- 1Yangzhou Power Supply Company, Jiangsu Electric Power Company, State Grid Cooperation of China, Yangzhou, China
- 2School of Electrical Engineering, Southeast University, Nanjing, China
With the increasing popularity of renewable energy, energy storage systems (ESSs) have now been used as an essential way to reduce energy bills and mitigate the impact of the uncertainty of renewable generators on the energy network. However, the high investment cost of ESS limits its application. This article proposes the concept of shared ESS (Shared-ESS) for microgrid owner/operator and applies it to the economic optimal dispatch of a microgrid cluster. In addition to the energy storage, the microgrids can achieve the peer-to-peer (P2P) transaction among each other with the use of the Shared-ESS, which significantly improves the energy utilization efficiency. The numerical analysis shows that the Shared-ESS can significantly reduce the energy bills of microgrid owner/operator, shift the usage of energy during peak time, and facilitate the renewable energy consumption.
Introduction
To tackle the increasingly serious climate change, a number of countries have set their own targets on carbon emission reduction. Renewable energy is considered as an essential way to reduce the carbon emission, and correspondingly, the penetration of renewable energy has been increasing significantly in the past decade. The high penetration of renewable energy will bring challenges to network operator to balance the network due to their fluctuation characteristics (Borghetti et al., 2010; Denholm and Hand, 2011). Therefore, to mitigate the fluctuation and balance the network, new technologies need to be adopted urgently.
Some scholars focused on the distributed mechanism of network-connected microgrids. Zhou et al. (2020) developed a distributed secondary H∞ consensus approach for DC microgrids. Full plug-and-play operation can be realized with the proposed strategy. Zhou et al. (2020a) studied an integrated hierarchical distributed coordinated control scheme for AC–DC microgrids interconnected by interlinking converters. Yushuai et al. (2020) established a distributed energy management model, which takes into consideration the maximum profits of overall energy generation and consumption and the minimum cost of energy delivery. Li et al. (2020) proposed a double-mode energy management model for multi microgrids, which include the island mode and the network-connected mode. A novel distributed dynamic event-triggered Newton–Raphson algorithm was adopted to solve the problem. Li et al. (2019) also used an event-trigger-based distributed algorithm, which features distributed algorithm, asynchronous communication, and independent calculation to solve the established day-ahead and real-time energy management models.
Energy storage system (ESS) is also an effective way to deal with the uncertainty of renewable energy. Although the price of battery has dropped continuously, the price and lifetime of ESSs still cannot be ignored (Swierczyński et al., 2013). Thus, exploring the approach of efficiently using ESSs is necessary to accommodate the increasing renewable energy.
A number of research have been focused on the shared use of ESSs. Roberts et al. (2019) pointed out that there are clear financial benefits to the deployment of embedded networks with combined solar and shared ESS (Shared-ESS) for many sites. The addition of a Shared-ESS to an embedded network with Photovoltaic (PV) can increase self-consumption and self-sufficiency and reduce peak demand. Broering and Madlener (2017) studied the economic merit of Shared-ESS in three distinct households in Aachen, Germany, by analyzing three scenarios with different grid-use tariffs. The authors drew out that profits can indeed be made below a feed-in tariff of 10 €/kWh. Liu N. et al. (2018) proposed a concept of energy-sharing provider for PV prosumer cluster, and the neighboring PV prosumers can trade electricity with each other by direct sharing or buffered sharing using the ESS. Yan et al. (2020) developed a plug-and-play device for customer-side energy storage and an internet-based energy storage cloud platform, aiming to investigate the operating state and service life of energy storage devices. A kind of energy optimization and deployment strategy for stratified partition is designed to reduce the operating cost of the energy storage device. By means of MATLAB/Simulink, Biech et al. (2016) presented a simulation tool for the computation of amortization curves for the investment of local ESS. It enables the exploration of a wide search space by manipulating settings on the levels of entire neighborhoods that might want to share in local energy storage.
Some scholars have studied the service model and the operation mechanism of Share-ESS. Oh and Son (2019) proposed a shared energy storage service model for apartment-type factory buildings. The proposed model aims to maximize the profit of the service participants and the service provider. Liu et al. (2017) described the control and communication technologies as well as the business model that is required for the implementation of Shared-ESS. In this model, users can use the Shared-ESS just like their own energy storage facilities, while the Shared-ESS operator will invest and operate centralized batteries to provide energy storage services to the users. Based on the business model and operation mechanism of Shared-ESS, Chen et al. (2018) established the basic Shared-ESS operation model, according to the charging and discharging behavior of the users. Lombardi and Schwabe (2017) developed a business model based on the sharing economy principle. The authors investigated two scenarios: in the first one, a battery owner offers the service of his/her batteries to other users, and in the second one, an independent operator manages the battery.
The optimal capacity of the Shared-ESS is also one of the directions worth studying. Kim and Kim (2017) proposed a method that finds an optimal capacity of Shared-ESS and individual PV system minimizing the total energy cost, which consists of the expenses to buy electricity and to install the Shared-ESS. Wang et al. (2018) raised a new concept of “charging envelop,” which can dynamically allocate the capacity of Shared-ESS between users and distribution network operators. Two goals can be achieved: minimizing the energy costs for users and releasing the distribution network constraints. Tushar et al. (2016) investigated the ownership sharing of ESS between residential units and multiple shared facility controllers. A modified auction-based mechanism is designed to determine the auction price and the allocation of energy storage shared by the residential units. Coincidentally, Zaidi et al. (2018) also applied the auction mechanism to carry out the capacity allocation of Shared-ESS. The households are allowed to submit bids in single bid format or in combinations of bids to the auctioneer to get storage capacity in Shared-ESS. The authors also presented a novel winner determination solution for combinatorial auctions, which combines the genetic algorithm with particle swarm optimization.
Besides, the service pricing of Shared-ESS is also of vital importance. Steriotis et al. (2019) presented a Shared-ESS real-time pricing model, which achieves an amazing tradeoff of the interest between the service provider and end users. In Zhang et al. (2020), the service price is determined by the marginal cost of the residential load aggregator, and the load dispatching is formulated as a fixed-point problem, which can better capture the marginal cost and the interaction between electricity and demand. Kim and Kim (2019) took the optimal capacity and service price of the Shared-ESS into consideration simultaneously and formulated a problem of optimal capacities of Shared-ESS for the residential users and an optimal unit price of Shared-ESS for the aggregator.
Plenty of research have paid attention to the energy management of Shared-ESS. Rahbar et al. (2016) proposed a shared energy storage management for high renewable penetrated smart grid. The profit allocation is determined by the profit coefficients of different users that were set by the system operator. AlSkaif et al. (2017) presented a centralized energy management system with a shared battery in microgrid. The proportion of energy in the shared battery is distributed based on the reputation function of the household users. Tascikaraoglu et al. (2019) proposed a direct load control approach for improving the electricity network using shared ESS and demand response of HVAC units. The shared ESS is controlled by the system operator to support the demand response event and maintain the comfort level of HVAC users. Carli and Dotoli (2017) presented a distributed control algorithm for the energy scheduling of a group of residential users. The algorithm is based on an iterative procedure combining parametric optimization. Dai and Charkhgard (2018) introduced three energy storage sharing strategies, namely, extreme free strategy, extreme fair strategy, and contract balance strategy, and developed a bi-objective mixed integer linear programming (MILP) model for each strategy. Taking into consideration the behavior uncertainty of the users, Hosseini et al. (2019) designed a tractable robust optimization scheme with a quadratic cost function, minimizing the total energy payment of each user. Also considering uncertainty, Zhu and Ouahada (2019a,b) described the energy management problem as a constrained stochastic programming problem and designed a centralized real-time sharing control algorithm based on the Lyapunov optimization theory. Liu J. et al. (2018) accounted for the investment and operation decisions of both the Shared-ESS operator and the users and exploited the complementarity of the users as well as economies of scale. Taşcikaraoglu (2018) proposed a methodology that provides an energy credit to each household for the excess renewable energy. These energy credits are then used during peak periods, resulting in a virtual shift of excess energy to the periods with higher load demand. Chen et al. (2017) formulated the energy management problem as a bi-level mathematical model. The upper level model optimizes the allocation of energy storage among the distribution company and users, while the lower level model minimizes the electricity purchase costs of the distribution company and users. The bi-level model is transformed into a single-level mathematical program with the equilibrium constraints model.
However, the aforementioned research work mainly focuses on the control and pricing of the energy exchange between ESS and the energy users and the behind-meter trading among the users, though the commonly connected ESS is less considered. According to the modular bi-directional converter infrastructure proposed in Sickel et al. (2005), the trading among various users behind the meter can be achieved by using multiple individual AC/DC converter modules and shared DC/DC module, and a demonstration project is adopted in Malmo, Sweden (Fu et al., 2016). Therefore, benefiting from the power flow controllability of AC/DC modules, the power trading among the participants is controllable, and the billing of energy trading or selling/buying electricity to/from the ESS can be achieved behind the meter by adding metering unit on the AC/DC module. In this article, we proposed the concept of Shared-ESS for microgrid cluster, which enables the peer-to-peer (P2P) trading among participants and trading between participants and Shared-ESS. The proposed concept can bring benefits to both participants and the Shared-ESS operator.
The main contributions of this article are summarized below:
• Proposed the Shared-ESS concept that enables the behind-meter P2P trading among microgrids and the trading between microgrids and external grid.
• Introduced an optimized day-ahead operational scheduling approach for microgrids cluster with Shared-ESS.
• Performed a comprehensive analysis of Shared-ESS under different scenarios of microgrid clusters and evaluated the influence of Shared-ESS capacity and the number of microgrids to the cluster operation.
The remainder of this article is organized as follows. Section The Architecture of Microgrid Cluster With Shared-ESS presents the architecture of the proposed microgrid cluster with Shared-ESS. Section Problem Formulation and Optimization presents the problem formulation and optimization of the Shared-ESS in microgrid cluster. Section Comparative Performance Scenarios presents the evaluation of the proposed system performance under different scenarios, and the conclusion is drawn in section Conclusion.
The Architecture of Microgrid Cluster With Shared-ESS
The general infrastructure of microgrid cluster with Shared-ESS is presented in Figure 1. The microgrid contains a number of electricity consumers and usually has the renewable generators like wind turbines and photovoltaic generators. The microgrid is able to buy/sell electricity from/to the external grid, store/buy electricity in/to the Shared-ESS, and buy/sell electricity from/to neighborhood microgrid through the common DC bus of Shared-ESS.
As the core equipment that helps micro-grid achieve P2P transaction and temporary energy storage, the infrastructure of Shared-ESS is given in Figure 2. The microgrid is connected to both external grid and the AC/DC module of the Shared-ESS. Each of the microgrid within the cluster will connect to one AC/DC module of Shared-ESS, and the DC terminal of AC/DC module is connected to the common DC bus, so that the microgrids can exchange energy flow through the common DC bus. In addition, if the surplus energy generated by renewable sources cannot be consumed by the microgrids, the surplus energy will be stored in the Shared-ESS during off-peak time unless the Shared-ESS is fully charged.
The advantages of adopting Shared-ESS for microgrid cluster are summarized below:
• During peak-time, Shared-ESS can reduce the amount of electricity bought from the grid by discharging the stored power to the microgrids.
• The Shared-ESS can reduce the fluctuation of electricity generation caused by renewable generations.
• The Shared-ESS enables the energy exchange by transmitting electricity through common DC bus rather than by charging/discharging the battery itself, which is not necessary to charge/discharge the battery frequently.
Problem Formulation and Optimization
In this section, the objective function, mathematical models of core equipment, and constraints of the day-ahead microgrid cluster optimal dispatch problem are described. Taking the output of renewable generators and electric load of each microgrid and the electricity price of external grid as inputs, the optimal scheduling strategy can be obtained by solving the mathematical model to achieve the economic optimal operation of the microgrid cluster.
Objective Function
The objective function is to minimize the total cost of the microgrid cluster. The total cost includes the operation and maintenance cost of renewable generators, the cost of purchasing electricity from the grid, the cost of buying electricity from Shared-ESS, and the payoff of selling surplus electricity to the external grid and the Shared-ESS:
where the Cost represents the total operational cost of microgrid cluster; stands for the operation and maintenance cost of the renewable generators in microgrid i; / express the cost of exchanging power of microgrid i with the external grid and the Shared-ESS, respectively; IMG means the number of microgrids; T indicates the scheduling cycle; denotes the scheduling time resolution; / represent the operation and maintenance cost factor of photovoltaic generators and wind turbines, respectively; /// are the electricity prices at which microgrid i buy/sell electricity from/to external grid/Shared-ESS at time t, respectively; stand for the output power of photovoltaic generators and wind turbines in microgrid i at time t; and /// express the electric power that microgrid i buy/sell from/to extern grid/Shared-ESS at time t, respectively.
The purchase/sale electricity price of Shared-ESS is determined by Equations (5–7) based on the pricing methodology proposed in Zhou et al. (2020b):
where the / mean the base electricity price at which microgrid i buy/sell electricity from/to Shared-ESS at time t; indicates the power of electric load in microgrid i at time t; represents the ratio of electric load power to renewable energy power; and is the value of normalized to the interval [−1, 1].
Constraints
Constraints on the Electric Power Balance of Microgrids
The electric power balance has to be met in each microgrid:
Constraints on the Electric Power Balance of Common DC Bus in Shared-ESS
The electric power balance has to be met in the common DC bus inside Shared-ESS, as shown in (9). If there is a surplus in the power generated by renewable energy, the remaining power will be sold to other microgrids first. If there is still power remaining on the common DC bus, the battery of the Shared-ESS will be charged. Thus, though the common DC bus, the P2P transaction between the microgrids can be realized.
where the / stand for the value of the electric power bought/sold from/to Shared-ESS transmitted to the common DC bus in microgrid i at time t and / express the value of the charge/discharge power of the battery in Shared-ESS transmitted to the DC bus in microgrid i at time t.
Constraints on the Upper and Lower Limits of Electric Power Purchased/Sold From/to External Grid
Due to the capacity limitations of the transmission lines and transformers, the interaction power of each microgrid and external grid has upper and lower limits. Meanwhile, at each moment, the power purchase/sale behavior between microgrids and external grid cannot occur at the same time:
where the / are 0–1 variables, meaning that the microgrid i and external grid power buy/sale status at time t and / indicate the upper limit of electric power bought/sold from/to external grid.
Constraints on Upper and Lower Limits of Electric Power Purchased/Sold From/to Shared-ESS
Due to the capacity limitations of the transmission lines and AC/DC converters, the interaction power of each microgrid and Shared-ESS has upper and lower limits. Meanwhile, at each moment, the power purchase/sale behavior between microgrids and Shared-ESS cannot occur at the same time:
where the / are 0–1 variables, representing that the microgrid i and Shared-ESS power buy/sale status at time t and / are the upper limit of electric power bought/sold from/to Shared-ESS.
Constraints on Preventing the Transactions Between Shared-ESS and External Grid via Microgrids
In consideration of economy and safety, microgrids are not allowed to sell the electricity purchased from external grid to Shared-ESS or sell the electricity purchased from Shared-ESS to external grid at the same time:
Constraints on the Charge and Discharge Power of the Battery in Shared-ESS
Due to the capacity limitations of the battery and the DC/DC converter, there is an upper limit on the charge and discharge power of the battery in Shared-ESS. Meanwhile, in order to prevent unnecessary battery loss, this power has a lower limit. In addition, the charge and discharge behavior of the battery cannot occur at the same time:
where the / are 0–1 variables, standing for the charge/discharge status of the battery in Shared-ESS at time t; / express the charge/discharge power of the battery at time t; and /// mean the upper/lower limit of the charge/discharge power of the battery at time t.
Constraints on the Cycle Power of the Battery in Shared-ESS
In order to reduce the loss of the battery and thus ensure the service life of the battery, constraints on the charge and discharge cycle power of the battery have to be added:
where the indicates the maximum cycle power of the battery.
Constraints on the Energy of the Battery in Shared-ESS
In order to ensure the normal operation of the battery, the upper and lower limits of the energy of the battery in Shared-ESS should be set. In addition, the energy of the battery at each time has a certain relationship with the charge or discharge power of the previous time. Moreover, to ensure the sustainability of the scheduling strategy, the energy of the battery has to be made equal at the beginning and the end of each scheduling cycle:
where the represents the energy of the battery in Shared-ESS at time t; / are the lower/upper limit of the energy of the battery; and σESS stands for the self-discharge rate of the battery.
Constraints on the Efficiency of Energy Conversion in Shared-ESS
Because of the power loss of bidirectional converters in Shared-ESS, the efficiency constraints of each device should be added:
where the ηESS, DC, AC expresses the efficiency of DC/AC converters to convert electricity from DC to AC; ηESS, AC, DC means the efficiency of DC/AC converters to convert electricity from AC to DC; ηESS, dis indicates the efficiency of DC/DC converter when the battery is dischargeηESS, DC, ACd; and ηESS, ch denotes the efficiency of DC/DC converter when the battery is charged.
Comparative Performance Scenarios
To evaluate the performance of the proposed Shared-ESS in microgrid cluster, a number of case studies have been performed in this section. The power exchanged among the microgrids, the charging/discharging actions of shared-ESS, and the influence of the capacity of the battery in Shared-ESS to the whole system operation in different scenarios are discussed in this section as well. The modeling, solving, and analysis of the numerical analysis are programmed based on MATLAB R2018b, and the IBM ILOG CPLEX Optimization Studio is used as the solver to find the optimal day-ahead scheduling of the proposed model.
The six scenarios used for evaluating the Shared-ESS are listed in Table 1. The microgrid models are of three types: (1) commercial type, (2) domestic type, and (3) industrial type. The Time of Use (TOU) tariff is adopted for purchasing electricity from external grid by microgrid. The peak time duration is between 08:00–11:00 and 18:00–23:00; the off-peak time duration is between 07:00–08:00 and 11:00–18:00; and the valley time duration is between 23:00 and 07:00. The real-time trading electricity price is shown in Table 2. The feed-in tariff of export renewable generation to external grid is set as 0.34 RMB/(kWh).
The operational parameters for the system are listed in Table 3.
Basic Scenario
The basic scenario refers to scenario 1 in Table 1, the capacity of Shared-ESS is 3,000 kWh, and the microgrid cluster contains 4 microgrids. The operational performance of the microgrid cluster is shown in Figures 3–5.
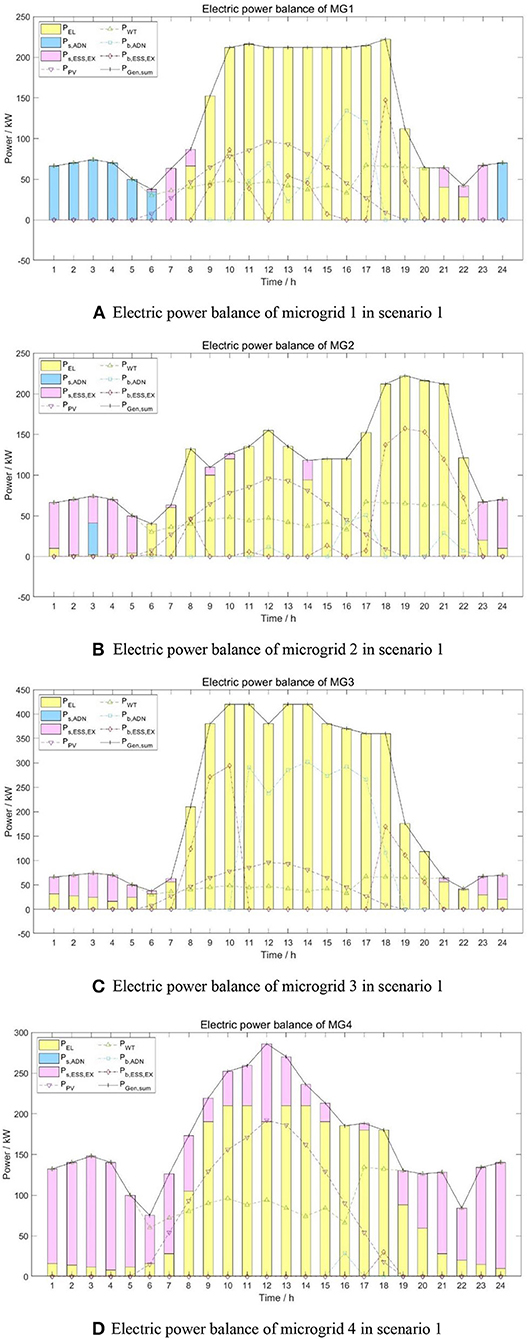
Figure 3. Electric power balance of microgrids in scenario 1. (A) Electric power balance of microgrid 1 in scenario 1. (B) Electric power balance of microgrid 2 in scenario 1. (C) Electric power balance of microgrid 3 in scenario 1. (D) Electric power balance of microgrid 4 in scenario 1.
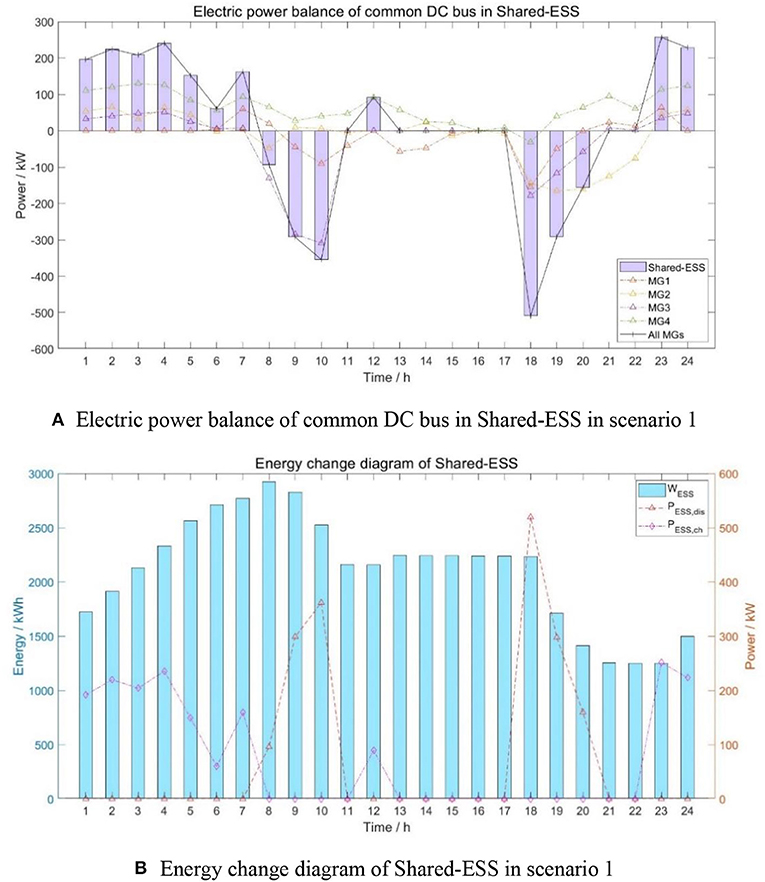
Figure 4. The operating status of Shared-ESS in scenario 1. (A) Electric power balance of common DC bus in Shared-ESS in scenario 1. (B) Energy change diagram of Shared-ESS in scenario 1.
Operation Overview
According to Figure 3, it can be found that electricity demand can be satisfied by purchasing electricity from Shared-ESS rather than from the external grid during most of the peak time; during the off-peak time duration, the microgrids will purchase the inexpensive electricity from the external grid to feed the demand gap that the renewable generator cannot support; during the valley time, the surplus electricity generated by wind turbines will be stored into the Shared-ESS rather than selling to the external grid, which bring extra benefits to the microgrids.
However, due to the limited capacity of the battery in Shared-ESS, a considerable portion of the surplus power in microgrid 1 is sold to the external grid. The reason why the electricity of microgrid 1 is sold instead of other microgrids is because during this period, microgrid 1 has the lowest electricity price to Shared-ESS. Therefore, microgrid 1 sells surplus power to the external grid, so that the entire microgrid group can obtain the maximum economic benefits.
Power Exchange Among Microgrids
During 13:00–16:00 and 21:00–23:00, since the renewable energy of microgrid 4 generates more electricity, the power needs to be sold to meet the power balance of microgrid 4. However, other microgrids are still unable to make ends meet. At this time, it is not necessary to choose to sell electricity to the external grid with a low feed-in-tariff, and it is also not necessary to charge the battery in Shared-ESS. Electricity can be sold to microgrid 2 and microgrid 1 indirectly through the common DC bus inside Shared-ESS. In this way, the energy transmission efficiency is improved, the battery loss is reduced, and the cost of electricity is reduced too. Similarly, as for the selection of the microgrid, the algorithm will automatically choose to make microgrid 4 interact with the microgrid with the lowest cost of purchasing power from Shared-ESS.
Economic Benefit Analysis
The energy bills of each microgrid within the cluster are presented in Figure 5. It can be seen that the microgrid 3 with a higher electric load purchases more electricity from the external grid, so the cost is higher; and the microgrid 4 with more renewable energy sources sells more electricity to Shared-ESS, so as to obtain more profits.
As shown in Figure 4, during the period when the renewable energy generation of microgrids exceed the electric load (23:00–7:00 the next day), the battery in Shared-ESS is charged; and during the period when the electricity price of external grid is very high (08:00–11:00 and 18:00–21:00), the battery in Shared-ESS is discharged. It can be seen that the use of Shared-ESS can not only play a role in shifting the usage of energy during peak time but also achieve the consumption of renewable energy.
Non-Shared-ESS Scenario
The non-Shared-ESS scenario refers to scenario 2 in Table 1. It has no Shared-ESS, and the load profile of the microgrid cluster is the same as scenario 1. The energy bills of each microgrid within the cluster are presented in Figure 6.
Operation Overview
Since there are no Shared-ESS in this scenario, the microgrid can only sell surplus electricity to the external grid and purchase the electricity from the external grid when necessary, which will result in compressed profit margins.
Economic Benefit Analysis
The daily electricity bill of the microgrid cluster without Shared-ESS is 4,070.6475RMB, which is 699.8284RMB higher than the cost of scenario 1. For the high renewable penetrated microgrid 4, the payoff is reduced from 557.6242RMB to 402.2410RMB (38.63% lower).
In general, compared with the microgrid cluster without Shared-ESS, the cluster equipped with Shared-ESS can reduce the total cost of purchasing electricity from the grid and improve the profitability of the microgrid that acts as a prosumer in the cluster.
Influence of the Capacity of the Battery in Shared-ESS to Microgrid Cluster
Larger capacity of Shared-ESS can correspondingly improve the flexibility of microgrid clusters. However, larger Shared-ESS means higher investment toward the physical equipment. In this part, various capacities (1,000 and 5,000 kWh) of the battery in Shared-ESS are evaluated and compared to scenario 1. These scenarios refer to scenario 3 and cenario 4 in Table 1. The operational results are given in Figures 7, 8.
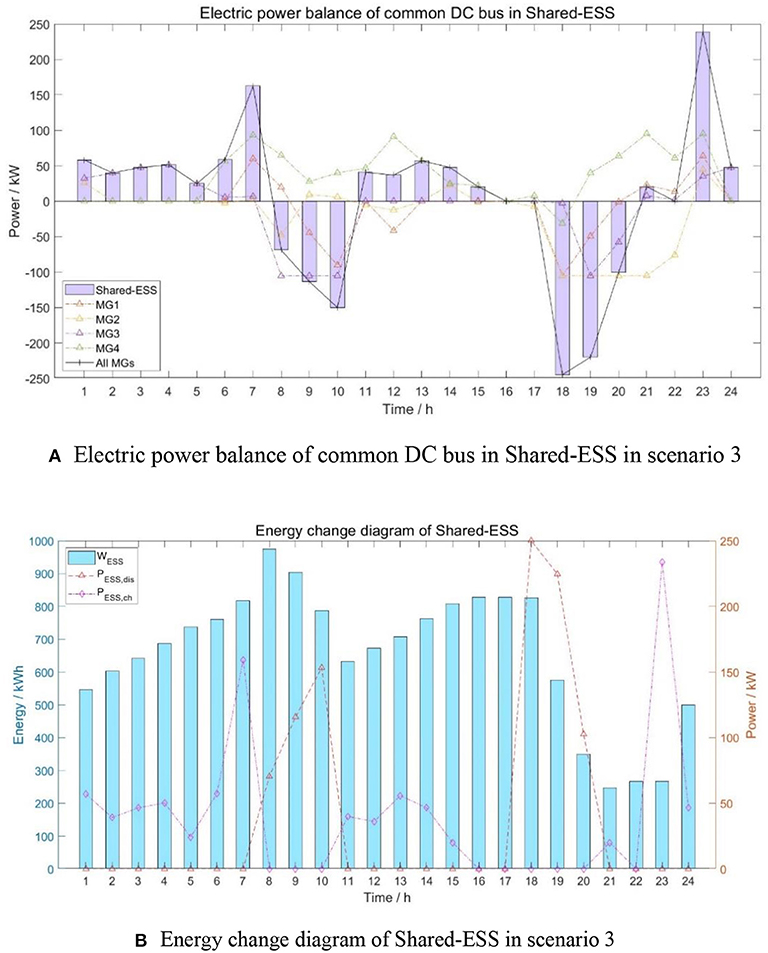
Figure 7. The operating status of Shared-ESS in scenario 3. (A) Electric power balance of common DC bus in Shared-ESS in scenario 3. (B) Energy change diagram of Shared-ESS in scenario 3.
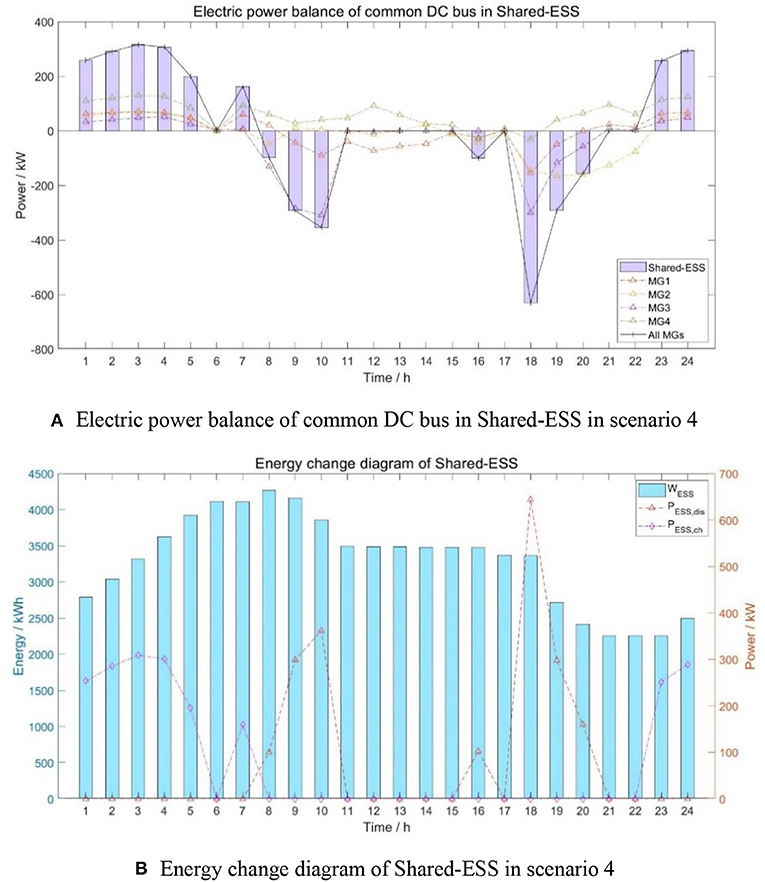
Figure 8. The operating status of Shared-ESS in scenario 4. (A) Electric power balance of common DC bus in Shared-ESS in scenario 4. (B) Energy change diagram of Shared-ESS in scenario 4.
Operation Overview
When the capacity of the battery in Shared-ESS is 1,000 kWh, due to the insufficient battery capacity, a considerable part of the energy generated by renewable energy is not stored in the battery but is sold to the external grid due to lower feed-in-tariff. As a result of the power limitation of the AC/DC converters, during the peak period of power consumption, the demand of the load could not be met when the Shared-ESS reached the upper limit of the power sold to the microgrids. Therefore, it is still necessary to purchase electricity from the external grid at a high price. In fact, even if the power limitation of the AC/DC converter is not considered, the ESS still could not meet the load demand during the peak period of power consumption, because the battery capacity is so small that the stored energy is not enough.
When the capacity of the battery in Shared-ESS is 5,000 kWh, almost all the output of renewable energy is stored into Shared-ESS. During the period when the electricity price is high, most of the load demand is satisfied by Shared-ESS. There is still a small part that needs to be purchased from the external grid, because the battery of Shared-ESS needs to keep the energy equal to the beginning after the end of a scheduling cycle.
Power Exchange Among Microgrids
For scenario 3, as the renewable energy output of microgrid 4 is relatively large, a part of the energy still needs to be sold to other microgrids through the common DC bus, but this part of energy reduces a lot, for the battery of Shared-ESS needs to be charged at noon to meet the electricity demand in the evening.
For scenario 4, the situation in which the microgrid 4 with more renewable energy output supplies energy to other microgrids through the common DC bus is not much different from the basic scenario.
Economic Benefit Analysis
When the capacity of the battery in Shared-ESS is reduced from 3,000 to 1,000 kWh, the total electricity cost of the microgrid cluster increases from 3,360.8191RMB to 3,564.7461RMB, which is 203.927RMB higher. According to the different electric load, the cost of microgrids 1–3 increases to a different extent. It can be seen that, in this situation, reducing the capacity of the battery in Shared-ESS is inconducive not only to shift the usage of energy during peak time but also to significantly increase the cost of electricity.
When the capacity of the battery in Shared-ESS is increased from 3,000 to 5,000 kWh, the total electricity cost of the microgrid cluster reduces from 3,360.8191RMB to 3,302.0391RMB, which is 58.78RMB lower. Each microgrid has a slight decrease in cost or increase in profit, but not significantly. It can be seen that, in this situation, increasing the capacity of the battery in Shared-ESS does not play an important role, and the cost reduction is very small. Obviously, this reduction is not worth the additional investment costs.
In summary, about 3,000 kWh is an ideal capacity for the battery in Shared-ESS.
Influence of the Number of Microgrids to Microgrid Cluster
This case corresponds to scenarios 5 and 6 in Table 1. The battery in Shared-ESS has a capacity of 3,000 kWh, and 6 microgrids in the microgrid cluster are connected to Shared-ESS. The results of the optimization are shown in Figures 9–11.
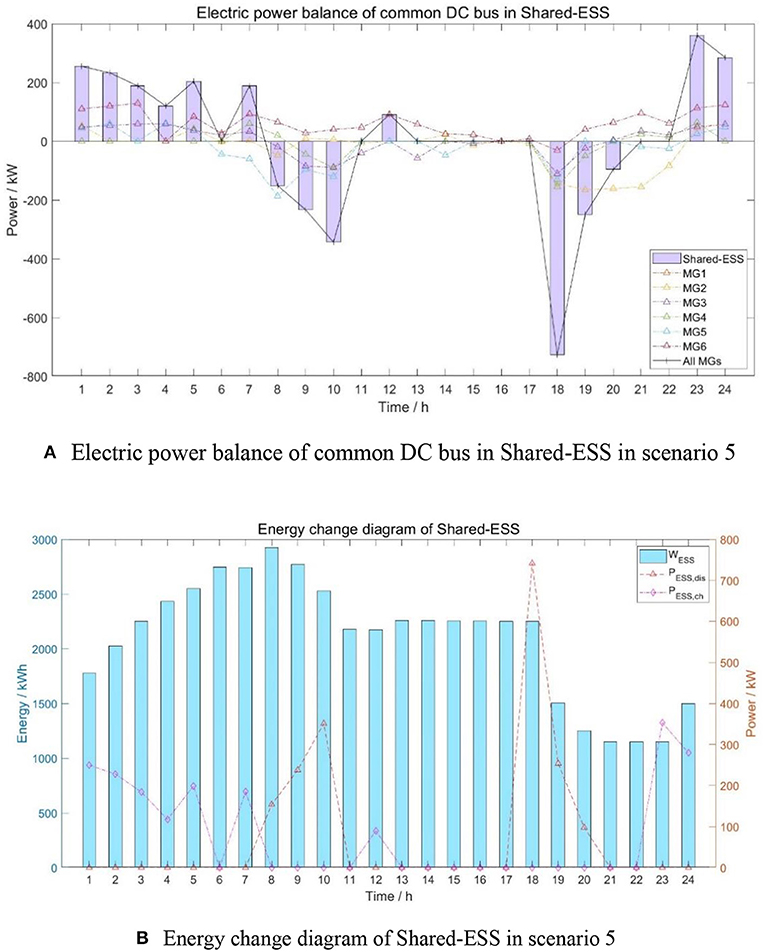
Figure 9. The operating status of Shared-ESS in scenario 5. (A) Electric power balance of common DC bus in Shared-ESS in scenario 5. (B) Energy change diagram of Shared-ESS in scenario 5.
Operation Overview
When the number of the microgrids increases to 6, the 3,000 kWh battery capacity is not enough. At this time, some of the renewable generation output could not be stored in the battery in Shared-ESS, but is sold to the external grid. And during the peak period of power consumption, a considerable part of electric load is satisfied by purchasing electricity from the external grid (of course, microgrids with higher electricity purchase prices from Shared-ESS at this time are chosen to buy electricity from the external grid).
However, when the number of microgrids is larger, the battery in Shared-ESS and microgrids with more renewable energy generation can choose microgrids for energy trading more freely at different time. Therefore, the algorithm will choose the microgrid with the lowest electricity purchase price from Shared-ESS, thereby saving more electricity cost for the microgrid cluster.
Power Exchange Among Microgrids
The microgrid 6 with more renewable energy generation sells electricity to microgrid 5, microgrid 3, and microgrid 2 at different time, depending on which microgrid has a lower electric purchase price. Under this circumstance, there are more opportunities for energy transactions between microgrids, and more economical transaction ways will be selected, thereby achieving better economic benefits.
Economic Benefit Analysis
When the number of microgrids is 6, the saved electricity cost is 847.7616RMB, which is much more than the 699.8284RMB when the number of microgrids is 4. It can be seen that when the number of microgrids increases, for the microgrid cluster with Shared-ESS, it facilitates reducing the total energy cost of the system.
Conclusion
This article proposes an economic optimal scheduling method for microgrid clusters with Shared-ESS. The global energy optimization management of the microgrid cluster can be achieved based on the established model and the optimization method. The microgrids can realize bidirectional energy flow with Shared-ESS through the common connected DC bus, thereby achieving energy storage and P2P transaction among each other. Case studies show that after equipping with the Shared-ESS, the microgrid cluster can save up to 17.23% of the total electricity cost, which is quite significant. For this microgrid cluster system, 3,000 kWh is a relatively reasonable battery capacity, based on the evaluations of different sizes of the Shared-ESS. The greater the number of microgrids connected to Shared-ESS, the more the electricity consumption costs can be saved, benefiting from the better power exchange among the microgrids. It can be concluded that the Shared-ESS effectively facilitates the consumption of renewable energy, plays an important role in shifting the usage of energy during peak time, improves energy utilization efficiency, and reduces electricity costs. Therefore, by optimizing the charge and discharge status and the power of the battery in Shared-ESS, and the purchase and sale strategy of the microgrids, the operating cost of the microgrid cluster system can be significantly reduced, and the day-ahead economic optimal dispatch of the microgrid cluster can be achieved.
Data Availability Statement
The raw data supporting the conclusions of this article will be made available by the authors, without undue reservation.
Author Contributions
SC and SZ conceptualized the study and contributed to the investigation. HZ and MC contributed to the study methodology. YW contributed to the software. KC and SZ contributed to the validation and writing—review and editing. HZ and SZ contributed to the formal analysis and visualization. SC and HZ contributed to the writing—original draft preparation. MC and SC contributed to the supervision. KC, YW, MC, and SC contributed to the funding acquisition. All authors have read and agreed to the published version of the manuscript.
Funding
The authors declare that this study received funding from Science and Technology Project of Jiangsu Electric Power Company “Key Technologies and Research of Smart Energy Service for City-Scale Energy Internet” under the grand number J2019089. The funder was not involved in the study design, collection, analysis, interpretation of data, the writing of this article or the decision to submit it for publication.
Conflict of Interest
SC, KC, MC, and YW was employed by Yangzhou Power Supply Company, Jiangsu Electric Power Company, State Grid Cooperation of China.
The remaining authors declare that the research was conducted in the absence of any commercial or financial relationships that could be construed as a potential conflict of interest.
Acknowledgments
The authors would like to thank the sponsorship of Science and Technology Project of Jiangsu Electric Power Company.
References
AlSkaif, T., Luna, A. C., Zapata, M. G., Guerrero, J. M., and Bellalta, B. (2017). Reputation-based joint scheduling of households appliances and storage in a microgrid with a shared battery. Energy Build. 138, 228–239. doi: 10.1016/j.enbuild.2016.12.050
Biech, M., Bigdon, T., Dielitz, C., Fromme, G., and Remke, A. (2016). “A smart neighbourhood simulation tool for shared energy storage and exchange,” in Analytical and Stochastic Modelling Techniques and Applications. ASMTA 2016. Lecture Notes in Computer Science. eds S. Wittevrongel and T. Phung-Duc (Cham: Springer), 76–91. doi: 10.1007/978-3-319-43904-4_6
Borghetti, A., Bosetti, M., Grillo, S., Massucco, S., Nucci, C. A., Paolone, M., et al. (2010). Short-term scheduling and control of active distribution systems with high penetration of renewable resources. IEEE Syst. J. 4, 313–322. doi: 10.1109/JSYST.2010.2059171
Broering, H., and Madlener, R. (2017). Simulation and evaluation of the economic merit of cloud energy storage for prosumers: the case of Germany. Energy Proc. 105, 3507–3514. doi: 10.1016/j.egypro.2017.03.804
Carli, R., and Dotoli, M. (2017). Cooperative distributed control for the energy scheduling of smart homes with shared energy storage and renewable energy source. IFAC Papers Online 50, 8867–8872. doi: 10.1016/j.ifacol.2017.08.1544
Chen, H., Cheng, H., Xu, G., Zhou, W., Zhang, X., and Sang, D. (2018). “Research on consumer side energy storage optimization configuration based on cloud energy storage,” in 2018 3rd International Conference on Smart City and Systems Engineering (Xiamen: ICSCSE; IEEE). doi: 10.1109/ICSCSE.2018.00102
Chen, H., Yu, Y., Hu, Z., Luo, H., Tan, C., and Rajagopal, R. (2017). “Energy storage sharing strategy in distribution networks using bi-level optimization approach,” in 2017 IEEE Power & Energy Society General Meeting (Chicago, IL). doi: 10.1109/PESGM.2017.8274595
Dai, R., and Charkhgard, H. (2018). Bi-objective mixed integer linear programming for managing building clusters with a shared electrical energy storage. Comput. Oper. Res. 96, 173–187. doi: 10.1016/j.cor.2018.01.002
Denholm, P., and Hand, M. (2011). Grid flexibility and storage required to achieve very high penetration of variable renewable electricity. Energy Policy 39, 1817–1830. doi: 10.1016/j.enpol.2011.01.019
Fu, H., Wu, Z., Li, J., Zhang, X., and Brandt, J. (2016). A configurable VPP with managed energy services: a malmo western harbour case. IEEE Power Energy Technol. Syst. J. 3, 166–178. doi: 10.1109/JPETS.2016.2596779
Hosseini, S. M., Carli, R., and Dotoli, M. (2019). “Robust energy scheduling of interconnected smart homes with shared energy storage under quadratic pricing,” in 2019 IEEE 15th International Conference on Automation Science and Engineering (Vancouver, BC: CASE). doi: 10.1109/COASE.2019.8843230
Kim, I., and Kim, D. (2017). “Optimal capacity of shared energy storage and photovoltaic system for cooperative residential customers,” in 2017 International Conference on Information and Communications (Hanoi: ICIC). doi: 10.1109/INFOC.2017.8001682
Kim, I., and Kim, D. (2019). “Pricing-based shared energy storage optimization for residential users with photovoltaic generation system and demand-side load management,” in 2019 Eleventh International Conference on Ubiquitous and Future Networks (Zagreb: ICUFN). doi: 10.1109/ICUFN.2019.8806069
Li, Y., Gao, D. W., Gao, W., Zhang, H., and Zhou, J. (2020). Double-mode energy management for multi-energy system via distributed dynamic event-triggered newton-raphson algorithm. IEEE Trans. Smart Grid 11, 5339–5356. doi: 10.1109/TSG.2020.3005179
Li, Y., Zhang, H., Liang, X., and Huang, B. (2019). Event-triggered-based distributed cooperative energy management for multienergy systems. IEEE Trans. Smart Grid 4, 2008–2022. doi: 10.1109/TII.2018.2862436
Liu, J., Zhang, N., Kang, C., Kirschen, D., and Xia, Q. (2017). Cloud energy storage for residential and small commercial consumers: a business case study. Appl. Energy 188, 226–236. doi: 10.1016/j.apenergy.2016.11.120
Liu, J., Zhang, N., Kang, C., Kirschen, D. S., and Xia, Q. (2018). Decision-making models for the participants in cloud energy storage. IEEE Trans. Smart Grid 9, 5512–5521. doi: 10.1109/TSG.2017.2689239
Liu, N., Cheng, M., Yu, X., Zhong, J., and Lei, J. (2018). Energy-sharing provider for pv prosumer clusters: a hybrid approach using stochastic programming and stackelberg game. IEEE Trans. Ind. Electron. cs 65, 6740–6750. doi: 10.1109/TIE.2018.2793181
Lombardi, P., and Schwabe, F. (2017). Sharing economy as a new business model for energy storage systems. Appl. Energy 188, 485–496. doi: 10.1016/j.apenergy.2016.12.016
Oh, E., and Son, S. (2019). Shared electrical energy storage service model and strategy for apartment-type factory buildings. IEEE Access 7, 130340–130351. doi: 10.1109/ACCESS.2019.2939406
Rahbar, K., Moghadam, M. R. V., Panda, S. K., and Reindl, T. (2016). “Shared energy storage management for renewable energy integration in smart grid,” in 2016 IEEE Power & Energy Society Innovative Smart Grid Technologies Conference (Minneapolis, MN: ISGT). doi: 10.1109/ISGT.2016.7781230
Roberts, M. B., Bruce, A., and MacGill, I. (2019). Impact of shared battery energy storage systems on photovoltaic self-consumption and electricity bills in apartment buildings. Appl. Energy 245, 78–95. doi: 10.1016/j.apenergy.2019.04.001
Sickel, R., Vetters, D., Mehlich, H., Bodach, M., Bocklisch, T., and Lutz, J. (2005). “Modular converter for fuel cell systems with buffer storage,” in 2005 European Conference on Power Electronics and Applications (Dresden: IEEE). doi: 10.1109/EPE.2005.219721
Steriotis, K., Tsaousoglou, G., Efthymiopoulos, N., Makris, P., and Varvarigos, E. (2019). Real-time pricing in environments with shared energy storage systems. Energy Effic. 12, 1085–1104. doi: 10.1007/s12053-018-9723-8
Swierczyński, M., Stroe, D. I., Stan, A. I., and Teodorescu, R. (2013). “Primary frequency regulation with Li-ion battery energy storage system: a case study for Denmark,” in 2013 IEEE ECCE Asia Downunder (Melbourne, VIC: IEEE). doi: 10.1109/ECCE-Asia.2013.6579141
Taşcikaraoglu, A. (2018). Economic and operational benefits of energy storage sharing for a neighborhood of prosumers in a dynamic pricing environment. Sustain. Cities Soc. 38, 219–229. doi: 10.1016/j.scs.2018.01.002
Tascikaraoglu, A., Paterakis, N. G., Erdinc, O., and Catalao, J. P. S. (2019). Combining the flexibility from shared energy storage systems and DLC-based demand response of HVAC units for distribution system operation enhancement. IEEE Trans. Sustain. Energy 10, 137–148. doi: 10.1109/TSTE.2018.2828337
Tushar, W., Chai, B., Yuen, C., Huang, S., Smith, D. B., Poor, H. V., et al. (2016). Energy storage sharing in smart grid: a modified auction-based approach. IEEE Trans. Smart Grid 7, 1462–1475. doi: 10.1109/TSG.2015.2512267
Wang, Z., Gu, C., and Li, F. (2018). Flexible operation of shared energy storage at households to facilitate PV penetration. Renew. Energy 116, 438–446. doi: 10.1016/j.renene.2017.10.005
Yan, T., Liu, J., Niu, Q., Chen, J., Xu, S., Niu, M., et al. (2020). Distributed energy storage node controller and control strategy based on energy storage cloud platform architecture. Glob. Energy Interconnect. 3, 166–174. doi: 10.1016/j.gloei.2020.05.008
Yushuai, L., Gao, W., Gao, W., Zhang, H., and Zhou, J. (2020). A distributed double-newton descent algorithm for cooperative energy management of multiple energy bodies in energy internet. IEEE Trans. Ind. Inform. 8:1. doi: 10.1109/TII.2020.3029974
Zaidi, B. H., Bhatti, D. M. S., and Ullah, I. (2018). Combinatorial auctions for energy storage sharing amongst the households. J. Energy Stor. 19, 291–301. doi: 10.1016/j.est.2018.08.010
Zhang, W., Wei, W., Chen, L., Zheng, B., and Mei, S. (2020). Service pricing and load dispatch of residential shared energy storage unit. Energy 202, 117543. doi: 10.1016/j.energy.2020.117543
Zhou, J., Xu, Y., Sun, H., Li, Y., and Chow, M. (2020). Distributed power management for networked ac–dc microgrids with unbalanced microgrids. IEEE Trans. Ind. Inform. 16, 1655–1667. doi: 10.1109/TII.2019.2925133
Zhou, J., Xu, Y., Sun, H., Wang, L., and Chow, M. (2020a). Distributed event-triggered H∞ consensus based current sharing control of DC microgrids considering uncertainties. IEEE Trans. Ind. Inform. 16, 7413–7425. doi: 10.1109/TII.2019.2961151
Zhou, S., Zou, F., Wu, Z., Gu, W., Hong, Q., and Booth, C. (2020b). A smart community energy management scheme considering user dominated demand side response and P2P trading. Int. J. Electr. Power Energy Syst. 114:105378. doi: 10.1016/j.ijepes.2019.105378
Zhu, H., and Ouahada, K. (2019a). “Cost minimization energy storage sharing management,” in 2019 IEEE International Conference on Communications, Control, and Computing Technologies for Smart Grids (Beijing: SmartGridComm; IEEE). doi: 10.1109/SmartGridComm.2019.8909720
Keywords: shared energy storage system, microgrid cluster, peer-to-peer transaction, economic optimal dispatch, global energy management
Citation: Cao S, Zhang H, Cao K, Chen M, Wu Y and Zhou S (2021) Day-Ahead Economic Optimal Dispatch of Microgrid Cluster Considering Shared Energy Storage System and P2P Transaction. Front. Energy Res. 9:645017. doi: 10.3389/fenrg.2021.645017
Received: 22 December 2020; Accepted: 29 March 2021;
Published: 21 April 2021.
Edited by:
Yi Ding, Zhejiang University, ChinaCopyright © 2021 Cao, Zhang, Cao, Chen, Wu and Zhou. This is an open-access article distributed under the terms of the Creative Commons Attribution License (CC BY). The use, distribution or reproduction in other forums is permitted, provided the original author(s) and the copyright owner(s) are credited and that the original publication in this journal is cited, in accordance with accepted academic practice. No use, distribution or reproduction is permitted which does not comply with these terms.
*Correspondence: Suyang Zhou, c3V5YW5nLnpob3VAc2V1LmVkdS5jbg==