- 1Department Process Systems Engineering, Max Planck Institute for Dynamics of Complex Technical Systems, Magdeburg, Germany
- 2Department Process Systems Engineering, Otto-von-Guericke-University Magdeburg, Magdeburg, Germany
Distillation processes are an essential component of any chemical plant for the separation and purification of condensable mixtures. However, distillation columns account for the largest contribution to the total energy consumption of chemical processes due to their high heat demands. Consequently, there is a strong need for energy efficient column designs to reduce drastically the carbon dioxide emissions and thus to reach the settled climate goals of the chemical industry. In this paper, the FluxMax approach is used to identify energy optimal column designs. The representation of the distillation process by the elementary processes mixing, heating/cooling and phase separation leads to increased degrees of freedom of optimization compared to classical column modeling approaches. In this way, a non-conventional design with minimal energy consumption is identified. The energy-optimized configuration is a column with improved heat transfer between vapor and liquid streams. MESH equations are used for the validation of the FluxMax design, as they are state-of-the-art in process modeling tools. While the new design reduces the energy demand by up to 64% compared to the classical design, additional heat exchange area is required to exploit the energy reduction potential. A multi-objective optimization—energy duty vs. heat exchanger area—is carried out to identify the optimal trade-off between energy demand and heat exchanger area related capital cost. The highly energy-efficient designs identified by the FluxMax approach may be realized in practice by horizontal columns or modularized container solutions.
1. Introduction
In order to achieve the ambitious goal of greenhouse gas emission neutrality within the European Union by 2050, the chemical industry is required to reduce drastically carbon dioxide emissions (European Commission, 2018). In addition to the substitution of raw materials by renewable resources, an increase in material and energy efficiency would reduce greenhouse gas emissions. A critical aspect for the resource efficiency of chemical processes is product purification due to the high energy demand and low energy efficiency of distillation columns (de Koeijer and Kjelstrup, 2000). Especially the backmixing of already separated streams within the column and large temperature differences in reboiler and condenser lead to large energy losses (Halvorsen and Skogestad, 2011). As a consequence, many papers were published in recent years that aim to identify energy optimal distillation column designs. Most of these publications are based either on shortcut methods such as the Fenske-Underwood-Gilliland method (Fenske, 1932; Gilliland, 1940; Underwood, 1949) or on rigorous tray-by-tray model formulations (Biegler et al., 1997). Bausa et al. (1996) introduced a shortcut method using a new energy efficiency criterion by determining the pinch points of both column sections. In this way the minimum energy demand of non-ideal multi-component mixtures can be estimated efficiently. Another shortcut method was proposed by Adiche and Vogelpohl (2011), which allows the design of distillation columns for the separation of azeotropic mixtures. Caballero and Grossmann (2014) provided a broad overview of the optimization of both individual distillation columns and distillation processes. Halvorsen and Skogestad (2011) focused on energy-efficient distillation arrangements such as thermally coupled divided wall columns or multi-effect columns with additional heat transfer by pressure adjustment, which are particularly suitable for the modernization of existing plants. Ledezma-Martinez et al. (2018) investigated crude oil distillation plants and showed that a preflash unit can reduce the heat demand. Jiang et al. (2018b) compared different process intensification strategies for multi-component distillation and investigated the possibility of using synergy effects to design distillation systems that are both energy and economically efficient. In particular, the reduction potential by thermal coupling and simultaneous heat and mass integration is emphasized (Jiang et al., 2018a). An overview of heat integrated distillation columns (HIDiC) is given by Nakaiwa et al. (2003). HIDiCs are a special configuration of multi-effect columns that allow internal heat transfer from the rectification section to the stripping section. In combination with the use of heat pumps this leads to significant energy savings (Agrawal and Yee, 1994). The use of heat pumps is also the focus of the selection scheme proposed by Kiss et al. (2012). The scheme identifies the most promising technologies for a given separation task. Similarly, an enumeration-based framework of Cui et al. (2019) evaluates a variety of multi-effect processes, using shortcut methods to enable quick decision making (Cui et al., 2016). In the literature there are further surrogate models that try to overcome challenges posed by dynamic operations (Schäfer et al., 2019) or the need for global optimization (Keßler et al., 2019). In contrast, Waltermann and Skiborowski (2019) proposed a superstructure approach based on rigorous modeling to identify economically attractive distillation processes. In addition to the strong need for methods that allow a fast and efficient selection of different alternatives, there is also a necessity for process synthesis approaches to generate promising process alternatives. Papalexandri and Pistikopoulos (1996) introduced a modular representation framework using a mass and heat exchange superstructure for process synthesis, which was also applied to distillation processes (Ismail et al., 1999). Shah and Kokossis (2002) invented a synthesis framework that replaced the superstructure with a supertask representation. Since these methods are often only valid for ideal mixtures, Brüggemann and Wolfgang (2004) proposed a rapid screening approach for non-ideal mixtures, in which the distillation alternatives are ordered according to a suitable measure, such as energy consumption. A graph-theoretical approach that represents a chemical process as a network of nodes and edges leading to a mixed-integer optimization problem was presented by Friedler et al. (1992). In another work, this concept was used to solve separation network synthesis problems (Heckl et al., 2010). In contrast, Holiastos and Manousiouthakis (2004) used an infinite dimensional linear programming approach to identify globally optimal distillation network designs. The recently introduced FluxMax approach (Liesche et al., 2019; Schack et al., 2020) enables the optimization of chemical processes across different length scales while avoiding binary decision variables. The main features of FluxMax are simultaneous process design and heat integration as well as effective decoupling of process-based non-linearities by discretization of the thermodynamic state space. The proof-of-concept for application to separation processes was demonstrated in a recent publication (Schack et al., 2019). It was shown that each individual column tray can be represented by the elementary processes mixing, heating, cooling, and phase separation, as shown in Figure 1. In this paper it is shown that the FluxMax approach not only allows the design of classical distillation columns, but also the identification of novel designs with minimum energy consumption by using additional degrees of freedom due to the FluxMax formulation.
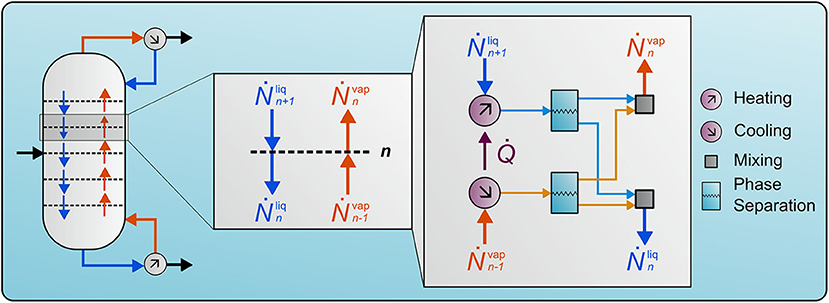
Figure 1. Representation of the overall distillation process as a sequence of elementary processes mixing (gray), heating/cooling (magenta), and phase separation (cyan) as well as single tray fluxes. Adapted from Schack et al. (2019).
Binary methanol-water separation is selected as case study because of exceptional relevance of methanol (CH3OH) in the context of Renewable-to-Chemicals. Methanol is very often regarded as a key molecule for the storage of electrical surplus energy (Surya Prakash et al., 2011; Räuchle et al., 2016; Moioli et al., 2019). The main reasons are: Methanol (i) is already an important platform chemical, (ii) can be used as direct gasoline substitute or can be further processed as Diesel substitute to DME or OME, (iii) is liquid at ambient conditions and thus has beneficial storage properties, and (iv) can be synthesized in a one step reaction from carbon dioxide (CO2) and hydrogen (H2). Furthermore, it was shown that an economically competitive production process can be designed even when using renewable energies (Schack et al., 2018) and under uncertain market situations (Adams et al., 2018).
After an introduction to the FluxMax approach and its formulation for distillation processes in section 2, the case study of the methanol-water separation is introduced in section 3. In addition to the objective functions for identifying the most energy efficient design as well as the stage minimal configuration, an extension of the classical modeling approach based on the MESH equations is derived. This serves as a benchmark of the results obtained by the FluxMax formulation. Finally, the designs obtained with the FluxMax approach and based on the MESH equations are compared in section 4. It is shown that the novel FluxMax design has a signifcantly lower energy demand than distillation column designs based on the regular MESH equations.
2. FluxMax Approach for Distillation Processes
The key idea of the FluxMax approach is to decouple process-based non-linearities from the subsequent flow optimization problem by discretization of the thermodynamic state space. Therefore, the chemical process has to be represented by elementary processes. Figure 1 shows the reprensentation of the distillation process by the elementary processes mixing, heating, cooling and phase separation. In a distillation column the mixing of hot vapor and cold liquid stream results in a distinct mixing temperature of each tray n that enables the phase separation. The FluxMax approach considers each flux separately: The hot vapor stream has to be cooled to meet the tray temperature, and the cold liquid stream has to be heated accordingly. Then, the phase separation of both streams takes place and the new vapor and liquid fractions are mixed together accordingly. The resulting liquid and vapor streams leave tray n and enter the neighboring trays.
The introduction of nodes and edges allows the representation of a chemical process as a directed graph. The FluxMax approach can be divided into three steps as shown in Figure 2: (i) discretization of the thermodynamic state space, (ii) modeling of the transitions between discrete state points, and (iii) simultaneous flow optimization and heat integration. The following introduction of the FluxMax formulation for separation processes is based on a prior contribution (Schack et al., 2019). However, the heat integration section is extended to enable not only the representation of classical column designs but also exploiting additional degrees of freedom due to the consideration of additional heat transfer options. A more comprehensive description of the FluxMax approach can be found in Schack et al. (2020) and Liesche et al. (2019).
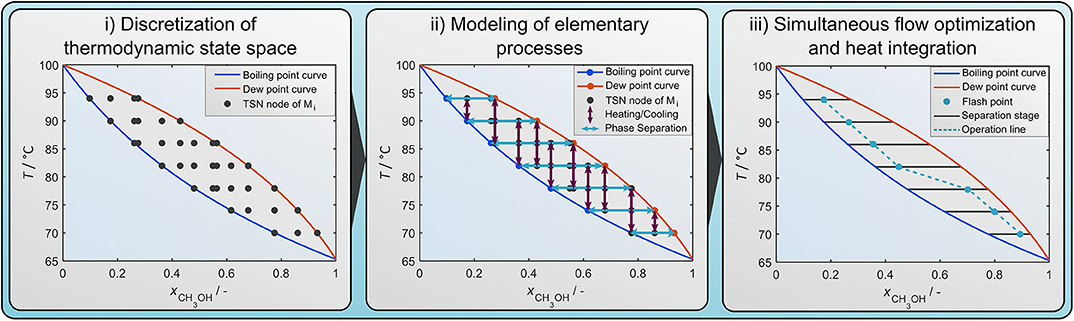
Figure 2. Schematic procedure of the FluxMax approach for distillation column design. Adapted from Schack et al. (2019).
2.1. Directed Graph Representation of Distillation Processes
In case of an isobaric distillation process, the thermodynamic state space is characterized by the thermodynamic coordinates temperature T and molar compositions xα of the components , where is the set of all pure substances. Three types of nodes are introduced: Thermodynamic substance nodes (TSN) , elementary process nodes (EPN) , and utility nodes (UN) , where , and denote the sets of all TSNs, EPNs, and UNs, respectively. A TSN is a discrete state point in the thermodynamic state space (T, x) and thus corresponds to a specific chemical mixture at distinct temperature and composition. The transition from one TSN Mi to another Mi+1 indicates a change of at least one thermodynamic coordinate, which is facilitated by the EPN Ej. For each EPN, a stoichiometric equation with generalized stoichiometric coefficients is introduced, which expresses the molar change of Mi during its transition through Ej. According to Figure 2, two types of EPN are required, corresponding to the elementary processes of heating/cooling and phase separation. It is important to mention that no unique EPN is required for the mixing process as only mixtures with identical thermodynamic coordinates are mixed. Thus the TSNs themselves can be interpreted as mixers. The stoichiometric coefficients for heating and cooling equal unity and minus unity (M1 → M2), respectively, as the number of moles remains constant. The stoichiometric equation for each elementary phase separation process Sj is given as follows:
The stoichiometric coefficients are determined by the following equation system, where xα, F denotes the molar composition of component α of the flash inlet flux, and xα, B, xα, D denote the corresponding compositions at the boiling (B) and dew (D) point curves at flash temperature:
Here, it is assumed that the flash outlet is described by the vapor-liquid equilibrium. In general, non-ideal effects corresponding to efficiency losses or degradation effects could also be included, e.g., by introducing efficiency factors. In addition to the stoichiometric coefficients, a generalized process extent number (PEN) is introduced for each EPN that measures the process extent. Since equals zero if Ej is inactive, the PEN also determines active EPNs in the subsequent optimization problem. The third class of nodes, the UNs Ul, are introduced for two reasons. First, the UNs provide the external heating and cooling duties at predefined temperature levels. Secondly, the UNs enable the indirect heat integration as an integral part of the optimization problem by introducing additional inequality constraints as described in section 2.3.
2.2. Conservation Laws of the Distillation Process
Steady state behavior is assumed, which results in no accumulation of mass and/or energy within the nodes. Table 1 shows the mass- and energy balances for the three nodes introduced above.
The TSNs distribute mixtures with the same thermodynamic state within the chemical process network. Consequently, a TSN is connected by internal mass fluxes corresponding to the input or output fluxes of EPNs, and by external fluxes that correspond to initially provided reactants or the final product . No energy balances are required for TSNs as the thermodynamic state of all fluxes interacting with Mi is identical by definition, i.e., a mixture of the same composition but different temperature results in different TSNs. The chemical transition within the thermodynamic state space takes place via the EPNs. As a result, energy balances are formulated in addition to mass balances. The molar ratio of outlet and inlet fluxes is determined by the generalized stoichiometric coefficient . The heat fluxes and connect an EPN to an UN and are dependent on the specific heating or cooling duty. These specific duties are calculated a priori, e.g., by non-linear equations of state. In addition, and correspond to internal heat fluxes that directly link two EPNs Ej and Ei. The direct heat transfer between EPNs is only considered in case of direct heat integration as introduced in the following section. While the superscripts of the fluxes denote the origin node, the subscript denotes the destination node. The utility nodes do not interact with mass fluxes, but only with heat fluxes. Besides the internal fluxes connecting EPNs and UNs, external heat fluxes are introduced, corresponding to the external heating and cooling fluxes of the utility node Ul.
2.3. Heat Integration Model
In this work the heat integration model introduced by Schack et al. (2020) is used. There, two distinct approaches—direct and indirect heat integration—were proposed. Indirect heat integration refers to the use of UNs at a predefined temperature that provide the internal heat transfer. In contrast, the direct approach considers the direct coupling of hot and cold streams as illustrated in Figure 3. Two inequalities are introduced to limit the heat fluxes transferred from hot streams to the cold stream or the cold utility, respectively, (Equation 4a) or from hot the utility/stream toward the cold streams (Equation 4b), respectively. Here, , and denote the inlet and outlet temperatures of the corresponding cold or hot streams, and ΔTmin the minimum temperature difference between both streams. The utilities are assumed to be heating or cooling reservoirs at a constant temperature. As a consequence, the in- and outlet temperature of i-th utility is set to the same utility temperature . In addition, and denote the m-th row of the set of all possible permutations and , respectively. These sets contain all possible combinations of interacting hot and cold streams for which heat transfer constraints must be formulated as derived in Schack et al. (2020).
The inequalities introduced in Equation (4) do not take into account in which order a hot stream transfers its heat to distinct cold streams if more than one partner stream is possible. Likewise applies to cold streams that are connected to more than one hot stream. It becomes obvious that the available heat that can be transferred to the second cold stream is lower than to the first cold stream since the energetic content of the hot stream is steadily decreasing. Therefore, two additional inequalities are introduced to account for the actual energy content.
The choice of the heat integration approach—direct or indirect—as well as the number of utilities affect the degrees of freedom of the subsequent optimization. In Schack et al. (2019), it was demonstrated how the FluxMax approach enables the design of classic distillation columns by introducing one dedicated UN for each discrete flash temperature. For an assumed minimum temperature driving force of ΔTmin = 0, a single column tray is represented as illustrated in Figure 3A. The magenta arrows in Figure 3A indicate the internal heat transfer from hot vapor stream (red arrow) toward cold liquid stream (blue arrow) via the corresponding UN.
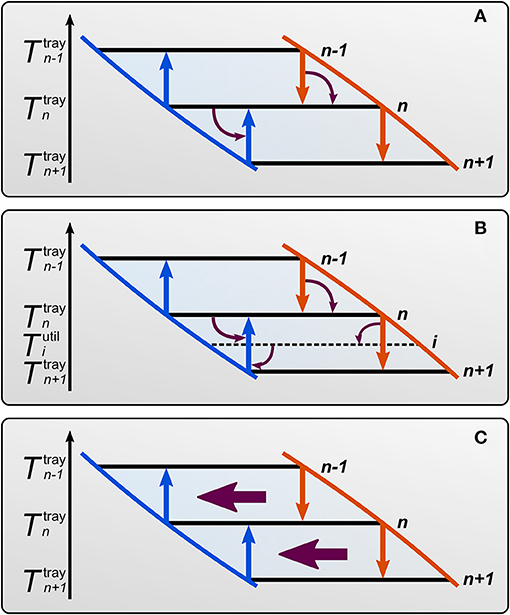
Figure 3. Schematic illustration of the heat integration model: For classic distillation columns by consideration of one utility for each tray (A), and for the exploitation of additional degrees of freedom: Applying indirect heat integration (B), and direct heat integration (C).
Figure 3B shows how the consideration of additional utilities—with predefined temperatures between tray temperatures—increases the heat transfer options and thus the degrees of freedom. The additional UNs enable the heat transfer between vapor and liquid flows entering and leaving the same two trays n and n+1 as well as the classical transfer between vapor and liquid flow entering the same tray n. The consideration of an infinite number of utilities is equivalent to the direct heat integration approach, where the hot vapor stream can transfer its heat directly to the cold liquid stream as illustrated in Figure 3C. While the direct approach leads to an increased computational effort, the calculation of the heat integration potential corresponds to the reduction potential obtained by pinch analysis—and thus to the maximum thermodynamically achievable—as shown in Schack et al. (2020). In contrast, the indirect approach limits the computational complexity, but is only an approximation of the thermodynamically possible energy reduction potential.
3. Case Study
In this section the case study—the methanol-water separation—is introduced, followed by the derivation of the resulting optimization problems for both the FluxMax as well as the MESH formulation.
3.1. Methanol-Water Separation
Equation (6) shows the reaction equation of direct synthesis from carbon dioxide and water, which can be regarded as a combination of the reverse water-gas-shift reaction and the carbon monoxide synthesis process (Ott et al., 2012).
While the obvious advantage lies in the direct use of the greenhouse gas CO2, the coupled by-production of water (H2O) is an unavoidable shortcoming that requires a downstream separation of the reactor output stream, even if all other possible side reactions—such as the production of higher alcohols or ethers (Ott et al., 2012)—are neglected. The unconverted gaseous reactants can be easily separated and recycled by cooling the reactor output stream and condensing the methanol-water mixture. However, a 50/50 mixture of methanol and water is fixed by the chemistry and must be further separated.
This separation task constitutes the case study of the present work. Whereas previous work (Schack et al., 2019) emphasized the feasibility and applicability of the FluxMax approach to the distillation design problem, the focus of this publication is the identification of non-conventional distillation designs with increased energy efficiency. The additional degrees of freedom provided by the consideration of additional heat integration possibilities introduced in section 2.3 are used.
The specifications of the scenario under consideration are listed in Table 2, assuming that the products are saturated liquid or vapor streams.
Following the three step procedure of the FluxMax approach introduced in section 2 the thermodynamic state space is discretized. The relevant thermodynamic state space for the isobaric binary separation of methanol and water is defined by the temperature T and the molar fractions of methanol xCH3OH and water xH2O. In addition, the entire thermodynamic state space is further limited by the vapor-liquid equilibrium which determines the attainable region. Assuming ideal conditions in both liquid and vapor phase, the boiling point curve which bounds the attainable region is computed as follows:
where p is the operation pressure and the saturation pressure of component α. The corresponding dew point curve is calculated by the equilibrium condition (Equation 8) and the definition of the equilibrium constant Kα: = yα/xα.
The saturation pressure depends on the temperature and can be expressed by the semi-empirical Antoine equation:
The Antoine parameters Aα, Bα, and Cα for pure water and methanol are listed in Table 3. They are valid if the pressure is given in bar and the temperatures in K.
3.2. Formulation of Optimization Problems
Based on the model derived in the previous chapter, the optimization problem is derived in this section. In addition, the design optimization problem based on the MESH equations is introduced for validation purposes.
3.2.1. FluxMax Formulation
The FluxMax formulation results in a linear feasible region due to the discretization of the thermodynamic state space (Liesche et al., 2019; Schack et al., 2020). If the objective function is also linear in terms of the fluxes (decision variables), the problem can be solved as a purely linear optimization problem (LP). The LP is given in Equation (10).
The vector of decision variables contains all fluxes, namely mass fluxes , heat fluxes , and the generalized process extent numbers .
The mass and energy balances of TSNs, EPNs, and UNs (see Table 1) act as equality constraints and are expressed by the coefficient matrix Aeq and the vector of right-hand sides beq. To limit the maximum amount of heat internally transferred, Equations (4) and (5) provide the inequality constraints, that are expressed by the coefficient matrix of inequalities Aiq and corresponding right hand sides biq. In addition, the lower φlb and upper bounds φub correspond to non-negativity constraints as well as external feed and product specifications according to the case study introduced in section 3.1.
The FluxMax approach is applied to two different objective functions: (i) minimization of number of separation trays (fI), and (ii) minimization of the total energy duties (fII). The number of trays is minimal if the liquid reflux stream from the condenser to the top tray is maximized. According to the FluxMax formulation, this liquid reflux stream corresponds to the sum of all liquid fluxes that are heated at the lowest tray temperature under consideration by means of the heater .
The second objective function fII seeks to identify the most energy efficient column design and can be written as sum of external heating and cooling duties , which are desired to be minimized:
A key parameter of distillation columns is the reflux ratio r,which is defined as ratio between liquid reflux stream from the condenser and the total top product stream Ṅtop:
3.2.2. MESH Formulation
The results that are obtained from the FluxMax formulation of the column design problem are benchmarked with results that are obtained from the solution of the MESH equations. With the MESH equations, the distillation column design is addressed using a tray-by-tray formulation (Biegler et al., 1997). The characteristic of the MESH equations is the solution of mass (M), equilibrium (E), summation (S), and energy balance in its enthalpy form (H) for each tray. Each tray is considered an equilibrium tray, which means that vapor and liquid flows leave a tray at thermodynamic equilibrium. Figure 4A illustrates the flows interacting with trays n and n+1. An overall distillation column for a binary mixture with reboiler and condenser duties has five degrees of freedom that are typically fixed using the feed condition as well as top and bottom product specifications. If heating and cooling duties can be theoretically provided at each tray, the degrees of freedom are increased by ntray−2 where ntray is the total number of trays including reboiler and condenser trays. Thus the tray temperatures can be set to any desired values.
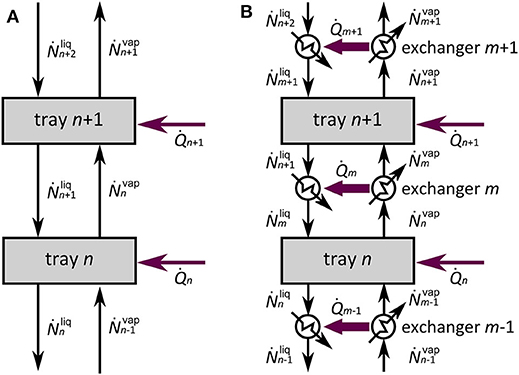
Figure 4. Schematic illustration of tray n and neighboring tray n+1 for the regular formulation of MESH equations (A) and for the case where intermediate heat exchangers m−1, m and m+1 are considered (B).
Optimizing the tray temperature for a given feed and top/bottom product specification leads to the optimization problem in Equation (15) where all mass fluxes between trays, tray duties and tray temperatures are decision variables that are contained in the vector . is the vector of feed mass fluxes that is not illustrated in Figure 4. The objective function is the sum of external heating and cooling requirements (Equation 14) of all trays n which is a linear objective function.
However, the equality constraints that contain the tray models are non-linear equations as derived in the Supplementary Material of this contribution. The resulting non-linear programming problem (NLP) is given as:
The system of the MESH modeling equations is contained in h(φ) and the lower and upper bounds correspond to non-negativity constraints and external feed and product specifications.
As an additional benchmark for the FluxMax results, the MESH equations are extended with nHX = ntray−1 heat exchangers in order to enable heat exchange between vapor and liquid fluxes between trays as depicted in Figure 4B and derived in the Supplementary Material. Mass and energy balances for the heat exchangers are added to the system of modeling equations h(φ) and the heat flows of the heat exchangers are added as new decision variables. Furthermore, feasibility constraints for the inter-tray heat exchange must be fulfilled:
These feasibility constraints are added to the optimization problem as inequality constraints g(φ). They ensure that the temperature from the bottom to the top of the column is decreasing monotonically.
4. Results
The presented results are divided into three parts. The first part is the application of the FluxMax approach to classical distillation design tasks. Particularly the influence of the selected discretization is analyzed. In contrast, the second part emphasizes the exploitation of additional degrees of freedom by the FluxMax formulation. It is shown that an improved heat transfer between vapor and liquid streams drastically reduces the energy demand. Since the exploitation of the energy reduction potential requires an improved heat transfer, the third part evaluates implementation of the additional heat transfer and the influence of the minimum driving force of the heat transfer on the required heat exchange area.
4.1. Application to Conventional Distillation Design Tasks
4.1.1. Optimal Design of Distillation Processes
Figure 5 depicts the equilibrium diagram as temperature T vs. molar fraction xCH3OH of the low boiler methanol for a temperature discretization of 17 stages. The heat integration model for identifying classical distillation columns according to Figure 3A is used. The vapor-liquid area between boiling and dew curve is the attainable region (gray area) of the thermodynamic state space for the column design: All EPNs/TSNs are located in this region. The molar fraction xH2O = 1−xCH3OH of the missing component water can easily be calculated. The attainable region is enclosed by the boiling point curve (blue) and the dew point curve (red). In addition, the inlet and desired product specifications are marked as magenta and green dots. The black dots in Figure 5 correspond to the TSNs and thus to the discrete points in the thermodynamic state space, which are connected by the elementary processes heating or cooling in vertical direction and phase separation in horizontal direction (see also Figure 2). The left-hand side of Figure 5 shows the actual state space representation of the optimal pathways according to the considered objective function. The active elementary processes along the different TSNs from the feed TSN—magenta point—to the lower and upper product TSNs—green points—are indicated by the cyan lines.
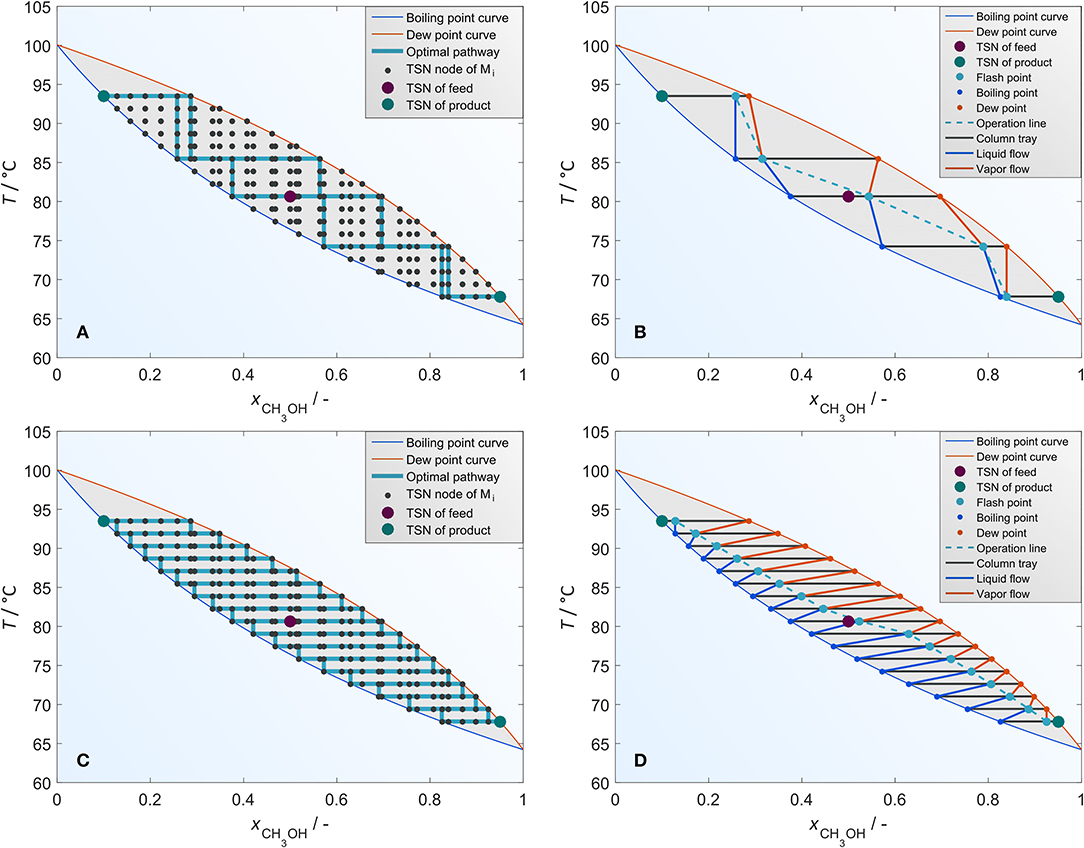
Figure 5. Optimal pathway within the thermodynamic state space for the two objective functions (Equations 11, 12; left-hand sides), and corresponding operation line for a temperature discretization of 17 (right-hand sides); minimization of number of trays (A,B), and total energy minimization (C,D).
Once the optimal solution has been found, all liquid and vapor flows entering or leaving a particular tray and the corresponding molar composition are known. This allows the calculation of the actual mixing points (Figures 5B,D cyan points) at each tray. The resulting operation lines (dashed cyan line) are shown on the right-hand side of Figure 5. In addition, the corresponding liquid (red line) and vapor (blue line) flows are shown.
The two upper diagrams (Figures 5A,B) show the optimal results for the first objective: Minimizing the number of trays (Equation 11). The optimization algorithm reveals that the separation task in this scenario (Table 2) requires at least five separation trays. In order to achieve the desired product purification with the minimum number of trays, stages with as different temperatures as possible are selected. The resulting operation line has the shape of a zigzag curve, with the slopes between the first two or the last two trays being as steep as possible. While the number of trays is minimal, the energy duty (534.0 kW/molfeed) and the reflux ratio (7.83) are very high. The beginning of each distillation column design is the calculation of the minimum number of stages. This is typically achieved using the Fenske equation (Fenske, 1932), but the results in Figure 5A show, that the FluxMax approach returns the same minimum number of trays.
The lower two plots (Figures 5C,D) correspond to the most energy efficient column design. As expected, the optimal path is found when phase separation occurs at all possible discrete temperature levels. It can be seen that starting from the TSN of the feed, the mixture is split into the corresponding saturated equilibrium states, which are then cooled or heated to the next possible temperature considered, which represents a different column tray. The resulting operation line in Figure 5D is located in the stripping section of the column near the boiling point curve and in the rectifying section near the dew point curve. The total energy demand is 36.0 kW/molfeed and the reflux ratio is 0.25.
Both results are consistent with the expectation that the total energy demand is inversely proportional to the number of trays. In the following, the FluxMax approach is utilized using additional degrees of freedom. It is shown that improved heat exchange leads to the same energy reduction as an increase in the number of stages. To illustrate the effect for a different number of stages, it is necessary to force the optimizer to select a certain number of trays. However, unlike the MESH equations, FluxMax formulations are based on a flux optimization on a predefined temperature grid. There the energy minimum is always found for the maximum number of stages, which corresponds to temperature discretization as can be seen in Figure 5C. In order to be able to use the same discretization—which guarantees the comparability of the results—and still force the optimization algorithm to select only a limited number of trays, integer variables yn are introduced below for each tray. In this way, the actual number of selected trays ntray is limited by the following inequality:
Although the remaining FluxMax formulation remains the same, it is important to note that the following figures are based on a mixed integer linear program (MILP) solved with the efficient MILP solver SCIP (Gleixner et al., 2018).
Figure 6 illustrates again the operation lines for the most energy efficient design identified for a discretization of 17 temperature levels. However, unlike Figure 5, the newly added constraints limit the selectable levels to 9 (Figure 6A), and 11 (Figure 6B), respectively. Interestingly, the optimization algorithm selects the stages so that more trays are active near the feed point and only a few in the remaining stripping and rectifying section. By selecting trays with smaller temperature differences in the feed section of the column, a better approximation to the optimal operating line for the unlimited case is achieved (Figure 5D). Again, the calculated results correspond well with the known temperature profiles of classical distillation columns (Biegler et al., 1997).
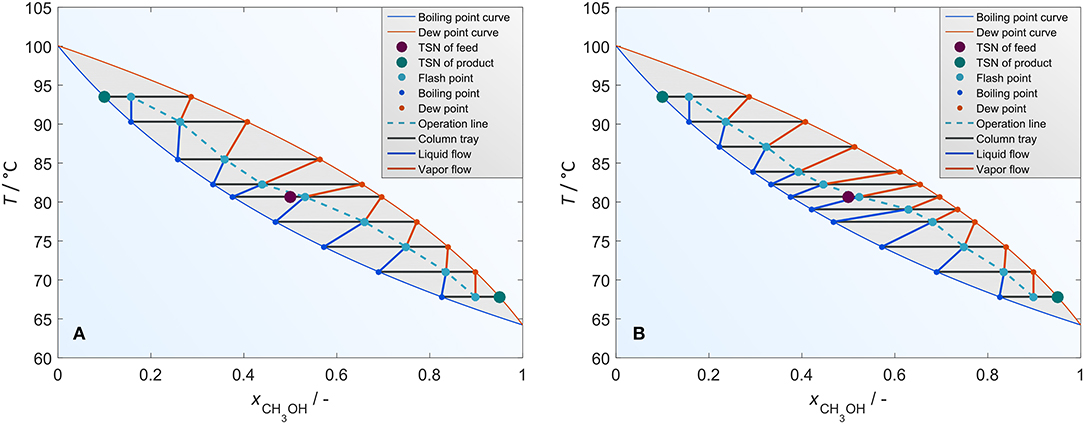
Figure 6. Operation line for a temperature discretization of 17 if the number of selectable tray stages is limited to 9 (A), and 11 (B).
4.1.2. Influence of the Temperature Discretization
While the benefit of the FluxMax formulation is the decoupling of the non-linearities from the optimization step by discretization of the thermodynamic state space, the disadvantage is the grid dependence of the obtained results. Therefore, in the following the energy demand is analyzed as a function of different temperature discretizations, corresponding to the maximum number of trays. Figure 7 shows the influence of the selected temperature discretization on the energy demand for the classical column design task as shown in Figure 3A. For each discretization—5, 9, 17, 33—the temperatures are distributed equidistantly between the desired top and bottom product temperatures. This ensures two prerequisites: i) a separate tray is always considered at the assumed feed temperature, and ii) for each finer discretization, the same temperatures of the coarser grids are considered in addition to the newly added temperatures. While the first condition ensures that no pre-heating or pre-cooling of the feed is required, the second condition allows a fair comparison of the different cases. In addition to the results obtained by the FluxMax formulation, the energy duties calculated by classical MESH equations are shown in Figure 7.
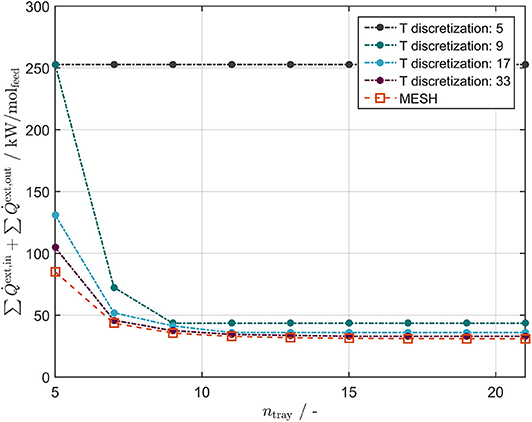
Figure 7. Influence of number of separation stages on the total energy duty if no additional heat transfer is considered. Illustrated are the results of the FluxMax formulation considering different temperature discretizations (5, 9, 17, and 33 temperature levels) as well as of the classical MESH formulation.
As expected, Figure 7 shows that the calculated total energy demand depends strongly on the discretization. The finer the grid, the more the calculated energy duties correspond to the MESH results. In case of the coarsest discretization of only 5 temperature steps, the calculated energy demand does not depend on the limitation of the selectable stages, because the maximum number of trays is only 5. In the other cases, however, the grid dependency decreases with increasing number of actually selected trays. Even with a discretization of 9 temperature levels, the FluxMax results are quite close to the MESH results if the number of selected trays is bigger than 7. In contrast, the selection of an appropriate grid is crucial if the number of trays is low. However, it is important to note that the accuracy of the FluxMax formulation can be improved not only by finer grids, but also by the selected temperatures. Both formulations have the same energy requirement when the actual tray temperatures calculated with the MESH equations are used as input for the FluxMax formulation. The discretization procedure with adding new temperature levels is bound to this example. Separation tasks with for example azeotropic points or other non-ideal behavior may require different discretization schemes.
4.2. Energy Efficient Designs by Additional Heat Transfer
After presenting the general applicability of the FluxMax approach to separation tasks, the following shows the influence of the additional heat transfer options as shown in Figures 3B,C. Within the FluxMax approach, each mass and heat flux is modeled separately. The formulation thus allows the identification of non-conventional configurations. In the case of the distillation column, the consideration of improved heat transfer between liquid and vapor streams—enabled either by direct or indirect heat integration—results in a lower energy consumption compared to classical column design. Figure 8 illustrates the energy requirements for the configurations with improved heat integration. A minimum temperature difference of ΔTmin = 0 K is assumed. In this way, the theoretically maximum amount of heat that can be transferred is evaluated. Later, the influence of different minimum driving forces will be examined in more detail. Furthermore, the energy requirements calculated with the MESH equations are presented as a benchmark. For indirect heat integration, the discretization, which corresponds to the number of utility nodes, plays a role: An additional utility node is introduced at the intermediate temperature of two neighboring separation stages. Thus, the more auxiliary nodes are considered, the more additional heat transfer options are available, resulting in lower energy requirements. In any case the energy demand is lower compared to non-heat integrated columns (see Figure 7). Compared to the benchmark case, the largest energy saving potential is observed when the maximum number of stages is small (≤ 9). In this range, even rather coarse discretizations lead to more energy efficient configurations: Even a temperature discretization of 9—corresponding to 17 utility levels—result in a lower energy consumption as the classical MESH design. For a larger number of stages, however, the optimizer cannot find better configurations because the discretization is limited.
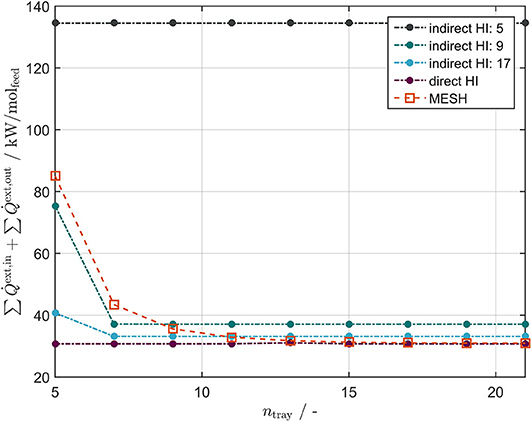
Figure 8. Influence of number of separation stages on the total energy duty if additional heat transfer is considered. Illustrated are the results of the FluxMax formulation considering different temperature discretizations (5, 9, and 17 temperature levels) as well as of the classical and extended MESH formulations as a benchmark.
However, if direct heat integration is considered, which corresponds to an infinite number of utility nodes, the calculated results are independent of the temperature discretization. For any number of stages, the same theoretically feasible minimum energy requirement is calculated at 30.7 kW for each mole of feed flux, resulting in energy savings of up to 64% compared to classical MESH designs. The calculated heat integration potential has also been validated using the extended MESH equations, which result in the same results as the direct heat integration approach. Since the energy demand of standard columns decreases rapidly with an increasing number of stages, the potential for columns with improved heat transfer decreases with each additional tray.
If the direct heat integration result is taken as the minimum energy requirement and thus the most energy efficient configuration, it is evident that an improved heat transfer (with ΔTmin = 0 K) corresponds to a classical column design with an infinite number of trays. Consequently, there is a trade-off between additional separation stages—corresponding to the column area—and additional heat transfer area. Before discussing the influence of considering different minimum driving forces ΔTmin on the required heat transfer area, Figure 9A shows the additional heat transfer within the column schematically. A two-phase heat exchanger transfers the heat provided by the hot vapor stream flowing between two stages to the cold liquid stream flowing in the opposite direction. The practical realization of two-phase heat exchangers is beyond the scope of this work, therefore the interested reader is referred to adequate literature (Bejan and Kraus, 2003; Kuznetsov, 2018). In contrast to the MESH formulation, the FluxMax formulation is not associated to a particular design. As a consequence, besides the representation as classic distillation column (Figure 9A), the resulting configuration can also be interpreted as a series of heat integrated flash drums with intermediate heat exchange (Figure 9B). The interpretation as flash drums allows a simpler technical implementation as well as the modular design of the separation system. The latter is particularly interesting in the context of Power-to-X, where smaller decentralized plants can be built to make efficient use of excess electrical energy. According to Figure 8, a sequence of a minimum number of flash drums is sufficient to reduce the total energy demand drastically.
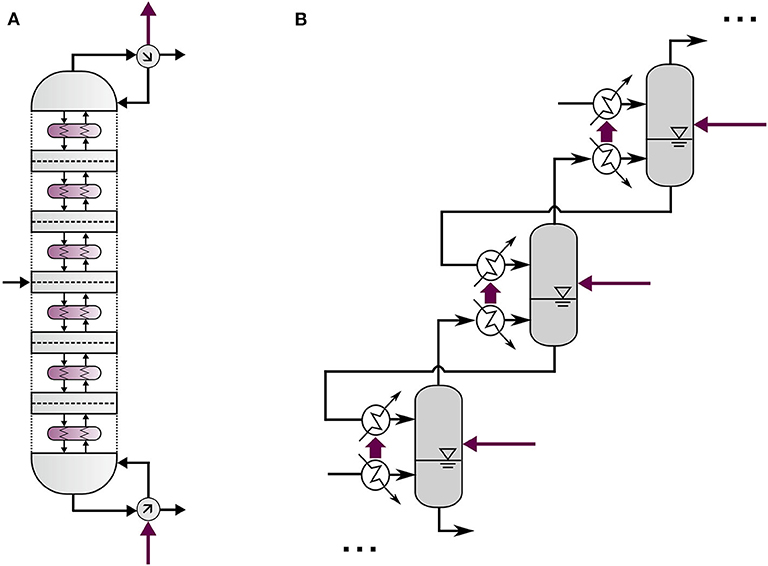
Figure 9. Schematic illustration of a column with intermediate heat exchangers (A), and corresponding interpretation as a series of heat integrated flash drums (B).
4.3. Implementation of the Improved Heat Transfer
4.3.1. Influence of the Minimum Driving Force ΔTmin
In all calculations up to this point, a minimum driving force of ΔTmin = 0 K is assumed, which is technically not possible, since it would require an infinitely large heat exchange area. To obtain finite areas, a minimum driving force ΔTmin>0 K must be present. Figure 10 illustrates the influence of the assumed minimum temperature difference ΔTmin on the actual energy demand. In addition to the results obtained by considering four different minimum driving forces (ΔTmin = 0, 1, 2, 3 K), the non-heat integrated case is presented as a benchmark. All results are based on a discretization of 17 temperature levels.
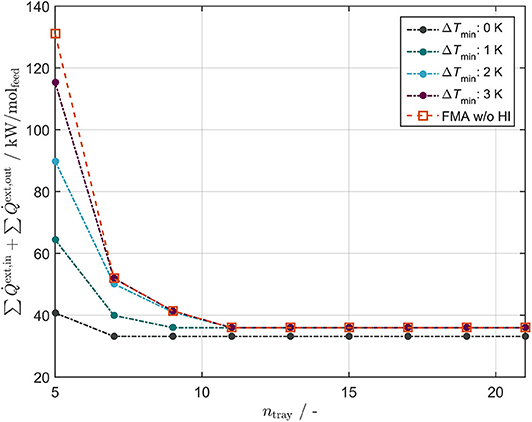
Figure 10. Influence of the selected minimum temperature difference ΔTmin of the additional heat transfer. Illustrated are the heat integrated results for ΔTmin = 0, 1, 2, 3 K as well as the non-integrated result as a benchmark for discretization of 17 temperature stages.
The black curve (ΔTmin = 0 K) and the red benchmark curve (non-heat integrated case) are the same as the corresponding curves in Figures 7, 8. They correspond to both the best and worst case scenarios for a given temperature discretization. Two observations can be made: (i) the bigger the minimum driving force becomes, the bigger the energy duties become and the closer the curve becomes to the benchmark curve, and (ii) for a certain number of stages there is no heat integration potential for ΔTmin>0 K. Both observations indicate for the case study under consideration that, on the one hand, there is an enormous energy saving potential for a small number of stages, but that this potential can only be used for very small driving forces. Even at very small minimum temperature differences of ΔTmin = 3 K, which are already very small compared to the usually technically applied minimum driving forces of ΔTmin>10 K, the potential almost disappears. However, there may be technical solutions that can exploit the identified energy saving potential. A possible realization of the calculated design could be the concept of horizontal columns, where the contact time between vapor and liquid streams is increased and adjusted by the angle of the column (Kim et al., 2013; Jang and Kim, 2015).
4.3.2. Evaluation of the Required Heat Exchanger Area
The previously presented results show that improved heat transfer reduces drastically the energy demand of distillation columns. However, the heat has to be transferred across very small temperature differences, which results in a low driving force for the heat transfer and thus large heat exchange areas. The heat exchange area that is required for each EPN Ej depends on the sum of received heat fluxes and the corresponding mean logarithmic temperature differences and of the internal heat transfers between EPNs Ei as well as UNs Ul toward Ej. Furthermore, the transfer area is inversely proportional to the heat transfer coefficient kHX:
The heat transfer coefficient kHX depends on the fluids and the type of heat exchanger. A constant value of 3000 W/m2K is assumed for the two-phase heat exchange, which corresponds to a vapor-liquid heat transfer in a spiral plate heat exchanger (Roetzel and Spang, 2010). It is evident that there is a trade-off between an increase in internal heat flows—corresponding to a reduction in operating costs—and more heat transfer area corresponding to an increase in capital costs. A multi-objective optimization is performed by applying the ϵ-constraint method (Miettinen, 1998). Therefore, one of the competing targets (Equation 18) is added as an additional constraint to the optimization problem. As a result, the Pareto-optimal point of the second objective (Equation 12) is calculated. By systematic variation of the heat exchanger area, the Pareto curves for distinct number of stages and a minimum driving force ΔTmin = 0 are generated and plotted in Figure 11.
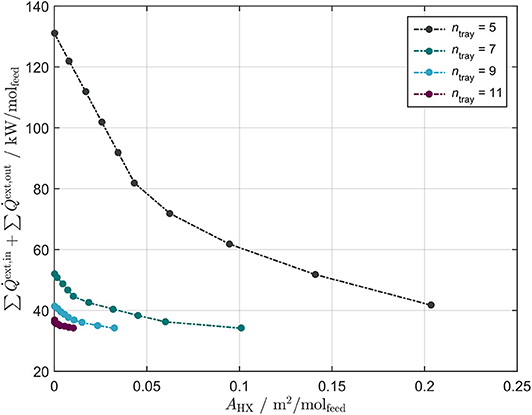
Figure 11. Pareto curve of the two competing objectives illustrating the trade-off between minimization of total energy duty and minimization of additional heat transfer area for different number of separation stages: 5, 7, 9, and 11 stages.
The Pareto curves denote the limits at which an improvement of one objective is only possible if the second objective is simultaneously worsened. According to Figure 8, it can be seen that the energy saving potential decreases with an increasing number of stages. Interestingly, the slope of the curves is steepest for small heat exchanger areas. This means that a rather small heat exchanger area leads to a rather large energy saving potential, especially for small columns with a small number of stages. After the first steep decline, the curves become flatter, which corresponds only to a small energy reduction. Consequently, the required area is very high if the full integration potential is to be exploited at the minimum number of stages. The heat transfer area required to save a separation tray also becomes smaller for larger columns. While a heat exchange area of almost 0.14 is needed to achieve the same energy demand for 5 stages compared to a column with 7 stages, only 0.025 is sufficient to save two additional trays.
Figure 11 also summarizes the main results of this paper. The results show that an improved heat exchange reduces the energy demand up to 64% compared to classic distillation columns, and thus can contribute to the reduction of carbon dioxide emissions. For the case study under consideration, however, heat transfer across very small temperature differences is required, which result in additional heat exchanger areas up to 0.21 m2 per mole feed stream. Nevertheless, the separation of different mixtures—with more distant boiling points—could have a greater potential for larger temperature differences and thus less heat exchange area.
5. Conclusion
This paper proposes an approach to design distillation columns with significantly reduced energy requirements. In order to achieve optimal designs, the FluxMax approach decouples process-based non-linearities from the optimization problem by discretizing the thermodynamic state space and representing the distillation process by three elementary processes: Mixing, heating/cooling and phase separation. The versatility of the FluxMax approach is demonstrated by applying two different objective functions: Minimizing (i) the number of trays, and (ii) the energy demand. As a consequence of the simultaneous consideration of heat integration by introducing inequality constraints, the design space is increased compared to classical design methods based on the MESH equations. The case study under consideration is the methanol-water separation, which is a key step of the methanol production process that is of high interest in the context of Renewables-to-Chemicals. A non-conventional design has been identified that outperforms classical distillation column designs based on the MESH equations. It is shown that an improved heat transfer between hot vapor streams and cold liquid streams—with the consequence of additional heat exchange area—reduces the energy consumption in the same way as additional separation stages of classical columns. In this way, the optimized design based on the FluxMax formulation reduces the energy consumption by up to 64% compared to conventional designs. The trade-off between the reduction in energy demand—corresponding to lower operating costs—and the increase in heat exchange area—corresponding to higher capital costs—is analyzed through multi-objective optimization. The MESH equations are extended and used to validate the FluxMax design and prove that the achieved results are thermodynamically consistent. The results underline the strength of the FluxMax approach to design distillation processes with increased degrees of freedom, which allows the identification of highly energy efficient designs.
However, the presented results show that heat must be transferred across very small temperature differences to fully exploit the identified energy reduction potential. Nevertheless, it is possible that separation tasks of other mixtures—with more different boiling points of the pure components—also have a high potential for higher temperature differences, which would lead to a lower requirement for heat exchange area. The practical implementation of the highly energy-efficient designs by using horizontal columns is also possible, which reduces the surface area by improving the heat transfer by extending the contact time of vapor and liquid streams. The identified energy potential is of particular interest for applications with a limited number of trays, e.g., decentralized or modularized container systems, as the FluxMax design can be represented as a cascade of heat-integrated flash drums with internal heat exchange.
Data Availability Statement
The original contributions presented in the study are included in the article/Supplementary Material, further inquiries can be directed to the corresponding author/s.
Author Contributions
DS and GL developed the design methodology. DS extended the methodology to distillation processes, performed the main calculations, and wrote the manuscript. AJ supported the calculations. GL contributed to the calculations and supported the writing of the manuscript. KS supervised the project. All authors contributed to the article and approved the submitted version.
Conflict of Interest
The authors declare that the research was conducted in the absence of any commercial or financial relationships that could be construed as a potential conflict of interest.
Supplementary Material
The Supplementary Material for this article can be found online at: https://www.frontiersin.org/articles/10.3389/fenrg.2020.00134/full#supplementary-material
References
Adams, T. A. II., Thatho, T., Le Feuvre, M. C., and Swartz, C. L. E. (2018). The optimal design of a distillation system for the flexible polygeneration of dimethyl ether and methanol under uncertainty. Front. Energy Res. 6:41. doi: 10.3389/fenrg.2018.00041
Adiche, C., and Vogelpohl, A. (2011). Short-cut methods for the optimal design of simple and complex distillation columns. Chem. Eng. Res. Design 89, 1321–1332. doi: 10.1016/j.cherd.2011.02.014
Agrawal, R., and Yee, T. F. (1994). Heat-pumps for thermally linked distillation-columns - an exercise for argon production from air. Indus. Eng. Chem. Res. 33, 2717–2730. doi: 10.1021/ie00035a023
Ambrose, D., and Sprake, C. H. S. (1970). Thermodynamic properties of organic oxygen compounds XXV. Vapour pressures and normal boiling temperatures of aliphatic alcohols. J. Chem. Thermodyn. 2, 631–645. doi: 10.1016/0021-9614(70)90038-8
Bausa, J., Watzdorf, R. v., and Marquardt, W. (1996). Minimum energy demand for nonideal multicomponent distillations in complex columns. Comput. Chem. Eng. 20, S55–S60. doi: 10.1016/0098-1354(96)00020-8
Biegler, L. T., Grossmann, I. E., and Westerberg, A. W. (1997). Systematic Methods of Chemical Process Design. Upper Saddle River, NJ: Prentice Hall.
Brüggemann, S., and Wolfgang, M. (2004). Rapid screening of design alternatives for nonideal multiproduct distillation processes. Comput. Chem. Eng. 29, 165–179. doi: 10.1016/j.compchemeng.2004.07.009
Caballero, J. A., and Grossmann, I. E. (2014). Optimization of Distillation Processes. Boston, MA: Academic Press. doi: 10.1016/B978-0-12-386547-2.00011-9
Cui, C., Xi, Z., Liu, S., and Sun, J. (2019). An enumeration-based synthesis framework for multi-effect distillation processes. Chem. Eng. Res. Design 144, 216–227. doi: 10.1016/j.cherd.2019.02.018
Cui, C., Yin, H., Yang, J., Wei, D., Sun, J., and Guo, C. (2016). Selecting suitable energy-saving distillation schemes: Making quick decisions. Chem. Eng. Process. Process Intensif. 107, 138–150. doi: 10.1016/j.cep.2016.05.009
de Koeijer, G. M., and Kjelstrup, S. (2000). Minimizing entropy production rate in binary tray distillation. Int. J. Thermodyn. 3, 105–110.
European Commission (2018). A Clean Planet for all A European Strategic Long-Term Vision for a Prosperous, Modern, Competitive and Climate Neutral Economy. Report.
Fenske, M. R. (1932). Fractionation of straight-run Pennsylvania gasoline. Indus. Eng. Chem. 24, 482–485. doi: 10.1021/ie50269a003
Friedler, F., Tarjan, K., Huang, Y. W., and Fan, L. T. (1992). Graph-theoretic approach to process synthesis - axioms and theorems. Chem. Eng. Sci. 47, 1973–1988. doi: 10.1016/0009-2509(92)80315-4
Gilliland, E. R. (1940). Multicomponent rectification estimation of the number of theoretical plates as a function of the reflux ratio. Indus. Eng. Chem. 32, 1220–1223. doi: 10.1021/ie50369a035
Gleixner, A., Bastubbe, M., Eifler, L., Gally, T., Gamrath, G., Gottwald, R. L., et al. (2018). The SCIP Optimization Suite 6.0. Report, Optimization Online.
Halvorsen, I. J., and Skogestad, S. (2011). Energy efficient distillation. J. Nat. Gas Sci. Eng. 3, 571–580. doi: 10.1016/j.jngse.2011.06.002
Heckl, I., Friedler, F., and Fan, L. T. (2010). Solution of separation-network synthesis problems by the P-graph methodology. Comput. Chem. Eng. 34, 700–706. doi: 10.1016/j.compchemeng.2010.01.019
Holiastos, K., and Manousiouthakis, V. (2004). Infinite-dimensional state-space (IDEAS) approach to globally optimal design of distillation networks featuring heat and power integration. Indus. Eng. Chem. Res. 43, 7826–7842. doi: 10.1021/ie010434i
Ismail, S. R., Pistikopoulos, E. N., and Papalexandri, K. P. (1999). Modular representation synthesis framework for homogeneous azeotropic separation. AIChE J. 45, 1701–1720. doi: 10.1002/aic.690450809
Jang, D. J., and Kim, Y. H. (2015). A new horizontal distillation for energy saving with a diabatic rectangular column. Korean J. Chem. Eng. 32, 2181–2186. doi: 10.1007/s11814-015-0048-4
Jiang, Z., Madenoor Ramapriya, G., Tawarmalani, M., and Agrawal, R. (2018a). Minimum energy of multicomponent distillation systems using minimum additional heat and mass integration sections. AIChE J. 64, 3410–3418. doi: 10.1002/aic.16189
Jiang, Z., Ramapriya, G. M., Tawarmalani, M., and Agrawal, R. (2018b). Process intensification in multicomponent distillation. Chem. Eng. Trans. 69, 841–846. doi: 10.3303/CET1869141
Keßler, T., Kunde, C., McBride, K., Mertens, N., Michaels, D., Sundmacher, K., et al. (2019). Global optimization of distillation columns using explicit and implicit surrogate models. Chem. Eng. Sci. 197, 235–245. doi: 10.1016/j.ces.2018.12.002
Kim, B. C., Chun, H. H., and Kim, Y. H. (2013). Energy-efficient diabatic distillation using a horizontal distillation column. Indus. Eng. Chem. Res. 52, 14927–14935. doi: 10.1021/ie4013997
Kiss, A. A., Flores Landaeta, S. J., and Infante Ferreira, C. A. (2012). Towards energy efficient distillation technologies-making the right choice. Energy 47, 531–542. doi: 10.1016/j.energy.2012.09.038
Kuznetsov, V. V. (2018). Two-Phase Heat Exchangers. Cham: Springer International Publishing. doi: 10.1007/978-3-319-26695-4_20
Ledezma-Martinez, M., Jobson, M., and Smith, R. (2018). Simulation-optimization-based design of crude oil distillation systems with preflash units. Indus. Eng. Chem. Res. 57, 9821–9830. doi: 10.1021/acs.iecr.7b05252
Liesche, G., Schack, D., and Sundmacher, K. (2019). The FluxMax approach for simultaneous process synthesis and heat integration: production of hydrogen cyanide. AIChE J. 65:e16554. doi: 10.1002/aic.16554
Miettinen, K. (1998). Nonlinear Multiobjective Optimization, 1st Edn. New York, NY: Springer US. doi: 10.1007/978-1-4615-5563-6
Moioli, E., Mutschler, R., and Züttel, A. (2019). Renewable energy storage via CO2 and H2 conversion to methane and methanol: assessment for small scale applications. Renew. Sustain. Energy Rev. 107, 497–506. doi: 10.1016/j.rser.2019.03.022
Nakaiwa, M., Huang, K., Endo, A., Ohmori, T., Akiya, T., and Takamatsu, T. (2003). Internally heat-integrated distillation columns: a review. Chem. Eng. Res. Design 81, 162–177. doi: 10.1205/026387603321158320
Ott, J., Gronemann, V., Pontzen, F., Fiedler, E., Grossmann, G., Kersebohm, D. B., et al. (2012). Methanol. Weinheim: Wiley-VCH Verlag GmbH & Co; KGaA. doi: 10.1002/14356007.a16_465.pub3
Papalexandri, K. P., and Pistikopoulos, E. N. (1996). Generalized modular representation framework for process synthesis. AIChE J. 42, 1010–1032. doi: 10.1002/aic.690420413
Räuchle, K., Plass, L., Wernicke, H. J., and Bertau, M. (2016). Methanol for renewable energy storage and utilization. Energy Technol. 4, 193–200. doi: 10.1002/ente.201500322
Roetzel, W., and Spang, B. (2010). C3 Typical Values of Overall Heat Transfer Coefficients. Berlin; Heidelberg: Springer. doi: 10.1007/978-3-540-77877-6_6
Schack, D., Liesche, G., and Sundmacher, K. (2019). Simultaneous heat and mass flow optimization of a distillation column applying the FluxMax approach. Chem. Eng. Trans. 76, 337–342. doi: 10.3303/CET1976057
Schack, D., Liesche, G., and Sundmacher, K. (2020). The FluxMax approach: simultaneous flux optimization and heat integration by discretization of thermodynamic state space illustrated on methanol synthesis process. Chem. Eng. Sci. 215:115382. doi: 10.1016/j.ces.2019.115382
Schack, D., Rihko-Struckmann, L., and Sundmacher, K. (2018). Linear programming approach for structure optimization of renewable-to-chemicals (R2Chem) production networks. Indus. Eng. Chem. Res. 57, 9889–9902. doi: 10.1021/acs.iecr.7b05305
Schäfer, P., Caspari, A., Kleinhans, K., Mhamdi, A., and Mitsos, A. (2019). Reduced dynamic modeling approach for rectification columns based on compartmentalization and artificial neural networks. AIChE J. 65:e16568. doi: 10.1002/aic.16568
Shah, P. B., and Kokossis, A. C. (2002). New synthesis framework for the optimization of complex distillation systems. AIChE J. 48, 527–550. doi: 10.1002/aic.690480311
Stull, D. R. (1947). Vapor pressure of pure substances. organic and inorganic compounds. Indus. Eng. Chem. 39, 517–540. doi: 10.1021/ie50448a022
Surya Prakash, G. K., Olah, G. A., and Goeppert, A. (2011). “Beyond oil and gas: the methanol economy, in ECS Transactions, Vol. 35, eds S. Creager, I. Fritsch, and N. Sridhar (Montreal, QC), 31–40. doi: 10.1149/1.3645178
Underwood, A. J. V. (1949). Fractional distillation of multicomponent mixtures. Indus. Eng. Chem. 41, 2844–2847. doi: 10.1021/ie50480a044
Keywords: process design, distillation columns, energy-efficiency, heat exchange, heat integration, methanol
Citation: Schack D, Jastram A, Liesche G and Sundmacher K (2020) Energy-Efficient Distillation Processes by Additional Heat Transfer Derived From the FluxMax Approach. Front. Energy Res. 8:134. doi: 10.3389/fenrg.2020.00134
Received: 26 March 2020; Accepted: 02 July 2020;
Published: 03 July 2020.
Edited by:
Francois M. A. Marechal, École Polytechnique Fédérale de Lausanne, SwitzerlandReviewed by:
Massimiliano Errico, University of Southern Denmark, DenmarkRajagopalan Srinivasan, Indian Institute of Technology Madras, India
Copyright © 2020 Schack, Jastram, Liesche and Sundmacher. This is an open-access article distributed under the terms of the Creative Commons Attribution License (CC BY). The use, distribution or reproduction in other forums is permitted, provided the original author(s) and the copyright owner(s) are credited and that the original publication in this journal is cited, in accordance with accepted academic practice. No use, distribution or reproduction is permitted which does not comply with these terms.
*Correspondence: Kai Sundmacher, sundmacher@mpi-magdeburg.mpg.de