridge, TN: Oak Ridge National Laboratory. doi:10.2172/1615832
Chandrashekar, G., and Sahin, F. (2014). A Survey on Feature Selection Methods. Comput. Electr. Eng. 40, 16–28. doi:10.1016/j.compeleceng.2013.11.024
Chen, G., and Chen, J. (2015). A Novel Wrapper Method for Feature Selection and its Applications. Neurocomputing 159, 219–226. doi:10.1016/j.neucom.2015.01.070
Chen, J. G., Davis, A., Wadhwa, N., Durand, F., Freeman, W. T., and Büyüköztürk, O. (2017). Video Camera–Based Vibration Measurement for Civil Infrastructure Applications. J. Infrastruct Syst. 23, 1–11. doi:10.1061/(ASCE)IS.1943-555X.0000348
Chen, J., Jing, H., Chang, Y., and Liu, Q. (2019). Gated Recurrent Unit Based Recurrent Neural Network for Remaining Useful Life Prediction of Nonlinear Deterioration Process. Reliability Eng. Syst. Saf. 185, 372–382. doi:10.1016/j.ress.2019.01.006
Chen, K. P. (2018). High Spatial Resolution Distributed Fiber-Optic Sensor Networks for Reactors and Fuel Cycle Systems. University of Pittsburgh. Pittsburgh, PA: Final Report for DE-NE0008303. doi:10.2172/1475174
Cho, S., and Jiang, J. (2018). Optimal Fault Classification Using Fisher Discriminant Analysis in the Parity Space for Applications to NPPs. IEEE Trans. Nucl. Sci. 65, 856–865. doi:10.1109/TNS.2018.2803658
Coble, J. B., Ramuhalli, P., Bond, L. J., Hines, J. W., and Upadhyaya, B. R. (2012). Prognostics and Health Management in Nuclear Power Plants: A Review of Technologies and Applications. Technical Report, PNNL-21515. Richland, WA: Pacific Northwest National Laboratory. doi:10.2172/1047416
Coble, J., Ramuhalli, P., Bond, L., Hines, J. W., and Upadhyaya, B. (2015). A Review of Prognostics and Health Management Applications in Nuclear Power Plants. Int. J. Progn Heal Manag. 6, 1–22. doi:10.36001/ijphm.2015.v6i3.2271
Costelha, H., and Lima, P. (2012). Robot Task Plan Representation by Petri Nets: Modelling, Identification, Analysis and Execution. Auton. Robot 33, 337–360. doi:10.1007/s10514-012-9288-x
Cuc, A., Giurgiutiu, V., Joshi, S., and Tidwell, Z. (2007). Structural Health Monitoring with Piezoelectric Wafer Active Sensors for Space Applications. AIAA J. 45, 2838–2850. doi:10.2514/1.26141
Da Silva, R. R., Costa, E. D. S., De Oliveira, R. C. L., and Mesquita, A. L. A. (2017). Fault Diagnosis in Rotating Machine Using Full Spectrum of Vibration and Fuzzy Logic. J. Eng. Sci. Technol. 12, 2952–2964.
Das, S. (2001). Filters, Wrappers and a Boosting-Based Hybrid for Feature Selection. Proc. Eighteenth Int. Conf. Mach. Learn., 74–81. doi:10.5555/645530.658297
Davis, A., Bouman, K. L., Chen, J. G., Rubinstein, M., Buyukozturk, O., Durand, F., et al. (2017). Visual Vibrometry: Estimating Material Properties from Small Motions in Video. IEEE Trans. Pattern Anal. Mach. Intell. 39, 732–745. doi:10.1109/TPAMI.2016.2622271
Daw, J., Rempe, J., Palmer, J., Ramuhalli, P., Keller, P., Montgomery, R., et al. (2014). NEET In-Pile Ultrasonic Sensor Enablement-Final Report. INL/EXT-14-32505. Idaho Falls, ID: Idaho National Laboratory. doi:10.2172/1166037
Deleplace, A., Atamuradov, V., Allali, A., Pellé, J., Plana, R., and Alleaume, G. (2020). Ensemble Learning-Based Fault Detection in Nuclear Power Plant Screen Cleaners. IFAC-PapersOnLine 53, 10354–10359. doi:10.1016/j.ifacol.2020.12.2773
Deng, X.-W., Gao, Q.-S., Zhang, C., Hu, D., and Yang, T. (2017). Rule - Based Fault Diagnosis Expert System for Wind Turbine. ITM Web Conf. 11, 07005. doi:10.1051/itmconf/20171107005
Deng, Y., Li, Z., Chen, J., and Qi, X. (2018). The Effects of the Structure Characteristics on Magnetic Barkhausen Noise in Commercial Steels. J. Magnetism Magn. Mater. 451, 276–282. doi:10.1016/j.jmmm.2017.11.041
Dewey, H. H., DeVries, D. R., and Hyde, S. R. (2019). “Uncertainty Quantification in Prognostic Health Management Systems,” in 2019 IEEE Aerospace Conference, Big Sky, MT, USA, 1–13. doi:10.1109/AERO.2019.8741821
Diez-Olivan, A., Del Ser, J., Galar, D., and Sierra, B. (2019). Data Fusion and Machine Learning for Industrial Prognosis: Trends and Perspectives towards Industry 4.0. Inf. Fusion 50, 92–111. doi:10.1016/j.inffus.2018.10.005
Dourado, A., and Viana, F. A. C. (2020). Physics-Informed Neural Networks for Missing Physics Estimation in Cumulative Damage Models: A Case Study in Corrosion Fatigue. J. Comput. Inf. Sci. Eng. 20, 061007. doi:10.1115/1.4047173
Downs, J. J., and Vogel, E. F. (1993). A Plant-wide Industrial Process Control Problem. Comput. Chem. Eng. 17, 245–255. doi:10.1016/0098-1354(93)80018-I
Droguett, E. L. (2020). “The PHM Perspective,” in ASME-SERAD and UMD-CRR Interactive Seminar & Pre-workshop on the Intersection of PRA and PHM, October 2, 2020.
Du, W., Guo, X., Wang, Z., Wang, J., Yu, M., Li, C., et al. (2020). A New Fuzzy Logic Classifier Based on Multiscale Permutation Entropy and Its Application in Bearing Fault Diagnosis. Entropy 22, 27. doi:10.3390/e22010027
Dziendzikowski, M., Kurnyta, A., Dragan, K., Klysz, S., and Leski, A. (2016). In Situ Barely Visible Impact Damage Detection and Localization for Composite Structures Using Surface Mounted and Embedded PZT Transducers: A Comparative Study. Mech. Syst. Signal Process. 78, 91–106. doi:10.1016/j.ymssp.2015.09.021
Ebrahimkhanlou, A., Dubuc, B., and Salamone, S. (2016). Damage Localization in Metallic Plate Structures Using Edge-Reflected Lamb Waves. Smart Mater. Struct. 25, 085035. doi:10.1088/0964-1726/25/8/085035
Eker, O. F., Camci, F., and Jennions, I. K. (2019). A New Hybrid Prognostic Methodology. Int. J. Progn Heal Manag. 10, 1–13. doi:10.36001/ijphm.2019.v10i2.2727
Elsheikh, A., Yacout, S., and Ouali, M.-S. (2019). Bidirectional Handshaking LSTM for Remaining Useful Life Prediction. Neurocomputing 323, 148–156. doi:10.1016/j.neucom.2018.09.076
Ewing, M. E., Liechty, B. C., and Black, D. L. (2018). A General Methodology for Uncertainty Quantification in Engineering Analyses Using a Credible Probability Box. J. Verif. Valid. Uncertain. Quantif. 3, 021003. doi:10.1115/1.4041490
Fan, S., Paul, P., Wu, T., Rong, H., and Zhang, G. (2020). On Applications of Spiking Neural P Systems. Appl. Sci. 10, 7011. doi:10.3390/app10207011
Farsi, M. A., and Zio, E. (2019). Industry 4.0: Some Challenges and Opportunities for Reliability Engineering. Int. J. Reliabl Risk Safe Theor. App 2, 23–34. doi:10.30699/IJRRS.2.1.4
Fleming, A., Al Rashdan, A., Jensen, C., and Calderoni, P. (2019). An Impedance-Based Diameter Gauge for In-Pile Fuel Deformation Measurements. Instrumentation Sci. Technol. 47, 611–626. doi:10.1080/10739149.2019.1616207
Forman, R. G. (1972). Study of Fatigue Crack Initiation from Flaws Using Fracture Mechanics Theory. Eng. Fracture Mech. 4, 333–345. doi:10.1016/0013-7944(72)90048-3
Gao, Y., Gao, X., and Zhang, X. (2017). The 2 °C Global Temperature Target and the Evolution of the Long-Term Goal of Addressing Climate Change-From the United Nations Framework Convention on Climate Change to the Paris Agreement. Engineering 3, 272–278. doi:10.1016/J.ENG.2017.01.022
Gautam, S., Tamboli, P. K., Roy, K., Patankar, V. H., and Duttagupta, S. P. (2019). Sensors Incipient Fault Detection and Isolation of Nuclear Power Plant Using Extended Kalman Filter and Kullback-Leibler Divergence. ISA Trans. 92, 180–190. doi:10.1016/j.isatra.2019.02.011
Ge, Z., Song, Z., and Gao, F. (2013). Review of Recent Research on Data-Based Process Monitoring. Ind. Eng. Chem. Res. 52, 3543–3562. doi:10.1021/ie302069q
Ghahramani, Z. (2001). An Introduction to Hidden Markov Models and Bayesian Networks. Int. J. Patt. Recogn. Artif. Intell. 15, 9–42. doi:10.1142/S0218001401000836
Goebel, K., Eklund, N., and Bonanni, P. (2006). “Fusing Competing Prediction Algorithms for Prognostics,” in IEEE Aerospace Conference, Big Sky, MT, USA. doi:10.1109/AERO.2006.1656116
Goldberg, D. E. (1989). Genetic Algorithms in Search, Optimization and Machine Learning. Boston, MA, USA: Addison-Wesley Longman Publishing Co., Inc.
Gomes, C. R., and Canedo Medeiros, J. A. C. (2015). Neural Network of Gaussian Radial Basis Functions Applied to the Problem of Identification of Nuclear Accidents in a PWR Nuclear Power Plant. Ann. Nucl. Energ. 77, 285–293. doi:10.1016/j.anucene.2014.10.001
Guillén, A. J., Crespo, A., Macchi, M., and Gómez, J. (2016). On the Role of Prognostics and Health Management in Advanced Maintenance Systems. Prod. Plann. Control. 27, 991–1004. doi:10.1080/09537287.2016.1171920
Gurgen, A., Lin, L., and Dinh, N. (2020). Development and Assessment of Physics-Guided Machine Learning for Prognosis System. Trans. Am. Nucl. Soc., 272–275. doi:10.13182/T123-33503
Guyon, I., and Elisseeff, A. (2003). An Introduction to Variable and Feature Selection. J. Mach Learn. Res. 3, 1157–1182. doi:10.5555/944919.944968
Guyon, I., Weston, J., Barnhill, S., and Vapnik, V. (2002). Gene Selection for Cancer Classification Using Support Vector Machines. Mach Learn. 46, 389–422. doi:10.1023/A:1012487302797
Haider, M. F., Lin, B., Yu, L., and Giurgiutiu, V. (2017). Sensing Capabilities of Piezoelectric Wafer Active Sensors in Extreme Nuclear Environment. Proc. Soc. Photo-Optical Instrum. Eng. 10169. doi:10.1117/12.2260162
Hanna, B. N., Trieu, L. L. T., Son, T. C., and Dinh, N. T. (2020). An Application of ASP in Nuclear Engineering: Explaining the Three Mile Island Nuclear Accident Scenario. Theor. Pract. Logic Program. 20, 926–941. doi:10.1017/S1471068420000241
Harrou, F., Nounou, M. N., Nounou, H. N., and Madakyaru, M. (2013). Statistical Fault Detection Using PCA-Based GLR Hypothesis Testing. J. Loss Prev. Process Industries 26, 129–139. doi:10.1016/j.jlp.2012.10.003
Hashemian, H. M. (2011). On-line Monitoring Applications in Nuclear Power Plants. Prog. Nucl. Energ. 53, 167–181. doi:10.1016/j.pnucene.2010.08.003
Hess, A. (2002). “Prognostics, from the Need to Reality-From the Fleet Users and PHM System Designer/developers Perspectives,” in Proceedings, IEEE Aerospace Conference, Big Sky, MT, USA. doi:10.1109/AERO.2002.1036118
Hong, X., Liu, Y., Liufu, Y., and Lin, P. (2018). Debonding Detection in Hidden Frame Supported Glass Curtain walls Using the Nonlinear Ultrasonic Modulation Method with Piezoceramic Transducers. Sensors 18, 2094. doi:10.3390/s18072094
Hotelling, H. (1933). Analysis of a Complex of Statistical Variables into Principal Components. J. Educ. Psychol. 24, 417–441. doi:10.1037/h0071325
Hu, Y., Baraldi, P., Di Maio, F., and Zio, E. (2016). Online Performance Assessment Method_newline for a Model-Based Prognostic Approach. IEEE Trans. Rel. 65, 718–735. doi:10.1109/TR.2015.2500681
Husain, Z. (2018). Fuzzy Logic Expert System for Incipient Fault Diagnosis of Power Transformers. Ijeei 10, 300–317. doi:10.15676/ijeei.2018.10.2.8
IAEA (1996). Defence in Depth in Nuclear Safety. INSAG-10, a Report by the International Nuclear Safety Advisory Group. Vienna, Austria: International Atomic Energy Agency.
IAEA (2021). “The Database on Nuclear Power Reactors,” in Power Reactor Information System (Vienna, Austria: International Atomic Energy Agency) Retrieved in January 2021. https://pris.iaea.org/pris/.
IEA and NEA (2020). Projected Costs of Generating Electricity. 2020 Edition. Paris, France: International Energy Agency and OECD Nuclear Energy Agency.
Isermann, R., and Ballé, P. (1997). Trends in the Application of Model-Based Fault Detection and Diagnosis of Technical Processes. Control. Eng. Pract. 5, 709–719. doi:10.1016/S0967-0661(97)00053-1
Jamil, F., Abid, M., Haq, I., Khan, A. Q., and Iqbal, M. (2016). Fault Diagnosis of Pakistan Research Reactor-2 with Data-Driven Techniques. Ann. Nucl. Energ. 90, 433–440. doi:10.1016/j.anucene.2015.12.023
Jamil, F., Abid, M., Adil, M., Haq, I., Khan, A. Q., and Khan, S. F. (2018). Kernel Approaches for Fault Detection and Classification in PARR-2. J. Process Control. 64, 1–6. doi:10.1016/j.jprocont.2018.01.003
Jardine, A. K. S., Lin, D., and Banjevic, D. (2006). A Review on Machinery Diagnostics and Prognostics Implementing Condition-Based Maintenance. Mech. Syst. Signal Process. 20, 1483–1510. doi:10.1016/j.ymssp.2005.09.012
Javed, K., Gouriveau, R., and Zerhouni, N. (2014). SW-ELM: A Summation Wavelet Extreme Learning Machine Algorithm with A Priori Parameter Initialization. Neurocomputing 123, 299–307. doi:10.1016/j.neucom.2013.07.021
Jia, Q., and Zhang, Y. (2016). Quality-related Fault Detection Approach Based on Dynamic Kernel Partial Least Squares. Chem. Eng. Res. Des. 106, 242–252. doi:10.1016/j.cherd.2015.12.015
Jiang, Q., and Yan, X. (2018). Parallel PCA-KPCA for Nonlinear Process Monitoring. Control. Eng. Pract. 80, 17–25. doi:10.1016/j.conengprac.2018.07.012
Jiao, J., Zhao, N., Wang, G., and Yin, S. (2017). A Nonlinear Quality-Related Fault Detection Approach Based on Modified Kernel Partial Least Squares. ISA Trans. 66, 275–283. doi:10.1016/j.isatra.2016.10.015
John, G. H., Kohavi, R., and Pfleger, K. (1994). Irrelevant Features and the Subset Selection Problem. Mach. Learn. Proc. New Brunswick, NJ: Morgan Kaufmann Publishers, 121–129. doi:10.1016/B978-1-55860-335-6.50023-4
Karami, M., and Wang, L. (2018). Fault Detection and Diagnosis for Nonlinear Systems: A New Adaptive Gaussian Mixture Modeling Approach. Energy and Buildings 166, 477–488. doi:10.1016/j.enbuild.2018.02.032
Kennedy, J., and Eberhart, R. (1995). “Particle Swarm Optimization,” in Proceedings of ICNN'95 - International Conference on Neural Networks, November 27-December 1, 1995, Perth, WA (Perth, WA, Australia: IEEE). doi:10.1109/ICNN.1995.488968
Khan, S., and Yairi, T. (2018). A Review on the Application of Deep Learning in System Health Management. Mech. Syst. Signal Process. 107, 241–265. doi:10.1016/j.ymssp.2017.11.024
Khan, S. A., Prosvirin, A. E., and Kim, J.-M. (2018). “Towards Bearing Health Prognosis Using Generative Adversarial Networks: Modeling Bearing Degradation,” in 2018 International Conference on Advancements in Computational Sciences (ICACS), Lahore, Pakistan. doi:10.1109/ICACS.2018.8333495
Kharoufeh, J. P., and Cox, S. M. (2005). Stochastic Models for Degradation-Based Reliability. IIE Trans. 37, 533–542. doi:10.1080/07408170590929009
Kharoufeh, J. P., Solo, C. J., and Ulukus, M. Y. (2010). Semi-Markov Models for Degradation-Based Reliability. IIE Trans. 42, 599–612. doi:10.1080/07408170903394371
Kharoufeh, J. P. (2003). Explicit Results for Wear Processes in a Markovian Environment. Operations Res. Lett. 31, 237–244. doi:10.1016/S0167-6377(02)00229-8
Kim, T. K., Park, J. K., Lee, B. H., and Seong, S. H. (2019). Deep-learning-based Alarm System for Accident Diagnosis and Reactor State Classification with Probability Value. Ann. Nucl. Energ. 133, 723–731. doi:10.1016/j.anucene.2019.07.022
Kim, J., Zhao, X., Shah, A. U. A., and Kang, H. G. (2021). System Risk Quantification and Decision Making Support Using Functional Modeling and Dynamic Bayesian Network (under review).
Kochenderfer, M. J. (2015). Decision Making Under Uncertainty: Theory and Application. Cambridge, MA, USA: The MIT Press. doi:10.7551/mitpress/10187.001.0001
Kohavi, R., and John, G. H. (1997). Wrappers for Feature Subset Selection. Artif. Intelligence 97, 273–324. doi:10.1016/S0004-3702(97)00043-X
Komagome, Y., and Matsumoto, E. (2002). Visualization of Back Surface Defects by Piezoelectric Film. Jae 14, 425–428. doi:10.3233/jae-2002-418
Kozjek, D., Malus, A., and Vrabič, R. (2020). Multi-objective Adjustment of Remaining Useful Life Predictions Based on Reinforcement Learning. Proced. CIRP 93, 425–430. doi:10.1016/j.procir.2020.03.051
Krishnakumari, A., Elayaperumal, A., Saravanan, M., and Arvindan, C. (2017). Fault Diagnostics of spur Gear Using Decision Tree and Fuzzy Classifier. Int. J. Adv. Manuf Technol. 89, 3487–3494. doi:10.1007/s00170-016-9307-8
Lazar, C., Taminau, J., Meganck, S., Steenhoff, D., Coletta, A., Molter, C., et al. (2012). A Survey on Filter Techniques for Feature Selection in Gene Expression Microarray Analysis. IEEE/ACM Trans. Comput. Biol. Bioinf. 9, 1106–1119. doi:10.1109/TCBB.2012.33
Lee, C.-K., and Shin, Y.-J. (2018). Multi-core cable Fault Diagnosis Using Cluster Time-Frequency Domain Reflectometry. 2018 IEEE International Instrumentation and Measurement Technology Conference (I2MTC), May 14-17, 2018, Houston, TX1–6. doi:10.1109/I2MTC.2018.8409561
Lee, J., Ghaffari, M., and Elmeligy, S. (2011). Self-maintenance and Engineering Immune Systems: Towards Smarter Machines and Manufacturing Systems. Annu. Rev. Control. 35, 111–122. doi:10.1016/j.arcontrol.2011.03.007
Lee, H., Sohn, H., Yang, S., and Yang, J. (2014). Monitoring of Pipelines in Nuclear Power Plants by Measuring Laser-Based Mechanical Impedance. Smart Mater. Struct. 23, 065008. doi:10.1088/0964-1726/23/6/065008
Lee, G., Lee, S. J., and Lee, C. (2021). A Convolutional Neural Network Model for Abnormality Diagnosis in a Nuclear Power Plant. Appl. Soft Comput. 99, 106874. doi:10.1016/j.asoc.2020.106874
Lei, Y., Li, N., Guo, L., Li, N., Yan, T., and Lin, J. (2018). Machinery Health Prognostics: A Systematic Review from Data Acquisition to RUL Prediction. Mech. Syst. Signal Process. 104, 799–834. doi:10.1016/j.ymssp.2017.11.016
Li, R. F., and Wang, X. Z. (2002). Dimension Reduction of Process Dynamic Trends Using Independent Component Analysis. Comput. Chem. Eng. 26, 467–473. doi:10.1016/S0098-1354(01)00773-6
Li, Y., Xu, B., Hu, S., Li, Y., Li, Q., and Liu, W. (2015). Simulation of Magnetic Hysteresis Loops and Magnetic Barkhausen Noise of α-iron Containing Nonmagnetic Particles. AIP Adv. 5, 077168. doi:10.1063/1.4927548
Li, G., Qin, S. J., and Yuan, T. (2016). Data-driven Root Cause Diagnosis of Faults in Process Industries. Chemometrics Intell. Lab. Syst. 159, 1–11. doi:10.1016/j.chemolab.2016.09.006
Li, W., Peng, M., Liu, Y., Jiang, N., Wang, H., and Duan, Z. (2018). Fault Detection, Identification and Reconstruction of Sensors in Nuclear Power Plant with Optimized PCA Method. Ann. Nucl. Energ. 113, 105–117. doi:10.1016/j.anucene.2017.11.009
Li, W., Peng, M., and Wang, Q. (2018). False Alarm Reducing in PCA Method for Sensor Fault Detection in a Nuclear Power Plant. Ann. Nucl. Energ. 118, 131–139. doi:10.1016/j.anucene.2018.04.012
Li, W., Peng, M., and Wang, Q. (2019). Improved PCA Method for Sensor Fault Detection and Isolation in a Nuclear Power Plant. Nucl. Eng. Technol. 51, 146–154. doi:10.1016/j.net.2018.08.020
Li, X., Jiang, H., Xiong, X., and Shao, H. (2019). Rolling Bearing Health Prognosis Using a Modified Health index Based Hierarchical Gated Recurrent Unit Network. Mechanism Machine Theor. 133, 229–249. doi:10.1016/j.mechmachtheory.2018.11.005
Li, D., Wang, Y., Wang, J., Wang, C., and Duan, Y. (2020). Recent Advances in Sensor Fault Diagnosis: A Review. Sensors Actuators A: Phys. 309, 111990. doi:10.1016/j.sna.2020.111990
Liao, L., and Kottig, F. (2014). Review of Hybrid Prognostics Approaches for Remaining Useful Life Prediction of Engineered Systems, and an Application to Battery Life Prediction. IEEE Trans. Rel. 63, 191–207. doi:10.1109/TR.2014.2299152
Lin, T.-H., and Wu, S.-C. (2019). Sensor Fault Detection, Isolation and Reconstruction in Nuclear Power Plants. Ann. Nucl. Energ. 126, 398–409. doi:10.1016/j.anucene.2018.11.044
Lin, L., Athe, P., Rouxelin, P., Avramova, M., Gupta, A., Youngblood, R., et al. (2021). Development and Assessment of a Nearly Autonomous Management and Control System for Advanced Reactors. Ann. Nucl. Energ. 150, 107861. doi:10.1016/j.anucene.2020.107861
Liu, J., and Zio, E. (2018). A Scalable Fuzzy Support Vector Machine for Fault Detection in Transportation Systems. Expert Syst. Appl. 102, 36–43. doi:10.1016/j.eswa.2018.02.017
Liu, J., Wang, W., Ma, F., Yang, Y. B., and Yang, C. S. (2012). A Data-Model-Fusion Prognostic Framework for Dynamic System State Forecasting. Eng. Appl. Artif. Intelligence 25, 814–823. doi:10.1016/j.engappai.2012.02.015
Liu, Q., Qin, S. J., and Chai, T. (2013). Decentralized Fault Diagnosis of Continuous Annealing Processes Based on Multilevel PCA. IEEE Trans. Autom Sci. Eng. 10, 687–698. doi:10.1109/TASE.2012.2230628
Liu, J., Vitelli, V., Zio, E., and Seraoui, R. (2015). A Novel Dynamic-Weighted Probabilistic Support Vector Regression-Based Ensemble for Prognostics of Time Series Data. IEEE Trans. Rel. 64, 1203–1213. doi:10.1109/TR.2015.2427156
Liu, Y.-K., Wu, G.-H., Xie, C.-L., Duan, Z.-Y., Peng, M.-J., and Li, M.-K. (2016). A Fault Diagnosis Method Based on Signed Directed Graph and Matrix for Nuclear Power Plants. Nucl. Eng. Des. 297, 166–174. doi:10.1016/j.nucengdes.2015.11.016
Liu, Y-K., Ayodeji, A., Wen, Z., Wu, M., Peng, M., and Yu, W. (2017). A cascade Intelligent Fault Diagnostic Technique for Nuclear Power Plants. J. Nucl. Sci. Technol. 55, 254–266. doi:10.1080/00223131.2017.1394228
Liu, Y., Hu, X., and Zhang, W. (2019). Remaining Useful Life Prediction Based on Health index Similarity. Reliability Eng. Syst. Saf. 185, 502–510. doi:10.1016/j.ress.2019.02.002
Ma, B., Zhao, Y., Zhang, Y., Jiang, Q. L., and Hou, X. Q. (2019). Machinery Early Fault Detection Based on Dirichlet Process Mixture Model. IEEE Access 7, 89226–89233. doi:10.1109/ACCESS.2019.2927104
Makowska, K., Piotrowski, L., and Kowalewski, Z. L. (2017). Prediction of the Mechanical Properties of P91 Steel by Means of Magneto-Acoustic Emission and Acoustic Birefringence. J. Nondestruct Eval. 36, 43. doi:10.1007/s10921-017-0421-9
Mandal, S., Santhi, B., Sridhar, S., Vinolia, K., and Swaminathan, P. (2017). Nuclear Power Plant Thermocouple Sensor Fault Detection and Classification Using Deep Learning and Generalized Likelihood Ratio Test. IEEE Trans. Nucl. Sci. 64, 1. doi:10.1109/TNS.2017.2697919
McCloy, J. S., Ramuhalli, P., and Henager, Jr., C. (2013). Use of First Order Reversal Curve Measurements to Understand Barkhausen Noise Emission in Nuclear Steel. Proc. 39th Annu. Rev. Prog. Quant. Nondestruct. Eval. 1511, 1709–1716. doi:10.1063/1.4789247
Mei, L., Li, H., Zhou, Y., Li, D., Long, W., and Xing, F. (2020). Output-Only Damage Detection of Shear Building Structures Using an Autoregressive Model-Enhanced Optimal Subpattern Assignment Metric. Sensors 20, 2050. doi:10.3390/s20072050
Meng, J., Su, Y., and Xie, S. (2020). Loose Parts Detection Method Combining Blind Deconvolution with Support Vector Machine. Ann. Nucl. Energ. 149, 107782. doi:10.1016/j.anucene.2020.107782
Messai, A., Mellit, A., Abdellani, I., and Massi Pavan, A. (2015). On-line Fault Detection of a Fuel Rod Temperature Measurement Sensor in a Nuclear Reactor Core Using ANNs. Prog. Nucl. Energ. 79, 8–21. doi:10.1016/j.pnucene.2014.10.013
Mirnaghi, M. S., and Haghighat, F. (2020). Fault Detection and Diagnosis of Large-Scale HVAC Systems in Buildings Using Data-Driven Methods: A Comprehensive Review. Energy and Buildings 229, 110492. doi:10.1016/j.enbuild.2020.110492
MIT (2018). The Future of Nuclear Energy in a Carbon-Constrained World: An Interdisciplinary MIT Study. MIT Energy Initiative.
Mnassri, B., El Adel, E. M., and Ouladsine, M. (2015). Reconstruction-based Contribution Approaches for Improved Fault Diagnosis Using Principal Component Analysis. J. Process Control. 33, 60–76. doi:10.1016/j.jprocont.2015.06.004
Moradi, R., and Groth, K. M. (2020). Modernizing Risk Assessment: A Systematic Integration of PRA and PHM Techniques. Reliability Eng. Syst. Saf. 204, 107194. doi:10.1016/j.ress.2020.107194
Morana, A., Mellier, F., Cheymol, G., Destouches, C., Di Salvo, J., Girard, S., et al. (2016). Irradiation Campaign in the EOLE Critical Facility of Fiber Optic Bragg Gratings Dedicated to the Online Temperature Measurement in Zero Power Research Reactors. IEEE Trans. Nucl. Sci. 63, 2887–2894. doi:10.1109/TNS.2016.2618906
Moshkbar-Bakhshayesh, K. (2021). Investigating the Performance of the Supervised Learning Algorithms for Estimating NPPs Parameters in Combination with the Different Feature Selection Techniques. Ann. Nucl. Energ. 158, 108299. doi:10.1016/j.anucene.2021.108299
Mujica, L., Rodellar, J., Fernández, A., and Güemes, A. (2011). Q-statistic and T2-Statistic PCA-Based Measures for Damage Assessment in Structures. Struct. Health Monit. 10, 539–553. doi:10.1177/1475921710388972
Mundra, P. A., and Rajapakse, J. C. (2010). SVM-RFE with MRMR Filter for Gene Selection. IEEE Trans.on Nanobioscience 9, 31–37. doi:10.1109/TNB.2009.2035284
Nain, A., and Varde, P. V. (2013). “Fuzzy Rule-Based Approach for Diagnostics in Nuclear Plant Diesel Generators,” in Proceedings of the International Symposium on Engineering under Uncertainty: Safety Assessment and Management (ISEUSAM - 2012) January, 2012, Kolkata, India (Springer India), 981–996. doi:10.1007/978-81-322-0757-3_67
Narendra, P. M., and Fukunaga, K. (1977). A Branch and Bound Algorithm for Feature Subset Selection. IEEE Trans. Comput. C-26, 917–922. doi:10.1109/TC.1977.1674939
NEI (2011). Nuclear Energy’s Economic Benefits - Current and Future (White Paper). Washington, DC: Nuclear Energy Institute.
NEI (2021a). US Nuclear Generating Statistics. Washington, DC: Nuclear Energy InstituteAvailable at: https://www.nei.org/resources/statistics/us-nuclear-generating-statistics. Retrieved in January 2021
NEI (2021b). Nuclear Provides Carbon-free Energy 24/7. Washington, DC: Nuclear Energy InstituteAvailable at: https://www.nei.org/fundamentals/nuclear-provides-carbon-free-energy. Retrieved in January 2021
NEI (2021c). Initial License Renewal Filings for US Nuclear Power Plants. Washington, DC: Nuclear Energy InstituteAvailable at: https://www.nei.org/resources/statistics/us-nuclear-license-renewal-filings Accessed January 29, 2021. Retrieved in January 2021
Nguyen, H-P., Fauriat, W., Zio, E., and Liu, J. (2018). “A Data-Driven Approach for Predicting the Remaining Useful Life of Steam Generators,” in 2018 3rd International Conference on System Reliability and Safety (ICSRS). Barcelona, Spain. doi:10.1109/ICSRS.2018.0004910.1109/icsrs.2018.8688716
Paris, P., and Erdogan, F. (1963). A Critical Analysis of Crack Propagation Laws. J. Basic Eng. 85, 528–533. doi:10.1115/1.3656900
Park, W. H., Packo, P., and Kundu, T. (2017). Acoustic Source Localization in an Anisotropic Plate without Knowing its Material Properties - A New Approach. Ultrasonics 79, 9–17. doi:10.1016/j.ultras.2017.02.021
Peng, B.-S., Xia, H., Liu, Y.-K., Yang, B., Guo, D., and Zhu, S.-M. (2018). Research on Intelligent Fault Diagnosis Method for Nuclear Power Plant Based on Correlation Analysis and Deep Belief Network. Prog. Nucl. Energ. 108, 419–427. doi:10.1016/j.pnucene.2018.06.003
Peng, M.-j., Wang, H., Chen, S.-s., Xia, G.-l., Liu, Y.-k., Yang, X., et al. (2018). An Intelligent Hybrid Methodology of On-Line System-Level Fault Diagnosis for Nuclear Power Plant. Nucl. Eng. Technol. 50, 396–410. doi:10.1016/j.net.2017.11.014
Pham, B. T., Agarwal, V., Lybeck, N. J., and Tawfik, M. S. (2012). Prognostic Health Monitoring System: Component Selection Based on Risk Criteria and Economic Benefit Assessment. Technical Report, INL/CON-11-23571. Idaho Falls: Idaho National Laboratory.
Power Engineering International (2017). Offline Reactor Costing EDF $1.2m Per Day. Available at: https://www.powerengineeringint.com/decentralized-energy/equipment-technology/offline-reactor-costing-edf-1-2m-per-day/.
Pudil, P., and Somol, P. (2008). Identifying the Most Informative Variables for Decision-Making Problems - a Survey of Recent Approaches and Accompanying Problems. Aop 16, 37–55. doi:10.18267/j.aop.131
Qian, Y., Yan, R., and Hu, S. (2014). Bearing Degradation Evaluation Using Recurrence Quantification Analysis and Kalman Filter. IEEE Trans. Instrum. Meas. 63, 2599–2610. doi:10.1109/TIM.2014.2313034
Qing, X., Kumar, A., Zhang, C., Gonzalez, I. F., Guo, G., and Chang, F.-K. (2005). A Hybrid Piezoelectric/fiber Optic Diagnostic System for Structural Health Monitoring. Smart Mater. Struct. 14, S98–S103. doi:10.1088/0964-1726/14/3/012
Qiu, J., Seth, B. B., Liang, S. Y., and Zhang, C. (2002). Damage Mechanics Approach for Bearing Lifetime Prognostics. Mech. Syst. Signal Process. 16, 817–829. doi:10.1006/mssp.2002.1483
Qiu, L., Deng, X., Yuan, S., Huang, Y., and Ren, Y. (2018). Impact Monitoring for Aircraft Smart Composite Skins Based on a Lightweight Sensor Network and Characteristic Digital Sequences. Sensors 18, 2218–2227. doi:10.3390/s18072218
Ramuhalli, P., Bond, L. J., Griffin, J. W., Dixit, M., and Henager, C. H. (2010). “A Bayesian Prognostic Algorithm for Assessing Remaining Useful Life of Nuclear Power Components,” in Proc. 7th Int. Top. Meet. Nucl. Plant Instrumentation, Control. Human-Machine Interface Technol. (NPIC&HMIT 2010), Las Vegas, NV, USA, 875–886.
Ramuhalli, P., Griffin, J. W., Fricke, J. M., and Bond, L. J. (2012). An Assessment of Uncertainty in Remaining Life Estimation for Nuclear Structural Materials. Proc. 8th Int. Top. Meet. Nucl. Plant Instrumentation, Control. San Diego, CA, USA, 1325–1337.
Ramuhalli, P., Hirt, E. H., Coles, G. A., Bonebrake, C. A., Ivans, W. J., Wootan, D. W., et al. (2014). An Updated Methodology for Enhancing Risk Monitors with Integrated Equipment Condition Assessment. Technical Report, PNNL-23478. Richland, WA: Pacific Northwest National Laboratory. doi:10.2172/1168934
Ramuhalli, P., Veeramany, A., Bonebrake, C. A., Ivans, W. J., Coles, G. A., and Hirt, E. H. (2016). “Evaluation of Enhanced Risk Monitors for Use on Advanced Reactors,” in Proc. 24th Int. Conf. Nucl. Eng. Charlotte, NC, USA. doi:10.1115/ICONE24-60040
Ramuhalli, P., Bonebrake, C. A., Dib, G., Roy, S., and Cetiner, S. (2017). “Integration and Assessment of Component Health Prognostics in Supervisory Control Systems,” in Proc. 10th Int. Top. Meet. Nucl. Plant Instrumentation, Control Hum. Mach. Interface Technol. (San Francisco, CA, USA, 1423–1431.
Ramuhalli, P., Walker, C., Agarwal, V., and Lybeck, N. J. (2020). Development of Prognostic Models Using Plant Asset Data. Technical Report, ORNL/TM-2020/1697. Oak Ridge, TN: Oak Ridge National Laboratory. doi:10.2172/1661211
Rasmussen, C. E. (2004). Gaussian Processes in Machine Learning in Lect. Notes Comput. Sci. (Berlin, Heidelberg: Springer), 63–71. doi:10.1007/978-3-540-28650-9_4
Rato, T. J., and Reis, M. S. (2013). Fault Detection in the Tennessee Eastman Benchmark Process Using Dynamic Principal Components Analysis Based on Decorrelated Residuals (DPCA-DR). Chemometrics Intell. Lab. Syst. 125, 101–108. doi:10.1016/j.chemolab.2013.04.002
Reinhardt, B., Daw, J., and Tittmann, B. R. (2018). Irradiation Testing of Piezoelectric (Aluminum Nitride, Zinc Oxide, and Bismuth Titanate) and Magnetostrictive Sensors (Remendur and Galfenol). IEEE Trans. Nucl. Sci. 65, 533–538. doi:10.1109/TNS.2017.2775163
Ren, J., Ren, R., Green, M., and Huang, X. (2019). A Deep Learning Method for Fault Detection of Autonomous Vehicles. 2019 14th International Conference on Computer Science & Education (ICCSE), August 19-21, 2019, Toronto, ON, Canada. 749–754. doi:10.1109/ICCSE.2019.8845483
Rezaeianjouybari, B., and Shang, Y. (2020). Deep Learning for Prognostics and Health Management: State of the Art, Challenges, and Opportunities. Measurement 163, 107929. doi:10.1016/j.measurement.2020.107929
Rodríguez Ramos, A., Domínguez Acosta, C., Rivera Torres, P. J., Serrano Mercado, E. I., Beauchamp Baez, G., Rifón, L. A., et al. (2019). An Approach to Multiple Fault Diagnosis Using Fuzzy Logic. J. Intell. Manuf 30, 429–439. doi:10.1007/s10845-016-1256-4
Romero, E., and Sopena, J. M. (2008). Performing Feature Selection With Multilayer Perceptrons. IEEE Trans. Neural Netw. 19, 431–441. doi:10.1109/TNN.2007.909535
Saeed, H. A., Peng, M.-j., Wang, H., and Zhang, B.-w. (2020). Novel Fault Diagnosis Scheme Utilizing Deep Learning Networks. Prog. Nucl. Energ. 118, 103066. doi:10.1016/j.pnucene.2019.103066
Saeys, Y., Inza, I., and Larranaga, P. (2007). A Review of Feature Selection Techniques in Bioinformatics. Bioinformatics 23, 2507–2517. doi:10.1093/bioinformatics/btm344
Saha, B., and Goebel, K. (2008). “Uncertainty Management for Diagnostics and Prognostics of Batteries Using Bayesian Techniques,” in 2008 IEEE Aerospace Conference, Big Sky, MT, USA. doi:10.1109/AERO.2008.4526631
Sargent, , and Lundy, L. L. C. (2018). Nuclear Power Plant Life Extension Cost Development Methodology. Final Report, Project 13527-001. Chicago, IL: Eastern Research Group, Inc., US Environmental Protection Agency.
Saxena, A., Celaya, J., Saha, B., Saha, S., and Goebel, K. (2010). Metrics for Offline Evaluation of Prognostic Performance. Int. J. Progn Heal Manag. 1, 1–20. doi:10.36001/ijphm.2010.v1i1.1336
Setiono, R., and Liu, H. (1997). Neural-network Feature Selector. IEEE Trans. Neural Netw. 8, 654–662. doi:10.1109/72.572104
Severson, K., Chaiwatanodom, P., and Braatz, R. D. (2016). Perspectives on Process Monitoring of Industrial Systems. Annu. Rev. Control. 42, 190–200. doi:10.1016/j.arcontrol.2016.09.001
Sharma, T., Je, S.-S., Gill, B., and Zhang, J. X. J. (2012). Patterning Piezoelectric Thin Film PVDF-TrFE Based Pressure Sensor for Catheter Application. Sensors Actuators A: Phys. 177, 87–92. doi:10.1016/j.sna.2011.08.019
Shetgaonkar, S. S. (2017). Fault Diagnosis in Induction Motor Using Fuzzy Logic. 2017 International Conference on Computing Methodologies and Communication (ICCMC), July 18-19, 2017, Erode, India, 289–293. doi:10.1109/ICCMC.2017.8282693
Shi, Z., and Chehade, A. (2021). A Dual-LSTM Framework Combining Change point Detection and Remaining Useful Life Prediction. Reliability Eng. Syst. Saf. 205, 107257. doi:10.1016/j.ress.2020.107257
Shin, S. M., Choi, B. H., and Kang, H. G. (2016). Motor Health Monitoring at Standstill Through Impedance Analysis. IEEE Trans. Ind. Electron. 63, 4422–4431. doi:10.1109/TIE.2016.2541089
Si, L., and Baier, H. (2015). Real-time Impact Visualization Inspection of Aerospace Composite Structures with Distributed Sensors. Sensors 15, 16536–16556. doi:10.3390/s150716536
Si, X.-S., Wang, W., Hu, C.-H., and Zhou, D.-H. (2011). Remaining Useful Life Estimation - A Review on the Statistical Data Driven Approaches. Eur. J. Oper. Res. 213, 1–14. doi:10.1016/j.ejor.2010.11.018
Somol, P., Pudil, P., Novovičová, J., and Paclı́k, P. (1999). Adaptive Floating Search Methods in Feature Selection. Pattern Recognition Lett. 20, 1157–1163. doi:10.1016/S0167-8655(99)00083-5
Spasojevic, D., Bukvic, S., Milosevic, S., and Stanley, H. E. (1996). Barkhausen Noise: Elementary Signals, Power Laws, and Scaling Relations. Phys. Rev. E Stat. Phys. Plasmas Fluids Relat. Interdiscip. Top. 54, 2531–2546. doi:10.1103/physreve.54.2531
Stefanita, C.-G. (2008). From Bulk to Nano: The Many Sides of Magnetism, 117. Springer Berlin Heidelberg. doi:10.1007/978-3-540-70548-2
Stefatos, G., and Ben Hamza, A. (2010). Dynamic Independent Component Analysis Approach for Fault Detection and Diagnosis. Expert Syst. Appl. 37, 8606–8617. doi:10.1016/j.eswa.2010.06.101
Sun, B., Shengkui Zeng, S., Kang, R., and Pecht, M. (2010). “Benefits Analysis of Prognostics in Systems,” in 2010 Prognostics and System Health Management Conference. Macao, China. doi:10.1109/PHM.2010.5413503
Sun, B., Jiang, X., Ye, T., and Feng, Q. (2016). A Novel Concept and Assessment Method for Trustworthiness of Prognostics. Adv. Mech. Eng. 8, 168781401663880–10. doi:10.1177/1687814016638807
Sutharssan, T., Stoyanov, S., Bailey, C., and Yin, C. (2015). Prognostic and Health Management for Engineering Systems: a Review of the Data‐driven Approach and Algorithms. J. Eng. 2015, 215–222. doi:10.1049/joe.2014.0303
Tagaris, T., Ioannou, G., Sdraka, M., Alexandridis, G., and Stafylopatis, A. (2019). “Putting Together Wavelet-Based Scaleograms and Convolutional Neural Networks for Anomaly Detection in Nuclear Reactors,” in ICAAI 2019: Proceedings of the 2019 3rd International Conference on Advances in Artificial Intelligence, New York, NY, USA, 237–43. doi:10.1145/3369114.3369121
Taheri, E., Kolmanovsky, I., and Gusikhin, O. (2019). Survey of Prognostics Methods for Condition-Based Maintenance in Engineering Systems. arXiv:1912.02708.
Takahashi, T., and Matsumoto, E. (2009). Measurement and Simulation of Detection of Defects on Inner wall of Cylindrical Pipe by Piezoelectric High-Polymer Film. Proc. 6th Annu. Meet. Japan Soc. Maintenology, Sapporo. Hokkaido, Japan, 409–12.
Thrun, S., Montemerlo, M., Dahlkamp, H., Stavens, D., Aron, A., Diebel, J., et al. (2006). Stanley: The Robot that Won the DARPA Grand Challenge. J. Field Robotics 23, 661–692. doi:10.1002/rob.20147
Tolo, S., Tian, X., Bausch, N., Becerra, V., Santhosh, T. V., Vinod, G., et al. (2019). Robust On-Line Diagnosis Tool for the Early Accident Detection in Nuclear Power Plants. Reliability Eng. Syst. Saf. 186, 110–119. doi:10.1016/j.ress.2019.02.015
US DOE (2019). Infographic - Capacity Factor by Energy Source. Washington, DC: US Department of Energy, Office of Nuclear Energy Available at: https://www.energy.gov/ne/downloads/infographic-capacity-factor-energy-source-2019.
US DOE (2020a). What’s the Lifespan for a Nuclear Reactor? Much Longer Than You Might Think. Washington, DC: US Department of Energy, Office of Nuclear EnergyAvailable at: https://www.energy.gov/ne/articles/whats-lifespan-nuclear-reactor-much-longer-you-might-think.
US DOE (2020b). NRC Approves First US Small Modular Reactor Design. Washington, DC: US Department of Energy, Office of Nuclear EnergyAvailable at: https://www.energy.gov/ne/articles/nrc-approves-first-us-small-modular-reactor-design
US DOE (2020c). First US Small Modular Boiling Water Reactor Under Development. Washington, DC: US Department of Energy, Office of Nuclear EnergyAvailable at: https://www.energy.gov/ne/articles/first-us-small-modular-boiling-water-reactor-under-development.
US DOE (2020d). US Department of Energy Announces $160 Million in First Awards under Advanced Reactor Demonstration Program. Washington, DC: US Department of Energy, Office of Nuclear EnergyAvailable at: https://www.energy.gov/ne/articles/us-department-energy-announces-160-million-first-awards-under-advanced-reactor.
US EIA (2021a). Nuclear and Coal Will Account for Majority of US Generating Capacity Retirements in 2021. Washington, DC: US Energy Information AdministrationAvailable at: https://www.eia.gov/todayinenergy/detail.php?id=46436.
US EIA (2021b). How Old Are US Nuclear Power Plants, and when Was the Newest One Built? US Energy Information Administration. Available at: https://www.eia.gov/tools/faqs/faq.php?id=228&t=21. Retrieved in January 2021.
US EIA (2021c). Nuclear Explained: US Nuclear Industry. Washington, DC: US Energy Information AdministrationAvailable at: https://www.eia.gov/energyexplained/nuclear/us-nuclear-industry.php. Retrieved in January 2021.
US NRC (1995). Requirements for Renewal of Operating Licenses for Nuclear Power Plants. 10 CFR 54. Final Rule. Available at: https://www.nrc.gov/reading-rm/doc-collections/cfr/part054/full-text.html Accessed January 29, 2021
US NRC (2021a). Status of Initial License Renewal Applications and Industry Initiatives. Washington, DC: US Nuclear Regulatory CommissionAvailable at: https://www.nrc.gov/reactors/operating/licensing/renewal/applications.html. Retrieved in January 2021.
US NRC (2021b). Status of Subsequent License Renewal Applications. Washington, DC: US Nuclear Regulatory CommissionAvailable at: https://www.nrc.gov/reactors/operating/licensing/renewal/subsequent-license-renewal.html. Retrieved in January 2021.
US NRC (2021c). § 50.65 Requirements for Monitoring the Effectiveness of Maintenance at Nuclear Power Plants. US Nuclear Regulatory Commission. Available at: https://www.nrc.gov/reading-rm/doc-collections/cfr/part050/part050-0065.html. Retrieved in January 2021.
US NRC (2021d). § 50.55a Codes and Standards. US Nuclear Regulatory Commission. Available at: https://www.nrc.gov/reading-rm/doc-collections/cfr/part050/part050-0055a.html. Retrieved in January 2021.
Venkatasubramanian, V. (2005). Prognostic and Diagnostic Monitoring of Complex Systems for Product Lifecycle Management: Challenges and Opportunities. Comput. Chem. Eng. 29, 1253–1263. doi:10.1016/j.compchemeng.2005.02.026
Verikas, A., and Bacauskiene, M. (2002). Feature Selection with Neural Networks. Pattern Recognition Lett. 23, 1323–1335. doi:10.1016/S0167-8655(02)00081-8
Vogl, G. W., Weiss, B. A., and Donmez, M. A. (2014). Standards for Prognostics and Health Management (PHM) Techniques within Manufacturing Operations. Proc. Annu. Conf. Progn. Heal. Manag. Soc. Fort Worth, TX, USA.
Vogl, G. W., Weiss, B. A., and Helu, M. (2019). A Review of Diagnostic and Prognostic Capabilities and Best Practices for Manufacturing. J. Intell. Manuf 30, 79–95. doi:10.1007/s10845-016-1228-8
Wadhwa, N., Peng, B., Yang, B., Zhu, S., Zhang, J., et al. (2021). A Multi-Stage Hybrid Fault Diagnosis Approach for Operating Conditions of Nuclear Power Plant. Ann. Nucl. Energ. 153, 108015. doi:10.1016/j.anucene.2020.108015
Wang, X., Song, T., Gong, F., and Zheng, P. (2016). On the Computational Power of Spiking Neural P Systems with Self-Organization. Sci. Rep. 6, 27624. doi:10.1038/srep27624
Wang, Y., Liu, Y., Khan, F., and Imtiaz, S. (2017). Semiparametric PCA and Bayesian Network Based Process Fault Diagnosis Technique. Can. J. Chem. Eng. 95, 1800–1816. doi:10.1002/cjce.22829
Wang, Y., Yang, H., Yuan, X., and Cao, Y. (2018). An Improved Bayesian Network Method for Fault Diagnosis. IFAC-PapersOnLine 51, 341–346. doi:10.1016/j.ifacol.2018.09.443
Wang, J., Peng, H., Yu, W., Ming, J., Pérez-Jiménez, M. J., Tao, C., et al. (2019). Interval-valued Fuzzy Spiking Neural P Systems for Fault Diagnosis of Power Transmission Networks. Eng. Appl. Artif. Intelligence 82, 102–109. doi:10.1016/j.engappai.2019.03.014
Wang, H., Peng, M.-j., Wesley Hines, J., Zheng, G.-y., Liu, Y.-k., and Upadhyaya, B. R. (2019). A Hybrid Fault Diagnosis Methodology with Support Vector Machine and Improved Particle Swarm Optimization for Nuclear Power Plants. ISA Trans. 95, 358–371. doi:10.1016/j.isatra.2019.05.016
Wang, B., Lei, Y., Li, N., and Yan, T. (2019). Deep Separable Convolutional Network for Remaining Useful Life Prediction of Machinery. Mech. Syst. Signal Process. 134, 106330. doi:10.1016/j.ymssp.2019.106330
Wang, Y., He, M., Sun, L., Wu, D., Wang, Y., and Zou, L. (2020). Improved Kalman Filtering-Based Information Fusion for Crack Monitoring Using Piezoelectric-Fiber Hybrid Sensor Network. Front. Mater. 7, 300. doi:10.3389/fmats.2020.00300
Wang, Y., Zhao, Y., and Addepalli, S. (2020). Remaining Useful Life Prediction Using Deep Learning Approaches: A Review. Proced. Manufacturing 49, 81–88. doi:10.1016/j.promfg.2020.06.015
Wang, Z., Xia, H., Peng, B., Yang, B., Zhu, S., Zhang, J., et al. (2021). A Multi-Stage Hybrid Fault Diagnosis Approach for Operating Conditions of Nuclear Power Plant. Ann. Nucl. Energ. 153, 108015. doi:10.1016/j.anucene.2020.108015
Wood, R. T., Upadhyaya, B. R., and Floyd, D. C. (2017). An Autonomous Control Framework for Advanced Reactors. Nucl. Eng. Technol. 49, 896–904. doi:10.1016/j.net.2017.07.001
Wu, Z., Qing, X. P., and Chang, F-K. (2009). Damage Detection for Composite Laminate Plates with A Distributed Hybrid PZT/FBG Sensor Network. J. Intell. Mater. Syst. Struct. 20, 1069–77. doi:10.1177/1045389X08101632
Wu, C., Zhu, C., and Ge, Y. (2017). A New Fault Diagnosis and Prognosis Technology for High-Power Lithium-Ion Battery. IEEE Trans. Plasma Sci. 45, 1533–1538. doi:10.1109/TPS.2017.2706088
Wu, G., Tong, J., Zhang, L., Zhao, Y., and Duan, Z. (2018). Framework for Fault Diagnosis with Multi-Source Sensor Nodes in Nuclear Power Plants Based on a Bayesian Network. Ann. Nucl. Energ. 122, 297–308. doi:10.1016/j.anucene.2018.08.050
Wu, Y., Yuan, M., Dong, S., Lin, L., and Liu, Y. (2018). Remaining Useful Life Estimation of Engineered Systems Using Vanilla LSTM Neural Networks. Neurocomputing 275, 167–179. doi:10.1016/j.neucom.2017.05.063
Xin, M., Jiao, W., and Da-zi, L. (2019). Fault Diagnosis of Nuclear Power Plant Based on Simplified Signed Directed Graph with Principal Component Analysis and Support Vector Machine. Proc. 2019 Chin. Autom. Congr., Hangzhou, China, 3082–3087. doi:10.1109/CAC48633.2019.8997001
Xu, Z., King, I., Lyu, M. R., and Jin, R. (2010). Discriminative Semi-supervised Feature Selection via Manifold Regularization. IEEE Trans. Neural Netw. 21, 1033–47. doi:10.1109/TNN.2010.2047114
Xu, X., Yan, X., Sheng, C., Yuan, C., Xu, D., and Yang, J. (2017). A Belief Rule-Based Expert System for Fault Diagnosis of Marine Diesel Engines. IEEE Trans. Syst. Man. Cybern, Syst. 50, 1–17. doi:10.1109/TSMC.2017.2759026
Xu, B., Li, H., Pang, W., Chen, D., Tian, Y., Lei, X., et al. (2019). Bayesian Network Approach to Fault Diagnosis of a Hydroelectric Generation System. Energy Sci Eng 7, 1669–1677. doi:10.1002/ese3.383
Yadav, V., Agarwal, V., Gribok, A. V., and Smith, C. L. (2018). Modelling Component Failure Rates Utilizing Sensor-Based Degradation Data. Proc. 14th Probabilistic Saf. Assess. Manag. Conf. Los Angeles, CA, USA.
Yan, H., Xu, Y., Cai, F., Zhang, H., Zhao, W., and Gerada, C. (2019). PWM-VSI Fault Diagnosis for a PMSM Drive Based on the Fuzzy Logic Approach. IEEE Trans. Power Electron. 34, 759–768. doi:10.1109/TPEL.2018.2814615
Yang, J., and Ong, C.-J. (2011). Feature Selection Using Probabilistic Prediction of Support Vector Regression. IEEE Trans. Neural Networks 22, 954–62. doi:10.1109/TNN.2011.2128342
Yang, R., and Rizzoni, G. (2016). Comparison of Model Based vs. Data-Driven Methods for Fault Detection and Isolation in Engine Idle Speed Control System. Proc. Annu. Conf. Progn. Heal. Manag. Soc. 7, 25–33. doi:10.36001/phmconf.2016.v8i1.2502
Yang, Q. (2004). Model-based and Data Driven Fault Diagnosis Methods with Applications to Process Monitoring. Ph.D. Thesis. Cleveland, OH: Case Western Reserve University.
Ye, Z.-S., and Xie, M. (2015). Stochastic Modelling and Analysis of Degradation for Highly Reliable Products. Appl. Stochastic Models Bus. Ind. 31, 16–32. doi:10.1002/asmb.2063
Yildiz, B. (2003). Development of a Hybrid Intelligent System for On-Line Real-Time Monitoring of Nuclear Power Plant Operations. Ph.D. Thesis. MIT.
Yu, W., and Zhao, C. (2019). Online Fault Diagnosis for Industrial Processes With Bayesian Network-Based Probabilistic Ensemble Learning Strategy. IEEE Trans. Automat. Sci. Eng. 16, 1922–1932. doi:10.1109/TASE.2019.2915286
Yu, D., Chen, Z. M., Xiahou, K. S., Li, M. S., Ji, T. Y., and Wu, Q. H. (2018). A Radically Data-Driven Method for Fault Detection and Diagnosis in Wind Turbines. Int. J. Electr. Power Energ. Syst. 99, 577–584. doi:10.1016/j.ijepes.2018.01.009
Yu, S.-Z. (2010). Hidden Semi-Markov Models. Artif. Intelligence 174, 215–243. doi:10.1016/j.artint.2009.11.011
Yu, J. (2012). A Nonlinear Kernel Gaussian Mixture Model Based Inferential Monitoring Approach for Fault Detection and Diagnosis of Chemical Processes. Chem. Eng. Sci. 68, 506–519. doi:10.1016/j.ces.2011.10.011
Yucesan, Y. A., and Viana, F. A. C. (2020). A Physics-Informed Neural Network for Wind Turbine Main Bearing Fatigue. Int. J. Progn Heal Manag. 11, 1–17. doi:10.36001/ijphm.2020.v11i1.2594
Zhang, X., Wu, G., Dong, Z., and Crawford, C. (2015). Embedded Feature-Selection Support Vector Machine for Driving Pattern Recognition. J. Franklin Inst. 352, 669–685. doi:10.1016/j.jfranklin.2014.04.021
Zhang, X., Qiu, D., and Chen, F. (2015). Support Vector Machine with Parameter Optimization by a Novel Hybrid Method and its Application to Fault Diagnosis. Neurocomputing 149, 641–651. doi:10.1016/j.neucom.2014.08.010
Zhang, J., Wang, P., Yan, R., and Gao, R. X. (2018). Long Short-Term Memory for Machine Remaining Life Prediction. J. Manufacturing Syst. 48, 78–86. doi:10.1016/j.jmsy.2018.05.011
Zhao, X., and Golay, M. (2020). Symptom-Based Conditional Failure Probability Estimation for Selected Structures, Systems, and Components. Technical Report, MIT-ANP-TR-188. doi:10.13140/RG.2.2.26898.58569
Zhao, Y., Di Maio, F., Zio, E., Zhang, Q., Dong, C.-L., and Zhang, J.-Y. (2017). Optimization of a Dynamic Uncertain Causality Graph for Fault Diagnosis in Nuclear Power Plant. Nucl. Sci. Tech. 28, 34. doi:10.1007/s41365-017-0184-0
Zhao, R., Yan, R., Wang, J., and Mao, K. (2017). Learning to Monitor Machine Health with Convolutional Bi-Directional LSTM Networks. Sensors 17, 273. doi:10.3390/s17020273
Zhao, R., Wang, D., Yan, R., Mao, K., Shen, F., and Wang, J. (2018). Machine Health Monitoring Using Local Feature-Based Gated Recurrent Unit Networks. IEEE Trans. Ind. Electron. 65, 1539–1548. doi:10.1109/TIE.2017.2733438
Zhao, X., Shirvan, K., Salko, R. K., and Guo, F. (2020). On the Prediction of Critical Heat Flux Using a Physics-Informed Machine Learning-Aided Framework. Appl. Therm. Eng. 164, 114540. doi:10.1016/j.applthermaleng.2019.114540
Zhao, Y., Tong, J., Zhang, L., and Wu, G. (2020). Diagnosis of Operational Failures and On-Demand Failures in Nuclear Power Plants: An Approach Based on Dynamic Bayesian Networks. Ann. Nucl. Energ. 138, 107181. doi:10.1016/j.anucene.2019.107181
Zhou, T., Droguett, E. L., and Modarres, M. (2020). A Common Cause Failure Model for Components under Age-Related Degradation. Reliability Eng. Syst. Saf. 195, 106699. doi:10.1016/j.ress.2019.106699
Zhu, J., Chen, N., and Peng, W. (2019). Estimation of Bearing Remaining Useful Life Based on Multiscale Convolutional Neural Network. IEEE Trans. Ind. Electron. 66, 3208–3216. doi:10.1109/TIE.2018.2844856
Zio, E., Baraldi, P., and Pedroni, N. (2006). Selecting Features for Nuclear Transients Classification by Means of Genetic Algorithms. IEEE Trans. Nucl. Sci. 53, 1479–1493. doi:10.1109/TNS.2006.873868
Glossary
ADC analog-to-digital converter
AI artificial intelligence
ANN artificial neural network
BN Bayesian network
BWR boiling water reactor
CBM condition-based maintenance
CFR Code of Federal Regulations
CM condition monitoring
C-MAPSS commercial modular aero-propulsion system simulation
CNN convolutional neural network
DBN dynamic Bayesian network
DES discrete event system
DL deep learning
DOE Department of Energy (US)
DP dynamic programming
DT digital twin
ENN Elman neural network
ERM enhanced risk monitor
FBG fiber Bragg grating
FDA Fisher discriminant analysis
FFNN feed-forward neural network
FWCS feedwater and condensate system
GHG greenhouse gas
GMM Gaussian mixture model
GPR Gaussian process regression
GRU gated recurrent unit
I&C instrumentation and control
ICA independent component analysis
IEA International Energy Agency
IEEE Institute of Electrical and Electronics Engineers
ISO International Organization for Standardization
LMI laser-based mechanical impedance
LSTM long short-term memory
LTO long-term operation
LWR light water reactor
MAE magneto acoustic emission
MBN magnetic Barkhausen noise
MDP Markov decision process
ML machine learning
NAMAC nearly autonomous management and control
NEA Nuclear Energy Agency
NF neuro-fuzzy
NPP nuclear power plant
NRC Nuclear Regulatory Commission (US)
O&M operations & maintenance
OP operating procedure
PCA principal component analysis
PHM prognostics and health management
PLS partial least squares
POF probability of failure
POMDP partially observable Markov decision process
PRA probabilistic risk assessment
PWAS piezoelectric wafer active sensor
PZT piezoelectric
RBF radial basis function
RNN recurrent neural network
RPV reactor pressure vessel
RUL remaining useful life
SCS supervisory control system
SMR small modular reactor
SNN spiking neural network
SSC structure, system, and component
SVM support vector machine
US United States
V&V verification and validation
Keywords: prognostics and health management, planning and decision-making, condition-based maintenance, artificial intelligence, machine learning, data-driven methods, nuclear power plant
Citation: Zhao X, Kim J, Warns K, Wang X, Ramuhalli P, Cetiner S, Kang HG and Golay M (2021) Prognostics and Health Management in Nuclear Power Plants: An Updated Method-Centric Review With Special Focus on Data-Driven Methods. Front. Energy Res. 9:696785. doi: 10.3389/fenrg.2021.696785
Received: 17 April 2021; Accepted: 26 May 2021;
Published: 15 June 2021.
Edited by:
Wenzhong Zhou, Sun Yat-Sen University, ChinaReviewed by:
Sameer Al-Dahidi, German Jordanian University, JordanGyunyoung Heo, Kyung Hee University, South Korea
Copyright © 2021 Zhao, Kim, Warns, Wang, Ramuhalli, Cetiner, Kang and Golay. This is an open-access article distributed under the terms of the Creative Commons Attribution License (CC BY). The use, distribution or reproduction in other forums is permitted, provided the original author(s) and the copyright owner(s) are credited and that the original publication in this journal is cited, in accordance with accepted academic practice. No use, distribution or reproduction is permitted which does not comply with these terms.
*Correspondence: Xingang Zhao, zhaox2@ornl.gov