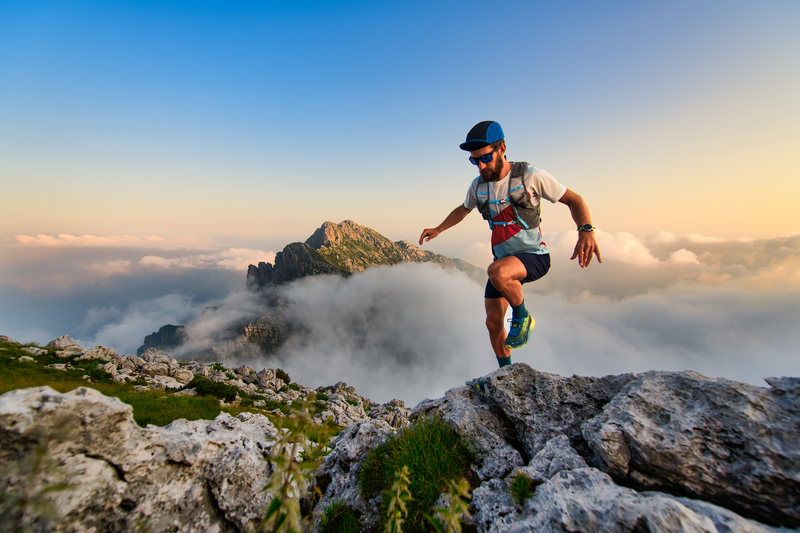
95% of researchers rate our articles as excellent or good
Learn more about the work of our research integrity team to safeguard the quality of each article we publish.
Find out more
ORIGINAL RESEARCH article
Front. Energy Res. , 09 July 2021
Sec. Sustainable Energy Systems
Volume 9 - 2021 | https://doi.org/10.3389/fenrg.2021.550877
This article is part of the Research Topic Renewable Energies, Storage Systems and Sustainable Mobility for Increasing the Energy Independence of Small Islands and Remote Areas View all 10 articles
Tidal energy is the most reliable and predictable form of renewable energy capable of ensuring energy security in coastal regions of the world. Many developing countries are prone to energy self-sufficiency due to a lack of tidal data, expensive commercial tidal modeling tools, and program codes. In the present study, an open-source finite element code along with available open-source data was used to predict the tidal resource potential of sites in both temperate and coastal waters. This paper also investigates the suitability of open-source code towards accurate tidal resource prediction and provides a comparative study on tidal resource prediction of sites in both temperate and coastal waters. Based on knowledge gained from tidal experts all around the globe, the straits of the Alderney race were selected as a temperate water site because of their high tidal flow conditions and high tidal energy resource potential. Singapore was selected as a tropical water site because of its low tidal flow conditions and lack of open-source tidal resource data in the tropical belt. From the results, temperate waters such as Alderney Race experience high tidal velocity in the range of 3.5–4.5 m/s with an average power density of about 15 kW/m2 in comparison with tropical waters such as Singapore that experiences tidal velocity in the range of 1–1.5 m/s with an average power density of about 1.5 kW/m2. The thrusting force behind the coastal dynamics is mainly due to tides, their interactions, and changes in seabed topography. The seabed roughness profile creates a drag force on the flow on the velocity field. Lack of understanding of the effects of seabed friction on tidal modeling might reduce the accuracy of the model prediction. Thus, the present study also focuses on the effects of seabed roughness on tidal prediction of Alderney race straits using the open-source finite element-based 2-dimensional depth average ocean model. It can be found that an increase in seabed friction reduces the flow velocity and thus the average power density of the location due to its major energy dissipating phenomenon for the energetic ocean flow.
Most of the remote coastal communities in South East Asian (SEA) countries lack electricity supply and, in some areas, they even burn fossil fuels such as diesel to produce electricity. This in turn contributes to the increase in global carbon emission and thereby to climate change and global warming, which also threatens the nation’s sustainable development goals. As per the United Nations Environmental protection agency’s estimation, global carbon emission has increased by about 90% compared to the emissions in 1970. It is also estimated that ASEAN countries and their remote coastal communities’ energy consumption will grow at an average annual rate of 3.9% from 343 MTOE 2005 to 901 MTOE 2030 as per the detailed analysis (Quirapas et al., 2021). But these areas are well surrounded by the ocean which has a huge potential to generate energy. As per International Renewable Energy Agency (Kempener and Newmann, 2014), there is a global tidal resource potential of about 3 TW which can be exploited. Thus, the tidal industry has begun to develop all over the world.
In general, tidal turbines can be classified as floating and seabed mounted tidal turbines. This can be further classified based on the rotational axis as follows:
1. Horizontal axis tidal turbine—which rotates in a horizontal axis with the incoming tidal current. Such tidal turbines have a higher power extraction capability up to 45% of total power available in the water.
2. Vertical axis tidal turbine—which rotates in a vertical axis with the incoming tidal current. Such tidal turbines have a lower power extraction capability up to 25% of total power available in the water.
Tidal device makers, project developers, and other related stakeholders are looking for potential sites for deployment of such tidal systems to harness a considerable amount of power. Since only about 45% of the total power available in the water can be extracted, it is essential to understand the tidal average power density of the site to match make and deploy the tidal turbine systems to harness maximum possible power. Average power density is a function of the cube of the velocity field, the depth-averaged velocity profile, and the density of water. This can be predicted mainly through tidal resource modeling simulations using accurate input data. Though there are various hydrodynamic models available, only a few are open source and the remaining need to be purchased from the developers which are very expensive. Thus, some of the global tidal experts and stakeholders are not able to afford and access such hydrodynamic models for their evaluation.
Tidal energy studies of Puget Sound were performed using hydrodynamic model Finite Volume Community Ocean Model (FVCOM) embedded with Marine and Hydrokinetic (MHK) module which showcased the three-dimensional methodology for modeling tides (Wang and Yang, 2017). Another approach using Telemac3D, which solves the free-surface RANS equations using a finite element method, coupled with k-ε turbulence model was used to study the tidal energy available in the Alderney Race (Bourgain, 2019). Simon M Waldman from the United Kingdom used the free surface three–dimensional Finite Volume Community Ocean Model (FVCOM) model to predict the effects of tidal energy extraction from the Tanoura, Naru, and Takigawara Straits in Goto Islands, Japan. They estimated that between 24 and 79 MW of power is available, depending on the level of development, from the designated tidal energy zone (Waldman et al., 2017). Wei Bo Chen from Taiwan used an unstructured-grid hydrodynamic model called the Semi-implicit Cross-scale Hydro-science Integrated System Model (SCHISM) to estimate the tidal current power in the coastal waters of Kinmen Island. They estimated a maximum average Tidal Current Power output of 45.51 kW on the Coast of Kinmen Island (Chen et al., 2017). Eduardo González-Gorbeña from Brazil used SisBaHiA, a finite element open model system to estimate the tidal current energy resource in São Marcos Bay. They estimated that a large amount of energy is available at São Marcos Bay with annual power densities ranging from 9.2 to 11.2 MWh/m2 (González-Gorbeña et al., 2015). Carballo et al., 2009 from Spain used the Delft3D-FLOW model, finite difference code that solves the baroclinic Navier–Stokes, and transport equations for accurate estimation of tidal stream energy resources in the Ria de Muros, Spain. They estimated annual energy output of 1,300 MWh and 500 MWh at two sites in the Ria de Muros, Spain (Carballo et al., 2009). Along with three-dimensional approaches, there has been a two-dimensional depth-averaged model which has provided a good correlation to the measurement data. From the knowledge gained from literature as summarized in Table 1, it can be seen most of the tidal resource modeling studies are mainly done for temperate waters and very few works are done on tidal resource modeling studies of tropical waters.
Tidal energy is mainly dissipated due to bottom friction and internal tides breaking (Le Provost et al., 2003). Thus, the effect of seabed friction also plays a major role in tidal energy modeling and prediction. Interactions between tidal energy devices and the marine environment can lead to localized and even system-wide changes in inflow fields and sediment transport (Bourgoin, 2019). The parameterization of bottom friction and surface roughness is typically done as a function of the physical landscape data (Medeiros et al., 2012), which contain some level of measurement uncertainties (Mayo et al., 2014). Also, some coastal areas were subjected to continuous erosion and sedimentation due to frequent storm surges, waves, and winds. These activities likely altered the bathymetry and topography of coastal areas over time. Increased bottom friction may reduce the tidal velocities while the decreased bottom friction increases the tidal velocity of a site. Nicolas Guillou from France used bi-dimensional horizontal (2DH) model TELEMAC 2D of the finite-element modeling system TELEMAC to study the impacts of roughness parameterization of rock outcrops on the seabed of Fromveur Strait off western Brittany. They found out that seabed friction varies the tidal available power prediction by 30% (Guillou and Thiébot, 2016). M.J. Kreitmair from the United Kingdom used theoretical models to study the effects of bottom friction uncertainty towards power potential estimates of tidal currents. They estimated that power uncertainty due to bottom friction is in the range of −5– +30% (Krietmair et al., 2019). Hence, seabed friction plays an important role in tidal energy modeling studies. Based on the knowledge from the literature, it can be seen that there is minimum or a lack of understanding of seabed roughness effects on tidal prediction and this may result in inaccurate predictions.
The main aim of this paper is to elucidate which developing nations, such as South East Asian Countries, have the required power source but are lacking energy measurement and prediction capabilities. This paper would highlight the minimum necessary tidal model using open-source ocean models along with available open-source bathymetry, coastlines, and tidal constituent’s data for accurate tidal resource prediction of both temperate and tropical waters as per IEC TS 62600-201, 2015 standard and European Marine Energy Centre (EMEC) guidelines on tidal resource assessment. In this present work, Advanced Circulation (ADCIRC)- an open-source finite element-based 2D depth average model-was used for tidal potential prediction. This was done to help various areas in the world, especially in tropical countries, to understand and adopt such open-source models for accurate tidal resource prediction of their sites. This present study also investigates the effects of varying seabed friction on the accuracy of tidal resource prediction using the open-source code for a high flow region such as the Alderney race.
Advance Circulation (ADCIRC) is a FORTRAN-based depth-averaged hydrodynamic model. It utilizes the finite element method in space allowing the use of highly flexible, unstructured grids. ADCIRC is a highly developed Ocean model for solving the equations of motion for a moving fluid on a rotating earth. These equations were formulated using the traditional hydrostatic pressure and Boussinesq approximations and were discretized in space using the finite element (FE) method and in time using the finite difference (FD) method. ADCIRC can be run either as a two-dimensional depth-integrated (2DDI) model or as a three-dimensional (3D) model. In either case, elevation is obtained from the solution of the vertically integrated continuity equation in Generalized Wave-Continuity Equation (GWCE) form (Eq. 1). Velocity is obtained from the solution of either the 2DDI or 3D momentum (Eqs 2, 3) (Luettich and Westerink, 2004). All nonlinear terms have been retained in these equations. In this study, we have used open-source models and open-source input data, such as coastline, bathymetry, and Tidal forcing constituents’ data, to help to promote Tidal Energy Modeling in countries that do not have enough information as well as licensed commercial models.
The vertically integrated continuity equation is as follows (Luettich and Westerink, 2004):
where,
U, V are depth-averaged velocities in the x, y directions.
H is total water column thickness.
The vertically integrated momentum equations are as follows (Luettich and Westerink, 2004):
where.
Dx and Dy are Momentum Dispersion.
Mx and My are vertically integrated lateral stress gradient.
Bx and By are vertically integrated baroclinic pressure gradient.
ρo is the reference density of water.
ρ is time and spatially varying density.
τsx, τsy = imposed surface stresses.
τbx, τby = bottom stress components. Ps = atmospheric pressure at the sea surface.
η = Newtonian equilibrium tide potential.
Straits of the Alderney race was selected as the temperate water site for the case study as shown in Figure 1. Due to its location, surrounded by islands and close to the French mainland, straits of the Alderney race experience high tidal velocities. Alderney race has been an area of focus as potential location for tidal energy extraction with an estimated tidal capacity of about 5 GW (Coles et al., 2017). Earlier, Bailly du Bois characterized the flow velocity in the region of the Alderney race to be 5 m/s, making it an ideal location for further investigation (Du Bois et al., 2012) through the open-source code for its comparison with tropical waters.
Singapore coastal waters were selected as the tropical water site for the case study as shown in Figure 2. Singapore, being a tropical nation, is well surrounded by neighboring countries along its border and thereby experiences low tidal velocities. It was estimated that there is a tidal resource potential of up to 250 MW and above just along the Singapore straits (Srikanth, 2016) alone making it an optimum site for further investigation towards comparison with temperate waters.
The selected regions for simulation models include the following:
1. Temperate waters—Alderney race and nearby areas along almost the whole of the English Channel.
2. Tropical waters—Singapore and its associated surrounding islands.
Such a large area was used for simulations so that the influence of most of the flow characteristics could be apprehended. Coastline data for the models were extracted from Global Self-consistent, Hierarchical, and High-Resolution Geography database (GSHHG) which was amalgamated from two datasets: World Vector Shorelines (WVS) and CIA World Data Bank II (WDBII) (Wessel and Smith, 1996). The shorelines are constructed entirely from hierarchically arranged closed polygons. In this study, 1-min arc gridded resolution data was used to create mainland and island boundaries. All the duplicate points in the datasets were removed, loose polygons were processed to closed polygons, and a distinction between land and water polygons was established.
Bathymetry data for the models were obtained from Generic Bathymetry Charts of the Ocean (GEBCO) as 30-second arc gridded data. The GEBCO data set is the gridded bathymetry obtained from a digital bathymetric model of the world ocean floor which has been merged with the land topography (Weatherall et al., 2015). After generating the domain, it was meshed using an unstructured meshing technique to incorporate the complex coastline features of arbitrary shapes (Pal et al., 2018).
The models were forced at the ocean boundaries with eight tidal constituents: K1, K2, M2, N2, O1, P1, Q1, and S2. These tidal constituents were predicted using Leprovost Tidal Database. Leprovost Tidal Database was generated by solving the rotating tidal motion in the sea using equations of motion and equation of continuity (Le Provost and Poncet, 1978). The models used in the study did not consider the effect of meteorological factors that affect the flow to a certain extent. The overall model methodology can be summarized in a flow chart as shown in Figure 3.
Simulations were run for a period of 30 days with a time step of 5 s and a ramp of 5 days for both locations. In numerical simulations of the tide, bottom friction is generally parameterized by the bottom friction coefficient. Based on the knowledge gained from the literature and based on measured experimental data, the seabed friction coefficient varied from 0.0025–0.01 for Alderney race simulations. These friction coefficients were applied constantly throughout the model domain. The seabed friction coefficient was chosen based on the type of seabed soil conditions such as sand, gravel, or rocks, and their properties. Velocity profiles and surface elevation profiles at both locations were obtained from the models and Average Power Density was calculated. Average power density is a function of the cube of the velocity field, the depth-averaged velocity profile, and the density of water. The regions with high flow velocities would have high Average Power Density values and vice versa. The simulation models have been validated with experimental measurement data for tropical waters and from results reported in the literature for temperate waters.
Tidal velocity faces resistance along its boundary at the seabed, creating rise reduction in flow velocity at the seabed. This resistance to shearing fluid motion is mainly caused by the roughness of the seabed caused by soil composition, seabed waviness, roughness, sediment type such as sand, mud, gravel, or shells, and morphology as it would alter the tidal velocity values which in turn would reduce the accuracy of tidal resource prediction. Thus, one of the principal objectives of this research was to study the effects of seabed friction on tidal prediction of a potential location of extraction using the open-source finite element-based 2-dimensional depth average model (Advance circulation- ADCIRC). The tides propagation in the region was solved by ADCIRC which provided temporal and spatially velocity fields in the region. It also helped in locating the region of high velocities in Alderney Race. The friction coefficient varied between two locations based on their seabed morphology. The friction coefficient values used in this model were chosen based on measured experimental data.
Velocity profiles show the transient variation of the scalar velocity at a point in the region of simulation. From the velocity profile of a location near the Alderney race, as shown in Figure 4, it can be seen that the maximum velocity in the region is around 3.5 m/s with a friction coefficient of 0.0025. The velocity profiles also show the repetitive velocity pattern on the location at a time interval of 15 days because of tidal constituents. The maximum velocity value decreases by about 40–45% with the increase in friction coefficient to about 0.01 as shown in Figure 5.
Surface elevation profile or tidal height profile is mainly due to interference of all tidal constituents. From the surface elevation profile of a location near the Alderney race, as shown in Figure 6, it can be found that the surface elevation in the region is in the range of around 4 m with the friction coefficient of 0.0025. Surface elevation increases by about two times with an increase in friction coefficient to about 0.005 as shown in Figure 7.
From the velocity profiles, Average Power Density plots for the location near the Alderney race were generated. From the Average Power Density plot of the location near the Alderney race, as shown in Figure 8, it can be found that the maximum Average Power Density at the location is about 15 kW/m2 for the friction coefficient of 0.0025. The Average Power Density decreases by 70–75% with the increase in friction coefficient to about 0.01 as shown in Figure 9.
The other objective of this research is to perform a comparative study on tidal prediction of the Alderney Race and Singapore using the 2D depth average model. Tidal prediction of temperate waters of the Alderney race was compared with tropical waters of Singapore to show the credibility of the chosen open-source model in tidal prediction of both temperate and tropical waters. This is also mainly done to understand and predict the tidal condition in a tropical location like Singapore with high accuracy and precision. As shown in Figure 10, the maximum depth in the Alderney race is around 166.5 m while the maximum depth in Singapore is only around 100 m. Simulations were run for the same time period of 30 days with a timestep of 5 s and a ramp of 5 days.
From Figure 11, the maximum velocity in a location near Singapore is only about 1.4 m/s while that of the Alderney race is around 3.5 m/s or more as shown in Figure 3. From Figure 12, it can also be seen that the surface elevation in Singapore is in the range of around 3 m while that of the Alderney race is around 4 m as shown in Figure 6. The average power density in Singapore is only around 1.5 kW/m2, as shown in Figure 13, while that of the Alderney race is about 15 kW/m2 as shown in Figure 8.
The results from the model show that it is a very useful open-source ocean model to predict the Tidal potential of a site and to understand the effects of Seabed friction on the flow prediction of a site. Its implementation could form the basis for a tidal energy assessment of the developing nations, such as South East Asian Countries, which have the required power source but are lacking energy measurement and prediction capabilities. This would become very helpful to even small island development states to understand their tidal resource potential. Such understanding would help to further size the tidal energy systems and deploy them in their waters. Developed tidal modeling tools using open-source ocean models along with available open-source bathymetry, coastlines, and tidal constituent data can accurately predict the tidal resource potential of both temperate and tropical waters as per IEC TS 62600-201, 2015 standards.
From the results, it can be noted that seabed roughness plays a major role in the depth average models due to its major energy dissipating phenomenon for the energetic ocean flow. It has the highest influence over the first water layer from the seabed and it diminishes as we move further up from the seabed due to its energy dissipation with the increase in velocity. To assess this effect of seabed roughness, models were run at various friction coefficient values and results such as velocity profiles, surface elevation profiles, and Average Power Density plots were obtained. These plots help in providing a detailed comparison between the results obtained from the different values of friction coefficient. As seen from results, a lower value of friction coefficient produces higher velocities as it results in less energy dissipation and vice versa. Also, since the Average Power Density is directly related to velocity, it has a major direct influence on friction over it. Further, surface elevation profiles do not show much variation after the friction coefficient value is increased by two times and the general nature of the curve remains the same. Therefore, it is important to optimize the seabed roughness of the location and include it in the models to obtain an accurate tidal prediction.
From the results, temperate water sites with higher water depths such as the Alderney race experience high tidal velocity in the range of 3.5–4 m/s in comparison with tropical water sites such as Singapore with shallow water depths that experience low tidal velocity in the range of 1–1.5 m/s. This low value of velocity and Average Power density of Singapore is mainly due to its geographical location surrounded by various countries around it preventing the ocean water to flow at a high velocity near Singapore. On the other hand, the Alderney race experience such high tidal velocity due to geographical location experiencing tidal waves from the Atlantic Ocean as well as due to the islands surrounding it. The tidal wave amplitude increases when it crosses the continental shelf and subsequently when this wave is reflected by the Cotentin Peninsula, acting as a barrier. This creates a significant tidal range in the Normandy-Brittany Gulf causing strong tidal currents in the Alderney race which is further amplified due to the presence of surrounding islands.
The open source ADCIRC model has been well validated by various tidal experts all-round the globe (Serhadlıoğlu et al., 2013; Luettich et al., 1992; Adcock et al., 2014; Kreitmair et al., 2019). The predicted results in this study were further validated with measured Acoustic Doppler Current Profiler (ADCP) data at the location near the coastline of the southern island of Singapore, as shown in Figure 14, and were found to be with Root Mean Square Error (RMSE) of about 5–10%.
Alderney race with high tidal energy extraction potential was selected as a location to study the effects of seabed roughness on tidal prediction of a potential location of extraction using the open-source finite element-based 2-dimensional depth average model (Advance circulation–ADCIRC). From the results, seabed roughness plays a major role in the tidal prediction using depth average models due to its major energy dissipating phenomenon for the energetic ocean flow. Hence, it is important to optimize the seabed roughness of the location and include it in the models to obtain an accurate tidal prediction. As seen from results, a higher value of friction coefficient produces lower velocities as it results in high energy dissipation and vice versa. Also, since the Average Power Density is directly related to velocity, it has a major direct influence on friction over it.
Secondly, simulation models were used to predict the tidal profile around the coast of Singapore and to compare it with that of the Alderney Race. Singapore’s model showed low tidal velocity and Average Power density mainly due to its geographical location surrounded by various countries around it preventing the ocean water from flowing at a high velocity near Singapore due to its shallow water depths. On the other hand, the Alderney race experiences high tidal velocity due to geographical location experiencing tidal waves from the Atlantic Ocean as well as due to the islands surrounding it.
The present work is mostly a 2D approach and further work is in progress towards 3D modeling. However, the proposed method meets the requirements of average power density calculations with the least computational time and also complies well with IEC standards and EMEC guidelines. Thereby, with the proposed methodology, IEC standards, and EMEC guidelines, one would be able to predict the energy availability of the sites.
One of the main limitations of this research would mainly be the accuracy of the input data used for the open-source ocean models. Highly accurate data would result in accurate tidal resource prediction of sites that need to be worked on further as a part of this study. Also, as further research work, the effect of meteorological factors, such as wind and wave, which affect the flow to a certain extent would be included in the models (Garg et al., 2018) and studied to further increase its accuracy of prediction. This would be done using measured highly accurate wind (Tieo et al., 2018) and wave data (Anh et al., 2018) and including it in the open source-based ocean models.
The datasets presented in this article are not readily available because the seabed bathymetry and other oceanography data are restricted from the purchased source. Requests to access the datasets should be directed to Srikanth Narasimalu, bnNyaWthbnRoQG50dS5lZHUuc2c=.
NS contribution is towards problem formulation, seabed friction studies, field measurements and simulation model setup, results analysis and correlation against field data for validation. LK contribution is towards input data collection and simulation model coding.
Frontiers Media SA remains neutral with regard to jurisdictional claims in published maps and institutional affiliations.
The authors declare that the research was conducted in the absence of any commercial or financial relationships that could be construed as a potential conflict of interest.
We would like to acknowledge Ocean Energy Systems (OES), an International Energy Agency Initiative as the study presented in the paper was carried out as a part of the OES project on Tidal Energy Modeling, Verification, and validation through the formation of International Tidal Resource Modeling Working group.
Anh, N., Prasad, M., Srikanth, N., and Sundaram, S. (2018). Wave Forecasting Using Meta-Cognitive Interval Type-2 Fuzzy Inference System. Proced. Comput. Sci. 144, 33–41. doi:10.1016/j.procs.2018.10.502
Bourgoin, A. (2019). Cherbourg, France: Normandie Université. PhD diss. Bathymetry Induced Turbulence Modelling the Alderney Race Site: Regional Approach with TELEMAC-LES.
Carballo, R., Iglesias, G., and Castro, A. (2009). Numerical model evaluation of tidal stream energy resources in the Ría de Muros (NW Spain). Renew. Energ. 34 (6), 1517–1524. doi:10.1016/j.renene.2008.10.028
Chen, W. B., Chen, H., Lin, L. Y., and Yu, Y. C. (2017). Tidal Current Power Resources and Influence of Sea-Level Rise in the Coastal Waters of Kinmen Island, Taiwan. Energies 10 (5), 652. doi:10.3390/en10050652
Coles, D. S., Blunden, L. S., and Bahaj, A. S. (2017). Assessment of the Energy Extraction Potential at Tidal Sites Around the Channel Islands. Energy 124, 171–186. doi:10.1016/j.energy.2017.02.023
Draper, S., Adcock, T. A. A., Borthwick, A. G. L., and Houlsby, G. T. (2014). Estimate of the Tidal Stream Power Resource of the Pentland Firth. Renew. Energ. 63, 650–657. doi:10.1016/j.renene.2013.10.015
Du Bois, P. B., Dumas, F., Solier, L., and Voiseux, C. (2012). In-situ Database Toolbox for Short-Term Dispersion Model Validation in Macro-Tidal Seas, Application for 2D-Model. Continental Shelf Res. 36, 63–82. doi:10.1016/j.csr.2012.01.011
Garg, N., Ng, E. Y. K., and Narasimalu, S. (2018). The Effects of Sea spray and Atmosphere-Wave Coupling on Air-Sea Exchange during a Tropical Cyclone. Atmos. Chem. Phys. 18 (8), 6001–6021. doi:10.5194/acp-18-6001-2018
González-Gorbeña, E., Rosman, P. C. C., and Qassim, R. Y. (2015). Assessment of the Tidal Current Energy Resource in São Marcos Bay, Brazil. J. Ocean Eng. Mar. Energ. 1 (4), 421–433. doi:10.1007/s40722-015-0031-5
Guillou, N., and Thiébot, J. (2016). The Impact of Seabed Rock Roughness on Tidal Stream Power Extraction. Energy 112, 762–773. doi:10.1016/j.energy.2016.06.053
Iec Ts 62600-201, (2015). Marine Energy—Wave, Tidal and Other Water Current Converters. Part 201: Tidal Energy Resource Assessment and Characterization. Geneva, Switzerland: International Electrotechnical Commission.
Kempener, R., and Newmann, F. (2014). Tidal Energy Technology Brief. Abu Dhabi: International Renewable Energy Agency (IRENA).
Kreitmair, M. J., Draper, S., Borthwick, A. G. L., and Van Den Bremer, T. S. (2019). The Effect of Uncertain Bottom Friction on Estimates of Tidal Current Power. R. Soc. Open Sci. London, UK: Royal Society open science 6, 180941. doi:10.1098/rsos.180941
Le Provost, C., Lyard, F., and de Midi-Pyrénées, O. (2003). The Impact of Ocean Bottom Morphology on the Modelling of the Long Gravity Waves, from Tides and Tsunami to Climate. Charting Secret World Ocean Floor GEBCO Project.
Le Provost, C., and Poncet, A. (1978). Finite Element Method for Spectral Modelling of Tides. Int. J. Numer. Meth. Engng. 12 (5), 853–871. doi:10.1002/nme.1620120510
Luettich, R. A., and Westerink, J. J. (2004). Formulation and Numerical Implementation of the 2D/3D ADCIRC Finite Element Model Version 44. XX. United States. University of North Carolina at Chapel Hill.
Luettich, R. A., Westerink, J. J., and Scheffner, N. W. (1992). ADCIRC: an Advanced Three-Dimensional Circulation Model for Shelves, Coasts, and Estuaries. Report 1, Theory and methodology of ADCIRC-2DD1 and ADCIRC-3DL.
Mayo, T., Butler, T., Dawson, C., and Hoteit, I. (2014). Data Assimilation within the Advanced Circulation (ADCIRC) Modeling Framework for the Estimation of Manning's Friction Coefficient. Ocean Model. 76, 43–58. doi:10.1016/j.ocemod.2014.01.001
Medeiros, S. C., Hagen, S. C., and Weishampel, J. F. (2012). Comparison of Floodplain Surface Roughness Parameters Derived from Land Cover Data and Field Measurements. J. Hydrol. 452-453, 139–149. doi:10.1016/j.jhydrol.2012.05.043
Pal, R. K., Srikanth, N., and Lakshmanan, K. (2018). IEEE, 1–8. Tidal Resource Modelling: Alderney Race. Asian Conference on Energy, Power and Transportation Electrification (ACEPT).
Quirapas, M. A. J., Srikanth, N., Abundo, Michael., and Lin, H. (2021). Electricity from the Ocean: A Path to Secure Energy in Southeast Asia. Bangkok, Thailand: ASEAN Green Growth, 19–21.
Roberts, A., Thomas, B., Sewell, P., Khan, Z., Balmain, S., and Gillman, J. (2016). Current Tidal Power Technologies and Their Suitability for Applications in Coastal and marine Areas. J. Ocean Eng. Mar. Energ. 2 (2), 227–245. doi:10.1007/s40722-016-0044-8
Serhadlıoğlu, S., Adcock, T. A., Houlsby, G. T., Draper, S., and Borthwick, A. G. (2013). Tidal Stream Energy Resource Assessment of the Anglesey Skerries. Int. J. Mar. Energ. 3, e98–e111. doi:10.1016/j.ijome.2013.11.014
Srikanth, N. (2016). Ocean Renewable Energy in Southeast Asia: Landscape, Opportunities and Challenges. Singapore: Research Publishing Services.
Srikanth, N., and Rao, S. S. (2017). IEEE, 1–9.Subsea Cable Health Monitoring SystemAsian Conference on Energy, Power and Transportation Electrification (ACEPT).
Tieo, J. J., Koh, T. Y., Skote, M., and Srikanth, N. (2018). Variance Characteristics of Tropical Radiosonde Winds Using a Vector-Tensor Method. Energies 11, 137. doi:10.3390/en11010137
Waldman, S., Yamaguchi, S., O’Hara Murray, R., and Woolf, D. (2017). Tidal Resource and Interactions between Multiple Channels in the Goto Islands, Japan. Int. J. Mar. Energ. 19, 332–344. doi:10.1016/j.ijome.2017.09.002
Wang, T., and Yang, Z. (2017). A Modeling Study of Tidal Energy Extraction and the Associated Impact on Tidal Circulation in a Multi-Inlet bay System of Puget Sound. Renew. Energ. 114, 204–214. doi:10.1016/j.renene.2017.03.049
Weatherall, P., Marks, K. M., Jakobsson, M., Schmitt, T., Tani, S., Arndt, J. E., et al. (2015). A New Digital Bathymetric Model of the World's Oceans. Earth Space Sci. 2 (8), 331–345. doi:10.1002/2015ea000107
Keywords: tidal energy, open source, depth average model, alderney race, Singapore, seabed friction
Citation: Srikanth N and Kannappan L (2021) Open-Source Code-Based Tidal Modeling of Tropical and Temperate Waters. Front. Energy Res. 9:550877. doi: 10.3389/fenrg.2021.550877
Received: 10 April 2020; Accepted: 28 May 2021;
Published: 09 July 2021.
Edited by:
Davide Astiaso Garcia, Sapienza University of Rome, ItalyReviewed by:
Sathyajith Mathew, University of Agder, NorwayCopyright © 2021 Srikanth and Kannappan. This is an open-access article distributed under the terms of the Creative Commons Attribution License (CC BY). The use, distribution or reproduction in other forums is permitted, provided the original author(s) and the copyright owner(s) are credited and that the original publication in this journal is cited, in accordance with accepted academic practice. No use, distribution or reproduction is permitted which does not comply with these terms.
*Correspondence: Narasimalu Srikanth, bnNyaWthbnRoQG50dS5lZHUuc2c=
Disclaimer: All claims expressed in this article are solely those of the authors and do not necessarily represent those of their affiliated organizations, or those of the publisher, the editors and the reviewers. Any product that may be evaluated in this article or claim that may be made by its manufacturer is not guaranteed or endorsed by the publisher.
Research integrity at Frontiers
Learn more about the work of our research integrity team to safeguard the quality of each article we publish.