- 1NRC Energy, Mining and Environment(EME), Vancouver, BC, Canada
- 2Institute of Energy and Climate Research, IEK-1: Materials Synthesis and Processing, Forschungszentrum Jülich, Jülich, Germany
- 3Jülich Aachen Research Alliance: JARA-Energy, Jülich, Germany
- 4Institute of Energy and Climate Research, IEK-13: Theory and Computation of Energy Materials, Forschungszentrum Jülich, Jülich, Germany
- 5Chair of Theory and Computation of Energy Materials, Division of Materials Science and Engineering, RWTH Aachen University, Aachen, Germany
Searching for next-generation electrocatalyst materials for electrochemical energy technologies is a time-consuming and expensive process, even if it is enabled by high-throughput experimentation and extensive first-principle calculations. In particular, the development of more active, selective and stable electrocatalysts for the CO2 reduction reaction remains tedious and challenging. Here, we introduce a material recommendation and screening framework, and demonstrate its capabilities for certain classes of electrocatalyst materials for low or high-temperature CO2 reduction. The framework utilizes high-level technical targets, advanced data extraction, and categorization paths, and it recommends the most viable materials identified using data analytics and property-matching algorithms. Results reveal relevant correlations that govern catalyst performance under low and high-temperature conditions.
Introduction
CO2 emissions are the main cause of human-made global warming (Al-Ghussain, 2019). To avert the direst consequences of this global change, the Paris Agreement calls for a net 80–95% reduction of CO2 emissions by 2050 (Rogelj et al., 2015). The rapid development of sustainable energy sources and environmentally benign storage and conversion technologies is thus a foremost goal in scientific research and technology development, pursued collectively in countries around the world.
CO2 can be used as a renewable feedstock for the production of synthetic fuels or fuel precursors such as CO, CH3OH, and CH4, addressing the problem of the intermittency of renewably generated energy from wind turbines and solar cells (Qiao et al., 2014; Lu and Jiao, 2016; Zhu et al., 2016; Liu et al., 2017; Wang et al., 2017). This energy storage pathway renders the CO2 reduction reaction (CO2RR) a crucial and extensively researched electrochemical process (Lin et al., 2020; Mandal, 2020).
CO2RR processes inside an electrochemical cell require stable, cost-effective and highly performing electrocatalyst materials. The challenge of optimizing catalytic materials, electrodes and devices for the CO2RR, calls for further investigation into factors that control their catalytic activity and stability. The electrocatalytic media are usually heterogeneous composites that embed the active material into a host medium with suitable transport properties for gaseous reactants, liquid products, dissolved ions, and electrons. These media can undergo significant changes in structure and composition under operation through various phenomena such as Ostwald ripening, particle detachment or coagulation in nanoparticle-based catalyst layers; surface reconstruction, oxidation or passivation by irreversible adsorption; or electrolyte disintegration. Besides, inhibited mass transport due to non-optimal wetting of the porous electrode could cause additional voltage loss or limit the current density (CD) that a cell could attain.
A recently performed cost-benefit analysis has shown that electrochemical CO2 conversion processes need to be economically viable at the system level, while the faradaic efficiency (FE) and energy efficiency (EF) must be maximized at the component and cell levels (Kibria et al., 2019; Lin et al., 2020). The hydrogen evolution reaction (HER) is an unwanted parallel process in CO2 reduction cells, which impacts the yield of synthetic fuel or fuel precursor production (Goyal et al., 2020). Minimization of hydrogen production requires electrocatalyst materials that are highly selective in terms of the reaction pathway to support.
The integration, testing and qualification of new catalyst materials is a tedious and time-consuming process as there are limitations even for the best catalysts due to specific compatibilities that are required with other components in a membrane electrode assembly (MEA), single cell or stack of the electrochemical device. Challenges in this context involve reactant and product transport as well as water and heat balances. These phenomena are coupled across multiple components and interfaces in a cell, and they determine 3D distributions of local reaction conditions in active electrode media. Assessing the impact of a new catalyst material on performance at cell and stack levels is thus a complex undertaking. An electrocatalyst material may show markedly improved activity and selectivity in a well-defined lab set-up under precisely controlled reaction conditions; but this improvement may not transpire when the material is incorporated into a real cell and tested under realistic conditions.
Complex electrocatalytic media cannot be studied solely with computational studies based on density functional theory (DFT). Usually, the complexity of materials, components and physicochemical phenomena to be considered as well as the interplay of solvation effects, charge transfer, and electric field effects at the interface, warrant a well-devised hierarchical framework in modeling and simulation. This framework should interweave computational approaches, including DFT as well as classical simulations, microkinetic modeling of reaction mechanisms, interface and charge transfer theory, and continuum modeling of transport processes at the electrode level, to rationalize local reaction conditions, decipher reaction mechanisms and calculate reaction rates. Considering all of these aspects, the theory-driven approach towards the development of highly active, selective, and stable electrocatalysts for the CO2RR remains a highly challenging task (Elouarzaki et al., 2019; Ju et al., 2019).
The discovery and scale-up of integrated materials, i.e., those materials that are integrated into a component, cell, and device to fulfill certain functionalities at the device level, require significant capacities for characterization, testing, and optimization at all structural levels. The discovery-to-demonstration pipeline of new electrocatalyst materials, including fabrication scale-up and integration with other cell components is thus more complex to follow through than it is for simpler, so-called “molecular materials,” where minimal integration and optimization is required beyond materials properties (Elouarzaki et al., 2019; Ju et al., 2019). Apart from performance metrics related to activity, yield and selectivity, the degradation of cell components, overall system durability and overall cell lifetime present essential issues to be addressed, which are related to the stability of a catalyst material for relevant environmental conditions and operating regimes.
The key attributes of successful design of CO2 reduction cells include high mass activity of electrocatalysts to perform well at low overpotential and reasonable materials cost, catalyst layer microstructure to facilitate charge and mass transfer, well-attuned wettabilities of porous transport media to optimize the water distribution across the cell, and mechanical and chemical durability. New approaches in materials design and integration are needed to realize the selective transformation of CO2 into desired products in scale-up pilot or industrial setups.
Numerous investigations have recently been made to design, synthesize and develop new CO2RR electrocatalysts (Lu and Jiao, 2016; Liu et al., 2019). Machine learning (ML) and data-driven methods provide a powerful set of methods and tools to accelerate materials discovery (De Luna et al., 2017; Cao et al., 2018). Fundamentally, ML is the practice of using statistical algorithms to parse data, learn from a set of indicators (performance metrics) and then make a fast determination or a prediction of target performance properties of any new data sets. ML in materials science is mostly concerned with supervised learning. One must realize that the selection of high-quality (accurate) datasets in addition to an appropriate set of descriptors is more important than the selection of the ML algorithm itself. The former would be considered as the first step for building any ML application. The suitable ML model, denoted as classification, regression, or rank ordering model, depends on the desired outcome (Elouarzaki et al., 2019).
Describing all the complexities of the electrochemical interface within a DFT model, considering the number and type of components (catalyst, solvent molecules, ions, etc.), as well as the fundamental physics involved (electric fields, solvation free energy, charge transfer kinetics etc.), is challenging due to computational limitations.
Classification models are designed to allocate a substance to a given number of categories such as active and inactive catalysts; they can be used to separate groups of molecules or materials according to the presence or absence of a target property. For instance, CO2RR electrocatalysts can be classified based on their Faradaic efficiency or product selectivity. In this context, several statistical tools, in particular, regression models attempt to determine a function that can represent a continuous hypersurface to relate indicator variance to observable electrocatalytic properties. Regression models are used where prediction and discovery of a missing physico-chemical property such as performance or selectivity are needed (Varnek et al., 2007). Ranking models put out the order of electrocatalysts for a specific property; they are highly useful for electrocatalyst design and discovery, where the priority of one property over another is more important than its exact value (Goldsmith et al., 2018; Lamoureux et al., 2019; Schleder et al., 2019).
Recent self-learning algorithms have greatly influenced catalysis research due to the availability of ML analysis tools, e.g., Python Scikit-learn, TensorFlow and workflow management tools such as ASE (Larsen et al., 2017) or Atomate (Mathew et al., 2017), and the proliferation of large public materials databases, including Materials Project (Jain et al., 2013), Novel Materials Discovery Laboratory (Draxl and Scheffler, 2019), Citrination (Citrine Informatics, 2013), CatApp (Hummelshøj et al., 2012), and AiiDA (Pizzi et al., 2016) and advancement of applied statistical algorithms and models.
ML models have been utilized in a variety of energy material applications to design and discover novel electrocatalyst materials with superior performance (e.g., higher energy density and higher energy conversion efficiency) (Meyer et al., 2018; Zahrt et al., 2019). Such models can have a transformative impact on the development of low cost CO2RR catalysts with high product selectivity and maximal performance (Goldsmith et al., 2018; Kitchin, 2018; Schlexer Lamoureux et al., 2019; Gusarov et al., 2020; Smith et al., 2020). For instance, ML models have been used to disentangle catalyst-adsorbate interactions for various reactions, including CO2RR (Ma et al., 2015; Tran and Ulissi, 2018). A combination of advanced optimization tools based on ML and other conventional approaches has been developed to predict electrocatalyst performance for CO2 reduction and H2 evolution (Tran and Ulissi, 2018).
In this work, we demonstrate a data-driven framework for materials screening, which is particularly applied to low and high temperate catalysts for CO2 reduction (Garza et al., 2018; Kibria et al., 2019; Malek et al., 2019; Chou et al., 2020). A viable electrocatalyst for the CO2RR must satisfy performance metrics related to current density, faradaic efficiency, energy efficiency, overpotential, production rate, and chemical stability. Correlations among these performance metrics at low or high temperature remain largely unknown and require extensive data analytics.
Our data-driven methodology is designed with the objective of integrating domain-specific data sources in order to eliminate difficulties in data collection and interpretation from multiple sources and data types. The integration process consists of a combination of “modular” sub-processes to build “standardized energy materials data” in real-time with advanced filtering, scale-up and cognitive insights, ML, and fundamental data analytics functionalities, including visualization and big-data management tools. The recommendation system and decision module utilize high-level technical targets as input data, which can be displayed in the form of radar (or spider) charts; advanced data extraction and categorization using deep learning techniques; property-matching algorithms to search for the best viable materials that satisfy selected high-level technical targets; and finally a multi-parameter optimization to recommend top choices in connection with ML algorithms.
Methodology
Application-Driven Architecture
In order to offer scale-bridging capabilities to connect crucial steps in materials design-to-device integration, an application-driven architecture has been introduced and demonstrated (Malek et al., 2019). The central part of this architecture is an embedded master data lake, consisting of large-scale metadata for electrocatalyst materials, which is assembled from various types and sources of materials data. Key technical targets such as activity (i.e., the faradaic efficiency), stability, and selectivity are usually defined at cell and device level and may also correlate differently at low or high temperatures with physicochemical properties of electrocatalysts and the operating conditions at cell or device levels (Chan and Li, 2014; Nitopi et al., 2019).
Figure 1 illustrates the functional layers of the ML-enabled data analytics approach and its underlying workflow. The workflow comprises various layers including user-defined or default data sources and databases, analytics modules, and self-driving algorithms, which are commonly used in materials discovery approaches, regardless of the corresponding field of application. The complexity with scale-up and discovery of integrated materials also implies the need for ad hoc communication among parallel or series of synthesis and characterization steps or equipment, in-device component integration, and device testing or validation. This all-embracing workflow along the complete development pipeline can enhance data communication and promote understanding of correlations among structure, functional properties, and performance indicators at all scales from materials discovery to device performance and optimization.
Master Data Lakes
A vital prerequisite for any type of ML application is the provision of a suitable dataset for a given domain. The search for new electrocatalyst materials essentially needs a minimal and sufficient set of performance indicators from the “chemical domain” and the “property domain” of different electrocatalyst materials (Flores-Leonar et al., 2020).
The master database is built from materials datasets collected from a wide range of sources and user-types, namely 1) unpublished records of academic researchers, 2) published articles, and 3) other public records and industry reports. The details of the data retrieval from images, tables and texts are described in ref Malek et al. (2019). The resulting database is stored in excel or CSV format with predefined and standardized headers that include metadata preprocessing and cleaning.
In this article, the CO2RR experimental databases were generated from literature sources on the basis of seven input variables: electrocatalyst type, faradaic efficiency, applied potential, current density, type of electrolyte, major product, and temperature. Each experimental data point is characterized by a set of performance indicators for catalyst formulation and reaction conditions, either as continuous values (such as current density) or as categorical values (such as catalyst type). The ranges of the corresponding input variables are summarized in Table 1.
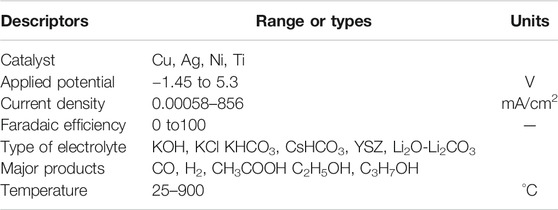
TABLE 1. Key performance indicators and their types or range of values as being set in the data extraction process.
Machine Learning Algorithms
ML classification models could be used to identify and classify materials or map them in terms of their properties (descriptors), which is the first essential requirement prior to any ML-based prediction. We use the Scikit-learn package in the ML modules (Pedregosa et al., 2011).
The ML algorithms employed for classification of electrocatalyst and product type include logistic regression (LR), linear discriminant analysis (LDA), k-nearest neighbors (KNN) classifier, and random forest (RF) classifier. In addition, we tried to classify groups of products by putting all possible products into two or three different larger groups of products. In order to compare the predictability of different models for finding missing data, four ensembles of ML algorithms were evaluated. The regression algorithms include Bagging Regression (BR), Gradient Boosting Regression (GBR), Random Forest Regression (RFR), and Extra Trees Regression (ETR). BR is an ensemble method that fits regressors on random subsets of the original dataset and makes a final decision based on aggregated prediction. The bagging method increases the robustness of the original set of models by introducing randomness during the training process and then ensembling their predictions. GBR builds a model in a forward stage-wize style, which enables optimization on any differentiable loss function. RF is a typical ensemble learning model that operates by building a set of decision trees and yielding average predictions of a separate tree. Random decision forests are superior to decision trees due to the ability to solve the over-fitting issue. Finally, extra trees implement a meta-estimator that fits several random decision trees on different sub-samples of the dataset and utilizes the mean of trees to boost the predictive performance and reduce the variance. ETR and RFR models have shown to be promising in the modeling of chemical systems. Each algorithm was trained on the training data for the CO2 reduction reaction. The algorithms were then implemented to predict faradaic efficiency, applied potential (AP), and current density for the test dataset. We used the ML hyperparameter optimization module to tune hyperparameters automatically.
The accuracy score (%) (i.e., the ratio of correct predictions to the total number of predictions) is used as a performance metric for the evaluation of each classification algorithm. The performance of each ML algorithm for prediction was evaluated by using several statistical indicators such as the mean squared errors (MSE), the root mean squared error (RMSE), and the coefficient of determination (R2),
in which
Modular Design
The complexity of the materials design-to-device integration calls upon a modular approach, in which various data management tasks and data analytics tools are built and tested in isolation, as stand-alone-modules. The suitable modules are then called and integrated into the main platform depending upon the application area, required analysis tools, and type of meta-data that the user needs for the analysis. In the following, we describe the adaptation of each module and their inter-dependencies for the analysis of electrocatalytic materials for the CO2RR.
Classification and Materials Data Extraction
This module utilizes a classification algorithm that categorizes catalyst materials in the form of performance range (e.g., potential or current density) or selectivity or type of products. The reference values for high-level technical targets are based on a “performance matrix” that is provided as the default for a particular field of application or as a user-entry table for the target values. These initial values can be seen as the first set of keywords for data mining and data discovery from the literature for a given material application field or sub-classes therein such as low-temperature catalysts or high-temperature catalysts. The extracted data is then mapped on these key technical parameters and other crucial measurement conditions for each class of materials.
Materials Property Prediction
This module can predict a specific electrocatalytic property such as the faradaic efficiency as a function of input or exploratory variables using embedded ML models. The results of these ML prediction models can refine the usefulness and relevance of the user input variables. The module also helps fill missing data points related to performance indicators or target properties in the database and thus enriches the master database. In this context, electrode type, current density, voltage, polarization resistance, conductivity, electrolyte type and composition, temperature, type of product, and (rarely) faradaic efficiency are among the key factors that can influence CO2RR performance.
Recommendation System and Decision Models
The performance tuning algorithm is the first layer of the recommendation module that uses the complete dataset to find the best electrocatalyst material based on performance and stability metrics’ target values. It displays the information using standard visualization tools, for example, using a radar chart. A radar chart is a typical visualization tool employed in benchmarking electrocatalyst materials for the purpose of quality and performance improvement of a system of materials or an electrochemical device (Basu, 2004). The use of radar charts makes two significant contributions: first, it provides a simple 2D visual representation of multiple performance indicators without the need of using dimensionality reduction on multivariate data; second, the enclosed area, formed by spikes (or axes), can be considered as an intuitive electrocatalyst performance indicator.
The ML-powered recommendation module uses the power of regression modeling to predict values for the missing data as accurately as possible. Supplementary Table S1 shows the sample data statistics used to train the regression models for predicting the missing data, specifically for applied potential, current density, and faradaic efficiency. Datapoints for four types of electrocatalyst material were selected, as there was not enough data for predicting other variables in the CO2 experimental database.
Data Matching and Validation
The ultimate criterion for ML-based predictive capabilities is experimental validation, which demonstrates how computer algorithms lead to real discoveries. After predicting the best candidate electrocatalyst material, the prediction can be validated by direct comparison to experimental data for the same or almost the same set of conditions and materials specifications (Malek et al., 2019).
In our predictive algorithm, CO2 electrocatalyst materials are generally categorized into three main groups: metallic, non-metallic, and molecular catalysts. Each category of electrocatalyst materials exhibits distinct physicochemical and electrocatalytic properties. Therefore, it is possible that the performance of an electrocatalyst material is restricted and limited to the group of catalyst materials it belongs to. Here, we used ML classification models to sort different electrocatalyst materials into different groups based on their performance. The numerical data are normalized between 0 and 1, and we encoded the categorical data using “OneHotEncoder” from the Scikit-learn data preprocessing module (Pedregosa et al., 2011).
Most of the data in our Master database at low temperature are for Cu electrocatalyst, with the key properties of AP, CD, and FE, type of electrolyte, and type of product. Material properties predictions thus focus on these attributes.
Results and Discussion
Materials Recommendation
Figure 2 shows the workflow of material recognition. In order to identify an electrocatalyst material for a given electrochemical process, it is expected that the performance metrics of the chosen electrocatalyst meet or exceed the target values set by the user. For this purpose, one needs to consider the key performance metrics, i.e., faradaic efficiency, current density, applied potential, selectivity, and production rate, to select the best electrocatalyst material.
In practice, keeping track of all variables and establishing correlations among optimization parameters in an electrochemical reaction path is a difficult task; once a set of properties is set to the optimum values, other properties of the catalyst can have values which are below user requirements. We attempt to address this challenge by introducing a penalty function for any value less than the desired value for a target application variable.
The recommendation process shown in Figure 2 initially takes input from the user-specified target values. The recommendation then selects the “best” electrocatalyst or recommends electrocatalyst materials primarily based on the targets for the set of performance metrics defined by the user. Global target values are provided as default if no user-entry target values are available. In order to minimize the optimization effort and for fast and better identification criteria, the user is provided with one of the following identification schemes: 1) find any electrocatalyst material for some desired value of a metric, with any chemical product; 2) find any electrocatalyst material for target value metrics for a specific set of chemical products; 3) find some desired performance metrics, within specific electrocatalyst material groups, with any product; 4) find some desired properties within specific electrocatalyst material groups, for a certain set of chemical products. The user is given target values for selected metrics, electrocatalyst type, and chemical products, where a user is able to filter data based on products and electrocatalyst material or simply select all the possibilities. If the user provides target values for all metrics, the recommendation algorithm selects an electrocatalyst material with properties equal (with less than 10% deviation) or better than the user target. If the user provides target values for a few properties and not all the properties, then the algorithm uses default global target values for those target properties that are not provided by the user.
Here, a simplified, yet straightforward method for selecting an electrocatalyst material is employed by using a radar chart to identify the material, which encloses the graph’s maximum area. Although this heuristic method can be seen as practically useful, it may lead to a biased selection with few performance indicators at high values, while others remain at low values. It ignores the ranking and importance of different variables.
Our optimization algorithm employs a special scoring factor where it scores positive values for properties that are higher or equal to the user target values and penalize properties that are less than the user target values. The value of the penalty function becomes more valuable for performance indicators that significantly less than the actual target values. This sub-routine recommends catalyst materials that exhibit high values in one or multiple attributes from the performance matrix table.
The scoring factor is defined by,
where k is the number of target properties (P). If
If
Here
The constraint for the penalty function is set at 5, representing the maximum error tolerated. Once the scoring factors for each row in the database are calculated, the algorithm recommends electrocatalyst materials with high score values, as illustrated in Supplementary Figure S1.
Low-Temperature Electrocatalyst Materials
Figure 3 shows the visualization of data, which is distributed among applied potential, current density, and faradaic efficiency for different types of electrocatalysts at low- or high-temperature. The diagonal graphs represent the density plot of each respective feature, providing useful information by giving a density of plots in the form of bar charts. Among the possible choices of electrocatalysts at low temperature, mainly four types of Cu-based electrocatalysts are used for the classification task. The dataset is divided into training and test datasets. The dataset consists of 228 different Cu electrocatalyst materials, among which training and test datasets account for 183 and 45 data points, respectively. Each data point consists of a set of properties for a given material. The same material may appear in different data points with different operating conditions. The materials space is then scanned using a set of descriptors, such as selectivity for a given product or performance indicators against a reference target range. The latter is performed using machine learning techniques. Model performance for classification of the type of electrocatalyst and type of products was evaluated through the calculation of an accuracy score.
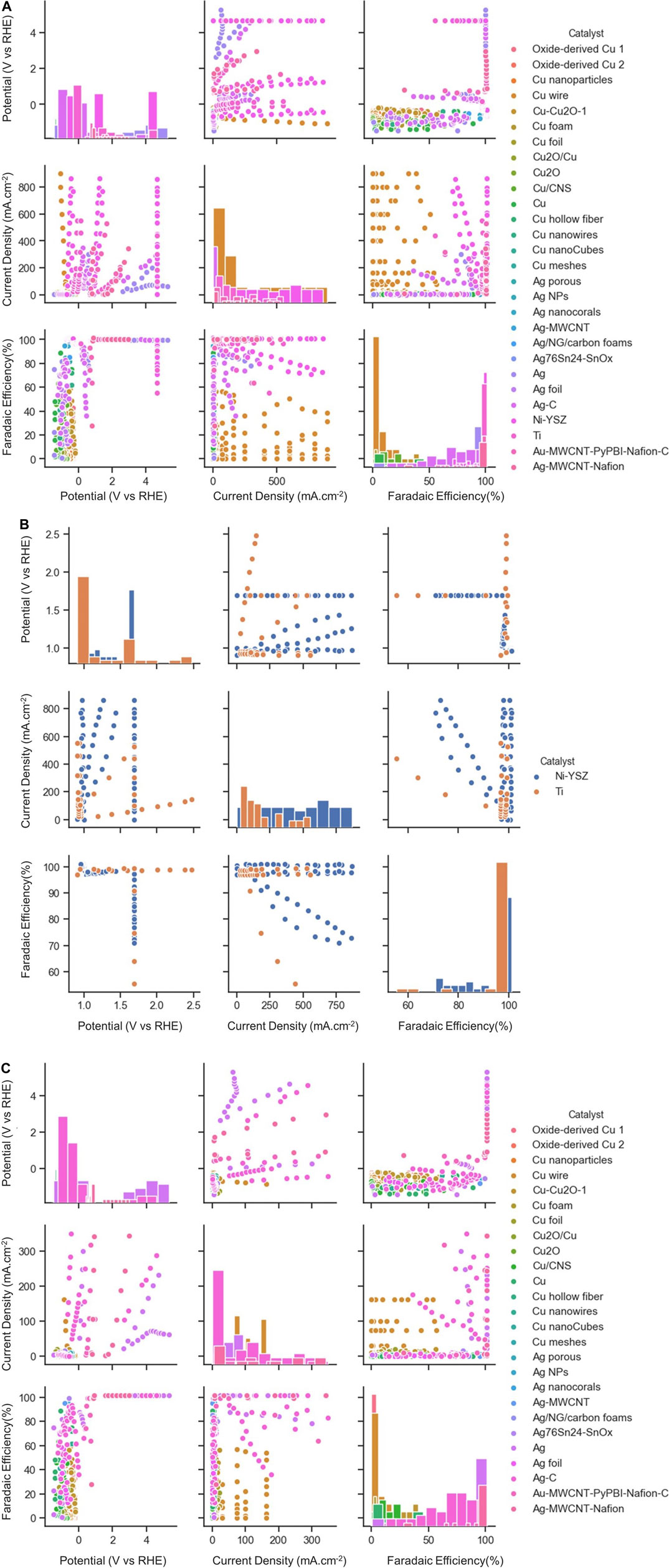
FIGURE 3. Scatter plot matrix showing the data distribution for (A) both High-T and low-T (B) High-T, (C) Low-T of CO2RR according to three performance metrics. The elements in the diagonal (upper left to lower right) represent the respected range of data points for each catalyst type.
As illustrated in Table 2, the key indicators (AP, CD, FE, Product selectivity) have high cross-validation scores, which can vary according to the ML algorithms. The LR and LDA classifiers are found to return the highest accuracy score of 81%, determining the type of electrocatalyst. QDA classifier has an accuracy score of 32%, which is remarkably lower than that for other classifiers.

TABLE 2. The results of cross-validation with six different classification algorithms against low-temperature catalyst types in four classes (Cu wire, Oxide-derived Cu 1, Oxide-derived Cu 2, Cu nanoparticles).
As shown in Table 3, the indicators of AP, CD, FE, and type of electrocatalyst yield a higher accuracy for classification of a group of two products (CH4, C2H5OH) in comparison with two other groups, each consisting of three different products. RF and LDA classifiers return value of 1 and 0.93, respectively, for the accuracy score of all test cases. In general, RF classifier has the best performance among other algorithms for the classification of the type of products regardless of the number of products.

TABLE 3. The results of cross-validation with six different classification algorithms against the type of products in three classes [a group of (CH4, C2H4, C2H5OH) (CH4, C2H5OH, C3H7OH), and (CH4, C2H5OH)].
LR, LDA, QDA, and GNB algorithms were unable to distinguish and single out one group of products, including those with three different products. Additionally, GNB returns an accuracy score of 26%, the lowest of all six algorithms. It is obvious that better performance of ML algorithms can be achieved for the group with two products than for the groups with three different products. The latter can be understood from the comparison of the values of accuracy score for classification of the type of electrocatalyst or products reported in Table 2 and Table 3. One would need more indicators such as the reaction conditions (pH, mass loading of catalyst, production rate, and concentration) for each reaction in order to have a better performance with the classification scheme.
Table 4 lists the performance of predictive analytics using MSE for various experimental numerical values, i.e., AP, CD, and FE. ETR is seen to have a better predictive capability with a minimum error, which is considered more accurate than other algorithms. In order to quantitively obtain a prediction model for FE, AP and CD, we employed the BR, GBR, ETR, and RFR algorithms. Models were based on the training data (80% of the full dataset), where 20% of is used to evaluate the test data.
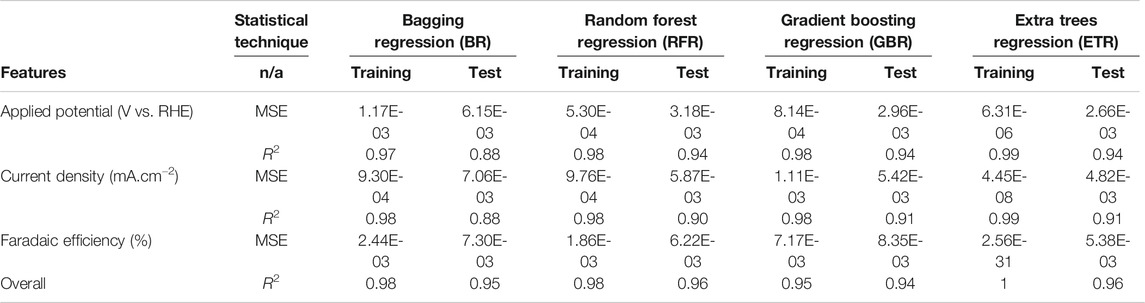
TABLE 4. Evaluation of predictive algorithms for applied potential, faradic efficiency and current density.
The scatter plots of the outputs vs. the actual values for the training, testing, and overall data sets using RFR and ETR algorithms are presented in Figure 4. The coefficient of determination (R2) indicates a strong correlation between outputs for CD and AP and actual values. The AP, CD, and FE results clearly show excellent agreement between the actual values and RFR, GBR, and ETR predictions, with R2 > 0.90 and MSE < 0.008 for all of the ensemble modeling cases. The R2 and MSE of test data for faradaic efficiency with ETR and RFR have better performance than that for other regressors.
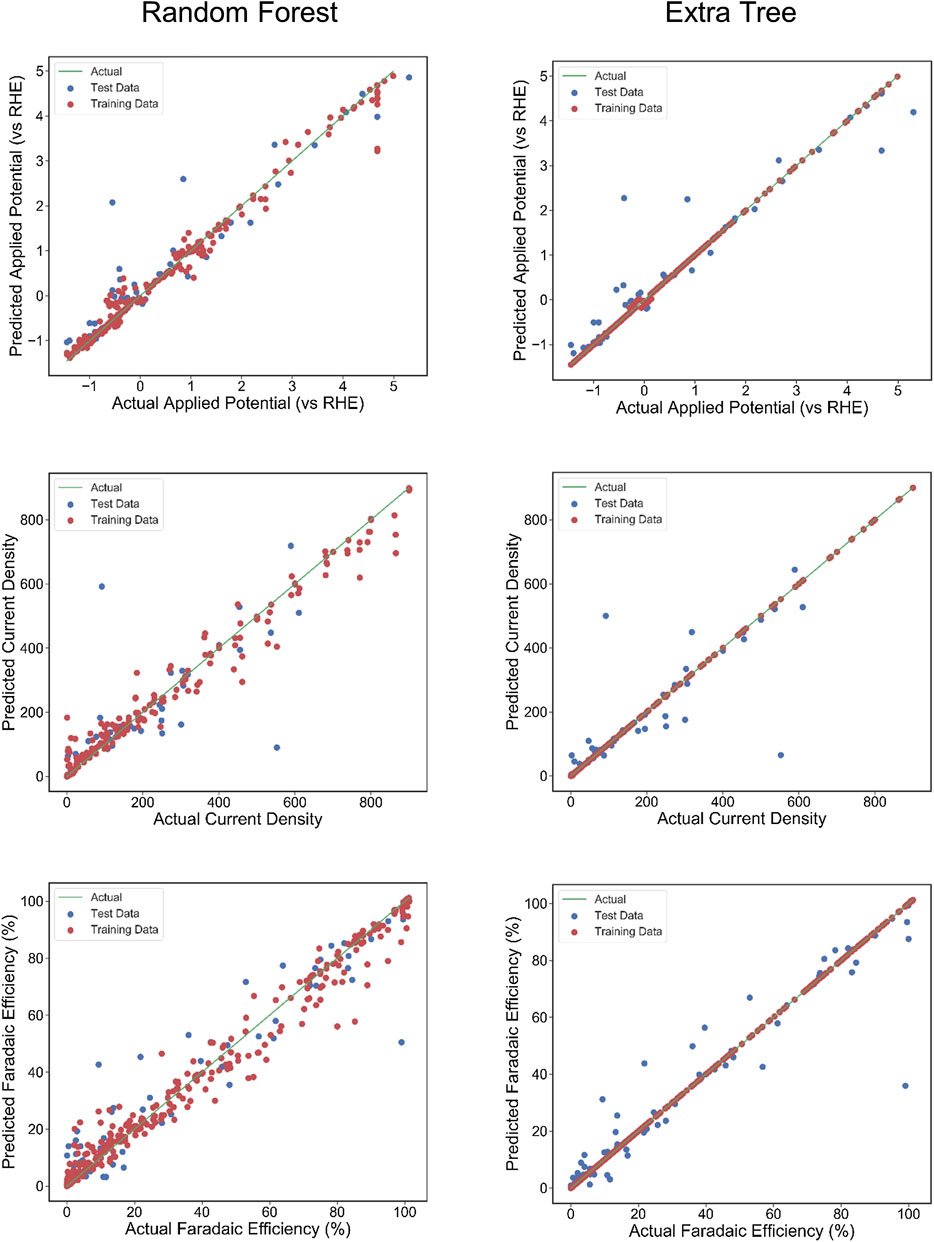
FIGURE 4. The actual Faradaic efficiency, applied potential, and Current density values compared with the predicted values using Random Forest regression and Extra Tree regression models. The coefficient of determination (R2) and mean squared error (MSE) are computed to estimate the prediction errors. The test and training points are shown in blue and red, respectively. The perfect correlation line is included for reference as a green line.
Success with ML depends on the number of descriptors and their correlations, as well as available large training data. The true benefit of structure-property relationships revealed through ML models lies in the multi-variant correlations and their interpretation in terms of the fundamental materials properties.
The missing values in the primary database can nonetheless be filled with values extrapolated from ML by building a model that relates known indicators of materials to target properties. Our ML model has successfully predicted different properties like FE or CD, or classification of the type of electrocatalyst, or major products related to specific type of catalyst. The latter process has been carried out iteratively. After filling missing values, the database is ready to screen the electrocatalyst performance through means of analytical and visualization tools.
Utilizing all available and supplemented databases, rapid screening of electrocatalyst materials was carried out, while the user would be able to specify target values for various properties. The optimization algorithm proposed in this work uses a scoring factor based on a rank-ordering approach. The best electrocatalyst material for selected chemical products was then estimated for a class of materials or products. Figure 5 shows the radar charts of the best electrocatalyst materials based on the target attributes selected by users or directly from a global target, which is set as a default. The figure indicates that Pt is the catalyst of choice when no specific fuel products are considered.
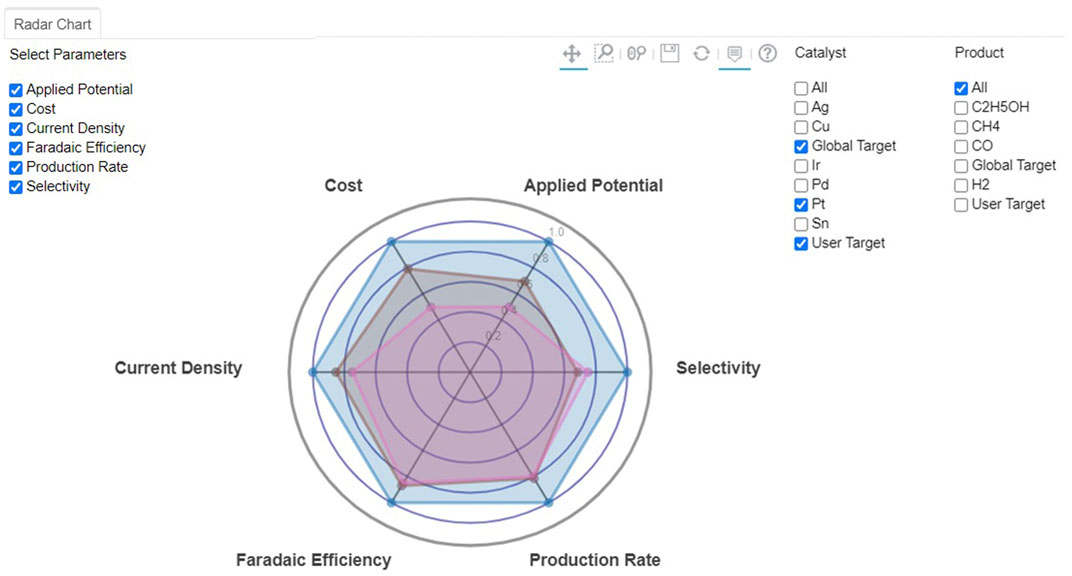
FIGURE 5. Screenshot of radar chart for CO2 reduction to fuels of Pt-based on different classification of electrocatalysts and selected target values by default global (blue) or user-entry (brown) targets.
High-Temperature Electrocatalyst Materials
Despite recent advances in electrolytic systems for CO2 conversion at high temperature (>800°C), the overall efficiency and performance of the system remain far from being sufficiently understood for commercialization and practical usage (Gorte et al., 2000). Among the technological shortcomings are low conversion efficiency and high degradation rates of materials and components, including membrane and electrocatalysts. The latter is mainly due to the fact that the high catalytic conversion will inherently result in low electrochemical stability of catalyst materials at higher temperatures. The fundamental understanding of the elementary kinetic processes involved in CO2 electrochemical conversion at high temperature is a subject of ongoing research (Tran and Ulissi, 2018). Notably, the cost-effectiveness of catalytic processes at high temperature primarily depends upon the trade-off between the system efficiency and production cost of the fuel, while the operating condition of the solid oxide electrolyzer cells (SOECs) remains very narrow due to high heat requirements and the sensitivity to temperature fluctuations (Ma et al., 2015). CO is the major product as all other competing chemical reaction products are desorbed from the surface to produce CO at high temperatures. Therefore, additional down-stream processes need to be performed in order to achieve other products such as methanol. For co-electrolysis of CO2 and H2O, SOEC provides high flexibility in the carbon to hydrogen ratio (C/H) and, thus, state-of-the-art technologies such as Fischer Tropsch (FT) synthesis can be utilized downstream for achieving high product flexibility (Zhang et al., 2017; Zheng et al., 2017).
Here, we present preliminary results and a discussion for a data-driven analysis of selected electrocatalyst systems in SOECs that address a few of the above technological challenges. In high-temperature electrolysis of CO2, the co-electrolysis process in the presence of steam is taking place at temperatures >600°C. High-temperature CO2 electrochemical conversion using SOEC generally has a better selectivity compared to that at low temperatures. Correlations among AP, CD and FE at low or high temperature are not known yet and require extensive data analytics.
State-of-the-art high-temperature electrocatalyst materials in SOECs contain Ni-YSZ. A key factor for the stability and activity of these materials at high-temperatures is Ni% in the range of 40–60%. This range is required to fulfill the catalytic reforming and satisfies the matching requirement of the thermal expansion coefficients of the catalyst layer and the YSZ electrolyte (Gorte et al., 2000). Similar to solid oxide fuel cell (SOFC) electrodes, electrocatalytic reactions in SOECs take place at the triple phase boundary (TPB) where the Ni phase provides electrons, and YSZ particles offer the required oxygen ion vacancies for the reduction of adsorbed CO2 and the removal of oxygen ions, respectively.
Recent progress suggests that the electrochemical reduction of CO2 in solid oxide electrolysis cells takes place at high current densities. Degradation rates are higher in electrolysis mode compared to those in fuel cell mode based on enhanced effects of metal particle migration and/or oxidation, carbon deposition, grain coarsening, and contamination by impurities. This adds complexity to the choice of electrocatalyst materials and, thus drives significant research activity. In particular, electrochemical reduction of CO2 in the temperature range of 573–873 K is worth exploring in order to match the temperature levels of electrolysis with required downstream FT-processes; however, there are no proper material systems for electrodes and electrolytes in that temperature regime at the current stage.
Here we consider a few conventional classes of electrode materials and explore the impact of Ni or Ti addition in various proportions on the overall catalytic activity via extensive data analytics. Figure 3B provides scatter plots and distributed values for applied potential, current density, and faradaic efficiency for Ti and Ni-YSZ catalyst systems. Ti-based electrocatalyst exhibits different dependencies for applied potential and faradaic efficiency compared to that for the Ni-YSZ system, while both catalyst materials are relatively similar in view of current densities. Overall, the Ti-based catalytic system shows high correlations among FE and CD, in particular in the range of data obtained at higher applied potentials (>2 V). Figures 3A,B clearly reveal differences in the correlations among key attributes such as FE and AP for catalysts at low and high temperatures. The correlations are more pronounced among FE and AP for high-temperature electrocatalysts, whereas CD and AP are the main indicators at low temperature. Among all electrocatalyst materials studied at high temperatures, Ni-YSZ shows the highest correlation between FE and AP, although the correlation factors can vary depending upon Ni ratios and type of electrolytes or products, as illustrated in the binary correlations in Figure 6.
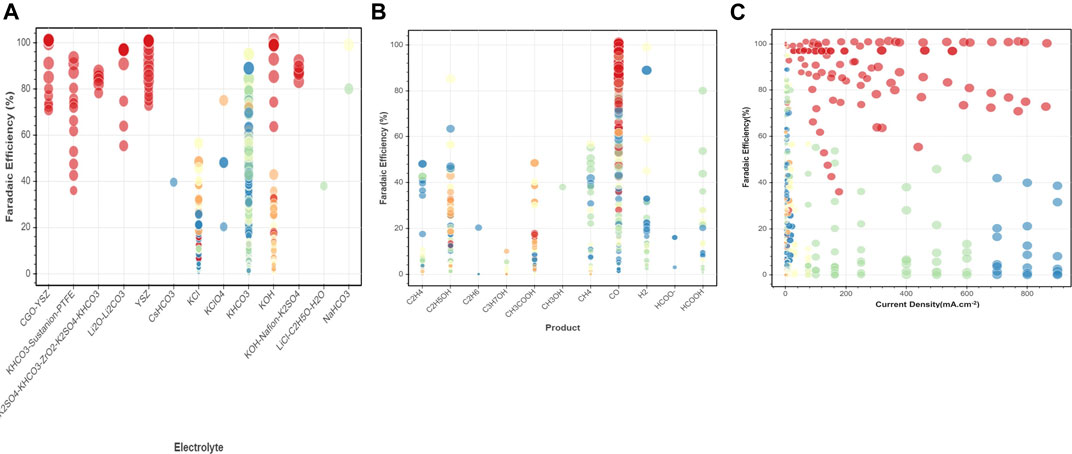
FIGURE 6. Binary correlations among key attributes (A) FE-electrolyte, (B) FE-product, (C) FE-current density for sample extracted datasets of electrocatalyst materials at low and high temperature.
The dataset for high-temperature catalysts consists of 180 test data points distributed among five different catalyst types. This amount of data is insufficient for accurate prediction of missing properties in the data set, and thus further predictions using ML techniques and identification thereof are not feasible based on the existing size of the dataset. Moreover, the atomic ratios of the composite electrocatalysts are not taken into consideration in these databases. The current results, however, will be expanded in the future to generate further insights for the correlation of key attributes at high-temperatures using larger and more diverse training and test data sets.
Recommendation and Decision System
Here, we only focused on high-level correlations among selected indicators. Supplementary Table S2 provides the complete test data and other operational conditions that are assumed for each data point. The type of electrolyte is another important factor to be considered as it influences the extend of correlations among FE and AP for various high-temperature electrolysis technologies and the respective electrocatalysts. In particular, future work can include the analysis for the following use cases and comparison based on phase ratios and catalyst types such as Ag, Ni|YSZ or Ag|YSZ and for at least one cell configuration such as Ag/GDC|YSZ|YSZ/LSM|LSM [La 0.8 Sr 0.2 Cr 0.5 Mn 0.5 O 3 −δ(LSCM)]. Further analysis is still ongoing to improve the test and training databases for high-temperature catalysts and provide a robust recommendation framework for this system. Here, the analysis is primarily built upon existing and extracted historical data. There is an emerging need for employing sophisticated decision algorithms and recommendation systems to “close-the-loop.” Such algorithms will emerge from predictive models of key materials properties under different experimental conditions or modeling assumptions. They also identify weighting factors that govern specifications and limitations imposed at the components and device-level. Such algorithms are trained over time as more historical data and use cases become available.
Conclusion
The discovery and optimization of electrocatalyst materials are driven in large part by collecting and analyzing experimental data. The ML-assisted development of electrocatalysts is still an emerging field despite its success in molecular and material science; it cannot yet lead directly to novel electrocatalyst materials.
In this article, we proposed a recommendation framework for the benchmarking of existing electrocatalyst materials. A multi-attribute decision process was adopted, which was mapped on radar charts, from which the analysis of best-performed electrocatalyst is carried out based on user-entry or global technological targets. This recommendation framework provides the choice of dimensions, indicators, and appropriate correlations for benchmarking purposes and materials screenings process, purely based on historical data. With the availability of reliable process and materials economic data, the latter can lead to comprehensive techno-economic insights into what performance levels are required for commercially viable electrocatalyst materials for the use in electrochemical energy conversion and storage devices.
We used ML to supplement missing data in CO2RR databases prior to deploying ML algorithms to identify the best catalytic system. The ML module is primarily built for the classification and prediction of electrocatalyst materials. Different models for classification of the type of electrocatalyst materials and chemical products are used with reasonable accuracy within the limit of available test and training data. Among different regression algorithms, the Random Forest model showed a better capability for the prediction of electrochemical attributes. The proposed recommendation system provides interactive visual analysis of different indicators for the exploration of uploaded electrocatalyst data. High-level correlation analytics was also provided for catalyst materials at high temperatures, and the intensity of correlations was compared to that for catalyst materials at low temperature.
Finally, rapid screening and benchmarking studies of electrocatalysts material via data-driven visualization can significantly reduce the discovery time for the best materials and to understand or compare vital performance trends and correlations for given classes of materials, from initial discovery to component or device integration and for full-scale component or device production. The major limitations of the framework presented here are the incompleteness of datapoints, un-clarity or lack of consistency around key numerical or categorical attributes, and missing values for the attributes that are collected from the literature. The framework, however, can be applied to other sustainable electrochemical processes such as electrochemical NH3 synthesis through N2 and H2O electrolysis.
The interactive visualization tools assist researchers in discovering trends and patterns hidden with the electrocatalyst material based on historical experimental and modeling data. Further ML and analytics functionalities are currently under development, which will offer higher accuracy and better inter-operability of the recommendation framework for idea-creation and the screening of electrocatalyst materials for various applications.
Data Availability Statement
The original underlying data presented in the study are included in the article/Supplementary Material, further inquiries for access to Github can be directed to the corresponding author.
Author Contributions
All authors contributed to the writing and editing of this manuscript. KM and ME contributed equally to the design of the initial concept and implementation of the research method. AM, KM, and ME collectively led and designed the underlying data analytics concept and ML methodology, and backbone of the data visualization and analysis tools.
Funding
This work was supported by the German-NRC collaboration project.
Conflict of Interest
The authors declare that the research was conducted in the absence of any commercial or financial relationships that could be construed as a potential conflict of interest.
Acknowledgments
KM and QW would like to thank NRC international office and NRC’s Materials for Fuel Challenge program for their financial support. The authors also greatly acknowledge researchers in IEK-13 and IEK-1 at the Forschungszentrum Jülich for valuable insights and contributions to this project. Contribution from Gagandeep Singh Bajwa at NRC-EME for the extraction, cleaning, and analysis of CO2RR databases is greatly acknowledged. ME acknowledges the support from the Forschungszentrum Jülich GmbH.
Supplementary Material
The Supplementary Material for this article can be found online at: https://www.frontiersin.org/articles/10.3389/fenrg.2021.609070/full#supplementary-material.
References
Al-Ghussain, L. (2019). Global warming: review on driving forces and mitigation. Environ. Prog. Sustain. Energ. 38, 13–21. doi:10.1002/ep.13041
Basu, R. (2004). Implementing quality: a practical guide to tools and techniques: enabling the power of operational excellence. Boston, MA: Cengage Learning EMEA.
Cao, B., Adutwum, L. A., Oliynyk, A. O., Luber, E. J., Olsen, B. C., Mar, A., et al. (2018). How to optimize materials and devices via design of experiments and machine learning: demonstration using organic photovoltaics. ACS Nano 12, 7434–7444. doi:10.1021/acsnano.8b04726
K.-Y. Chan, and C.-Y. V. Li (2014). Electrochemically enabled sustainability: devices, materials and mechanisms for energy conversion. Boca Raton, FL: CRC Press.
Chou, T.-C., Chang, C.-C., Yu, H.-L., Yu, W.-Y., Dong, C.-L., Velasco-Vélez, J.-J., et al. (2020). Controlling the oxidation state of the Cu electrode and reaction intermediates for electrochemical CO2 reduction to ethylene. J. Am. Chem. Soc. 142, 2857–2867. doi:10.1021/jacs.9b11126
Citrine Informatics (2013). Available at: https://citrination.com (Accessed March 10, 2021).
De Luna, P., Wei, J., Bengio, Y., Aspuru-Guzik, A., and Sargent, E. (2017). Use machine learning to find energy materials. Nature 552, 23–27. doi:10.1038/d41586-017-07820-6
Draxl, C., and Scheffler, M. (2019). The NOMAD laboratory: from data sharing to artificial intelligence. J. Phys. Mater. 2, 036001. doi:10.1088/2515-7639/ab13bb
Elouarzaki, K., Kannan, V., Jose, V., Sabharwal, H. S., and Lee, J. M. (2019). Recent trends, benchmarking, and challenges of electrochemical reduction of CO2 by molecular catalysts. Adv. Energ. Mater. 9, 1900090. doi:10.1002/aenm.201900090
Flores-Leonar, M. M., Mejía-Mendoza, L. M., Aguilar-Granda, A., Sanchez-Lengeling, B., Tribukait, H., Amador-Bedolla, C., et al. (2020). Materials acceleration platforms: on the way to autonomous experimentation. Curr. Opin. Green Sustain. Chem. 25, 100370. doi:10.1016/j.cogsc.2020.100370
Garza, A. J., Bell, A. T., and Head-Gordon, M. (2018). Mechanism of CO2 reduction at copper surfaces: pathways to C2 products. ACS Catal. 8, 1490–1499. doi:10.1021/acscatal.7b03477
Goldsmith, B. R., Esterhuizen, J., Liu, J. X., Bartel, C. J., and Sutton, C. (2018). Machine learning for heterogeneous catalyst design and discovery. AIChe J. 64 (7), 2311–2323. doi:10.1002/aic.16198
Gorte, R. J., Park, S., Vohs, J. M., and Wang, C. (2000). Anodes for direct oxidation of dry hydrocarbons in a solid-oxide fuel cell. Adv. Mater. 12, 1465–1469. doi:10.1002/1521-4095(200010)12:19<1465::aid-adma1465>3.0.co;2-9
Goyal, A., Marcandalli, G., Mints, V. A., and Koper, M. T. M. (2020). Competition between CO2 reduction and hydrogen evolution on a gold electrode under well-defined mass transport conditions. J. Am. Chem. Soc. 142, 4154–4161. doi:10.1021/jacs.9b10061
Gusarov, S., Stoyanov, S. R., and Siahrostami, S. (2020). Development of fukui function based descriptors for a machine learning study of CO2 reduction. J. Phys. Chem. C 124, 10079–10084. doi:10.1021/acs.jpcc.0c03101
Hummelshøj, J. S., Abild‐Pedersen, F., Studt, F., Bligaard, T., and Nørskov, J. K. (2012). CatApp: a web application for surface chemistry and heterogeneous catalysis. Angew. Chem. Int. Ed. 51, 272–274. doi:10.1002/anie.201107947
Jain, A., Ong, S. P., Hautier, G., Chen, W., Richards, W. D., Dacek, S., et al. (2013). Commentary: the materials project: a materials genome approach to accelerating materials innovation. APL Mater. 1, 011002. doi:10.1063/1.4812323
Ju, H., Kaur, G., Kulkarni, A. P., and Giddey, S. (2019). Challenges and trends in developing technology for electrochemically reducing CO2 in solid polymer electrolyte membrane reactors. J. CO2 Utilization 32, 178–186. doi:10.1016/j.jcou.2019.04.003
Kibria, M. G., Edwards, J. P., Gabardo, C. M., Dinh, C. T., Seifitokaldani, A., Sinton, D., et al. (2019). Electrochemical CO2 reduction into chemical feedstocks: from mechanistic electrocatalysis models to system design. Adv. Mater. 31, 1807166. doi:10.1002/adma.201807166
Kitchin, J. R. (2018). Machine learning in catalysis. Nat. Catal. 1, 230–232. doi:10.1038/s41929-018-0056-y
Lamoureux, P. S., Winther, K. T., Torres, J. a. G., Streibel, V., Zhao, M., Bajdich, M., et al. (2019). Machine learning for computational heterogeneous catalysis. ChemCatChem 11 (16), 3581–3601. doi:10.1002/cctc.201900595
Larsen, A. H., Mortensen, J. J., Blomqvist, J., Castelli, I. E., Christensen, R., Dułak, M., et al. (2017). The atomic simulation environment—a Python library for working with atoms. J. Phys. Condens. Matter 29, 273002. doi:10.1088/1361-648X/aa680e
Lin, R., Guo, J., Li, X., Patel, P., and Seifitokaldani, A. (2020). Electrochemical reactors for CO2 conversion. Catalysts 10, 473. doi:10.3390/catal10050473
Liu, X., Xiao, J., Peng, H., Hong, X., Chan, K., and Nørskov, J. K. (2017). Understanding trends in electrochemical carbon dioxide reduction rates. Nat. Commun. 8, 1–7. doi:10.1038/ncomms15438
Liu, Y., Leung, K. Y., Michaud, S. E., Soucy, T. L., and Mccrory, C. C. L. (2019). Controlled substrate transport to electrocatalyst active sites for enhanced selectivity in the carbon dioxide reduction reaction. Comments Inorg. Chem. 39, 242–269. doi:10.1080/02603594.2019.1628025
Lu, Q., and Jiao, F. (2016). Electrochemical CO2 reduction: electrocatalyst, reaction mechanism, and process engineering. Nano Energy 29, 439–456. doi:10.1016/j.nanoen.2016.04.009
Ma, X., Li, Z., Achenie, L. E. K., and Xin, H. (2015). Machine-learning-augmented chemisorption model for CO2 electroreduction catalyst screening. J. Phys. Chem. Lett. 6, 3528–3533. doi:10.1021/acs.jpclett.5b01660
Malek, A., Eslamibidgoli, M. J., Mokhtari, M., Wang, Q., Eikerling, M. H., and Malek, K. (2019). Virtual materials intelligence for design and discovery of advanced electrocatalysts. Chemphyschem. 20, 2946–2955. doi:10.1002/cphc.201900570
Mandal, M. (2020). CO2 electroreduction to multicarbon products. ChemElectroChem. 7 (18), 3712–3715.doi:10.1002/celc.202000798
Mathew, K., Montoya, J. H., Faghaninia, A., Dwarakanath, S., Aykol, M., Tang, H., et al. (2017). Atomate: a high-level interface to generate, execute, and analyze computational materials science workflows. Comput. Mater. Sci. 139, 140–152. doi:10.1016/j.commatsci.2017.07.030
Meyer, B., Sawatlon, B., Heinen, S., Von Lilienfeld, O. A., and Corminboeuf, C. (2018). Machine learning meets volcano plots: computational discovery of cross-coupling catalysts. Chem. Sci. 9, 7069–7077. doi:10.1039/c8sc01949e
Nitopi, S., Bertheussen, E., Scott, S. B., Liu, X., Engstfeld, A. K., Horch, S., et al. (2019). Progress and perspectives of electrochemical CO2 reduction on copper in aqueous electrolyte. Chem. Rev. 119, 7610–7672. doi:10.1021/acs.chemrev.8b00705
Pedregosa, F., Varoquaux, G., Gramfort, A., Michel, V., Thirion, B., Grisel, O., et al. (2011). Scikit-learn: machine learning in Python. J. Machine Learn. Res. 12, 2825–2830. doi:10.5555/1953048.2078195
Pizzi, G., Cepellotti, A., Sabatini, R., Marzari, N., and Kozinsky, B. (2016). AiiDA: automated interactive infrastructure and database for computational science. Comput. Mater. Sci. 111, 218–230. doi:10.1016/j.commatsci.2015.09.013
Qiao, J., Liu, Y., Hong, F., and Zhang, J. (2014). A review of catalysts for the electroreduction of carbon dioxide to produce low-carbon fuels. Chem. Soc. Rev. 43, 631–675. doi:10.1039/c3cs60323g
Rogelj, J., Schaeffer, M., and Hare, B. (2015). Timetables for zero emissions and 2050 emissions reductions: state of the science for the ADP agreement. Berlin, Germany: Climate Analytics.
Schleder, G. R., Padilha, A. C., Acosta, C. M., Costa, M., and Fazzio, A. (2019). From DFT to machine learning: recent approaches to materials science–a review. J. Phys. Mater. 2, 032001. doi:10.1088/2515-7639/ab084b
Schlexer Lamoureux, P., Winther, K. T., Garrido Torres, J. A., Streibel, V., Zhao, M., Bajdich, M., et al. (2019). Machine learning for computational heterogeneous catalysis. ChemCatChem. 11, 3581–3601. doi:10.1002/cctc.201900595
Smith, A., Keane, A., Dumesic, J. A., Huber, G. W., and Zavala, V. M. (2020). A machine learning framework for the analysis and prediction of catalytic activity from experimental data. Appl. Catal. B: Environ. 263, 118257. doi:10.1016/j.apcatb.2019.118257
Tran, K., and Ulissi, Z. W. (2018). Active learning across intermetallics to guide discovery of electrocatalysts for CO2 reduction and H2 evolution. Nat. Catal. 1, 696–703. doi:10.1038/s41929-018-0142-1
Varnek, A., Kireeva, N., Tetko, I. V., Baskin, I. I., and Solov’ev, V. P. (2007). Exhaustive QSPR studies of a large diverse set of ionic liquids: how accurately can we predict melting points? J. Chem. Inf. Model. 47, 1111–1122. doi:10.1021/ci600493x
Wang, Y., Liu, J., Wang, Y., Al-Enizi, A. M., and Zheng, G. (2017). Tuning of CO2 Reduction selectivity on metal electrocatalysts. Small 13, 1701809. doi:10.1002/smll.201701809
Zahrt, A. F., Henle, J. J., Rose, B. T., Wang, Y., Darrow, W. T., and Denmark, S. E. (2019). Prediction of higher-selectivity catalysts by computer-driven workflow and machine learning. Science 363, eaau5631. doi:10.1126/science.aau5631
Zhang, X., Song, Y., Wang, G., and Bao, X. (2017). Co-electrolysis of CO2 and H2O in high-temperature solid oxide electrolysis cells: recent advance in cathodes. J. Energ. Chem. 26, 839–853. doi:10.1016/j.jechem.2017.07.003
Zheng, Y., Wang, J., Yu, B., Zhang, W., Chen, J., Qiao, J., et al. (2017). A review of high temperature co-electrolysis of H2O and CO2 to produce sustainable fuels using solid oxide electrolysis cells (SOECs): advanced materials and technology. Chem. Soc. Rev. 46, 1427–1463. doi:10.1039/c6cs00403b
Keywords: CO2 reduction, high and low temperature, machine learning, artificial intelligence, materials discovery, data analytics, classification
Citation: Malek A, Wang Q, Baumann S, Guillon O, Eikerling M and Malek K (2021) A Data-Driven Framework for the Accelerated Discovery of CO2 Reduction Electrocatalysts. Front. Energy Res. 9:609070. doi: 10.3389/fenrg.2021.609070
Received: 22 September 2020; Accepted: 29 January 2021;
Published: 13 April 2021.
Edited by:
Kai S. Exner, Sofia University, BulgariaReviewed by:
Guangfeng Wei, Tongji University, ChinaVenkatasubramanian Viswanathan, Carnegie Mellon University, United States
Copyright © 2021 Malek, Wang, Baumann, Guillon, Eikerling and Malek. This is an open-access article distributed under the terms of the Creative Commons Attribution License (CC BY). The use, distribution or reproduction in other forums is permitted, provided the original author(s) and the copyright owner(s) are credited and that the original publication in this journal is cited, in accordance with accepted academic practice. No use, distribution or reproduction is permitted which does not comply with these terms.
*Correspondence: Kourosh Malek, koroush.malek@nrc-cnrc.gc.ca