- 1Department of Energy Systems, University of Thessaly, Larissa, Greece
- 2Department of Chemical Engineering, University of Western Macedonia, Kozani, Greece
- 3School of Chemical Engineering, National Technical University of Athens, Athens, Greece
Biofuel production from microalgae non-food feedstock is a challenge for strengthening Green energy nowadays. Reviewing the current technology, there is still reluctance in investing towards the production of new algal strains that yield more oil and maximize capital gains. In the current work, the microalgal feedstock selection problem is investigated for increased lipid production and nano-catalytic conversion into clean biofuel. For that purpose, a variety of Fuzzy Multi-Criteria Decision Making processes and a multitude of Optimization criteria spanning to technological, environmental, economic, and social aspects are used. The strains selected for the analysis are Chlorella sp., Schizochytrium sp., Spirulina sp., and Nannochloropsis sp. The methods applied are fuzzy analytic hierarchy process, FTOPSIS (fuzzy technique for the order of preference to the ideal solution), and FCM (fuzzy cognitive mapping). Pairwise comparison matrices were calculated using data from extensive literature review. All aforementioned fuzzy logic methodologies are proven superior to their numeric equivalent under uncertain factors that affect the decision making, such as cost, policy implications, and also geographical and seasonal variation. A major finding is that the most dominant factor in the strain selection is the high lipid content. Moreover, the results indicate that the Chlorella Vulgaris microalgae is ranked as the best choice by the FTOPSIS method followed by the Nannochloropsis strain, and Spirulina Platensis was found to be the last in performance. The best and worst case scenario run with FCM experimentally verify this choice indicating that Chlorella Vulgaris follows this trend of selection mostly with the technological and the economic criteria for both the sigmoid and the hyperbolic tangent deep-learning functions used.
Introduction
Bioenergy and biofuel production in the framework of sustainability has gained significant interest in recent years and represents one of the main priorities in energy research. Biofuel, especially, has proven to be the ultimate alternative for green and renewable energy production, which drastically reduces greenhouse emissions despite the high operating cost and the technological process irregularities in the involved supply chain (Chandel et al., 2018; Gardy et al., 2019). Due to renewable biofuel potential for green recovery, biomass production has recently attracted enormous attention worldwide for contributing to climate change mitigation (Roussos et al., 2019). In this context, biomass production is a non-toxic technology that creates renewable energy utilizing established procedures specific to the desired biofuel production (Sindhu et al., 2017). Lately, special interest has been shown and directed to the microbial fuel cells, among which microalgae in particular presents a promising environmental adaptability in preserving nutrients and storing lipids that can be extracted to provide a sustainable energy source. This can migitate the gap between economic development and environmental preservation (Nizami et al., 2017).
Microalgae is the primary third generation biofuel (non-food) source that has been proved to be superior for biofuel production among other conventional terrestrial crops in terms of cell structure, growth rate, and photosynthetic yield (Viswanath et al., 2010; Dutta, Daverey, and Lin 2014). Specifically, microalgae are individual plant cells that can be cultivated either in photo-bioreactors, or in specially constructed open ponds or even in a hybrid combination of both systems (usually raceway open ponds and closed photo-bioreactors). They utilize CO2, micronutrients, and solar light to multiply and produce raw microalgal biomass containing carbohydrates, lipids, and proteins (Arora et al., 2019; Papapolymerou et al., 2019; Zhang et al., 2020).
Cultivation of microalgae offers distinct advantages that ensure process viability and sustainability as feedstock for clean biofuel energy production (Chisti 2008; Li et al., 2008; Singh and Gu 2010; Carmichael et al., 2017; Yadav et al., 2020). The most important advantages include: 1) increased biomass production capability, 2) minimal nutrient requirements, fewer water needs and even possibility of using wastewaters containing residual nutrients and also no requirements for herbicides or pesticides, with expected environmental and economic gains, 3) less land demand, thus avoiding competition with other land-based crops also taking into account the potential for cultivation in non-arable areas, and 4) resistance of several species to salinity or even growth in marine environment with obvious benefits for cultivation in coastal areas.
Especially, microalgal biomass is a renewable energy source with its carbon content being totally derived from carbon dioxide (CO2) and also with the perspective of fixing and recycling CO2 exhausts from fossil fuel burning, (e.g. from co-located thermal power plants) in algal biomass production towards CO2 bio-mitigation and global carbon emission reductions. In the context of circular bio-economy, additional advantages include: 1) a more efficient microalgal oil extraction ability, 2) an increased biofuel biodegradability and non-toxicity, 3) the possibility of co-generation of other products for use in food, cosmetics and medical industry, 4) the potential for exploitation of new by-products as crop fertilizers or food additives, (e.g. the remaining algal biomass being rich-in protein, carbohydrates and small quantities of non-extractable lipids and micronutrients).
According to (Nwokoagbara et al., 2015), the stages in producing biofuels from microalgae are the following:
• microalgae strain selection and cultivation,
• harvesting,
• algal oil extraction via pretreatment (drying) and treatment (fluid extraction) via various physicochemical processes, and finally
• oil transformation to produce the desired type of biofuel.
For the conversion of microalgal biomass-derived oil feedstock into biofuel by the catalytic transesterification reaction, alkalis (KOH or NaOH) have been used as homogeneous catalysts (Campbell 2008), while porous microspheres, (e.g., zirconia, titania and alumina) have been used as heterogeneous ones (Raehtz. 2009). At the boundary between them, nanocatalysts with enhanced stability, catalytic activity, low cost, recyclability, and reusability are increasingly being considered to provide new pathways for efficient and environment-friendly biofuel production. The latest achievements in nanomaterial design for algal lipid transformation include a photochemically synthetized SrTiO3 nanocatalyst (for microalgae Chlorella vulgaris), a Fe2O3 nanocatalyst, a KOH-supported magnetic alumina-ferric oxide nanocatalyst, and a poly-ethylen-glycol encapsulated ZnOMn2+ nanocatalyst (for Nanochloropsis oculata) (Banerjee et al., 2019; Kazemifard et al., 2019; Vinoth Arul Raj et al., 2019; Aghilinategh, Barati, and Hamadanian 2020; Safakish et al., 2020).
Generally, the overall process optimization includes very complex research issues and involves diverse limitations in components and system parameters, in order to establish a viable large-scale microalgal oil production for biofuel (Carmichael et al., 2017). In order to maximize the quantities of biofuel generation, an optimization of microalgae cultivation with improved productivity is a prerequisite, taking into consideration the advancements in physicochemical parameters, including temperature light irradiation, CO2 incoming feed etc., for enhancing microalgal biomass yield (Gouveia 2011; N.; Hossain and Mahila, 2019). Moreover, apart from the harvesting, processing, and fuel extraction methodologies, according to (Viswanath et al., 2010), most critical decisions are especially related to the microalgal strain selection focusing on properties of critical significance such as: 1) the growth rate of the plants, 2) the lipid content, 3) the fatty acid profile, and 4) the ease of harvesting to make the technology one that is worth advancing and which has the corresponding economic impact to decisively contribute to the sustainability of the process. Actually, some microalgae strains favor high biomass production while others favor high lipid concentration, thus it is important to select an appropriate algae strain with both high biomass and oil productivity, which also can be grown at relatively low cost (Hossain et al., 2008; Yoo et al., 2010). Moreover, genetic and metabolic engineering approaches have lately been investigated for improving the valuable lipid content in microalgae that are increasingly considered as promising “cell factories” (Naghshbandi et al., 2020).
Nevertheless, extended research has shown that already over 50,000 microalgae species have been recorded and around 30,000 of them have been studied. Furthermore, there is not any classification of the already analyzed strains in terms of their properties (both chemical, physical, and biological). Having the aforementioned facts in mind, microalgal strain selection becomes a critical and multi-various decision making problem depending on a repertoire of criteria of diverse methodological categories and uneven weight importance. To deal with the microalgae strain selection problem using conventional MCDM methodologies is not convenient due to the fact that there is a multitude of decision making variables involved that are of non-numeric nature. Furthermore, the participation of experts’ opinion in the process automatically initiates the mixing of categorical properties having linguistic values. Additionally, attacking the problem using a participatory modeling methodology naturally inserts a variety of uncertainties related to the strain selection, and again all subjectivities of experts in the field can only be expressed using fuzzy linguistic variables as opposed to regular numeric quantities (Zhao, Xu, and Ren 2019; Lak Kamari et al., 2020). On the other hand, the involvement of fuzzy linguistic variables when applying multi-criteria decision making has shown to reveal the most influential criteria and parameters that are associated with the uncertainties occurring. So far, restricted information is reported in literature about the application of fuzzy MCDM methodologies in the critical task of the optimized selection of microalgae strains as feedstock for biofuel, given their ample differences in physico-chemical and biological properties that can strongly affect the overall production process.
The primary aim/objective of the present novel research is to systematically analyze and evaluate the potential of microalgae strains for clean biofuel production. For that purpose, an innovative holistic approach is proposed, which includes participatory modeling and a robust repertoire of advanced fuzzy logic-based multi-criteria processes/methodologies, namely FAHP, FTOPSIS, and FCM, to address and minimize uncertainties involved towards investing into microalgal potential plants that use selected feedstock. Moreover, the optimization criteria are classified into technological, environmental, social, and economic ones.
With the involvement of experts in the field, the most important parameters of the above four categories were identified. Then, a simple questionnaire was given to local and regional stakeholders of biofuel production industry, competent authorities officials and local residents to provide feedback relatively to the importance of each criterion used in relation to strain type. The set of available answers was scaled using intutionistic and linguistic values, thereby triggering the participation of the fuzzy MCDM methodologies mentioned above. The interoperability of the above criteria is not generally helpful, as there may exist negative or converse causalities between any two criteria elements. However, this is the reason that, in participatory modeling, regional and local stakeholders contribute on the creation, establishment and refinement of these causalities (Kokkinos and Karayannis 2020). The opinion of stakeholders is obtained using either surveys or focus groups. The answers acquired are treated using the aforementioned procedures to evaluate candidate decisional scenaria and/or to find near optimal solutions to the optimization problem at hand.
The structure and major contents of this study are: i. in section two, the methodologies are thoroughly presented with regard to the fuzzy processes used for finding the best suitable microalgal strain to produce biofuel. The criteria for the evaluation are taken mostly by the literature and experts on the field. A detailed description of the questionnaire used, the classification of criteria and the feedback of the local and regional stakeholders weighing and contemplating these criteria are also included; ii. Section three calculates the criteria priorities in a decision matrix for the case of FAHP, setting initially the hierarchy and the weight fuzzy scale. Similar approach is shown for the case of FTOPSIS, using triangular fuzzy membership functions. For the FCM case, a steady-state analysis is presented separately for each strain case scenario as well as best and worst case scenario of alternating the involved criteria. Furthermore, the results, discussion, and assessment are illustrated along with the overall procedure application in specific locations in Greece; and finally iii. main conclusions and future challenges of the problem under consideration are presented.
Methodologies
Microalgal Biomass Cultivation for Biofuel Production
Compliance With Ethical Principles
The present research methodology upholds high ethical standards. In that sense, ethics approval was not required in accordance with the local legislation and/or the institutional requirements. Indeed neither animal subjects nor human subjects/patients are involved. Moreover, identifiable personal human data are not included in the social surveys performed for the purpose of the study, which fully complied with the ethical principles. Specifically, all participants were given all the appropriate information regarding the aim and the content of the survey and especially the confidentiality and the anonymity of the participants, and finally they were asked whether they wanted to participate. Thus all participants volunteered to provide their opinions about the concepts of the study. At the same time, participants were informed about any possible shortcomings of the survey and particularly on the effort of the researchers to totally avoid the introduction of any bias either via questioning of certain scope or via the outcome statistics. Furthermore, the social survey was not sponsored by any organization or private sector business but it was solely aiming to record and assess the public opinion and potential acceptance relative to the growth and/or the worthiness of producing Green energy through the use of microalgal biomass renewable source and its processing into clean biofuel.
Participatory Modeling Process and Proposed Decision-Making Logical Architecture
The participatory modeling approach can be utilized to create the causal loop from all involved stakeholders to integrate: 1) microalgal cultivation methodologies, 2) social and environmental aspects and 3) economic aspects to introduce a comprehensive scheme of promoting specific strains that maximize profit. The goal is to understand the limitations and the extensions of the problem while at the same time, the stakeholders can discover all the drivers and factors that participate on this issue (Suprun et al., 2018). The work of Salim et al. (2019) identified the barriers, the drivers, and the enablers concerning the development and the management of renewable energy production plants. They also identified all criteria to initiate such development. Specifically for the case of microalgal cultivation for biofuel production, the strain selection is a multifaceted and complex problem involving various conflicting criteria. The multitude of interlinked factors affecting the process makes the strain selection a MCDM problem. According to (Wohlfahrt et al., 2019), there is a necessity to focus on criteria that improve the biomass transformation which corresponds to biofuel improvement with regard to sustainability. For that reason, we need to review the factors of critical significance in relation to bio-economy systems and how these systems interact, the level of their heterogeneity, the bending and flexibility of stakeholders’ strategies, the panoramic view of all spatiotemporal scales for the microalgal cultivation as well as other exogenous parameters such as the energy price market, the climate and various regional regulations and bylaws. The work of (Köhler. 2019) indicates the most important directions for sustainable innovation in terms of sustainability and biofuel production via evolutionary economics and technological innovation using agent based models that represent the neo-Schumpeterian ideas in terms of sustainable energy transitions. Towards the same lines of research, the work of Wang et al. (2019) assessed mostly the socioeconomic aspects in the production of aviation biofuel for the case of Brazil. This work provides a variety of scenarios illustrating the technological coefficients of the MCDM model. However, the above research works represent only a small sample of the available research directly related to the assessment of the multi-criteria problem of sustainable biofuel production. The survey of Hossard and Chopin. (2019) shows that there at least ∼2000 research articles up until 2019 that tackle the problem of sustainable biofuel production involving multi-criteria issues such as change, scale, pollution, biodiversity, practices, and terms on biophysical/regulatory conditions.
According to (Bekirogullari et al., 2020; Juan et al., 2020; Markou. 2020; Sung et al., 2020), the most popular systems for microalgal cultivation are summarized as follows:
• Outdoor cultivation systems which are open water repository systems (ponds, lakes etc.) that enable microalgae to treat nitrogen and phosphorus in the wastewater.
• Indoor cultivation such as large cultivation bags and tubular photobioreactors suitable for microalgae cultivation independently from the weather conditions avoiding contamination.
• Hybrid photobioreactor systems which use photobioreactors initially and then open systems to increase the biomass production.
• Microalgal biofilm systems that mostly deal with microalgal cultivation in wastewater, and
• Systems of microalgae cultivation in beads of alginate that trap algal cells in wastewater management plants.
To the best of our knowledge, the most relevant research that relates all categories of criteria to biomass and biofuel production belongs to (Bueyuektahtakin and Cobuloglu. 2014). The combination of the above literature with the surveying of a broad expert body gave us the following Table 1 of candidate criteria clustered into the four categories. Out of these criteria, according to (Viswanath et al., 2010) the most important technological criteria are the growth rate, the fatty acid profile, the ease of harvesting but above all the lipid content.
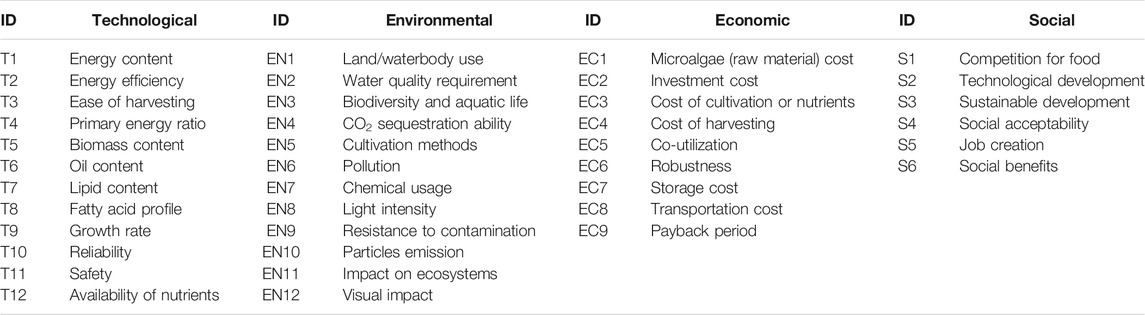
TABLE 1. Candidate Criteria for Microalgae Cultivation to optimize biofuel production. Courtesy from (Nwokoagbara, et al., 2015).
The above mentioned criteria are used to determine their fuzzy weights each in their specific category. For that reason four types of simple questionnaires were distributed to experts as well as to the competent authorities’ officials and other relevant stakeholders. The scaling on the answer choices was done using seven fuzzy linguistic values spanning from highly unimportant to highly important levels. The set of the methodologies used in the determination of the most important strains is shown in the following Figure 1 and it works as a logical architecture of the overall participatory modeling approach. More specifically, we first apply FAHP and FTOPSIS to calculate the pairwise comparison matrices of the criteria at hand. Four such matrices were computed, one for each category of criteria. This allowed us to do scenario analysis (steady state, worst and best case) for each one of aforementioned categories of criteria to evaluate their relative significance and importance.
Fuzzy Sets Theory and FAHP Method
Fuzzy sets were introduced by (Zadeh. 1965). Fuzzy sets differentiate from other set types because they contain elements that present a degree of membership i.e., making it possible to represent the imprecision level in non-numeric variables. Fuzzy relations are a generalization of L-Relations (Burgin and Kuznetsov. 1992) and they assign a membership grade in each set entity as a mapped value between 0 and 1. By permiting gradual assessment of the membership of set elements, fuzzy theory suceeds to provide significant results in fuzzy linguistics (Cock et al., 2000), in decision making (Kokkinos et al., 2019; Wang and Peng. 2020) and in clustering (Ullah et al., 2020).
The AHP method was introduced by (Saaty, 1987) to provide ratio scaling and since then, it has become the de facto tool to perform MCDM. The method however is applied only for problems of crisp numeric variables. To overcome the problem, FAHP was introduced by (Chang, 1996). FAHP calculates the relative importance between any pair of criteria in a hierarchy setup for a specific problem. The method uses triangular fuzzy numbers (TFNs) and a pairwise comparison to compute the fuzzy evaluation matrix. The TFNs are defined by the triplet
According to Altintas et al. (2020), the method performs a pairwise comparison between any two criteria translated from linguistic values to TFNs based on a nine-integer scale mapping which is depicted in Table 2. Intermediate numbers in the numeric scale get an analogous comproming in the triangular fuzzy scale. Additionally, the following set of algebraic operations is defined by Kahraman et al. (2003); Noorul Haq and Kannan. (2006); Chamodrakasv et al. (2010); and Chan et al. (2008) to calculate the pairwise comparison between criteria. The operations shown (apart from the representation of a TFN) are the following in this order: inverse of a fuzzy number, addition of two numbers, subtraction, multiplication, inner product, and summation of a set of fuzzy numbers respectively.
Assuming a set of n-criteria then the corresponding comparison matrix is given in the form:
Furthermore, the geometric mean is also needed to convert back the triangular fuzzy numers into crisp numeric and this operation is defined as:
Note that in the previous equation
Microalgal Biomass Selection Problem and FAHP–Related Works
Montingelli et al., 2015 have provided a comprehensive review related to biogas production from algal biomass proving that algae-derived biofuels are a promising alternative to the depleting of fossil fuels. However, the economic feasibility of massive biomass production from microalgae has not been established yet due to high energy requirement for harvesting and drying. For that reason, research based on FAHP was conducted by Tan et al. (2016) to prioritize the best harvesting and drying method at the same determining the degree of confidence of the experts who set up the criteria and the quantification process. FAHP has been used to evaluate global market shares in terms of the renewable bio-energies. Initially, the research of Ubando et al. (2020) evaluates new algal bioproducts in collaboration with existing industries. The authors created a Decision Support System (DSS) that helps the industry to choose the best among candidate sites and regions to cultivate microalgae based on the environmental impact, several economic burdens, social aspects, and the viewpoints of stakeholders via an FAHP that incorporates uncertainty of opinions. For the same area, a study by (Ubando et al., 2016a) focuses on the factored ranking of several criteria using FAHP and Artifical Neural Networks (ANN). The selected methodologies provided the ability to discover patterns of inconsistencies as well as a large amount of missing information in order to apply a complete participatory modeling process. Towards the same line of research, the work of (Correa et al., 2019) utilizes FAHP among other fuzzy modeling approaches to discover the most appropriate and feasible site for producing microalgal feedstock (but on a global and country level). In this work the optimization criterion is the revenue and profit while diminishing several collateral competitions of other targeted markets (food markets, cosmetics etc.). Their case studies mostly concentrate on the regions of Africa, Middle East, and western South America and this is the first sited work on the subject specifics. The same work in Ubando et al. (2016b) tries to analyze various microalgal cultivation systems based again on environmental impact, energy consumption, economic viability, social acceptability, and system robustness using FAHP via Monte Carlo simulations. These studies provided microalgal sites for cultivation for risk-averse and risk-inclined scenarios.
The majority of research that relates the FAHP method with the biofuel through microalgal biomass production can be represented by the work of Hamid et al., 2017. This work concentrates on the technological screening of the biomass production using FAHP for feature scaling normalization in order to select the optimum processing procedure for sustainable biofuels production from algae. But as it happens with other forms of renearble resource feedstocks (Katooli et al., 2019; Kheybari et al., 2019), the most important step towards sustainable profitability scenario is the development of an evaluation framework and a well established DSS that determines the importance of the selected criteria including all appearances of nature (climate, environment, technological, and social consequences).
FTOPSIS Method
The TOPSIS method is a numerical optimization methodology that utilizes a pseudo-polynomial heuristic based on the fact that, in a selection process, we must rank as the most appropriate the solution that close to the positive ideal decision candidate and far away from negative decision ideal candidate (Yoon and Hwang, 1995). The method was extended to include linguistic valued variables of fuzzy nature by Chen. (2000) and since then, it has been heavily used in many disciplines. Via FTOPSIS, policy makers can define each participating decision criterion using TFNs as shown in FAHP. Given any two TFNs
Most of therecent FTOPSIS research deals with the optimization of the renewable energy solution problem as presented in Rani et al. (2020; 2019); Cayir Ervural et al. (2018); Dinçer and Yüksel. (2019); Aikhuele et al. (2019); Krishankumar et al. (2020). We present the whole modeling approach as a seven step approach:
Step 1. Definition of the criteria involved and weight classification: The correspondence between the linguistic ratings and the TFNs is based on Table 2 as a Likert-scale type. According to this mapping, normalization of the TFNs is achieved using triplets within the space [0..1].
Step 2. Computation of the judgment matrix: For policy makers, the following matrix is computed as a combination of the available criteria
Step 3. Normalization of the judgment matrix: The JM matrix normalization is computed using the pre-clustering of the available criteria into the Benefit Criteria (BC) and Cost Criteria (CC) subsets. The result is denoted as NJM and the relevant equations follow:
Step 4. Construction of the weighted NJM denoted as WNJM and computed using:
Step 5. Computation of the the Fuzzy Positive Ideal Solution (FPIS) and the Fuzzy Negative Ideal Solution (FNIS), via calculation of two vectors
and
Step 6. Calculation of the distance between FPIS and FNIS, corresponding to the distance between
Step 7. Computation of the closeness coefficient of each policy and rating them in descending order
Microalgal Selection Problem and FTOPSIS–Related Works
The FTOPSIS methodology can be applied in the microalgae strain selection problem to produce biofuels as a holistic decision-making tool. Accordingly, the idea behind FTOPSIS is to choose one of the candidate elements that has the maximum possible distance from the negative ideal and the minimum possible distance from the positive ideal solution among the algal strain alternatives (Madugu and Collu, 2014). Even though it is distinguished that the production of biofuel from various agricultural and other wastes achieves higher overall performance compared to other technologies (Khishtandar et al., 2017), microalgal high sugar and oil is a promising source for biofuel production. For such production chains, FTOPSIS is the most valuable method to deal with the uncertainties of inventory deterioration issue, the applicability and efficiency of microalgal harvesting and the ranking of cultivation sites (Arabi et al., 2019; Peng et al., 2020).
Apart from the strain selection, other works try to evaluate the environmental risk of harmful algal bloom via fuzzy analytical methodologies such as FAHP and FTOPSIS that exert influence on the risk significance and severity (Gholami et al., 2019). Additionally, several scenario analyses deal with alternative techniques of biomass extraction from algae such as pyrolysis or phycoremediation of wastewater and compare the method and the properties of biofuel derived to various feedstocks using FTOPSIS and MCDM (Apandi et al., 2019; Mehta and Nirvesh, 2020). But the microalgal strain selection is extended only on the study of environmental social and economic impacts. Recent works investigate the appropriateness of fatty acid profiles to biodiesel characterization through the Preference Ranking Organization Method for Enrichment Evaluation (PROMETHEE) and the Graphical Analysis for Interactive Assistance (GAIA) analysis (Islam et al., 2013). Thus, there is no specific article, to the best of our knowledge, examining the microalgae strain ranking via multi-criteria analysis and FTOPSIS procedure.
Fuzzy Cognitive Mapping (FCM) for Multi-Criteria Decision Making
Fuzzy Cognitive Maps (FCMs) are semi-quantitative extensions of cognitive maps used for modeling and structuring expert knowledge that aims to determine the casual interrelations between participating concepts of a particular issue. Proposed by Kosko. (1986; 1992), FCMs are directed graphs of nodes representing the concepts and edges representing the causal relationships between any two concepts. The weight of the directed edges is fuzzified identifying a membership function for the degree of causality between the concepts. The activation of the concepts can be defined as a state vector thus, allowing the FCM to evolve as an ANN converging to a steady state. The causality of any concept pair can be quantified as a number between -1 and one spanning from a negative (inverse) very strong causality to a positive (analogous) very strong influence (Novak and Cañas, 2008). FCMs can carry out experts’ knowledge which is translated as an inclusion of the concepts and an initialization of the fuzzy causality weights between then. For several diverse conditions FCMs can be differentiated accordingly. For the case of decision making, let us denote the set of FCM concepts as
At every application of the activation function,
Threshold (transformation) functions for the inference procedure can be of: 1) bivalent, 2) trivalent, 3) sigmoid or 4) hyperbolic type as shown in the following equations respectively:
where
Microalgal Selection Problem and FCM–Related Works
Compared to the previous two methodologies, MCDM in relation to microalgal strain selection via FCM has not been studied. To the best of our knowledge, the most recently representative work is from (Naeini and Zandieh, 2020) that is focused on the microalgal biofuel production to be used for transportation. The authors apply a SWOT analysis to obtain realistic production strategies and then they rank them using stepwise weight assessment ratio analysis (SWARA). The method of utilizing the fuzzy linguistic environment involves FCMs to define the casual relationships between the criteria. Also worth mentioning, although not directly related to the topic under consideration, is the work by Falcone, Lopolito, and Sica. (2019). This research addresses the phases of the policy making cycle to find the best suitable strategy for the case of the Italian liquid biofuel niche. Through the application of FCMs and social network analysis indicate the highlights and several diversified policy making instruments in transitioning to renewable energies. Towards the same research direction, the work by Kokkinos et al. (2018) used a novel FCM modeling approach that studies the socio-economic and technological implications in relation to sustainable waste bio-refinery facilities’ establishment in order to overcome various uncertainties, by involving stakeholder participation in concept circumscription for the FCM and the decision making.
Logical Architecture of the System and Case Study
The logical architecture and the organizational context of the current research are depicted in Figure 1. Initially, the goal of the selection of the most suitable microalgal strain for the most economical viable biomass production is set for the research study. Among the huge variety of microalgal strains, for the case of Greece there few that have been studied either for wastewater treatment, biomass production or lipid concentration studies for other byproducts. Greece is a country with adequate climatological conditions (long sunshine periods, concentrations of high CO2 especially around highly urbanized regions etc.). Studies also show that marine microalgal species exhibit much higher growth rates when compared with the freshwater species (Aravantinou et al., 2013). Among those, we demonstrate the following strains which were chosen for our study:
• Chlorella vulgaris sp.: mostly occurred in Attica prefecture, Greece. C. vulgaris exhibit adequately high biomass and lipid productivity (up to 142.9 ± 6.5 mg L−1 d−1 can be achieved under sulfur limitation) (Savvides et al., 2019). These findings have also been justified in Mata et al. (2010); Sakarika and Kornaros (2019).
• Schizochytrium limacinum SR21: is considered as a low-cost nutrient cultivation source that produces high averages of docosahexaenoic acid (DHA) and total lipid content reaching high average levels with varying effluent concentrations (Bouras et al., 2020).
• Arthrospira (Spirulina) Platensis strains such as Chlorococcum sp. and Scenedesmus sp.: for which there have been various investigations in relation to the algal biomass growth rates and biochemical content mainly composed of proteins (up to 70%) and also polyunsaturated fatty acids and vitamins (Markou et al., 2019; Tsavatopoulou et al., 2019).
• Nannochloropsis sp.: that demonstrates a considerable fatty acid profile especially when using magnetic harvesting (Savvidou et al., 2020) and when it is cultivated in aquaculture wastewaters (Ansari et al., 2017; Dourou et al., 2020).
Using Table 1, criteria clustering experts from research and academia, education, competent authorities, and energy professionals were asked to provide a ranking of all involved parameters. Their evaluation was based on a questionnaire that directed them to evaluate each criterion in relation to all other criteria regardless of the category they fall in. Their evaluation was based on a Likert-scale in the range of 1–10, where one indicates the least important and 10 the most important criterion respectively. The summary of 14 expert responses is illustrated in the spider graph of Figure 4 with average values for each criterion rounded to the closest integer in the scale.
Results and Discussion
Ranking of Criteria for Microalgae Strain Selection Using Fuzzy-AHP Methodology
The primary goal using the Fuzzy AHP method is to find the relative importance between the factors and the criteria that affect either positive or negatively the microalgal strain selection for biofuel production. The method ranks these criteria assuming however that there is an initial input that relates the importance between any two pairs. For the pre-assumed clustering of Table 1, there is not any relevance between criteria of different clusters. For that reason, the Fuzzy AHP method was applied separately for each of the criteria clusters and these separate procedures allowed the completion of the reciprocal pairwise comparison matrices in every case.
The results are demonstrated in the following Tables 3–6 each depicting the: 1) initial pairwise comparison matrix before fuzzification, 2) the fuzzy geometric mean after the fuzzification, 3) the fuzzy weights of each criterion as a triplet of the lower, middle, and upper values, 4) the crisp numeric criteria weights and 5) the normalized crisp numeric weights. We only depict the pairwise comparison matrix before fuzzification to avoid the complexity and the density of the fuzzified version. We use the triangular membership functions and the fuzzy mapping of Table 2 allowing values in between these ranges.
Microalgal Strain Ranking via Fuzzy-TOPSIS Analysis
From the Fuzzy AHP described in the previous section, we choose from each of the four categories the two criteria that illustrated higher relative importance by the experts. For these eight in total criteria we calculate the relative importance pairwise comparison matrix including cross category comparisons. This is necessary since the values of the relative importance fuzzy weights are input to the FTOPSIS analysis. The inclusion of only two criteria per category introduces a minor error however, this is minimal since further inclusion of all criteria may cause the production of erroneous results for the case of totally irrelevant criteria. Table 7 below shows: 1) the produced crisp numeric relative importance weights, 2) the normalized crisp weights and the type of criteria clustering those into beneficial (i.e. the ones that need to be maximized) and into cost criteria (i.e. the ones that need to be minimized).
The criteria ID is denoted as T for technological, EN for environmental, EC for economics and S for social. The fourteen experts were asked to rate the four candidate microalgal strains for the eight aforementioned criteria. The experts assigned values to the strains for each criterion using linguistic values that correspond to normalized triangular fuzzy numbers as depicted in Table 8 allowing also intermediate values. Table 9 presents the Fuzzy Decision Matrix, the Fuzzy Positive Ideal Solution and the Fuzzy Negative Ideal Solution, according to steps. Finally, Table 10 shows the distance of each strain selection and for each criterion from the FPIS and the FNIS, the total distances for each strain and the ranking of the methodology for the four strains.
Microalgal Strain Selection Trend Using Fuzzy Cognitive Map Analysis
The FCM includes 40 concepts out of which only the “Biofuel Production” (denoted as BF) is the receiver (it only has incoming edges) and the rest are all ordinary concepts (they have incoming and outgoing edges). The FCM graph is not shown due to the number of concepts participating and the weighted causality edges (∼1,500) which makes the visualization of the graph impossible. However, the FCM statistics and concept metrics are depicted in Table 11 indicating the in-degree, out-degree, and the centrality of each concept. The results illustrate centrality similarities and a balance in the ratio of the in-degree/out-degree for concepts that belong to the same cluster/category. This is expected since the probability of interdependence and inter-causalities on same-cluster concepts always increases. At the same time, when there exists an apparent correlation between clusters (for example social issues affect environmental issues and vice versa) then, this reflects the corresponding interlinking between the interclass concepts.
Numerous simulations have been run in order to investigate: 1) the steady state of the FCM, 2) the FCM convergence using the clamping process as stated in Kosko 1986) and 3) the best- and worst-case scenarios for the trend of BF (increase/decrease). Note that separate simulations were performed for each microalgal strain fixing as constant the values for the concepts (especially the technological) when values have been established by other studies. These concepts included the lipid content according to the fixed daily lipid productivity findings given below:
• Chlorella vulgaris: 134 mg L−1d−1 (Kim et al., 2019)
• Schizochytrium limacinum: 108 mg L−1d−1 (S.-K. Wang et al., 2020)
• Arthrospira (Spirulina) Platensis: 89 mg L−1d−1 (Lu et al., 2019)
• Nannochloropsis sp: 105 mg L−1d−1 (Nogueira et al., 2020)
As for the clamping procedures, this cannot be applied to the driver concepts only because simply there are no driver concepts (concepts that have in-degree equal to 0). For that reason, our simulations will apply clamping using the most important concepts according to the criteria ranking summary produced by the experts as this is shown in Figure 4 making sure that all four criteria categories are represented in the analysis.
The steady state of the FCM is shown in Figure 5 depicting the final concept values as a defuzzified real number in the range [0.1] and the convergence curves for these concepts relatively to the number of iterations needed for the FCM to converge.
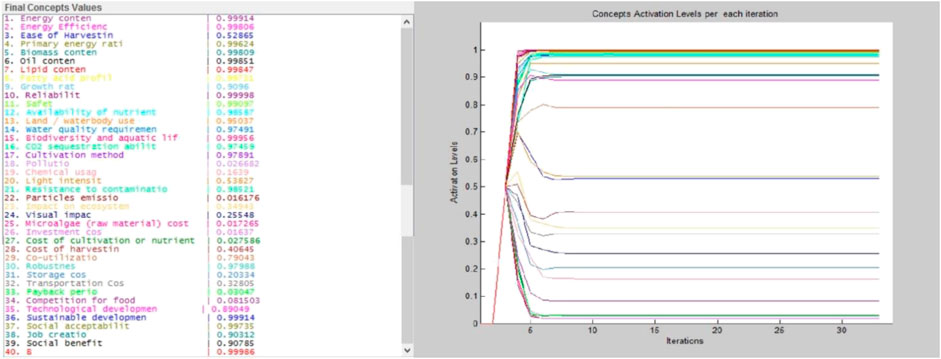
FIGURE 5. Steady State Analysis for the FCM and convergence curves relatively to the number of iterations.
To the best of our knowledge there does not exist any research that relates the importance and interrelation of criteria in an algal strain selection analysis using FCMs. For that reason, we used the aforementioned steady state FCM as a starting point to perform scenario analysis for the strain selection. More specifically, we did best and worst case analysis for each of the four strain candidates using both Sigmoidal and Hyperbolic Tangent learning methodologies. In all cases we fix the values of the lipid content (daily lipid productivity) with the values presented in this section previously. Furthermore, the rest of the participating concepts are set to values 0.1 for the worst case and one for the best case respectively. In total we performed 64 simulations to include all combinations of strains, learning methods, categories of criteria, and best/worst case scenarios.
Characteristically, in Figure 6, the best case scenario is shown, for the sigmoidal learning for the Schizochytrium limacinum sp. for the case of how the technological criteria affect the biofuel production. For this case, the lipid content criterion is set to 0.80 (after normalization of the daily lipid productivity values of all strains the value of 108 mg L−1d−1 corresponds to 0.90 in the range [0.1]). The rest of the technological criteria are all set to one and this configuration shows the potential percentage increase or decrease on the receiver concept i.e. to the biofuel production. Specifically for the described configuration we show an increase of about 5% to the biofuel production (see Figure 6).
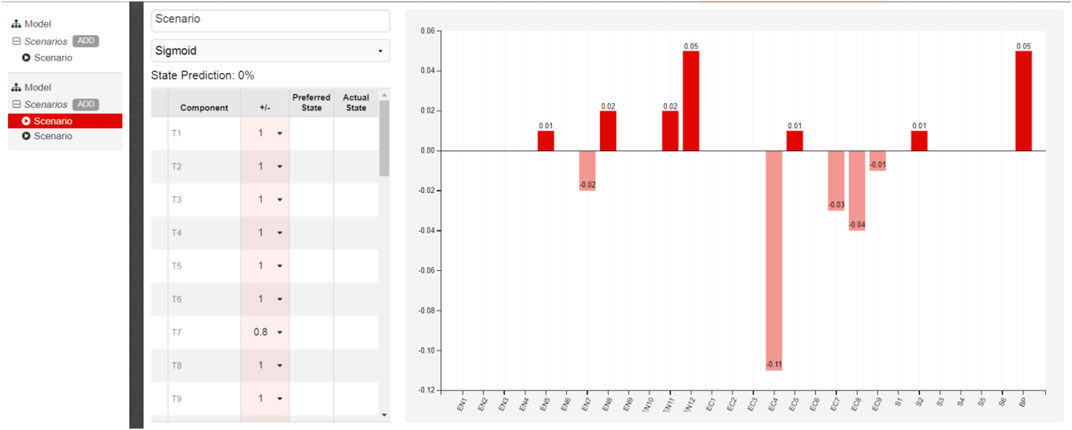
FIGURE 6. Best case scenario for the Schizochytrium limacinum sp with sigmoid learning and for the impact of the technological criteria.
On the other hand, Figure 7 illustrates the worst case scenario for the sigmoidal learning for the same strain. This is used for proof of correctness in terms on the negative impacts emanated from the technological criteria. More specifically, technological criteria that affect the concept of production BP are set to very small values (0.1 or even smaller than that) as shown for the concepts T1-T9 in Figure 7. Instead of using the lipid content criterion of 0.80 value, we use the worst possible value of 0.03. This negatively affects the production of the system by 12% while at the same time diminishes the cost of cultivation indicating a wrong choice of cultivation.
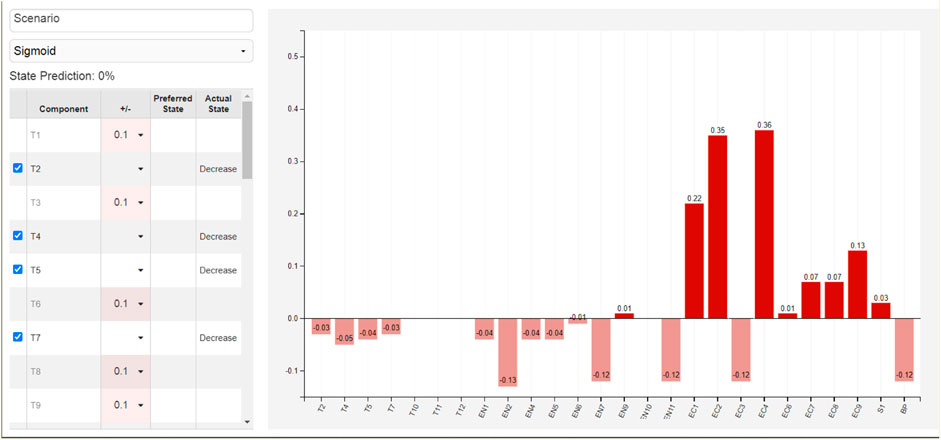
FIGURE 7. Worst case scenario for the Schizochytrium limacinum sp with sigmoid learning and for the impact of the technological criteria.
Finally we show the summary of all simulation findings in Table 12 for all cases of strains, learning methodologies criteria categories, and best/worst case scenarios.
The use of participatory modeling assumed the presence of regional stakeholders (with most of them to be experts in the field under study). The criteria and factors that influence the production of biomass from algae were set in Table 1, and according to the research specialists, they do cover all aspects spanning from technological to environmental, economic, and social. Furthermore, regional stakeholders were asked to individually rate these criteria using a fuzzy scale evaluation, which was defuzzified into a Likert-scale from 1 to 10. The experts’ weights were then used into a FAHP having as a primary goal the construction of the relative importance matrix using the fuzzy geometric mean of the aforementioned weights. Based on the findings and due to individual evaluations for each criteria category the most important criteria are:
• From the technological category, criteria T1 (Energy content) and T7 (Lipid content) outperform the rest with normalized weights of 13.68% and 11.61% of the whole category respectively as shown in the results of Table 3. The outperformance is relative to the overall descending ordering of all criteria. The rest of the technological criteria are ranked with importance weights to be much less than 10%. For that reason, we claim that energy content and lipid daily productivity seem to overcome all other criteria. The rest of the criteria are tightly coupled with those, thus the importance of these satisfies the technological requirements imposed. Safety and reliability are under-evaluated when compared to the purely technological criteria previously. This was expected, as most of the research community concentrates on the measurements of the lipid content and daily productivity, thus the microalgal energy efficiency becomes a primary factor.
• From the environmental category, three criteria get weight-percentages that exceed the 10%, namely ENV1 (Land/waterbody use), ENV2 (Water quality requirement) and ENV10 (Particles emission) with weight percentages 28.32%, 19.95%, and 11.55% respectively. This indicates that the land or waterbody availability is the most important factor, given that its absence totally abolishes the cultivation.
• From the economics category, four criteria out of nine are shown great importance, namely EC1 (Microalgae (raw material) cost) with 23.16%, EC2 (Investment cost) with 22.04%, EC4 (Cost of harvesting) with 18.90%, and EC7 (Storage cost) with the lowest performance of 14.41%. The equivalence of the above criteria indicates that costs of every level are most significant in investing into a biofuel production plant based on algae. The fact that EC1 leads the evaluation means that finding the raw material and harvesting is the most considerable cost compared to the investment cost; Finally, • From the social category, we experience almost equivalent performance from most of the criteria, as they are all highly interrelated. More specifically, S5 (Job creation) gets 29.54%, S6 (Social benefits) gets 18.96%, and S3 (Sustainable development) gets 16.54%. The interrelation between S5 and S6 is analogous since the higher the job creation the more the social benefits for the regional society. At the same time, the high numbers of social benefits and job creation for criteria indicate a sustainable environment where members of the regional society benefit from a stable economy due to steady income.
The FTOPSIS was applied only on the most substantial criteria of each category, on the basis of the FAHP findings. Taking the two more representative criteria from each category, we succeeded to evaluate the plants/investments of the most popular microalgal strains in the Greek territory. The results of FTOPSIS ranked Chlorella vulgaris and Nannochloropsis as the two strains having the best potential to produce higher amounts of biofuel according to the criteria imposed. More specifically, Chlorella vulgaris ranked as the most preferable (
The approach of the FCM investigation took care of this defficiency making sure that all criteria participated in the concept graph. The complexity of the graph is high, as almost for all concepts inter-causalities may be set. The evaluation of the four different strains is based on all criteria but the daily lipid productivity is predefined normalized and fixed for all FCMs under investigation. For each strain we invstigated the biofuel production trend in both the best and worst case scenarios, two FCM learning methods and trying to evaluate the impact of each category criteria to the trend. The results in Table 12 show:
A significant increase on biofuel (8.6%–9.3%) for Chlorella vulgaris due to the technological criteria increase in the best scenario (all technological criteria except lipid content are set to 1) using either Sigmoidal or Hyperbolic Tangent learning. Under the same conditions, Schizochytrium limacinum performs with an increase within the range of (5%–6.6%), Arthrospira (Spirulina) Platensis with an increase of (6.1%–6.8%) where Nannochloropsis shows an increase range of (2.7%–4.6%). In an analogous way, the results indicate a significant decrease of the biofuel production trend for the worst case scenario (where all technological criteria except lipid content are set to the value of 0.1). Similarly, the same trend is shown for the best and worst case scenario for the other three categories of criteria and the microalgal strains. Thus, the FCM can be used as a dependable Decision Support System that can help stakeholders, investors and competent authorities to select investment plans and support decision making.
Conclusion
The use of the appropriate microalgal strain as raw material for biomass feedstock towards nanocatalytic biofuel production is the most significant factor that controls investment land or waterbody plants, thus directly affecting the regional economy and local society. In this work, four microalgal strains for Greece as a case study were evaluated using a synthesized participatory modeling approach that: 1) aggreed on the criteria, which are imposed for the biofuel production, 2) applied FAHP method to find the relative importance of these criteria, 3) ranked the abovementioned criteria using the Fuzzy TOPSIS procedure; and finally 4) via the creation of a FCM, investigated the trend of increase/decrease of the biofuel production with a consideration of different learning methods, best and worst case scenarios and different criteria categories.
The overall methodology gave clear results as to the microalgae strain selection. Especially, the high lipid content appears to be the most dominant factor in the strain selection. Particularly, Chlorella vulgaris microalgae is ranked as the best choice followed by the Nannochloropsis strain via the FTOPSIS analysis. Furthermore, the best and worst case scenario runs using FCM methodology verify this choice, indicating that Chlorella Vulgaris follows this selection trend mostly with the technological and the economic criteria for both the sigmoid and the hyperbolic tangent functions applied. All fuzzy logic procedures employed were proven to be superior to their numeric equivalent ones under uncertain factors including cost, policy implications, and also geographical and seasonal variation, which affect the decision making process.
The above three MCDM processes act as alternative models to formal ones (algorithical and procedural). The formulation of assessment components with scaling procedures, elicitation, and estimation functionalities as well as stakeholder subjective criteria settings allow AHP, TOPSIS, and FCM to holistically address decision-making problems emanating from diverse environments. Numerous research from various fields such as energy, environment, medical informatics, and economics confirm that the abovementioned techniques are applied in a broad repertoire of problems. Additionally, the structural ability of the techniques regarding complex problems using multiple criteria with linguistic variables and fuzzy inference allows their application in an array of disciplines. From a long list of MCDM methodologies invented, the vast majority tackles problems of numeric nature and therefore belongs to the multiple objective mathematical programming ensemble of MCDM. However, when fuzzy inference is needed to model multi-objectives, then only a few methods are used, and in many cases, these overlap in terms of algorithms developed (VIKOR, Multi-Attribute Global Inference of Quality etc.). Thus the proposed triple view of the problem of microalgae strain selection to maximize biofuel production can be used as a paradigm for similar modeling in many other problems.
In this work, we provided the corresponding causality from microalgae cultivation for biomass energy production for the case of Greece. More specifically, we studied the impacts for microalgae production via nano-catalysis and we indicated how this is connected to the economic development via a set of technological environmental, economic and social criteria. The findings of the model outcomes provide a roadmap for selecting specific microalgae strains for cultivation in a specific region. Eventually, all models assert that biomass energy production via microalgal cultivation can be a circular economy efficient technique for environmentally sustainable development and it can similarly be promoted in other countries. At the same time, decision and policy makers must take into acount not only purely cultivation oriented constraints for the biomass production via microalgae but also must seriously consider environmental and social criteria in the strain selection problem. This work includes such constraints, though some other have been left out, including e.g., climatological conditions influencing microalgal biomass production and potential impact on climate change respectvely.
Nevertheless, there are few additional challenging issues that may be thoroughly investigated by a future research. Starting from the FTOPSIS application, it is worthy to evaluate all participating criteria without descriminating any of them. This may diminish the impact of some of the selected criteria or even increase the significance of others not taken into consideration. Moreover, the inclusion of similar but differentiated approaches to FTOPSIS, such as PROMETHE or the amalgamation of fuzzy and statistical methods, would be a future work challenge for improving ranking outcomes. Also, there are some further investigations that can be pursued in relation to the FCMs, indicatively: the lack of result consistency between the differences among the best and worst case scenarios for all categories, the application of FCM with additional machine learning methods and the exclusion of the inherited bias derived from the experts in terms of environmental and social issues.
Data Availability Statement
The original contributions presented in the study are included in the article/Supplementary Material, further inquiries can be directed to the corresponding author.
Ethics Statement
Ethical review and approval was not required for the study on human participants in accordance with the local legislation and institutional requirements. Written informed consent from the participants was not required to participate in this study in accordance with the national legislation and the institutional requirements.
Author Contributions
All authors listed have made a substantial, direct and intellectual contribution to the work, and approved it for publication.
Conflict of Interest
The authors declare that the research was conducted in the absence of any commercial or financial relationships that could be construed as a potential conflict of interest.
References
Aghilinategh, M., Barati, M., and Hamadanian, M. (2020). The modified supercritical media for one-pot biodiesel production from Chlorella vulgaris using photochemically-synthetized SrTiO3 nanocatalyst. Renew. Energy. 160 (November), 176–184. doi:10.1016/j.renene.2020.06.081
Aikhuele, D. O., Desmond Eseoghene, I., and Akinyele, D. (2019). Evaluation of renewable energy technology based on reliability attributes using hybrid fuzzy dynamic decision-making model. Technology and Economics of Smart Grids and Sustainable Energy. 4 (1), 16. doi:10.1007/s40866-019-0072-2
Altintas, K., Vayvay, O., Apak, S., and Cobanoglu, E. (2020). An extended GRA method integrated with fuzzy AHP to construct a multidimensional index for ranking overall energy sustainability performances. Sustainability 12 (4), 1602. doi:10.3390/su12041602
Ansari, F. A., Singh, P., Guldhe, A., and Bux, F. (2017). Microalgal cultivation using aquaculture wastewater: integrated biomass generation and nutrient remediation. Algal Research 21 (January), 169–177. doi:10.1016/j.algal.2016.11.015
Apandi, N. M., Mohamed, R. M. S. R., Al-Gheethi, A., and Kassim, A. H. M. (2019). Microalgal biomass production through phycoremediation of fresh market wastewater and potential applications as aquaculture feeds. Environ. Sci. Pollut. Res. Int. 26 (4), 3226–3242. doi:10.1007/s11356-018-3937-3
Arabi, M., Yaghoubi, S., and Tajik, J. (2019). Algal biofuel supply chain network design with variable demand under alternative fuel price uncertainty: a case study. Comput. Chem. Eng. 130 (November), 106528. doi:10.1016/j.compchemeng.2019.106528
Aravantinou, A. F., Theodorakopoulos, M. A., and Manariotis, I. D. (2013). Selection of microalgae for wastewater treatment and potential lipids production. Bioresour. Technol. 147 (November), 130–134. doi:10.1016/j.biortech.2013.08.024
Arora, N., Patel, A., Mehtani, J., Pruthi, P. A., Pruthi, V., and Poluri, K. M. (2019). Co-culturing of oleaginous microalgae and yeast: paradigm shift towards enhanced lipid productivity. Environ. Sci. Pollut. Res. Int. 26 (17), 16952–16973. doi:10.1007/s11356-019-05138-6
Banerjee, S., Rout, S., Banerjee, S., Arnab, A., and Das, D. (2019). Fe2O3 nanocatalyst aided transesterification for biodiesel production from lipid-intact wet microalgal biomass: a biorefinery approach. Energy Convers. Manag. 195 (September), 844–853. doi:10.1016/j.enconman.2019.05.060
Bekirogullari, M., Figueroa-Torres, G. M., Pittman, J. K., and Theodoropoulos, C. (2020). Models of microalgal cultivation for added-value products - a review. Biotechnol. Adv. 44, 107609. doi:10.1016/j.biotechadv.2020.107609
Bouras, S., Katsoulas, N., Antoniadis, D., and Ioannis, T. K. (2020). Use of biofuel industry wastes as alternative nutrient sources for DHA-yielding Schizochytrium limacinum production. Appl. Sci. 10 (12), 4398. doi:10.3390/app10124398
Bueyuektahtakin, I. E., and Cobuloglu, H. I. (2014). “A multi-criteria approach for biomass crop selection under fuzzy environment.” In Industrial and Systems Engineering Research Conference, Montreal, Canada, May 2014. https://soar.wichita.edu/handle/10057/11495
Burgin, M., and Kuznetsov, V. (1992). Fuzzy sets as named sets. Fuzzy Set Syst. 46 (2), 189–192. doi:10.1016/0165-0114(92)90131-M
Campbell, M. N. (2008). Biodiesel: algae as a renewable source for liquid fuel. Guelph Engineering Journal. 1, 2–7.
Carmichael, M. D., Petrides, D., and Siletti, C. (2017). “Large scale algal oil production for bio-fuel use: techno-economic analysis and evaluation,” in 2017 SIMB Annual Meeting and Exhibition, (Philadelphia, PA: SIMB).
Chamodrakas, I., Batis, D., and Martakos, D. (2010). Supplier selection in electronic marketplaces using satisficing and fuzzy AHP. Expert Syst. Appl. 37 (1), 490–498. doi:10.1016/j.eswa.2009.05.043
Chan, F. T. S., Kumar, N., Tiwari, M. K., Lau, H. C. W., and Choy, K. L. (2008). Global supplier selection: a fuzzy-AHP approach. Int. J. Prod. Res. 46 (14), 3825–3857. doi:10.1080/00207540600787200
Chandel, A. K., Garlapati, V. K., Singh, A. K., Antunes, F. A. F., and da Silva, S. S. (2018). The path forward for lignocellulose biorefineries: bottlenecks, solutions, and perspective on commercialization. Bioresour. Technol. 264 (September), 370–381. doi:10.1016/j.biortech.2018.06.004
Chang, D.-Y. (1996). Applications of the extent analysis method on fuzzy AHP. Eur. J. Oper. Res. 95 (3), 649–655. doi:10.1016/0377-2217(95)00300-2
Chen, T. C. (2000). Extensions of the TOPSIS for group decision-making under fuzzy environment. Fuzzy Set Syst. 114, 1–9.
Chisti, Y. (2008). Biodiesel from microalgae beats bioethanol. Trends Biotechnol. 26 (3), 126–131. doi:10.1016/j.tibtech.2007.12.002
Cock, M. D., Ulrich, B., and Etienne, E. K. (2000). Modelling linguistic expressions using fuzzy relations. In Proceedings of the 6th international conference on soft computing, Istanbul, Turkey, 2020, 353–360. doi:10.1.1.32.8117
Correa, D. F., Beyer, H. L., Hugh, P. P., Skye, R. T. H., and Schenk, P. M. (2019). Global mapping of cost-effective microalgal biofuel production areas with minimal environmental impact. GCB Bioenergy. 11 (8), 914–929. doi:10.1111/gcbb.12619
Dinçer, H., and Yüksel, S. (2019). Multidimensional evaluation of global investments on the renewable energy with the integrated fuzzy decision-making model under the hesitancy. Int. J. Energy Res. 43 (5), 1775–1784 doi:10.1002/er.4400
Dourou, M., Dritsas, P., Baeshen, M. N., Elazzazy, A., Al-Farga, A., and Aggelis, G. (2020). High-added value products from microalgae and prospects of aquaculture wastewaters as microalgae growth media. FEMS Microbiol. Lett. 367 (12). doi:10.1093/femsle/fnaa081
Dutta, K., Daverey, A., and Lin, J-G. (2014). Evolution retrospective for alternative fuels: first to fourth generation. Renew. Energy. 69 (September), 114–122. doi:10.1016/j.renene.2014.02.044
Ervural, C., Beyzanur, S. Z., Demirel, O. F., Aydin, Z., and Delen, D. (2018). “An ANP and fuzzy TOPSIS-based SWOT analysis for Turkey’s energy planning. Renew. Sustain. Energy Rev. 82 (February), 1538–1550 doi:10.1016/j.rser.2017.06.095
Falcone, P. M., Lopolito, A., and Sica, E. (2019). Instrument mix for energy transition: a method for policy formulation. Technol. Forecast. Soc. Change. 148 (November), 119706. doi:10.1016/j.techfore.2019.07.012
Gardy, J., Rehan, M., Hassanpour, A., Lai, X., and Nizami, A. S. (2019). Advances in nano-catalysts based biodiesel production from non-food feedstocks. J. Environ. Manag. 249, 109316. doi:10.1016/j.jenvman.2019.109316
Gholami, Z., Seddiq Mortazavi, M., and Karbassi, A. (2019). Environmental risk assessment of harmful algal blooms case study: Persian gulf and Oman sea located at hormozgan province, Iran. Hum. Ecol. Risk Assess. 25 (1–2), 271–296. doi:10.1080/10807039.2018.1501660
Gouveia, L. (2011). “Microalgae as a feedstock for biofuels,” in In Microalgae as a Feedstock for BiofuelsLuisa gouveia. SpringerBriefs in microbiology, 1–69 (Berlin, Germany: Springer). doi:10.1007/978-3-642-17997-6_1
Hamid, N. N. A., Martinez-Hernandez, E., and Lim, J. S. (2017). Technological screening of algae-based biorefinery for sustainable biofuels production using analytic hierarchy process (ahp) with feature scaling normalisation. CET. 61, 1369–1374. doi:10.3303/CET1761226
Hossain, A. S., Salleh, A., Boyce, A. N., Chowdhury, P., and Naqiuddin, M. (2008). Biodiesel fuel production from algae as renewable energy. Am. J. Biochem. Biotechnol. 4 (3), 250–254. doi:10.3844/ajbbsp.2008.250.254
Hossain, N., and Mahlia, T. M. I. (2019). Progress in physicochemical parameters of microalgae cultivation for biofuel production. Crit. Rev. Biotechnol. 39 (6), 835–859. doi:10.1080/07388551.2019.1624945
Hossard, L., and Chopin, P. (2019). Modelling agricultural changes and impacts at landscape scale: a bibliometric review. Environ. Model. Software 122 (December), 104513. doi:10.1016/j.envsoft.2019.104513
Islam, M. A., Magnusson, M., Brown, R. J., Ayoko, G. A., Nabi, M. N., and Heimann, K. (2013). Microalgal species selection for biodiesel production based on fuel properties derived from fatty acid profiles. Energies 6 (11), 5676–5702. doi:10.3390/en6115676
Juan, J. L. G. S., Mayol, A. P., Sybingco, E., Ubando, A. T., Culaba, A. B., Chen, W. H., et al. (2020). A scheduling and planning algorithm for microalgal cultivation and harvesting for biofuel production. IOP Conf. Ser. Earth Environ. Sci. 463 (April), 012010. doi:10.1088/1755-1315/463/1/012010
Kahraman, C., Cebeci, U., and Ulukan, Z. (2003). Multi‐criteria supplier selection using fuzzy AHP. Logist. Inf. Manag. 16 (6), 382–394. doi:10.1108/09576050310503367
Katooli, M. H., Aslani, A., Fatemeh Razi, A., Tania Mazzuca, S., and Bakhtiar, A. (2019). Multi-criteria analysis of microalgae production in Iran. Biofuels 0 (0), 1–7. doi:10.1080/17597269.2018.1542566
Kazemifard, S., Nayebzadeh, H., Saghatoleslami, N., and Safakish, E. (2019). Application of magnetic alumina-ferric oxide nanocatalyst supported by KOH for in-Situ transesterification of microalgae cultivated in wastewater medium. Biomass Bioenergy 129 (October), 105338. doi:10.1016/j.biombioe.2019.105338
Kheybari, S., Mahdi Rezaie, F., and Rezaei, J. (2019). Measuring the importance of decision-making criteria in biofuel production technology selection. IEEE Trans. Eng. Manag. 1, 15 doi:10.1109/TEM.2019.2908037
Khishtandar, S., Zandieh, M., and Dorri, B. (2017). A multi criteria decision making framework for sustainability assessment of bioenergy production technologies with hesitant fuzzy linguistic term sets: the case of Iran. Renew. Sustain. Energy Rev. 77 (September), 1130–1145. doi:10.1016/j.rser.2016.11.212
Kim, D. W., Shin, W.-S., Min-Gyu, S., Lee, B., and Chang, Y. K. (2019). Light intensity control as a strategy to improve lipid productivity in Chlorella sp. HS2 for biodiesel production. Biomass Bioenergy 126 (July), 211–219. doi:10.1016/j.biombioe.2019.05.014
Köhler, J. (2019). Advances in modelling sustainable innovation: from technology bias tosystem theories and behavioural dynamics. in Handbook of sustainable innovation, Chap 18, 310–330, Cheltenham, United Kingdom: Edward Elgar Publishinghttps://www.elgaronline.com/view/edcoll/9781788112567/9781788112567.00029.xml
Kokkinos, K., and Karayannis, V. (2020). Supportiveness of low-carbon energy technology policy using fuzzy multicriteria decision-making methodologies. Mathematics 8 (7), 1178. doi:10.3390/math8071178
Kokkinos, K., Lakioti, E., Papageorgiou, E., Moustakas, K., and Karayannis, V. (2018). Fuzzy cognitive map-based modeling of social acceptance to overcome uncertainties in establishing waste biorefinery facilities. Frontiers in Energy Research 6, 112. doi:10.3389/fenrg.2018.00112
Kokkinos, K., Lakioti, E., Samaras, P., and Karayannis, V. (2019). Evaluation of public perception on key sustainability indicators for drinking water quality by fuzzy logic methodologies. Desalination and Water Treatment 170, 378–393. doi:10.5004/dwt.2019.24642
Kosko, B. (1992). Neural networks and fuzzy systems: a dynamical systems approach to machine intelligence New York, NY, United States: Prentice-Hall.
Krishankumar, R., Mishra, A. R., Kattur, S. R., Peng, X., Edmundas, K. Z., Cavallaro, F., et al. (2020). A group decision framework for renewable energy source selection under interval-valued probabilistic linguistic term set. Energies 13 (4), 986. doi:10.3390/en13040986
Lak Kamari, M., Isvand, H., and Alhuyi Nazari, M. (2020). Applications of multi-criteria decision-making (MCDM) methods in renewable energy development: a review. Renewable Energy Research and Application 1 (1), 47–54. doi:10.22044/rera.2020.8541.1006
Li, Y., Horsman, M., Wu, N., Lan, C. Q., and Dubois-Calero, N. (2008). Biofuels from microalgae. Biotechnol. Prog. 24 (4), 815–820. doi:10.1021/bp070371k
Lu, W., Alam, M. A., Luo, W., and Asmatulu, E. (2019). Integrating Spirulina Platensis cultivation and aerobic composting exhaust for carbon mitigation and biomass production. Bioresour. Technol. 271 (January), 59–65. doi:10.1016/j.biortech.2018.09.082
Madugu, F., and Collu, M. (2014). Techno-economic modelling analysis of microalgae cultivation for biofuels and co-products. In 1st International Conference on Energy Production and Management in the 21st Century, Ekateringburg, Russia, April, 2014, 1091–1102. doi:10.2495/EQ141022
Markou, G. (2020). “Chapter 21 - overview of microalgal cultivation, biomass processing and application,” in Handbook of algal science, Technology and medicine. Editors: O. Konur (Cambridge, MA, United States: Academic Press), 343–352. doi:10.1016/B978-0-12-818305-2.00021-8
Markou, G., Kougia, E., Kefalogianni, I., Tsagou, V., Arapoglou, D., and Chatzipavlidis, I. (2019). Effect of glycerol concentration and light intensity on growth and biochemical composition of Arthrospira (Spirulina) Platensis: a study in semi-continuous mode with non-aseptic conditions. Appl. Sci. 9 (21), 4703. doi:10.3390/app9214703
Mata, T. M., Martins, A. A., and Caetano, N. S. (2010). Microalgae for biodiesel production and other applications: a review. Renew. Sustain. Energy Rev. 14 (1), 217–232. doi:10.1016/j.rser.2009.07.020
Mehta, A. V., and Nirvesh, S. M. (2020). Extraction of algae biodiesel for power generation and comparison of sustainable fuels using MCDM. Int. J. Ambient Energy 0 (0), 1–11. doi:10.1080/01430750.2020.1796785
Montingelli, M. E., Tedesco, S., and Olabi, A. G. (2015). Biogas production from algal biomass: a review. Renew. Sustain. Energy Rev. 43 (March), 961–972. doi:10.1016/j.rser.2014.11.052
Naeini, M. A., Zandieh, M., Seyyed, E. N., and Seyed, M. S. (2020). Analyzing the development of the third-generation biodiesel production from microalgae by a novel hybrid decision-making method: the case of Iran. Energy 195 (March), 116895. doi:10.1016/j.energy.2020.116895
Naghshbandi, M. P., Tabatabaei, M., Aghbashlo, M., Muhammad Nauman, A., and Iqbal, I. (2020). “Metabolic engineering of microalgae for biofuel production.” In biofuels from algae: methods and protocols, Editors K. Spilling Methods in molecular biology. New York, NY, United States: Springer, 153–172. doi:10.1007/7651_2018_205
Nizami, A. S., Rehan, M., Waqas, M., Naqvi, M., Ouda, O. K. M., Shahzad, K., et al. (2017). Waste biorefineries: enabling circular economies in developing countries. Bioresour. Technol. 241 (October), 1101–1117. doi:10.1016/j.biortech.2017.05.097
Nogueira, N., Nascimento, F. J. A., Cunha, C., and Cordeiro, N. (2020). Nannochloropsis gaditana grown outdoors in annular photobioreactors: operation strategies. Algal Research. 48 (June), 101913. doi:10.1016/j.algal.2020.101913
Noorul Haq, A., and Kannan, G. (2006). Fuzzy analytical hierarchy process for evaluating and selecting a vendor in a supply chain model. Int. J. Adv. Manuf. Technol. 29 (7), 826–835. doi:10.1007/s00170-005-2562-8
Novak, J. D., and Cañas, A. J. (2008). The theory underlying concept maps and how to construct and use ThemTechnical report IHMC CmapTools 2006-01 rev 01-2008. Pensacola, FL, United States: ” Florida Institute for Human and Machine Cognition[Online] URL: http://Cmap.Ihmc.Us/Publications/Researchpapers/Theoryunderlyingconceptmaps.Pdf
Nwokoagbara, E., Olaleye, A. K., and Wang, M. (2015). Biodiesel from microalgae: the use of multi-criteria decision analysis for strain selection. Fuel. 159 (November), 241–249. doi:10.1016/j.fuel.2015.06.074
Papapolymerou, G., Karayannis, V., Besios, A., Riga, A., Gougoulias, N., and Spiliotis, X. (2019). Scaling-up sustainable Chlorella vulgaris microalgal biomass cultivation from laboratory to pilot-plant photobioreactor, towards biofuel. Global NEST Journal 21 (1), 37–42. doi:10.30955/gnj.002777
Peng, L., Fu, D., Chu, H., Wang, Z., and Qi, H. (2020). Biofuel production from microalgae: a review. Environ. Chem. Lett. 18 (2), 285–297. doi:10.1007/s10311-019-00939-0
Raehtz, K. D. (2009). Challenges and advances in making microalgae biomass a cost efficient source of biodiesel. MMG 445 Basic Biotechnology 5 (1), 37–43
Rani, P., Mishra, A. R., Mardani, A., Cavallaro, F., Alrasheedi, M., and Alrashidi, A. (2020). A novel approach to extended fuzzy TOPSIS based on new divergence measures for renewable energy sources selection. J. Clean. Prod. 257 (June), 120352. doi:10.1016/j.jclepro.2020.120352
Rani, P., Mishra, A. R., Raj Pardasani, K., Mardani, A., Liao, H., and Streimikiene, D. (2019). A novel VIKOR approach based on entropy and divergence measures of pythagorean fuzzy sets to evaluate renewable energy technologies in India. J. Clean. Prod. 238 (November), 117936. doi:10.1016/j.jclepro.2019.117936
Roussos, A., Misailidis, N., Koulouris, A., Zimbardi, F., and Petrides, D. (2019). A feasibility study of cellulosic isobutanol production—process simulation and economic analysis. Processes. 7 (10), 667. doi:10.3390/pr7100667
Saaty, R. W. (1987). The analytic hierarchy process—what it is and how it is used. Math. Model. 9 (3), 161–176. doi:10.1016/0270-0255(87)90473-8
Safakish, E., Nayebzadeh, H., Saghatoleslami, N., and Kazemifard, S. (2020). Comprehensive assessment of the preparation conditions of a separable magnetic nanocatalyst for biodiesel production from algae. Algal Research. 49 (August), 101949. doi:10.1016/j.algal.2020.101949
Sakarika, M., and Kornaros, M. (2019). Chlorella vulgaris as a green biofuel factory: comparison between biodiesel, biogas and combustible biomass production. Bioresour. Technol. 273 (February), 237–243. doi:10.1016/j.biortech.2018.11.017
Salim, H. K., Stewart, R. A., Oz, S., and Michael, D. (2019). Drivers, barriers and enablers to end-of-life management of solar photovoltaic and battery energy storage systems: a systematic literature review. J. Clean. Prod. 211 (February), 537–554. doi:10.1016/j.jclepro.2018.11.229
Savvides, A. L., Moisi, K., Katsifas, E. A., Karagouni, A. D., and Hatzinikolaou, D. G. (2019). Lipid production from indigenous Greek microalgae: a possible biodiesel source. Biotechnol. Lett. 41 (4), 533–545. doi:10.1007/s10529-019-02658-6
Savvidou, M. G., Boli, E., Logothetis, D., Lymperopoulou, T., Ferraro, A., Louli, V., et al. (2020). A study on the effect of macro- and micro- nutrients on Nannochloropsis oceanica growth, fatty acid composition and magnetic harvesting efficiency. Plants 9 (5), 660. doi:10.3390/plants9050660
Sindhu, S., Nehra, V., and Luthra, S. (2017). Solar energy deployment for sustainable future of India: hybrid SWOC-AHP analysis. Renew. Sustain. Energy Rev. 72 (May), 1138–1151. doi:10.1016/j.rser.2016.10.033
Singh, J., and Gu, S. (2010). Commercialization potential of microalgae for biofuels production. Renew. Sustain. Energy Rev. 14 (9), 2596–2610. doi:10.1016/j.rser.2010.06.014
Sung, Y. J., Jeong, S., Hong, K., Ko, H., and Sim, S. J. (2020). “Outdoor cultivation of microalgae in a coal-fired power plant for conversion of flue gas CO2 into microalgal direct combustion fuels.” Systems microbiology and biomanufacturing, Berlin. Germany: Springer. doi:10.1007/s43393-020-00007-7
Suprun, E., Oz, S., Stewart, R. A., Panuwatwanich, K., and Shcherbachenko, Y. (2018). An integrated participatory systems modelling approach: application to construction innovation. Systems. 6 (3), 33. doi:10.3390/systems6030033
Tan, J., Kok, Y. L., Nik Meriam, N. S., Tan, R. R., and Promentilla, M. A. B. (2016). Fuzzy analytic hierarchy process (FAHP) for multi-criteria selection of microalgae harvesting and drying processes. Clean Technol. Environ. Policy. 18 (7), 2049–2063. doi:10.1007/s10098-016-1163-6
Tsavatopoulou, V. D., Aravantinou, A. F., and Manariotis, I. D. (2019). Biofuel conversion of Chlorococcum sp. and Scenedesmus sp. Biomass by one- and two-step transesterification. Biomass Convers. Biorefin. doi:10.1007/s13399-019-00541-y
Ubando, A. T., Charles, B. F., Ivan, H. V. G., Michael, A. B. P., and Alvin, B. C. (2020). A fuzzy analytic hierarchy process for the site selection of the philippine algal industry. Clean Technol. Environ. Policy. 22 (1), 171–185. doi:10.1007/s10098-019-01775-0
Ubando, A. T., Ivan, H. V. G., and Kyle Darryl, T. A. (2016a). Analytical hierarchy process with artificial neural network: a case study of algal biofuel production impact prioritization in the Philippines. In 2016 IEEE region 10 conference (TENCON), Singapore, November 2016, 961–965. doi:10.1109/tencon.2016.7848147
Ubando, A. T., Joel, L. C., El-Halwagi, M. M., Culaba, A. B., Promentilla, M. A. B., and Tan, R. R. (2016b). Application of stochastic analytic hierarchy process for evaluating algal cultivation systems for sustainable biofuel production. Clean Technol. Environ. Policy. 18 (5), 1281–1294. doi:10.1007/s10098-015-1073-z
Ullah, K., Garg, H., Mahmood, T., Jan, N., and Ali, Z. (2020). Correlation coefficients for T-spherical fuzzy sets and their applications in clustering and multi-attribute decision making. Soft Computing 24 (3), 1647–1659. doi:10.1007/s00500-019-03993-6
Vinoth Arul Raj, J., Bharathiraja, B., Vijayakumar, B., Arokiyaraj, S., Iyyappan, J., and Praveen Kumar, R. (2019). Biodiesel production from microalgae Nannochloropsis oculata using heterogeneous poly ethylene glycol (PEG) encapsulated ZnOMn2+ nanocatalyst. Bioresour. Technol. 282 (June), 348–352. doi:10.1016/j.biortech.2019.03.030
Viswanath, B., Mutanda, T., White, S., and Bux, F. (2010). “The microalgae – a future source of biodiesel. Dyn. Biochem. Process Biotechnol. Mol. Biol. 4 (No. 1), 37–47
Wang, S.-K., Wang, X., Tian, Y.-T., and Cui, Y.-H. (2020). Nutrient recovery from tofu whey wastewater for the economical production of docosahexaenoic acid by Schizochytrium sp. S31. Sci. Total Environ., 710, 136448. doi:10.1016/j.scitotenv.2019.136448
Wang, W.-M., and Peng, H.-H. (2020). A fuzzy multi-criteria evaluation framework for urban sustainable development. Mathematics 8 (3), 330. doi:10.3390/math8030330
Wang, Z., Pashaei Kamali, F., Osseweijer, P., and John, A. P. (2019). Socioeconomic effects of aviation biofuel production in Brazil: a scenarios-based input-output analysis. J. Clean. Prod. 230 (September), 1036–1050. doi:10.1016/j.jclepro.2019.05.145
Wohlfahrt, J., Ferchaud, F., Gabrielle, B., Godard, C., Kurek, B., Loyce, C., et al. (2019). Characteristics of bioeconomy systems and sustainability issues at the territorial scale. A review. J. Clean. Prod. 232 (September), 898–909. doi:10.1016/j.jclepro.2019.05.385
Yadav, G., Dubey, B. K., and Sen, R. (2020). A comparative life cycle assessment of microalgae production by CO2 sequestration from flue gas in outdoor raceway ponds under batch and semi-continuous regime. J. Clean. Prod. 258 (June), 120703. doi:10.1016/j.jclepro.2020.120703
Yoo, C., Jun, S. Y., Lee, J. Y., Ahn, C. Y., and Oh, H. M. (2010). Selection of microalgae for lipid production under high levels carbon dioxide. Bioresour TechnolConversion Technologies 101(1), S71–S74. doi:10.1016/j.biortech.2009.03.030
Yoon, K. P., and Hwang, C.-L. (1995). Multiple attribute decision making: an introduction CA, United States: SAGE Publications
Zhang, W., Zhao, C., Cao, W., Sun, S., Hu, C., Liu, J., et al. (2020). Removal of pollutants from biogas slurry and CO2 capture in biogas by microalgae-based technology: a systematic review. Environ. Sci. Pollut. Res. Int. 27 (23), 28749–28767. doi:10.1007/s11356-020-09282-2
Keywords: biofuel, clean energy, microalgal biomass, feedstock selection, optimization, fuzzy multi-criteria decision making, nanocatalyst, conversion
Citation: Kokkinos K, Karayannis V and Moustakas K (2021) Optimizing Microalgal Biomass Feedstock Selection for Nanocatalytic Conversion Into Biofuel Clean Energy, Using Fuzzy Multi-Criteria Decision Making Processes. Front. Energy Res. 8:622210. doi: 10.3389/fenrg.2020.622210
Received: 28 October 2020; Accepted: 24 December 2020;
Published: 02 February 2021.
Edited by:
Su Shiung Lam, University of Malaysia Terengganu, MalaysiaReviewed by:
Mukesh Kumar Awasthi, Northwest A and F University, ChinaSimona Bennici, UMR7361 Institut de Sciences des Matériaux de Mulhouse, France
Filiz B. Dilek, Middle East Technical University, Turkey
Ta Yeong Wu, Monash University Malaysia, Malaysia
Copyright © 2021 Kokkinos, Karayannis and Moustakas. This is an open-access article distributed under the terms of the Creative Commons Attribution License (CC BY). The use, distribution or reproduction in other forums is permitted, provided the original author(s) and the copyright owner(s) are credited and that the original publication in this journal is cited, in accordance with accepted academic practice. No use, distribution or reproduction is permitted which does not comply with these terms.
*Correspondence: Konstantinos Moustakas, a29ubW91c3RAY2VudHJhbC5udHVhLmdy