- 1Intelligent Efficiency Research Group (IERG), School of Engineering, University College Cork, Cork, Ireland
- 2Building Energy Research Group, Loughborough University, Loughborough, United Kingdom
Achieving energy flexibility is becoming a key concern for energy system planners that manage intermittent and variable generations. Industries have enormous potential to deliver large-scale energy flexibility through demand response (DR) programs. This industrial demand flexibility achieved through the demand response programs will enable widespread adoption of renewable sources in the electricity grid network. This paper aims to provide a comprehensive review of demand response and it’s industrial application by addressing: 1) Current research status, 2) Current stages of demand response applications in industries, and 3) Barriers in the deployment of DR programs. This study shows that there is significant research progress in recent years in the field of DR. It also shows potential applications of DR programs in industries. However, the study found several technical, policy, and financial barriers still exist, limiting the widespread adoption of DR. Thus, this paper offers recommendations on technical, policy, and financial measures needed to over-come the barriers and help facilitate the utilization of demand response potential, especially in industries.
Introduction
The need for cleaner energy generation has resulted in more Distributed Energy Resources (DER) integrating into electricity grids, creating new challenges to maintain a stable operation for power system operators. To accommodate this new energy generation mix, energy systems are forced to undergo rapid transformation (Antonopoulos et al., 2020). Most of the DERs are inherently intermittent, i.e., highly volatile depending on the weather dependencies. Hence it is quite challenging to predict their electrical power output. It also creates grid stability and reliability problem because of congestion and voltage issues (Eftekharnejad et al., 2013). As a result, the electricity grid operator requires more flexibility to safeguard their daily operation and maintain the grid’s stability.
The electrical grid operation is currently entering an era where many new services have been introduced thanks to the formulation of the smart grid concept to tackle the challenges posed by integrating DERs into the electricity grid. Demand Response (DR) is a modern service that helps the grid operator provide flexibility to their system. Traditionally DR is a mechanism in which customers are provided with incentives by the grid or utility operator in response to the change of their electricity consumption behavior by responding to the grid operator’s event signal (Palensky and Dietrich 2011). The grid operator aims to reduce the critical peak demand and transfer the peak consumption between different low consumption periods (Huang et al., 2019). The smart grid’s demand response capabilities enable supply and demand to interact with each other by exchanging price and demand information to decide on energy usage (Deng et al., 2015). Provided with sufficient incentives, the customers are willing to change their energy usage pattern to a trade-off between their comfort and electricity bills. Many pilot projects have already been developed involving demand response program demonstrations worldwide (Handa et al., 2008).
Industrial plants are extensive energy consumers and already have an infrastructure with sensors, metering technologies, and operators. Hence more substantial participation in demand response programs than residential or commercial might be expected. However, based on recent data, it is suggested an opposite trend exists. The lower participation rate in the industrial demand response program is due to the lack of proper knowledge sharing between the stakeholders, technical constraints, technical complexity of application due to variation in an industrial process, and dependencies and reliability concerns (Shafie-Khah et al., 2019).
Motivation and Scope of Review
The rising interest in demand response research is well illustrated by the sharp increase in research interest in this domain. The increased scientific publication in this field requires a comprehensive review that points out the key driving factors in DR research, current barriers, and challenges especially in industrial demand response application. This can then act as a guide based on the existing knowledge reported in the current literature.
This paper aims to provide a systematic review of progress in the demand response domain in general and its industrial applications. The overall goal of the review is as follows.
• First, we aim to provide a comprehensive review of demand response and the application demand response in industries. Therefore, we provide a broader perspective of the domain’s evolution and potential future research paths with specific industrial applications.
• Second, this review includes a systematic discussion of the barriers and challenges of demand response in industrial applications
• Third, this review also includes a systematic discussion of the initiatives required for widespread demand response programs in industries.
Related Reviews
There are numerous review papers published to date that covered demand response research, and it’s broader perspective. Most of the review papers mainly focused on the residential and commercial sector, and few articles are available to date that incorporate the industrial aspects of the demand response. Table 1 illustrates a summary of the top review papers that are reported.
The review papers published previously that addressed the evolution of demand response in smart grid mainly focused on smart grid technologies that helped implement demand response programs. The reviews also covered the modeling approaches and optimization framework for demand response, the pricing mechanism, market structure, and policy and regulatory requirements for implementing demand response programs.
In Samad and Kiliccote (2012), smart grid technologies such as automation systems for energy efficiency, direct load control, and automated demand response have been discussed using a real case study from Aluminium, food, Cement, and Refrigeration facilities. The study found that even though smart grid technologies are widely available in industries, more research is still required to fully integrate them into the industrial system. Developing technologies such as smart meters, energy controllers, and communication systems have been discussed to illustrate the demand response potentials and benefits in a smart grid ecosystem (Siano, 2014). The study was carried out by analyzing the industrial demand response projects and related research from the United States. Further research requirements have been suggested in measurement technologies, optimization and control systems, and communication technologies to facilitate the widespread utilization of demand response programs. Shafie-Khah et al. (2019) reported more recent advances in technologies, especially in the industrial sector for DR, explaining the potential of combined heat and power systems for DR application. Newer technologies and appliances such as energy storage (Batteries, Thermal storage) and electric vehicles can deem potential for DR application focused in industries.
Mathematical models and optimization approaches in DR have been reported in Deng et al. (2015), and different DR schemes have been classified in Vardakas et al. (2015). The deployment of a successful DR program relies mostly on a suitable DR scheme that matches the stakeholder’s expectation, accurate mathematical modeling of DR participating assets to get a realistic evaluation of revenue, qualifying DR participation requirements, and secure communication infrastructure to mitigate the privacy concerns. Thus further study on how these factors impact the current deployment of DR, especially in industries, is critical.
This paper aims to fill the gaps in previously published literature by formulating four research questions that examine recent research directions, current industrial demand response application status and identified barriers in demand response programs. This paper provided an in-depth review of recent progress in the demand program by synthesizing answers to four research questions.
Methodology
This review has been carried out to provide an in-depth analysis of demand response applications in the smart grid. Additionally, it also covered the current state of demand response applications in industries. Finally, the study further investigated the barriers and challenges in widespread adoption in demand response programs, especially in industries.
The review is based on a systematic process that was formulated by specific research questions. Search terms were developed in relation to the research question, and a primary search has been conducted in digital databases. Relevant paper metadata has been retrieved and stored for the next phase of the review process. A set of inclusion and exclusion criteria has been applied to screen the papers further. The final literature set that remained from screening was used to perform a bibliometric analysis for the first research question. Bibliometrix- an R-based tool has been used to perform the bibliometric analysis. To investigate the current research trend and identify the research themes in the demand response domain, a Multiple Correspondence Analysis (MCA) has been performed to cluster the literature’s keywords. MCA corresponds to each keyword frequently occurring in a bibliometric collection into points in a two-dimensional map where a group of points representing each keyword forms clusters expressed as common research themes. The secondary screening was done to find relevant papers for the rest of the research questions. The remainder of this section describes these steps in detail.
Research Questions
The research questions have been formulated to guide the review process into a specific scope. Each of the research questions is related to a different aspect of the topic and gives a broad overview.
• RQ1: “What are the current research trends in the demand response domain?”
The purpose of the first question is to give an overview of the research that has been undertaken in the demand response domain. It provides the emerging research themes in this domain and the research questions that are being addressed. A bibliometric analysis of keywords that are found in the metadata retrieved from the initial query identified six emerging research themes in this domain. It helps to quickly categorize the literature based on research themes and recognize the field's key contributions.
• RQ2: “What is the current state of demand response application in industries?”
The second question aims to give an overview of the current application of demand response programs in industries. Industries are one of the largest energy consumers and have good potential in terms of demand response application. Therefore, this research question intends to provide the current demand response applications in industries reported in the literature.
• RQ3: “What are the most significant barriers to adopting demand response programs in industries?”
This question aims to investigate the key barriers that slow down the adaptation of demand response in industries. The review focused on pointing out technical, social, financial, and policy barriers limiting the widespread deployment of demand response programs, especially in industries.
• RQ4: “What measures can influence the widespread adoption/deployment of demand response in industries?”
This question finds the answers to the measures that can influence participation in demand response programs by industries and other stakeholders. This takes account of previous research questions, especially where the demand response domain research is currently, industrial application status, and identified barriers limiting the adoption of demand response applications.
Table 2 describes the research questions that have been addressed throughout the paper.
Review Process
The principal repository used to identify relevant literature has been the Scopus search engine, the largest abstract and citation database of peer-reviewed literature. The query used in the search engine is the following.
“(TITLE-ABS-KEY ( ( “ Demand Response” OR “Demand Side Response”) AND “ Smart Grid”) OR TITLE-ABS-KEY (“Demand Response” AND “Industry”) OR TITLE-ABS-KEY (“Demand Response” AND ( “Policy” OR “Regulation”) ))”
The initial search yielded 5,592 records, which came down to 2,350 articles after applying the inclusion and exclusion criteria shown in Table 3. The metadata of 2,350 articles has been used to perform the bibliometric analysis, which formulated the findings of research question 1. The 80 most highly cited papers have been selected from bibliometric analysis findings, which are directly related to the emerging research themes that have been identified. An additional 66 articles resulted from the rest of the search record by Snowball approach. A secondary repository was formed with above mentioned 146 papers for full-text review. Figure 1 illustrates the methodology highlighting the details in each stage of the process.
Result
Research Question 1: “What is the Current Research Trend in the Demand Response Domain?”
By conducting a comprehensive review of the current literature and existing technologies in this area, it is possible to evaluate the topic and present specific findings. Many works of literature reported and identified the current research trend in the demand response domain. However, due to the larger number of studies published over the years, it is very time-consuming to identify the research topic’s critical aspects. Keywords are generally used to emphasize the research theme as well as the content of the publication. Hence it can be useful to derive a high-level overview of the field by using bibliometric techniques. Preliminary results of the bibliometric analysis were published in Shahnewaz Siddiquee et al. (2020). Figure 2 shows the conceptual structure from multiple correspondence analyses performed using the Bibliometrix tool. The figure's interpretation is based on the relative position of the points and their distribution along the dimensions; as words are more similar in distribution, the closer they represent in the figure (Aria and Cuccurullo 2017).
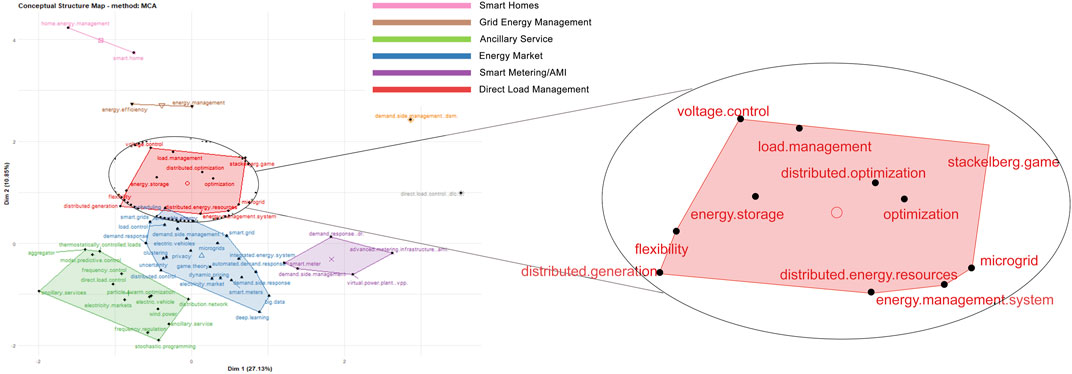
FIGURE 2. Conceptual structure of demand response research domain by highlighting the research themes found from multiple correspondence analysis (MCA) of the keywords.
Each of the color groups in Figure 2 is a cluster of keywords that represent a research theme. Table 4 provides the research themes identified in Figure 2 and summarizes the key research questions found in the literature related to each research theme. The key research questions have been synthesized by reviewing papers that represent each of the research themes.
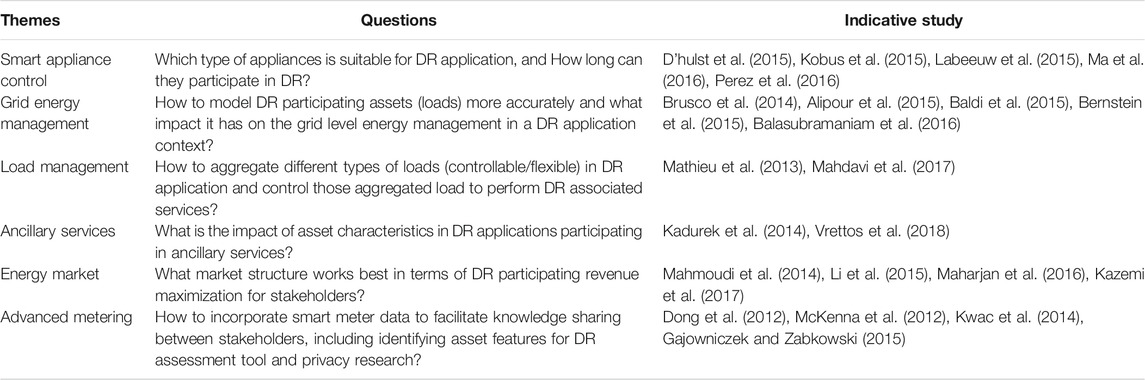
TABLE 4. Outlook on demand response research themes with key research questions and indicative studies listed`.
Among the research themes, articles have been published under Smart appliance control, where an emphasis was given to identifying suitable assets that can participate in a demand response program. Different optimization frameworks that considered the key optimization parameters such as cost, energy efficiency, user comfort have also been addressed (Pipattanasomporn et al., 2014; D’hulst et al., 2015; Kobus et al., 2015; Labeeuw et al., 2015; Ma et al., 2016; Perez et al., 2016). Pricing schemes remain a crucial challenge to identify the user’s willingness to participate in DR programs. Alternatively, the estimation of uncertainties in electrical load estimation and the frameworks to include the uncertainty measurement are getting significant attention (Yu et al., 2013; Pipattanasomporn et al., 2014).
Different DR control frameworks on grid-level energy management with distributed energy resources have been investigated to assess the impact of maximizing DER integration to the grid. Demand response facilitates DER integration by incorporating different resources (thermal loads, EVs, Energy Storage) and utilizing their flexible load changing capacity (Huang and Li 2013; Wang et al., 2013; Brusco et al., 2014; Bernstein et al., 2015; Alipour et al., 2015; Baldi et al., 2015; Aghaei et al., 2016; Balasubramaniam et al., 2016; Liu et al., 2017). However, accurate mathematical modeling of these resources is essential to fully harness their wability and integrate them into a DR framework.
The increasing number of thermal loads and EVs bring massive challenges to the traditional grid. However, some loads such as EVs can also inject the electrical power back to the electrical power grid. Therefore, it provides an excellent opportunity to achieve active demand response and provide associated services to facilitate grid stability in the presence of intermittent resources (Mathieu et al., 2013; Mahdavi et al., 2017). But how to aggregate different types of loads to participate in the DR framework, also coordinated control of these loads remains an important research question that is being addressed under the direct load management research theme.
Several works of literature were also focused on utilizing DR for ancillary services. As intermittent resources’ penetration is increasing continuously, the grid stability issues such as frequency and voltage regulations are getting attention under the DR paradigm. The effect of system parameters due to external disturbances, such as significant generation drop or large load drops, is studied. The impact of the load characteristics on system parameters has also been researched extensively in recent days (Kadurek et al., 2014; Vrettos and Andersson 2016).
There have been some extensive studies on the market behavior of demand response resources. Different market structures and strategies remain the primary focus of previously published studies. The lack of a market mechanism in the current market structure (Day-ahead/Real-Time) remains a great barrier for demand response in most countries (Li et al., 2014; Mahmoudi et al., 2014; Li et al., 2015; Maharjan et al., 2016). Without an accurate market mechanism and demand response model, estimating the demand response market’s real impact is hardly possible. Hence there will be a significant change in the consumers’ financial outcome, which has been estimated from the existing model (Nguyen et al., 2013; Kazemi et al., 2017).
The penetration of advanced metering infrastructure enables consumers to participate more in DR programs. Since the introduction of market deregulation and market-driven pricing, utilities have been searching to equalize consumption with generation. Smart meters provide site-specific information, which helps to make decisions in response to changing prices for electricity according to the day and season. Hence feature analysis of smart meter data has been getting significant research attention (Dong et al., 2012; Kwac et al., 2014;Gajowniczek and Zabkowski 2015; Haben et al., 2016). Alternatively, intelligent technologies such as Non-intrusive load monitoring (NILM) are getting popular for device-level detection. As more appliances are now becoming intelligent, i.e., IoT enabled (McKenna et al., 2012; Wang et al., 2016), it opens up a new dimension where research has been done to identify an individual device status to facilitate cost-effective scheduling under the DR program.
Research Question 2: “What is the Current State of Demand Response Application in Industries?”
This section aims to briefly introduce and then classify the type of industries that implemented DR programs. A summary of this classification is presented in Table 5. It illustrates the dominant industrial sector that has the potential to apply DR.
Aluminum Industry
Aluminum industries are considered to have good potential for DR applications in the previously published literature (Shoreh et al., 2016). The Aluminum production process involves Aluminum smelting, where chemical reduction of aluminum oxide happens to produce aluminum by an electrolytic method. The process requires a considerable amount of electricity which typically ranged hundreds of megawatt. Smelting absorbs about 46% of the overall resources consumed by aluminum production. In aluminum’s typical smelter, 30–40% of the overall manufacturing cost is devoted to electricity cost (Zhang and Hug 2015). Aluminum smelting typically occurs in smelting “Pots,” and a smelting process usually consists of multiple potlines. In an ideal process scenario, the potlines operate uninterrupted, and any interruption can hamper the final product. However, the potlines are used at a very high temperature to keep the produced alumina in a molten state. This creates an opportunity for existing smelting plants to provide demand response in two ways. Firstly small reduction of load can be achieved by controlling the input voltage of the potline. In this case, the deduction can be achieved for a short period of time, and the process needs to return to normal operating conditions to maintain the process integrity. Secondly, demand response can be achieved by turning off the entire potline to reduce electricity consumption in a large scale. Due to the specific plant limitation, the interruption period can be from minutes to 2 h. To enable a longer interruption duration, a factory with multiple potlines can rotate the shutdown procedure between multiple potlines. Generally, this is achieved by using the opening of circuit breakers. Aluminum electrolysis is categorized as an effective system for load shedding rather than load shifting in DR programs, based on the reasons mentioned above because the usage rate for this procedure is shown to be 95–98% annually.
Steel Industry
Steel processing industries are regarded as one of the most complex industries to schedule, contrary to the aluminum industry (Zhang et al., 2017). It is considered a large-scale, multi-level, and multi-product industry consisting of similar instruments, dynamic processes, and energy constraints. In the steel manufacturing process, heat is produced by an electric arc furnace or by induction that begins to melt scrap metal in the furnace. In a specific Demand Response scenario involving the steel manufacturing process, the process will stop immediately if the process interruption due to participation exceeds half an hour. The melting process needs to resume again as the scrap metal starts to cool down. If the process is delayed for more than half an hour, extra costs may result (Hubert and Grijalva 2012). As reported, up to 2011, approximately 50% of German steel mills have pre-qualified their furnaces as a positive capability in the tertiary reserve market as part of the DR participation program (Valdes et al., 2019). Compared to reserve energy costs, power prices tend to be fair and smaller, and this is dependent on the high valuation of missed loads in this process.
Cement Industry
The cement industry is one of the energy-intensive industry which has a good potential for demand response program. Cement industries consume over 350 trillion Btu of gasoline and 10 billion kWh of electricity annually in the United States only. As it is an energy-intensive industry, demand management is a standard practice in the cement industry. Many literatures also reported research on different factors that impacts energy cost and energy consumption in the cement industry. For example, study on energy prices in California (1977) analyzed the impact of multi-level tariffs on cement plants (Rose 1997). One example of the utilization of multi-level tariffs in Cement factory is the reduction of daily peak demand nearly half by two factories in England and France for higher price hours. In Mexico and Latin America also, cement plants reduced their consumption during peak hours. The presence of cement plants in DR programs is mostly with DR programs focused on price, especially real-time pricing (RTP) (Swanepoel et al., 2014). Based on this, the cement plants interested in the DR programs consider dynamic prices. The DR model optimizes the cement output that ensures actual throughput. When the actual throughput is guaranteed, the electricity cost is at the lowest amount, even though there are no improvements in electricity price. It should be noted that cement plant’s loads could be categorized into three types of load, namely, non-shift, semi-shift, and full-shift. In the literature, cement grinding and packaging are considered full-shift processes. Kiln, preheating, and coal grinding are non-shift processes, while crushing can be regarded as the semi-shift process.
Food Industry
The food industry is another sector with enormous potential and application of DR due to the nature of the process involved. The food industry, especially those that have refrigeration and cooling requirements in the process, has adequate returns for DR deployment. Thermal inertia is used as a buffer in these processes for moving and controlling cooling power consumption. Since industrial refrigeration and cooling warehouses generally have centralized control systems and energy management steps, they have Auto-DR capacity. Open ADR (Open Automatic Demand Response) protocol is used in Auto-DR to continuously transmit signals over the internet to allow facilities to automate their DR (Alcázar-Ortega et al., 2012). Refrigerated warehouses are good candidates for DR deployment. They are major energy users, especially during peak periods; these loads account for almost 16% of the food industry’s total consumption, based on a study (Wohlfarth et al., 2020). Moreover, because these systems are not vulnerable to short-term load curtailment, DR behaviors do not impact them because of the load’s intrinsic thermal inertia. Processes in this field, however, are basic and easy to understand. Any refrigerated warehouse applications include storage in cold and frozen areas used to freeze goods and construction services.
Other Industries
Both the pulp and paper mills are part of the industrial sector’s larger electricity consumer. For example, an enormous amount of electricity is used to separate Lignin fibers and make them smooth and suitable for the papermaking process. It is possible to adopt a system that responds to dynamic pricing in the pulp production process if the paper mill’s thermos mechanical pulping lines have a higher capacity than the paper machines. This also enables the industry to participate in priced-based DR applications and subsequently provide an opportunity for minimizing its total electricity cost (Hadera, 2015). Table 6 highlights some industrial application instances reported in the literatures.
Overall, some industries, such as aluminum, steel, cement and refrigeration, have demonstrated a high DR involvement capacity relative to other sectors. In terms of the relative similarities of the processes and their technologies’ versatility, refrigeration systems are desirable for DR deployment. The aluminum sector still has a reasonably straightforward manufacturing mechanism and hence encourages the introduction of DR programs. Cement and steel manufacturing have more complex and intertwined processes than the aluminum industry, but they are also suitable for DR because of their massive energy consumption. On this basis, the potential for DR implementation in these industries is reasonably valuable. The capacity of cement plants for DR depends, to some degree, on the amount of current material stock at the facility. Plants with less storage capacity do not have as much DR flexibility.
Research Question 3: “What are the Most Significant Barriers to Adopting Demand Response Programs in Industries?”
The barriers of industrial and commercial DR can be divided into several aspects. In the last decade, the conventional power system is facing major transformation, moving into a competitive market structure that enables smart grid services such as demand response. Despite this transformation, DR execution, especially in industries, faced some significant barriers such as lack of access to information, lack of proper market mechanism, technical constraints along with lack of policy and regulations (Good and Mancarella 2019). In terms of access to information, a potential DR Service provider may not have possible flexibility information shared among the service’s potential buyers (Brown 2001). One of the primary reasons is the cost of collecting and analyzing such information and the time required to perform such analysis. A real scenario of the impact of information sharing could be the forecast of electrical demand. If a consumer cannot forecast its electrical load, it creates uncertainty in providing necessary DR Service upon request. It could significantly impact the reliability of the consumer and create a potential risk for the DR Service provider.
The lack of appropriate market mechanisms in the current market structure is another barrier for demand response. Demand response is currently configured for emergency contingency service and ancillary services with participation in the day-ahead market. This market’s nature requires demand response to be planned ahead of time or with substantial advanced notice in an emergency scenario. Such requirements prevent the consumers from withdrawing from participation if the inconvenience becomes too great (Xenias et al., 2015).
The success of the entire demand response paradigm depends on the willingness and mindset of the consumers. There are many associated benefits of DR, but if the end-user is not satisfied with the benefit to inconvenience factor, it may disengage and possibly encourage them to withdraw from a DR participation. Currently, most end-users are on retail tariffs, which may not prove beneficial in demand response participation. However, the implementation of a time-based tariff showed a significant impact on demand reduction in peak hours. The time-based tariff allows the consumer to harness the full potential of demand response participation by enabling demand reduction in higher price periods (Nolan and O’Malley 2015).
At present, the technical requirements such as advanced monitoring and communication technologies required for widespread demand response are available. But lack of a unified standard measurement and verification procedure would harm consumer participation. To date, there are different measurement and verification procedures are in practice across utilities and service entities. Therefore, it potentially reduces the accurate assessment of DR benefits due to a lack of standard procedure (Oconnell et al., 2014).
Government decisions can sometimes cause a barrier in DR implementation. The effects of these policies like taxation of DR-enabling technology investment can create a barrier to the efficient DR operation (Vallés et al., 2016). All electrical system’s regulations are based on the assumption that the power flow is from large-scale generators to distributed users. Hence, there is no adequate regulation for demand-side participants in terms of characteristics related to DR. Additionally, rules limitations for locational price differentiation in markets will prevent all customers from receiving the right value of DR. The regulations and policies in various countries are different (Xenias et al., 2015). Thus, each country should have its regulation for DR. For example, the United States market model has DR aggregators who sell their DR capacity to the wholesale electricity market. However, in China, due to the lack of wholesale electricity market, grid companies make a contract with consumers, and there is no incentive for them to contract with DR aggregators because of lack of policy (Yang 2017). Table 7 illustrates the energy policies related to DR in United States, EU and China in recent years.
There is also a lack of data security at the policy level that discourages consumers, especially from industries to participate in demand response programs. A clear definition of what data needs to be exchanged between BRP, aggregator, and TSOs to ensure all can fulfill their obligations while not sharing commercially sensitive information is essential for encouraging the industrial stakeholder to participate in demand response programs. Table 8 shows the key issues that limit the participation in demand response programs, especially in industries.
Research Question 4: “What Measures Can Influence the Widespread Adoption/Deployment of Demand Response in Industries?”
Several measures can be suggested in the literature that can influence demand response programs in industries. Specially overcoming the factors that have been addressed in research question 3 is essential for the successful implementation of demand response. Some measures could be taken immediately, while others will take a longer time, requiring a change in current technology status, policy reforms, and financial reforms.
• Accurate knowledge about flexibility present in any industrial system is essential for both the consumer and potential buyer (aggregator or DSO or TSO) to understand how much service a consumer can offer. However, the lack of a standard assessment method and time-consuming, complex assessment process discourages the consumer from participating in demand response programs willingly. A standard method for demand flexibility assessment that is simple and faster is vital for ensuring participation in demand response programs. Recent advances in big data and data analytics can help to overcome these issues.
• Subsequently, the consumers needed to be assured of demand response’s impact on primary business or process. For industries, the effect of participation in demand response on the company or process is crucial due to its direct relationship with the revenue. Proper initiative needed to be taken to make the consumers well informed about the potential impact of demand response revenue on its annual energy expenditure and its risks.
• In terms of policy measures, DR programs need to be integrated into the overall energy policy and energy initiative. This will help DR to receive necessary policy and resource support to close the gap in policy initiatives and change in market dynamics and technology status.
• The proper financial mechanisms need to be introduced to allow DR participating consumers to recover their investment cost in participating in DR. This could be done by introducing incentives for participation and subsidies in a technology upgrade.
Conclusion
In this paper, a comprehensive review of demand response has been carried out to provide an in-depth view of the recent research trends by identifying key research questions and contributions. Additionally, the type of demand response applications in industries have been categorized to understand the current potential industrial sectors that can contribute to demand response programs. A further study was carried out to identify the barriers limiting demand response applications, especially in industries. Combining the current research direction in the demand response domain, potential industrial application of demand response, and the barriers limiting the potential, some measures have been suggested to help the widespread adaptation of the demand response program.
The review shows significant research on emerging topics in demand response such as smart appliance control, grid energy management, load management, ancillary services, energy market, and advanced metering. In addition, the study found large industries such as aluminum, steel, cement, food and refrigeration etc., to have the potential for DR application in their processes and applications. However, there are significant barriers that have been identified in the review process. Lack of access to information between the DR stakeholders, lack of proper financial mechanism, lack of adequate DR directed energy policy in different countries, and technical constraints have been identified as the critical barriers of DR deployment. The review suggested using big data and data analytics to develop standard flexibility assessment methods to tackle the information sharing barrier in demand response. It also encourages to bring proper policy initiative and the financial mechanism that can increase participation in demand response programs by industries.
Understanding the recent advances in research is crucial to comprehend technology development, suggesting a clear and concrete pathway for overcoming the barriers. The findings of this systematic approach to analyze the progress and evolution of the demand response domain will guide industry and the community by providing a clear and concise view of demand response progress and its industrial application hence bridging the information gap. From the findings, it is evident that much intellectual progress has been made over the years in the research community, addressing some key questions, which can enormously benefit in overcoming technical and information barriers of demand response considering the industrial application. However, it is still essential to bring policy and regulatory reforms that can encourage industrial consumers to participate in demand response programs.
Author Contribution
SMSS conceptualized and performed the review process. SMSS wrote the manuscript with contribution from BH, AB, KB, and DO'S. All the authors contributed to the manuscript revision, read and approved the submitted version.
Funding
This research was part-funded by Science Foundation Ireland (SFI) through MaREI, the SFI Research Centre for Energy, Climate, and Marine, and specially the Centre for Doctoral Training in Energy Resilience and the Built Environment (ERBE) (grant no: 12/RC/2302_P2), with supporting funding obtained from United Kingdom Engineering and Physical Sciences Research Council (EPSRC) (grant EP/S021671/1) and FlexTECC: Flexible Timing of Energy Consumption in Communities Innovation Fellowship (grant EP/S001670/1).
Conflict of Interest
The authors declare that the research was conducted in the absence of any commercial or financial relationships that could be construed as a potential conflict of interest.
References
Aghaei, J., and Alizadeh, M.-I. (2013). Demand Response in Smart Electricity Grids Equipped with Renewable Energy Sources: A Review. Renew. Sustainable Energ. Rev. 18, 64–72. doi:10.1016/j.rser.2012.09.019
Aghaei, J., Barani, M., Shafie-Khah, M., Sanchez de la Nieta, A. A., Catalao, J. P. S., Joao, P., et al. (2016). Risk-Constrained Offering Strategy for Aggregated Hybrid Power Plant Including Wind Power Producer and Demand Response Provider. IEEE Trans. Sustain. Energ. 7 (2), 513–525. doi:10.1109/TSTE.2015.2500539
Alcázar-Ortega, M., Álvarez-Bel, C., Escrivá-Escrivá, G., and Domijan, A. (2012). Evaluation and Assessment of Demand Response Potential Applied to the Meat Industry. Appl. Energ. 92, 84–91. doi:10.1016/j.apenergy.2011.10.040
Alipour, M., Mohammadi-Ivatloo, B., and Zare, K. (2015). Stochastic Scheduling of Renewable and CHP-Based Microgrids. IEEE Trans. Ind. Inf. 11 (5), 1049–1058. doi:10.1109/TII.2015.2462296
Antonopoulos, I., Robu, V., Couraud, B., Kirli, D., Norbu, S., Kiprakis, A., et al. (2020). Artificial Intelligence and Machine Learning Approaches to Energy Demand-Side Response: A Systematic Review. Renew. Sustainable Energ. Rev. 130 (June), 109899. doi:10.1016/j.rser.2020.109899
Aria, M., and Cuccurullo, C. (2017). Bibliometrix : An R-Tool for Comprehensive Science Mapping Analysis. J. Informetrics. 11 (4), 959–975. doi:10.1016/j.joi.2017.08.007
Aslam, W., Soban, M., Akhtar, F., and Zaffar, N. A. (2015). Smart Meters for Industrial Energy Conservation and Efficiency Optimization in Pakistan: Scope, Technology and Applications. Renew. Sustainable Energ. Rev. 44, 933–943. doi:10.1016/j.rser.2015.01.004
Balasubramaniam, K., Saraf, P., Hadidi, R., and Makram, E. B. (2016). Energy Management System for Enhanced Resiliency of Microgrids during Islanded Operation. Electric Power Syst. Res. 137, 133–141. doi:10.1016/j.epsr.2016.04.006
Baldi, S., Karagevrekis, A., Michailidis, I. T., and Kosmatopoulos, E. B. (2015). Joint Energy Demand and Thermal Comfort Optimization in Photovoltaic-Equipped Interconnected Microgrids. Energ. Convers. Management. 101, 352–363. doi:10.1016/j.enconman.2015.05.049
Behrangrad, M. (2015). A Review of Demand Side Management Business Models in the Electricity Market. Renew. Sustainable Energ. Rev. 47, 270–283. doi:10.1016/j.rser.2015.03.033
Bernstein, A., Reyes-Chamorro, L., Le Boudec, J.-Y., and Paolone, M. (2015). A Composable Method for Real-Time Control of Active Distribution Networks with Explicit Power Setpoints. Part I: Framework. Electric Power Syst. Res. 125, 254–264. doi:10.1016/j.epsr.2015.03.023
Brown, M. A. (2001). Market Failures and Barriers as a Basis for Clean Energy Policies. Energy Policy. 29, 1197-1207. doi:10.1016/S0301-4215(01)00067-2
Brusco, G., Burgio, A., Menniti, D., Pinnarelli, A., and Sorrentino, N. (2014). Energy Management System for an Energy District with Demand Response Availability. IEEE Trans. Smart Grid. 5 (5), 2385–2393. doi:10.1109/TSG.2014.2318894
Castro, P. M., Giancarlo Dalle, A., Dalle Ave, G., Engell, S., Grossmann, I. E., and Harjunkoski, I. (2020). Industrial Demand Side Management of a Steel Plant Considering Alternative Power Modes and Electrode Replacement. Ind. Eng. Chem. Res. 59, 13642–13656. doi:10.1021/acs.iecr.0c01714
Castro, P. M., Sun, L., and Harjunkoski, I. (2013). Resource-Task Network Formulations for Industrial Demand Side Management of a Steel Plant. Ind. Eng. Chem. Res. 52, 13046–13058. doi:10.1021/ie401044q
Deng, R., Yang, Z., Chow, M.-Y., and Chen, J. (2015). A Survey on Demand Response in Smart Grids: Mathematical Models and Approaches. IEEE Trans. Ind. Inf. 11 (3), 570–582. doi:10.1109/TII.2015.2414719
D’hulst, R., Labeeuw, W., Beusen, B., Claessens, S., Deconinck, G., and Vanthournout, K. (2015). Demand Response Flexibility and Flexibility Potential of Residential Smart Appliances: Experiences from Large Pilot Test in Belgium. Appl. Energ. 155, 79–90. doi:10.1016/j.apenergy.2015.05.101
Dong, J., Xue, G., and Li, R. (2016). Demand Response in China: Regulations, Pilot Projects and Recommendations - A Review. Renew. Sustainable Energ. Rev. 59, 13–27. doi:10.1016/j.rser.2015.12.130
Dong, M., Meira, P. C. M., Xu, W., and Freitas, W. (2012). An Event Window Based Load Monitoring Technique for Smart Meters. IEEE Trans. Smart Grid. 3 (2), 787–796. doi:10.1109/TSG.2012.2185522
Eftekharnejad, S., Vittal, V., Gerald Thomas, H., Keel, B., and Loehr, J. (2013). Impact of Increased Penetration of Photovoltaic Generation on Power Systems. IEEE Trans. Power Syst. 28 (2), 893–901. doi:10.1109/TPWRS.2012.2216294
Gajowniczek, K., and Ząbkowski, T. (2015). Data Mining Techniques for Detecting Household Characteristics Based on Smart Meter Data. Energies. 8 (7), 7407–7427. doi:10.3390/en8077407
Goli, S., McKane, A., and Olsen, D. (2012). Demand Response Opportunities in Industrial Refrigerated Warehouses in CaliforniaACEEE Summer Study on Energy Efficiency in Industry Report number : LBNL-4837E, Niagara Falls, NY: 2011 ACEEE Summer Study on Energy Efficiency in Industry.
Good, N., and Mancarella, P. (2019). Flexibility in Multi-Energy Communities with Electrical and Thermal Storage: A Stochastic, Robust Approach for Multi-Service Demand Response. IEEE Trans. Smart Grid. 10 (1), 503–513. doi:10.1109/TSG.2017.2745559
Grein, A., and Pehnt, M. (2011). Load Management for Refrigeration Systems: Potentials and Barriers. Energy Policy. 39, 5598–5608. doi:10.1016/j.enpol.2011.04.040
Haben, S., Singleton, C., and Grindrod, P. (2016). Analysis and Clustering of Residential Customers Energy Behavioral Demand Using Smart Meter Data. IEEE Trans. Smart Grid. 7 (1), 136–144. doi:10.1109/TSG.2015.2409786
Hadera, H., Wide, P., Harjunkoski, I., Mäntysaari, J., Ekström, J., Sand, G., et al. (2015). A Mean Value Cross Decomposition Strategy for Demand-Side Management of a Pulping Process. Computer Aided Chem. Eng. 37, 1931–1936. doi:10.1016/B978-0-444-63576-1.50016-9
Handa, T., Oda, A., Tachikawa, T., Ichimura, J., Watanabe, Y., and Nishi, H. (2008). KNIVES: A Distributed Demand Side Management System - Integration with ZigBee Wireless Sensor Network and Application -. IEEE Int. Conf. Ind. Inform. (Indin). doi:10.1109/INDIN.2008.4618117
Huang, H., and Li, F. (2013). Sensitivity Analysis of Load-Damping Characteristic in Power System Frequency Regulation. IEEE Trans. Power Syst. 28 (2), 1324–1335. doi:10.1109/TPWRS.2012.2209901
Huang, W., Zhang, N., Kang, C., Li, M., and Huo, M. (2019). From Demand Response to Integrated Demand Response: Review and Prospect of Research and Application. Prot. Control. Mod. Power Syst. 4 (1). doi:10.1186/s41601-019-0126-4
Hubert, T., and Grijalva, S. (2012). Modeling for Residential Electricity Optimization in Dynamic Pricing Environments. IEEE Trans. Smart Grid. 3 (4), 2224–2231. doi:10.1109/TSG.2012.2220385
Kadurek, P., Cobben, J. F. G., Kling, W. L., and Ribeiro, P. F. (2014). Aiding Power System Support by Means of Voltage Control with Intelligent Distribution Substation. IEEE Trans. Smart Grid. 5 (1), 84–91. doi:10.1109/TSG.2013.2289372
Kazemi, M., Zareipour, H., Ehsan, M., and Rosehart, W. D. (2017). A Robust Linear Approach for Offering Strategy of a Hybrid Electric Energy Company. IEEE Trans. Power Syst. 32 (3), 1949–1959. doi:10.1109/TPWRS.2016.2597338
Kobus, C. B. A., Klaassen, E. A. M., Mugge, R., and Schoormans, J. P. L. (2015). A Real-Life Assessment on the Effect of Smart Appliances for Shifting Households' Electricity Demand. Appl. Energ. 147, 335–343. doi:10.1016/j.apenergy.2015.01.073
Kwac, J., Flora, J., and Rajagopal, R. (2014). Household Energy Consumption Segmentation Using Hourly Data. IEEE Trans. Smart Grid. 5 (1), 420–430. doi:10.1109/TSG.2013.2278477
Labeeuw, W., Stragier, J., and Deconinck, G. (2015). Potential of Active Demand Reduction with Residential Wet Appliances: A Case Study for Belgium. IEEE Trans. Smart Grid. 6 (1), 315–323. doi:10.1109/TSG.2014.2357343
Li, H., Lin, X., Yang, H., Liang, X., Lu, R., and Shen, X. (2014). EPPDR: An Efficient Privacy-Preserving Demand Response Scheme with Adaptive Key Evolution in Smart Grid. IEEE Trans. Parallel Distrib. Syst. 25 (8), 2053–2064. doi:10.1109/TPDS.2013.124
Li, N., Chen, L., and Dahleh, M. A. (2015). Demand Response Using Linear Supply Function Bidding. IEEE Trans. Smart Grid. 6 (4), 1827–1838. doi:10.1109/TSG.2015.2410131
Liu, J., Chen, H., Zhang, W., Yurkovich, B., and Rizzoni, G. (2017). Energy Management Problems under Uncertainties for Grid-Connected Microgrids: A Chance Constrained Programming Approach. IEEE Trans. Smart Grid. 8 (6), 2585–2596. doi:10.1109/TSG.2016.2531004
Ma, K., Yao, T., Yang, J., and Guan, X. (2016). Residential Power Scheduling for Demand Response in Smart Grid. Int. J. Electr. Power Energ. Syst. 78, 320–325. doi:10.1016/j.ijepes.2015.11.099
Maharjan, S., Zhu, Q., Zhang, Y., Gjessing, S., and Basar, T. (2016). Demand Response Management in the Smart Grid in a Large Population Regime. IEEE Trans. Smart Grid. 7 (1), 189–199. doi:10.1109/TSG.2015.2431324
Mahdavi, N., Braslavsky, J. H., Seron, M. M., and West, S. R. (2017). Model Predictive Control of Distributed Air-Conditioning Loads to Compensate Fluctuations in Solar Power. IEEE Trans. Smart Grid 8 (6), 3055–3065. doi:10.1109/TSG.2017.2717447
Mahmoudi, N., Eghbal, M., and Saha, T. K. (2014). Employing Demand Response in Energy Procurement Plans of Electricity Retailers. Int. J. Electr. Power Energ. Syst. 63, 455–460. doi:10.1016/j.ijepes.2014.06.018
Mathieu, J. L., Koch, S., and Callaway, D. S. (2013). State Estimation and Control of Electric Loads to Manage Real-Time Energy Imbalance. IEEE Trans. Power Syst. 28 (1), 430–440. doi:10.1109/TPWRS.2012.2204074
McKenna, E., Richardson, I., and Thomson, M. (2012). Smart Meter Data: Balancing Consumer Privacy Concerns with Legitimate Applications. Energy Policy. 41, 807–814. doi:10.1016/j.enpol.2011.11.049
Nguyen, D. T., Negnevitsky, M., and De Groot, M. (2013). Market-Based Demand Response Scheduling in a Deregulated Environment. IEEE Trans. Smart Grid. 4 (4), 1948–1956. doi:10.1109/TSG.2013.2258410
Nolan, S., and O’Malley, M. (2015). Challenges and Barriers to Demand Response Deployment and Evaluation. Appl. Energ. 152, 1–10. doi:10.1016/j.apenergy.2015.04.083
Oconnell, N., Pierre, P., Madsen, H, and Omalley, M. (2014). Benefits and Challenges of Electrical Demand Response: A Critical Review. Renew. Sustainable Energ. Rev. 39, 686–699. doi:10.1016/j.rser.2014.07.098
Palensky, P., and Dietrich, D. (2011). Demand Side Management: Demand Response, Intelligent Energy Systems, and Smart Loads. IEEE Trans. Ind. Inf. 7 (3), 381–388. doi:10.1109/TII.2011.2158841
Paulus, M., and Borggrefe, F. (2011). The Potential of Demand-Side Management in Energy-Intensive Industries for Electricity Markets in Germany. Appl. Energ. 88 (2), 432–441. doi:10.1016/j.apenergy.2010.03.017
Perez, K. X., Baldea, M., and Edgar., T. F. (2016). Integrated HVAC Management and Optimal Scheduling of Smart Appliances for Community Peak Load Reduction. Energy and Buildings. 123, 34–40. doi:10.1016/j.enbuild.2016.04.003
Pipattanasomporn, M., Kuzlu, M., Rahman, S., and Teklu, Y. (2014). Load Profiles of Selected Major Household Appliances and Their Demand Response Opportunities. IEEE Trans. Smart Grid. 5 (2), 742–750. doi:10.1109/TSG.2013.2268664
Rose, M. P. (1997). Power Costs Reduction Measures at Lafarge Whitehall Plant. IEEE Cement Industry Technical Conference. doi:10.1109/citcon.1997.601995(Paper)
Samad, T., and Kiliccote, S. (2012). Smart Grid Technologies and Applications for the Industrial Sector. Comput. Chem. Eng. 47, 76–84. doi:10.1016/j.compchemeng.2012.07.006
Shafie-Khah, M., Pierluigi, S., Siano, P., Aghaei, J., Masoum, M. A. S., Li, F., et al. (2019). Comprehensive Review of the Recent Advances in Industrial and Commercial DR. IEEE Trans. Ind. Inf. 15 (7), 3757–3771. doi:10.1109/TII.2019.2909276
Shahnewaz Siddiquee, S. M., HowardO’Sullivan, B., O'Sullivan, D. T. J., and Bruton, K. (2020). “Demand Response in Smart Grid - A Systematic Mapping Study,” in 2020 2nd International Conference on Smart Power and Internet Energy Systems (Bangkok, Thailand: SPIES), 2020, 327–332. doi:10.1109/SPIES48661.2020.9243107
Shen, B., Ghatikar, G., Lei, Z., Li, J., Wikler, G., and Martin, P. (2014). The Role of Regulatory Reforms, Market Changes, and Technology Development to Make Demand Response a Viable Resource in Meeting Energy Challenges. Appl. Energ. 130 (October), 814–823. doi:10.1016/j.apenergy.2013.12.069
Shoreh, M. H., Siano, P., Shafie-khah, M., Loia, V., Catalão, J. P. S., and Catalão, S. (2016). A Survey of Industrial Applications of Demand Response. Electric Power Syst. Res. 141, 31–49. doi:10.1016/j.epsr.2016.07.008
Siano, P. (2014). Demand Response and Smart Grids-A Survey. Renew. Sustainable Energ. Rev. 30, 461–478. doi:10.1016/j.rser.2013.10.022
Starke, M. R., Kirby, B. J., Kueck, J. D., Todd, D., Caulfield, M., and Helms, B. (2009). Providing Reliability Services through Demand Response: A Preliminary Evaluation of the Demand Response Capabilities of Alcoa Inc. United States. doi:10.2172/948544
Sun, L., Harjunkoski, I., and Castro, P. (2013). Resource-Task Network Based Approach for Industrial Demand Side Management of Steel Production. Computer Aided Chem. Eng., 259–264. doi:10.1016/B978-0-444-63234-0.50044-0
Swanepoel, J. A., Mathews, E. H., Vosloo, J., and Liebenberg, L. (2014). Integrated Energy Optimisation for the Cement Industry: A Case Study Perspective. Energ. Convers. Management. 78 (February), 765–775. doi:10.1016/j.enconman.2013.11.033
Valdes, J., Poque González, A. B., Ramirez Camargo, L., Valin Fenández, M., Masip Macia, Y., and Dorner, W. (2019). Industry, Flexibility, and Demand Response: Applying German Energy Transition Lessons in Chile. Energ. Res. Soc. Sci. 54, 12–25. doi:10.1016/j.erss.2019.03.003
Vallés, M., Reneses, J., Cossent, R., and Frías, P. (2016). Regulatory and Market Barriers to the Realization of Demand Response in Electricity Distribution Networks: A European Perspective. Electric Power Syst. Res. 140, 689–698. doi:10.1016/j.epsr.2016.04.026
Vardakas, J. S., Zorba, N., and Verikoukis, C. V. (2015). A Survey on Demand Response Programs in Smart Grids: Pricing Methods and Optimization Algorithms. IEEE Commun. Surv. Tutorials. 17 (1), 152–178. doi:10.1109/COMST.2014.2341586
Vrettos, E., and Andersson, G. (2016). Scheduling and Provision of Secondary Frequency Reserves by Aggregations of Commercial Buildings. IEEE Trans. Sustain. Energ. 7 (2), 850–864. doi:10.1109/TSTE.2015.2497407
Vrettos, E., Kara, E. C., MacDonald, J., Andersson, G., and Callaway, D. S. (2018). Experimental Demonstration of Frequency Regulation by Commercial Buildings-Part II: Results and Performance Evaluation. IEEE Trans. Smart Grid. 9 (4), 3224–3234. doi:10.1109/TSG.2016.2628893
Wang, Q., Wang, J., and Guan, Y. (2013). Stochastic Unit Commitment with Uncertain Demand Response. IEEE Trans. Power Syst. 28 (1), 562–563. doi:10.1109/TPWRS.2012.2202201
Wang, Y., Chen, Q., Kang, C., and Xia, Q. (2016). Clustering of Electricity Consumption Behavior Dynamics toward Big Data Applications. IEEE Trans. Smart Grid. 7 (5), 2437–2447. doi:10.1109/TSG.2016.2548565
Wohlfarth, K., Klobasa, M., and Gutknecht, R. (2020). Demand Response in the Service Sector - Theoretical, Technical and Practical Potentials. Appl. Energ. 258 (October 2019), 114089. doi:10.1016/j.apenergy.2019.114089
Xenias, D., Axon, C. J., Whitmarsh, L., Whitmarsh, N. B-O., Connor, P. M., Balta-Ozkan, N., et al. (2015). UK Smart Grid Development: An Expert Assessment of the Benefits, Pitfalls and Functions. Renew. Energ. 81, 89–102. doi:10.1016/j.renene.2015.03.016
Yang, C.-J. (2017). Opportunities and Barriers to Demand Response in China. Resour. Conservation Recycling 121, 51–55. doi:10.1016/j.resconrec.2015.11.015
Yu, Z., Jia, L., Murphy-Hoye, M. C., Murphy-Hoye, M. C., Pratt, A., and Tong, L. (2013). Modeling and Stochastic Control for Home Energy Management. IEEE Trans. Smart Grid. 4 (4), 2244–2255. doi:10.1109/TSG.2013.2279171
Zhang, X., and Hug, G. (2015). “Bidding Strategy in Energy and Spinning reserve Markets for Aluminum Smelters' Demand Response,” in In 2015 IEEE Power and Energy Society Innovative Smart Grid Technologies Conference (Washington, DC, United States: ISGT), 2015. doi:10.1109/ISGT.2015.7131854
Keywords: demand response, smart grid, systematic review, industrial demand response, demand response policy, demand side flexibility
Citation: Siddiquee SMS, Howard B, Bruton K, Brem A and O'Sullivan DTJ (2021) Progress in Demand Response and It’s Industrial Applications. Front. Energy Res. 9:673176. doi: 10.3389/fenrg.2021.673176
Received: 26 February 2021; Accepted: 09 June 2021;
Published: 23 June 2021.
Edited by:
Ke Meng, University of New South Wales, AustraliaReviewed by:
Narottam Das, Central Queensland University, AustraliaAlessandro Burgio, Independent Researcher, Rende, Switzerland
Copyright © 2021 Siddiquee, Howard, Bruton, Brem and O'Sullivan. This is an open-access article distributed under the terms of the Creative Commons Attribution License (CC BY). The use, distribution or reproduction in other forums is permitted, provided the original author(s) and the copyright owner(s) are credited and that the original publication in this journal is cited, in accordance with accepted academic practice. No use, distribution or reproduction is permitted which does not comply with these terms.
*Correspondence: S. M. Shahnewaz Siddiquee, 119222676@umail.ucc.ie