- 1Department of Energy, Politecnico di Milano, Milan, Italy
- 2Department of Energy and Process Engineering, The Norwegian University of Science and Technology (NTNU), Trondheim, Norway
This paper presents the optimization of organic Rankine cycles (ORCs) for recovering waste heat from a hypothetical aluminum production plant to be installed in Norway. The case study is particularly interesting because it features two hot streams at different temperatures (the pot exhaust gases and the cell wall cooling air), which make available about 16 MWth below 250°C. First, a recently proposed cycle optimization approach is adopted to identify the most promising working fluid and optimize the cycle variables (pressures, temperatures, mass flow rates) for the maximum energy performance. The analysis includes both pure fluids, including recently synthesized refrigerants, and binary zeotropic mixtures assessing in total 102 working fluids. The best pure fluid in terms of exergy efficiency turns out to be HFE-347mcc (which can achieve a target exergy efficiency of 85.28%), followed by neopentane, butane, and R114. HFO-1336mzz appears to be one of the most promising non-flammable alternatives with low Global Warming Potential (GWP). The mixture leading to the highest exergy efficiency is isobutane–isopentane, which can increase the net electrical power output by up to 3.3% compared to pure fluids. The systematic techno-economic optimization, repeated for two different electricity prices, shows that RE347mcc is the best option in both low and high electricity prices. The cost of the cycle using HFO-1336mzz is penalized by the larger evaporation heat (negatively influencing the heat integration) and the smaller regenerator.
Introduction
With the current aim of energy saving in order to reduce emission and effort to diminish environmental impact of process and manufacturing plant, energy recovery from industrial processes is becoming a major topic. For instance, it was estimated that the “energy wasted by all U.S. industrial facilities could produce power equivalent to 20% of U.S. electricity generation capacity, without burning any fossil fuel, and could help many industries to meet recent global warming regulation” (Sami, 2010), as a proof of how much potential heat recovery may have. In addition, given the target of reducing about 20% the emission of the EU countries, increasing efficiency and heat recovery from industrial processes will be crucial. A study carried out by Campana et al. (2013) found that energy coming from waste heat conversion could be up to 2% of the European Industry energy use, leading to a substantial decrease in greenhouse gases (GHG). The industrial sectors of most concern for waste heat recovery are the ones producing cement, steel, and other metal alloys. Aluminum manufacturing plants demand a large amount of electricity for their production process, with about half of the energy lost as heat (Ladam et al., 2011). The aluminum industry world's average power consumption was 13.4 kWh/kgAl produced as of 2017 (World Aluminium Website of the International Aluminium Institute, 2019). Considering the total amount of aluminum produced in the world, 63.4 millions metric tons (63.4 billions kg) in 2017 (World Aluminium Website of the International Aluminium Institute, 2019), the heat recovery potential is vast. Ladam et al. (2011) estimate that 0.9 kWh of electric energy per kilogram of aluminum can be generated with heat recovery cycles exploiting the heat available at the cathode (above 300°C pot shell temperature), anode, and the pot flue gas (120–160°C with current technology but an increase is expected with near-future technologies). Current and future technology development on the electrolysis process will lead to lower power consumption. Today's state of the art is around 12 kWh/kgAl with a target of 10 kWh/kgAl (Solheim, 2018). In order to achieve such low targets, lower pot shell temperatures (e.g., 250°C) and recovery of heat from the electrolysis cells may be essential (Solheim, 2018).
Given the heat source temperatures and relatively small size of the heat recovery Rankine cycle, organic fluids are expected to outperform steam as working fluid (Cavallini, 2017). Karellas et al. (2013) investigated waste heat recovery cycles for the cement industry, finding that, if the exhaust gas temperature is below 310°C, the organic Rankine cycle (ORC) is more efficient than the steam cycle. Zhang et al. (2013) compared ORC, steam cycle, and cascaded steam-organic cycle options for heat recovery from steel plants. For exhaust gas temperatures between 200 and 300°C, the best option found was an ORC with R141b as working fluid. As far as aluminum production plants are concerned, recently, Yu et al. (2018) assessed the performance of heat recovery ORCs for an aluminum production plant located in Iceland wasting about 88 MW of thermal power from the smelter exhaust gases.
In this work, a future aluminum plant case study, based on Norwegian conditions, is selected to evaluate the benefits of heat recovery. The plant features two hot streams at different temperatures: one stream from the cooling medium of the pot cell walls (cathode) and one from the pot flue gases. The objective of the work is to identify the optimal ORC design [working fluid, pressures, temperatures, mass flow rates, layout, and heat exchanger network (HEN)] as well as the energy and economic performance with state-of-the-art computer-aided tools. The main peculiarity of the plant is the availability of two hot streams with different temperatures, which calls for the use of heat integration methodologies. For this reason, working fluid and cycle pressure and temperatures are optimized with the systematic optimization approaches recently proposed by Scaccabarozzi et al. (2018), while the ORC design, mass flow rates, and HEN are optimized with the approach developed by Martelli et al. (2017) and Elsido et al. (2018). Both approaches can handle the optimization of Rankine cycles integrated with multiple hot and cold streams. Thanks to the use of such efficient computer-aided tools, the study considers 72 pure fluids and 30 binary zeotropic mixtures, making it possible to compare the energy and economic performance achievable with different fluids.
Problem Statement
The case study is a future aluminum production plant based on Norwegian conditions. The plant is based on several electrolytic reduction cells (also called “smelters”), which operate at high temperature (about 940–980°C) absorbing about 10 kWh/kgAl. It is assumed that in novel aluminum production processes, about 35% of the electricity is converted into heat and released with the stream of flue gases leaving the pot at about 180°C and the pot shell cooling air at approximately 250°C. Figure 1A shows a schematic flow diagram of the plant. In this study, it is assumed that the two hot streams make available the same thermal power (about 7.8 MW each), and both can be used as heat sources for a heat recovery ORC. While the pot exhaust gases must be cooled down to 80°C because of the specifications of the downstream filters, the pot cooling air can be cooled down to 20°C (minimum outlet temperature). Sea water at 10°C was assumed to be available near the plant site as cold utility for the ORC condenser and to waste the unrecovered thermal power. A summary of the stream properties is reported in Figure 1. The composite curve of the two hot streams, which is obtained by applying the same principles of “pinch analysis” described by Linnhoff (1979) and Kemp (2006), is reported in Figure 1B. The peculiarity compared to having a single hot stream is the variation of the overall available heat capacity flow rate due to the presence of two heat sources.
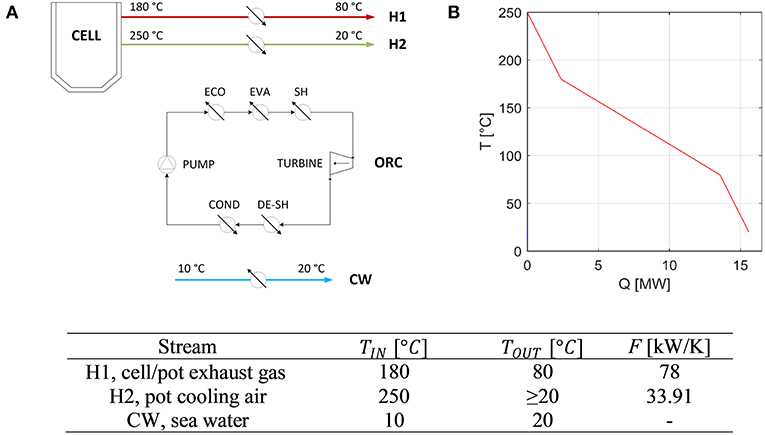
Figure 1. General scheme of the case study (A) and composite curve of the available hot process streams (B), and data for the aluminum manufacturing plant case study.
Optimization of Working Fluid Selection
Since performing a detailed techno-economic optimization of the ORC for each possible working fluid is clearly not practicable because of the required computational time, three classes of computationally efficient approaches have been proposed:
- Screening approaches: numerical methods are employed to select the cycle configuration and operating parameters for a set of candidate fluids, which is identified based on engineering criteria [see, e.g., (Wang et al., 2011; Scaccabarozzi et al., 2018)];
- Computer-aided molecular design methods [see, e.g., (Papadopoulos et al., 2010; Palma-Flores et al., 2014)]: the synthesis of the molecule of the working fluid is automatically optimized with a challenging Multiple Integer Non-Linear Programming (MINLP) problem to find the fluid with the most suitable properties; the problem can include the optimization of the cycle variables;
- Molecular targeting methods [see, e.g., (Lampe et al., 2014)]: the molecule design and properties of the ideal (“target”) fluid are simultaneously optimized with the cycle configuration and cycle variables; the real working fluids with the most similar properties are identified in a second phase using an ad hoc procedure.
For a thorough review of computer-aided fluid selection and ORC design optimization methods, the reader is referred to Linke et al. (2015).
In this work, the screening approach proposed by Scaccabarozzi et al. (2018) is used. First, a set of pure fluids and binary zeotropic mixtures is identified; then, for each fluid, the maximum achievable energy performance is determined with an ad hoc optimization algorithm. Fluids are ranked in terms of energy performance, and those with the most promising combination of performance indices (including ODP, GWP, cycle operating pressures, etc.) are considered for a more detailed technoeconomic optimization study. The methodology has also the advantage of finding the values of the cycle pressures and temperatures that maximize the efficiency. These values of cycle pressures and temperatures can be passed as input data to the technoeconomic optimization methodology (see Technoeconomic Optimization).
Set of Candidate Fluids
The complete list of pure fluids and mixtures considered in this work is listed in Table 1. Differently from Scaccabarozzi et al. (2018), nearly all the pure working fluids available in REFerence fluid PROPerties REFPROP v9.1 (REFerence fluid PROPerties) have been considered. Fluids that are flammable and/or with positive ODP and/or high GWP are also included with the aim of comparing them with newer alternatives. Only fluids with too low critical temperatures (i.e., 50°C) and/or thermal stability below 200°C have been discarded. Fluids with unknown thermal stability limits have been included in the analysis with the objective of assessing the achievable performance and guiding future research efforts: if they turn out to be promising, their thermochemical stability limits can be determined with future ad hoc experimental campaigns [as done in (Invernizzi et al., 2016)]. As far as mixtures are concerned, given the large number of possible combinations of fluids, it is necessary to perform a preliminary screening. On the basis of the results of Scaccabarozzi et al. (2018), for a similar waste heat recovery application, the most promising binary zeotropic mixtures feature a critical temperature within 250 and 500°C and an appreciable temperature glide (>5°C) in condensation (with condensation pressures evaluated ranging from 0.05 to 5.05 bar). Mixtures proposed in previous studies have been included as well. It is important to notice that for 24 mixtures denoted in Table A2, due to the lack of experimental data to calibrate the equation of states, REFPROP estimates the thermodynamic properties with the general approach proposed in Lemmon and McLinden (2001).
Thermodynamic Optimization Methodology
Given the set of available hot and cold streams of the plant, for each candidate working fluid (pure fluids and/or mixtures), the algorithm of Scaccabarozzi et al. (2018), which was adopted for this study, can determine the cycle pressure, temperature, and mass flow rates, which maximize a relevant energy performance index. For heat recovery applications, the most appropriate energy performance index is the net power generated by the ORC or, equivalently, the exergy efficiency (Scaccabarozzi et al., 2018):
Where ẆORC is the net ORC power output, is the thermal power of the hot stream and TML denotes the mean logarithmic temperature between the hot stream inlet and outlet temperatures and T0 is the dead state (ambient) temperature. Another suitable performance index is the first law efficiency, defined as follows:
Since the denominator of Equation (1) and (2) are problem data, maximizing ẆORC, ηex or ηI is equivalent. In this study Equation (1) was chosen as objective function of the optimization problem.
The methodology (Scaccabarozzi et al., 2018) combines the evolutionary algorithm PGS-COM (Particle Generating Set-Complex algorithm) (Martelli and Amaldi, 2013) with the well-known energy targeting methodology of Kalitventzeff and Maréchal (1999). The block-flow diagram of the algorithm is shown in Figure 2.
The evolutionary algorithm PGS-COM optimizes the independent cycle variables, namely, the evaporating and condensing pressure, the turbine inlet temperature, and the mixture composition. Each combination of independent cycle variables sampled by PGS-COM is given as input to the black-box, which solves the cycle model to calculate the dependent variables (e.g., streams temperature profiles, mass flow rates, etc.) and the objective function (second law efficiency).
In the black-box, the ORC model is implemented in Matlab® R2015 (The MathWorks Inc, 2015). All the intensive and specific properties of the cycle streams, such as the temperatures, pressures, and enthalpies are calculated with REFPROP v9.1 (Lemmon et al., 2013), for both pure fluids and mixtures. The cycle model checks also whether the following constraints of the cycle optimization problem are met:
• Vapor fraction of the flow evolving in the expander greater or equal to 0.88;
• Temperature difference in the heat exchangers ≥ 5°C;
• Condensation pressure ≥ 0.03 bar.
These constraints are handled within PGS-COM with the extreme barrier approach (see Astolfi et al., 2017). Since the methodology must screen a large number of working fluids, the ideal Rankine cycle is considered in the assessment of the performance (i.e., isentropic pump and turbine, and heat exchangers without pressure drops and heat losses) so as to save computational time compared to more sophisticated models.
Once all the cycle-specific and intensive properties are determined, only the mass flow rates of the ORC and cooling water are missing. The heat integration between ORC streams and heat sources/heat sinks is optimized with the methodology proposed by Kalitventzeff and Maréchal (1999), which determines the maximum mass flow rate of the working fluid that can be generated (the so-called “maximum heat recovery target”), and therefore the maximum cycle net power, for a given minimum allowed heat transfer temperature difference. The associated linear program can be formulated as follows:
where x denotes the vector of decision variables (including ORC mass flow rate, cooling water mass flow rate and heat cascade residual heat flows), Equation (4) denotes the linear system of equations of the heat cascade (i.e., heat balance of each temperature interval) (Linnhoff, 1979; Kemp, 2006), and w is the vector of net specific works associated to each utility mass flow rate (ORC and cooling water). The linear program defined by Equations 3 through 5 features N − 1 + M variables and N constraints, where N denotes the number of temperature intervals and M is the number of utilities with unknown mass flow rates (two for our case). Compared to the plain heat cascade approach for streams featuring constant heat capacity, we considered 300 intervals so as to closely approximate the temperature profiles of supercritical working fluids (in each interval, the heat capacity variation is negligible). The linear program is solved in Matlab® with the default algorithm of the “linprog” function in <1 s.
For each fluid, the average total computational time to reach convergence of the PGS-COM algorithm is approximately 30 min, corresponding to about 2,000 function evaluations.
Fluid Ranking and Selection
Tables 2, 3 report the results of the thermodynamic optimization for the first 10 pure fluids and mixtures, respectively, whose thermodynamic properties were all evaluated by means of REFPROP v9.1. The complete ranking of fluids is reported in Table A1 and Table A2 in the Appendix. The pure fluid leading to the highest exergy efficiency (and net power output) is RE347mcc, a hydrofluoroether, with GWP = 530. The maximum recoverable electric power is 3530.63 kW. The other nine fluids are either flammable (Neopentane, Butane, Isobutene, 1-Butene, Propylene) or with positive ODP (R114, R115) or with high GWP (R143, R245fa2). The first non-flammable fluid with zero ODP and near-zero GWP is R1336mzz, ranked 16th in terms of exergy efficiency. Using such fluid, the maximum recoverable power output is only 3.5% lower than the first one (RE347mcc). Slightly lower efficiency can be reached using R1233zde, another non-flammable fluid with zero ODP and near-zero GWP, which ranks 23rd.
Similar to what was done by Scaccabarozzi et al. (2018), who plotted the second law efficiency achieved with each working fluid against the ratio between its critical temperature and the inlet temperature of the hottest heat source, Figure 3A shows that there is an evident relation between the maximum achievable efficiency and the already mentioned temperature ratio. Specifically, fluids with a critical temperature either in the range 66–69 or 81–84% of the maximum heat source temperature (i.e., 523.15 K) are those ones that show highest efficiency. The former group of fluids (namely, R115, R143a, and propylene) exploits supercritical turbine inlet pressures while the latter features pressures close to the critical one (slightly subcritical or supercritical). On the other hand, the critical temperature is not the unique fluid property affecting the maximum achievable efficiency. Indeed, although RE347mcc and R1233zde have a negligible difference in critical temperatures (about 1°C), the exergy efficiency is appreciably different (85.3% vs. 81.3%), indicating that the critical temperature is the fluid property with the major effect on cycle efficiency, but there are other relevant thermodynamic properties. Such properties are those which control the shape of the saturation curve in the temperature–entropy (T–s) diagram, mainly the liquid specific heat capacity and the molecular complexity (influencing the slope of the saturated vapor line) (Invernizzi, 2013). These properties influence not only the efficiency of the thermodynamic cycle but also the heat integration with the available hot and cold streams of the process (i.e., pinch point positions and heat recovery factor), as shown in the composite curves of Figures 4A,B. It is worth noting that the composite curves include the hot and cold streams of the ORC in addition to the hot process streams (H1, H2) and the cooling water (i.e., the cold curve is made by the cooling water, economizer, evaporator, and superheater of the ORC, while the hot curve is made by H1, H2, ORC desuperheater, and condenser). For instance, the composite curve of the cycle using RE347mcc features a very small heat transfer temperature difference from 150 to 30°C. Surprisingly, in the optimized heat integration solution, the ORC regenerator is used to compensate for the relative reduction of process heat below 80°C (as the stream of exhaust gases below 80°C).
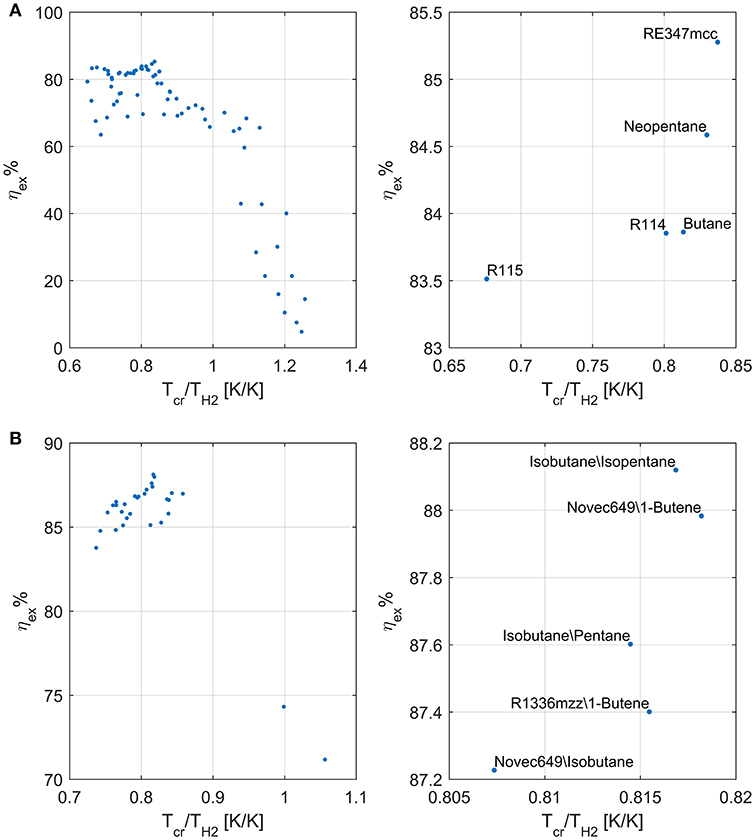
Figure 3. Exergy efficiency of the considered pure fluids (A) and mixtures (B) as a function of the ratio between their critical temperature and the inlet temperature of the hottest source.
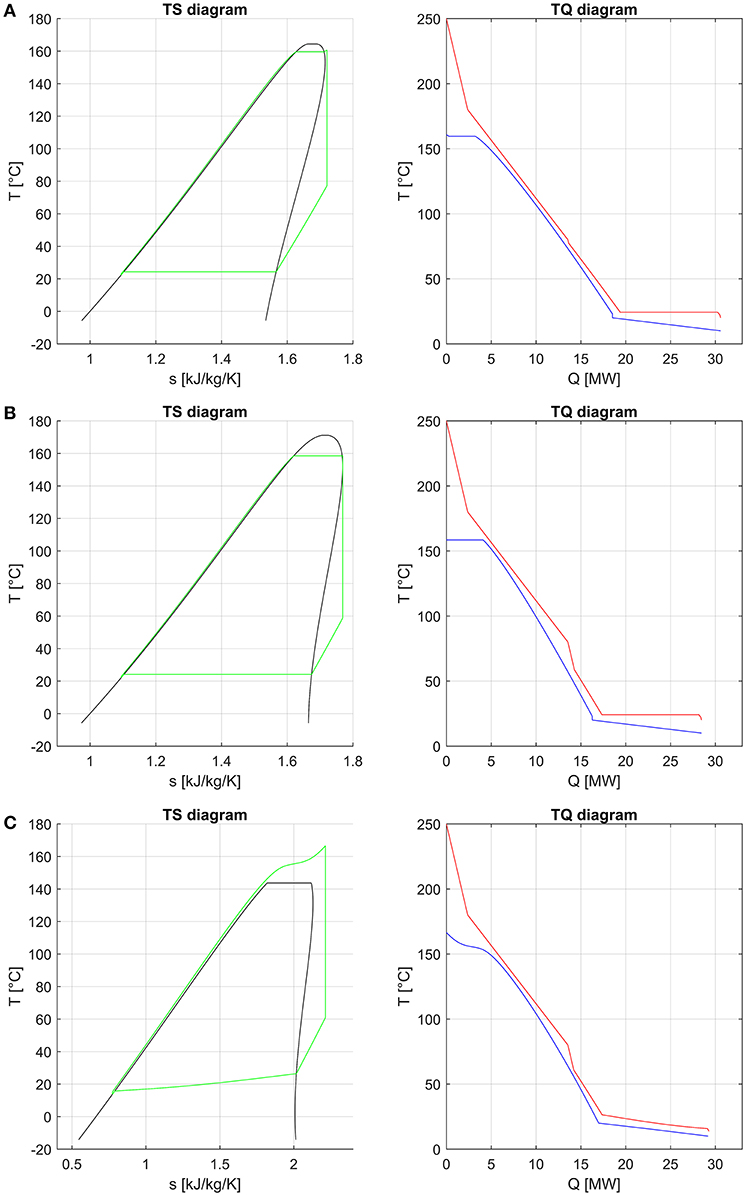
Figure 4. Temperature–entropy (T–s) diagram and composite curves (T–Q diagrams) of the optimized organic Rankine cycle (ORC) employing RE347mcc (A), R1336mzz (B), and the binary zeotropic mixture isobutane/isopentane (0.66/0.34) (C). The saturation curve of this last one could not be computed in a reliable way in the region close to the critical temperature due to convergence issues of the flash calculation.
As far as mixtures are concerned, the best zeotropic mixture (in terms of energy efficiency) is isobutane (66% mass basis)–isopentane (34%), leading to an exergy efficiency gain of about 2.8 percentage points with respect to the best pure fluid. The corresponding increase in net power output of the ORC is 3.3%. The optimal cycle is supercritical for the first 10 mixtures, except for the sixth and seventh (butane/pentane, isopentane/R245fa). Unfortunately, all the mixtures in the first positions contain a high concentration of hydrocarbons, which may lead to safety issues related to fluid flammability. Figure 3B shows the relation between the maximum achievable exergy efficiency and the critical temperature of the mixture. It indicates that the mixtures with the highest exergy efficiency have a critical temperature lying in the range 80–82% of the maximum heat source temperature. Figure 4C shows the T–s and composite curves (temperature–heat) diagrams for the first mixture. Compared to pure fluids, the condensation temperature glide (about 10°C) reduces the irreversibility (exergy loss) occurring in the heat transfer process between the condensing fluid and the cooling water. Furthermore, the mixture features also a better matching at high temperatures with the hot process stream thanks to the supercritical cycle configuration.
Given the results of the thermodynamic optimization, the safety issues of flammable fluids and the current limitations on the ODP, HFE-RE347mcc, and HFO-R1336mzz are selected for the technoeconomic optimization. Despite its flammability, the mixture isobutene/isopentane is also considered in the technoeconomic optimization with the goal of assessing the potential economic advantage of using zeotropic mixtures compared to pure fluids.
Technoeconomic Optimization
The pure fluids and the mixture selected in Fluid Ranking and Selection are compared from a technoeconomic point of view, keeping the cycle parameters (i.e., cycle pressures and temperatures) fixed at the optimal values found in thermodynamic optimization. The goal of the technoeconomic optimization is to determine the best trade-off between energy efficiency and capital costs of the ORC and the heat exchanger network (HEN). To the best of the authors' knowledge, the problem of the technoeconomic optimization of the design of the integrated HEN and ORC has been addressed only by few works in literature. Desai and Bandyopadhyay (2009) were the first authors to consider process integration of ORCs for waste heat recovery. They assumed fixed ORC schemes (i.e., with turbine bleeding and regeneration) and imposed that the ORC can utilize the low-temperature heat below the process pinch point; only after determining the operating conditions of the ORC are heuristics employed to derive a feasible HEN. Chen et al. (2016) evaluated the use of an intermediate heat transfer fluid or the direct integration of ORC and heat sources/sinks with an optimization method. They solved the heat integration problem with the objective to maximize the net power output of the ORC. The economic feasibility of the solutions is considered only after the optimization. Hipólito-Valencia et al. (2013) proposed a method to simultaneously optimize the design of HEN and ORC based on two simplifying assumptions: they assumed fixed ORC schemes and considered a limited set of fixed heat integration options between ORC streams and heat sources/sinks. Yu et al. (2017) addressed the problem of technoeconomic ORC optimization for waste heat recovery from multiple streams. For safety and controllability reasons, they only considered indirect integration with hot water as an intermediate heat transfer fluid between heat sources/sinks and ORC. The well-known energy targeting model proposed by Duran and Grossmann (1986) is used to address the heat integration, and then a suboptimal HEN is derived heuristically.
Martelli et al. (2016, 2017), and Elsido et al. (2017a,b) proposed a simultaneous method for the combined synthesis of HENs and utility systems for one or more available heat sources, including heat recovery cycles, and the selection and design of the latter ones, while considering all the possible integration options between process heat sources/sinks and utility systems. Compared to sequential or separated approaches, the advantage of the simultaneous approach is that it allows one to systematically optimize not only the cycle configuration but also the heat integration and HEN while rigorously taking into account the trade-off between energy efficiency and costs. For this reason, the methodology proposed by Martelli et al. (2017) has been selected for this work.
Technoeconomic Optimization Methodology
The method proposed by Martelli et al. (2017) is based on the use of the general “p-h superstructure” for Rankine cycles (such as steam cycles, ORCs, and heat pump cycles) (Elsido et al., 2017a) and the extension of the SYNHEAT (Yee and Grossmann, 1990) superstructure for HEN design, able to generate a very wide variety of possible matches between process streams and utility streams. The ORC streams in the superstructure are characterized with variable mass flow rate and binary variables for their selection. Thanks to the combination of the two superstructures, the methodology allows one to configure and optimally design the ORC and HEN simultaneously, integrate the heat sources/sinks with the cycle, while considering energy efficiency and capital costs (i.e., targeting the minimum total annual cost, rather than just efficiency). A scheme of the extended superstructure for ORC and HEN design is shown in Figure 5. In this work, since only single pressure level ORC designs are considered, all the stream mass flow rates of the ORC can be represented with a single activation variable yORC and mass flow rate ṁORC.
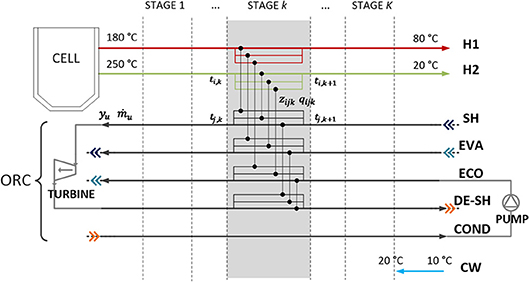
Figure 5. Scheme of the combined HEN + ORC superstructure for techno-economic optimization. Hot and cold streams are represented with arrows pointing respectively to the right and to the left. In temperature stage k, all the possible heat exchangers between hot stream i and cold stream j in stage k, that can be selected with binary variables (zijk), are represented with black vertical connectors. In addition, each ORC stream u is characterized by variable mass flow rate ṁu and a binary variable for its selection yu.
The mathematical model is characterized by the following variables, constraints, and objective function.
Decision Variables
• Heat exchanged between each hot stream and each cold stream (process, ORC, and cooling water streams) in each temperature stage of the HEN (positive continuous variables),
• Temperature of each hot and cold non-isothermal stream at hot end of each stage (continuous variables),
• Temperature difference for each heat exchanger between each hot and cold stream at hot end of each stage (positive continuous variables),
• Activation of the heat exchanger between each hot stream and each cold stream in each stage (binary selection variables),
• Area of the heat exchanger between each hot stream and each cold stream in each stage (positive continuous variables),
• Activation of each ORC stream (binary selection variables),
• Mass flow rate of each ORC stream (positive continuous variables).
Constraints
• Overall energy balances for all hot and cold streams in the HEN,
• Stage energy balances for all hot and cold streams in the HEN,
• Monotonicity of temperature profiles of streams along the stages,
• Logical constraints relating the heat exchanger load and area with the corresponding binary variables for the selection of the heat exchangers, for all possible matches between hot and cold streams,
• Logical constraints relating the continuous variables representing the mass flow rate for all streams of the ORC and the corresponding binary variables for the selection of the streams,
• Additional logical constraints relating the existence of ORC streams with the existence of all possible heat exchangers involving them,
• Mass and energy balances at each header of the p-h superstructure,
• Constraints to calculate the temperature differences for the selected heat exchangers,
• Relation between heat exchanger areas, mean logarithmic temperature differences, global heat transfer coefficient, and heat duty of the heat exchanger.
Objective Function
The non-linear objective function to be minimized is the total annual cost (TAC) of the overall HEN and ORC, which includes the sum of the investment costs for the HEN, for the ORC, the operational costs due to cooling water pumping and auxiliaries' consumption, and the avoided costs for buying the produced electricity from the grid at the wholesale price:
The variables that appear in the formula above are as follows: ṁORC which represents the mass flow rates of the ORC working fluid; Aijk and ACW, i, which represent the area of a heat exchanger between each stream i in the set I of hot streams (both process and ORC streams) and each stream j in the set J of cold streams or cooling water (CW) in each temperature stage k in the set K; and qCW, i, which represent the heat exchanged between each stream i in the set I of hot streams (both process and ORC streams) and the cooling water (CW). The cost functions of heat exchangers, utilities, and components of the Rankine cycle are dependent on the size of the equipment according to the well-known economy of scale law: for the area of the heat exchangers, CA is the area-specific cost at the reference size AREF and β is the exponent; for the ORC turbine, CS is the specific cost at the reference size SREF and α is the exponent. and are the specific enthalpy differences while ηME, T and ηME, P are the mechanical/electrical efficiency employed to calculate the nominal power of turbine and pump, respectively. The investment costs are multiplied for the factor MF accounting for installation costs, construction, contingencies, etc. Then, the total plant cost is annualized using the levelized annual capital carrying charge rate, CCR, defined by the EPRI (Electric Power Research Institute) methodology (E. P. R. Institute, 1993), which takes into account the expected plant lifetime, debt/equity ratio, corporate income taxes, owner costs, etc. The parameter pEL represents the electricity price, and hEQ is the number of full-load equivalent operating hours.
As shown in Martelli et al. (2017), the resulting non-convex MINLP problem is very challenging. It can be effectively solved using the ad hoc bilevel decomposition method recently proposed by Elsido et al. (2018, in press). The method is based on a decomposition of the full Mixed-Integer NonLinear Programming (MINLP) problem into a master MILP problem and an Nonlinear Programming (NLP) subproblem. The master problem is a relaxed and linearized version of the original MINLP problem, combining the outer-approximation linearization technique with McCormick relaxation, valid redundant constraints, piecewise linearization of the cost functions, and “nested” integer cuts. The integer variables found by the master problem are then fixed and used to generate the NLP subproblem. The NLP subproblem includes all the continuous variables of the full problem, and all the linear and non-linear constraints of the full problem, with fixed integer variables found by the master problem. The objective function is the minimization of the TAC. From the resolution of the NLP sub problem, at each iteration, an upper bound to the objective function is found and the linearization of the master problem is updated; in addition, new integer cuts are included in the master problem. The interested reader can find the mathematical details of the algorithm in Elsido et al. (2018, in press).
Assumptions for Technoeconomic Optimization
The main assumptions used for the technoeconomic optimization are reported in Table 4. The outlet temperature of hot process stream H2 is assumed as variable, constrained to be ≥ 20°C. In the case of the isobutane/isopentane (0.66/0.34) mixture, the supercritical heating has been assumed as equivalent to two transformations with constant specific heat, one between 18 and 150°C and the other between 150 and 165°C.
Two scenarios for the technoeconomic optimization study are considered:
• Low electricity price, pEL equal to 70 $/MWh
• High electricity price, pEL equal to 140 $/MWh, to account for possible financial incentives to waste heat recovery.
Due to large power consumption of the aluminum production process, the electricity generated by the ORC is not sold to the electric grid but self-consumed by the plant, leading to savings in the electricity bill (i.e., it represents an avoided cost). For this reason, the economic value of electricity is representative of the average electricity purchase price of the plant (not the electricity selling price). The low electricity price of 70 $/MWh in this work is meant to represent a realistic price within the next 10 years in Norway. The average electricity spot price of the Nordpool (Nordpool, 2019) market for the Oslo area in Norway was 50.2 $/MWh in 2018. The network cost including transmission and distribution was approximately 6.8 $/MWh to be added to the spot price, according to ENTSO-E (European Network of Transmission System Operators for Electricity) (ENTSOE, 2019). The electricity price is expected to increase until 2030 with ~20% in Norway, according to International Energy Agency (IEA) (IEA and Nordicetp, 2016), meaning an electricity price of 70 $/MWh is a reasonable assumption for this work.
Due to the lack of reliable literature data, the extra equipment costs required to prevent fluid leakages and to perform fluid makeup are not considered. These additional costs are expected to be higher for the zeotropic mixture as better sealing systems are required. Indeed, as reported in Kruse and Rinne (1992), leakages may cause changes in the composition of the working fluid (e.g., the more volatile compound may leak out more quickly than the other one), causing variations of the cycle performance. However, considering such effect requires a more detailed engineering study, which is out of the scope of this work.
As far as the HEN superstructure is concerned, five temperature stages have been considered for all working fluids, yielding MINLP problems with 592 single equations, 332 continuous variables, and 73 binary variables. The computational time on a single-core computer for convergence of the bilevel decomposition algorithm (Elsido et al., 2018, in press) (i.e., no solution improvement for 20 iterations or zero gap between upper and lower bound) is about 600–1,800 s (20–60 iterations of the algorithm).
Results of Technoeconomic Optimization
The optimization results for the low and high electricity price case are presented, respectively, in Tables 5, 6 and Figures 6, 7. The scheme of the plant using RE347mcc is shown in Figure 6A. The economizer is split into two parallel branches heated, respectively, by H1 and H2. The heat for the evaporator is provided by H2. The scheme of the plant using the isobutane/isopentane (0.66/0.34) mixture is shown in Figure 6C. In this case, the two exchangers in series coupled with H1 could be merged in a single larger heat exchanger. This option would lead to some savings in the investment cost, but it cannot be handled by the HEN synthesis methodology that assumes constant specific heat capacity within each heat exchanger. Similarly, the desuperheater and condenser in series coupled with cooling water (i.e., in Figures 6A,B for the low electricity price case, respectively, for RE347mcc and R1336mzz, and also in Figures 7A,B for the high electricity price scenario, respectively, for RE347mcc and R1336mzz) could be merged in a single larger heat exchanger, but it cannot be obtained by the adopted HEN synthesis methodology.
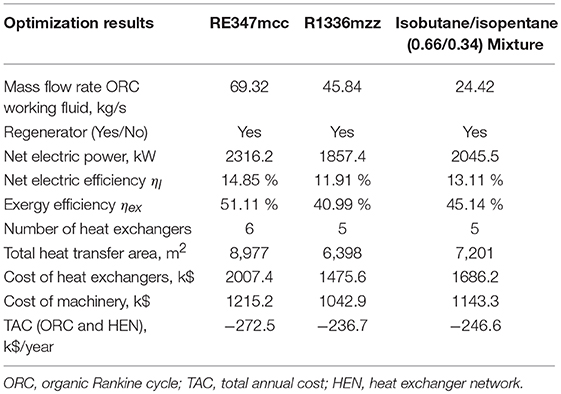
Table 5. Results of the techno-economic optimization for the low electricity price scenario (70 $/MWh).
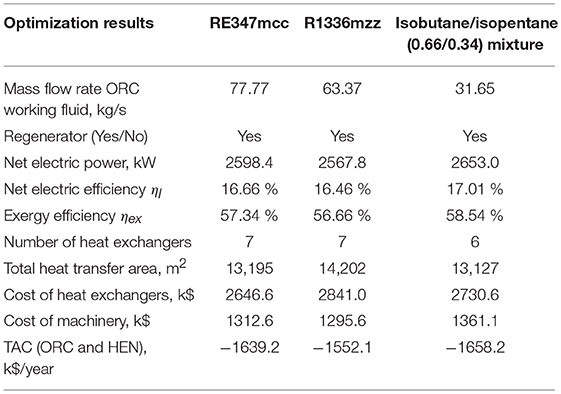
Table 6. Results of the techno-economic optimization for the high electricity price scenario (140 $/MWh).
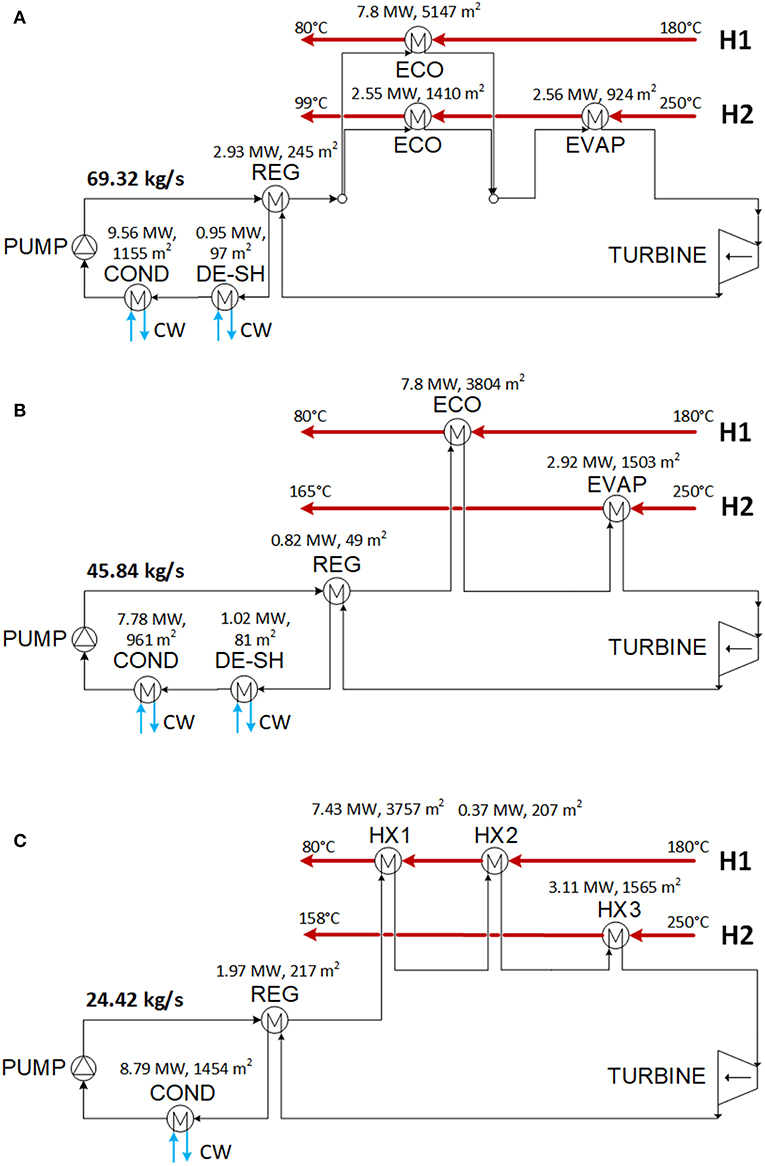
Figure 6. Optimized schemes of the optimal solutions found with fluid RE347mcc (A), R1336mzz (B), and isobutane/isopentane mixture (C) for the electricity price equal to 70 $/MWh.
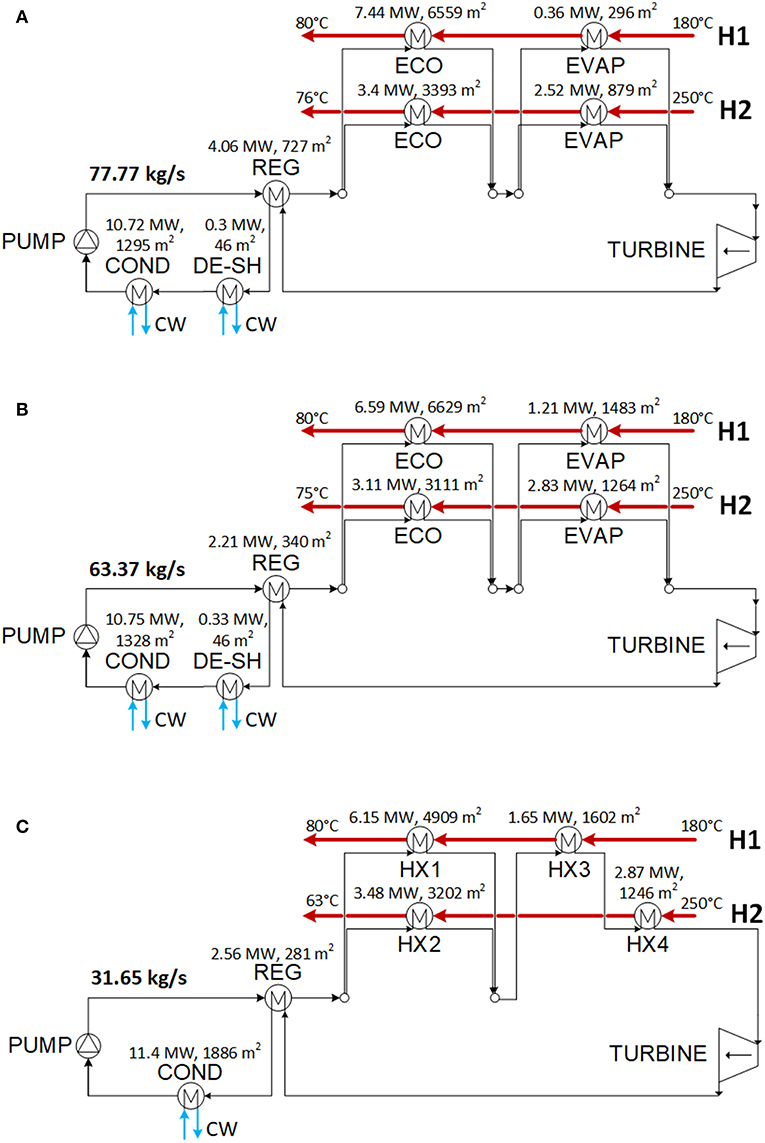
Figure 7. Optimized schemes of the optimal solutions found with fluid RE347mcc (A), R1336mzz (B), and isobutane/isopentane (C) for the electricity price equal to 140 $/MWh.
For all working fluids and in both price scenarios, the optimal solution found by the algorithm is a single pressure level ORC cycle with regenerator and the TAC is negative (meaning that there is an economic advantage in installing the ORC). The regenerator is used in all cases because organic vapors (on the hot side of the regenerator) feature a heat transfer coefficient higher than streams H1 and H2 (air): to preheat the liquid, from an economic point of view, it is more advantageous to use the regenerator instead of the economizers. In the low electricity price case, the cycle using RE347mcc achieves the best efficiency and economic performance, with net electric power output equal to 2316.2 kW (−34% compared to the ideal target) and TAC equal to −272.5 k$/year (being negative, it is a revenue). The difference in electric power output between the economic optimization solution and the thermodynamic target is due to 1) the expansion losses in the real cycle turbine featuring an isentropic efficiency equal to 80% and 2) the decrease of heat recovered from the heat sources. Concerning the last point, the economically optimal solution has −13.5% working fluid mass flow rate compared to the target found with the thermodynamic optimization owing to the need of containing the investment cost of the heat exchangers. Compared to RE347mcc, the economic profit of the solutions obtained using R1366mzz and the isobutane/isopentane mixture is −15.1 and −9.5%, respectively. The main reason appears to be the lower working fluid mass flow rate (25–28% lower than the target), which limits the net electric power output (−24.7 and −11.7%, respectively, compared to RE347mcc). Interestingly, the reduction of mass flow rate compared to the thermodynamic target is about double of that found for RE347mcc (−13.5%). This is due to (1) pinch point at the evaporator, which limits the working fluid mass flow rate, and (2) costly recovery of low-temperature heat from heat source H2. Concerning the evaporator, in the low electricity price scenario, there is no evaporator coupled with heat source H1 (or the high-temperature heat exchanger in the case of the mixture) in any of the three fluids, because it would be highly expensive due to the low heat transfer coefficient of the hot stream and the small temperature difference (stream H1 has an inlet temperature quite close to the evaporation temperatures). Therefore, the mass flow rate of fluid that can be evaporated is limited by the heat of H2 available from 250°C to the pinch point with the evaporation temperature (or critical temperature in case of supercritical fluid). RE347mcc has a smaller enthalpy of evaporation (equal to 36.95 kJ/kg) compared to R1336mzz (63.65 kJ/kg), and this allows the generation of a larger mass flow rate of vapor using only the heat available for the evaporator in H2. For R1336mzz, the mass flow rate of generated vapor is limited by the heat available in H2 for evaporation, and this causes a considerable reduction of heat recovery compared to the thermodynamic optimization. The ORC using the mixture shares the same issue as R1336mzz because of the large heat capacity of the supercritical fluid around the critical temperature (between 150 and 165°C), equal to 143 kJ/kg. For R1336mzz and the mixture given the limited mass flow rate of working fluid raised by the evaporator (for the mixture, the high-temperature heat exchanger HX3), it is sufficient to use the regenerator and the economizer in H1 for liquid preheating. For RE347mcc, given the larger mass flow rate of fluid, another economizer is necessary to preheat the liquid recovering heat from H2 leading to a higher heat recovery rate compared to the other two fluids (this is evident looking at the outlet temperatures of stream H2 reported in Figures 6A–C).
For the scenario with high electricity prices (e.g., the plant benefits from incentives), the amount of recovered heat and the mass flow rate of working fluids are very close to the thermodynamic target. As already pointed out by Elsido et al. (2019) in a similar analysis, since the electricity revenues more than compensate for the investment cost, the energy performance has the major weight on the plant total annual cost (or net present value). Consequently, the economic optimum lies close to the thermodynamic one. Compared to the solutions found for the low electricity price, the HENs of the solutions found for the high electricity price scenario use one more heat exchanger for RE347mcc and the mixture, and two more heat exchangers for R1336mzz (see Figures 7A–C). The cost of the HENs increases by +31% (RE347mcc), +62% (isobutane/isopentane mixture), and +92.5% (R1336mzz) with respect to the low electricity price solutions. As far as the economic performance is concerned, the cycles using RE347mcc and the isobutane/isopentane mixture achieve the best economic performance, an annualized net profit of about 1.65 M$/year, with a negligible (1%) difference. The ORC using R1336mzz features similar power output to RE347mcc, but its TAC is mainly penalized by the higher heat exchanger costs (+7.3%). Due to the small availability of vapor desuperheating heat, the regenerator gives only a marginal contribution and liquid economization needs to be performed to recover low temperature heat from H1 and (specially) H2, which features a very poor heat transfer coefficient. In comparison, the cycles with R347mcc and the mixture can preheat more the liquid with the regenerator and save some heat transfer area of the economizers. This is clear from the comparison of the solutions obtained for RE347mcc and R1336mzz (see Figures 7A,B): the ORC configuration and the HEN scheme are the same for the two fluids, as well as the amount of heat recovered from the heat sources (the outlet temperature of H2 is equal to 75–76°C in the two cases), but the amount of heat exchanged in the regenerator for the case using RE347mcc is 84% larger than in the case employing R1336mzz. Therefore, the overall HEN cost of the latter is 7.3% larger than the heat exchangers cost of the case with RE347mcc, leading to a worse economic performance.
Conclusions
For both pure fluids and mixtures, there is clear relation between the maximum achievable exergy efficiency and the critical temperature. The fluids achieving the highest efficiency feature a critical temperature in the range 80–84% of the maximum heat source temperature. The maximum efficiency cycle turns out to have a turbine inlet pressure close to the critical one. As an exception, R115, R143a, and propylene achieve close to maximum efficiency employing a highly supercritical turbine inlet pressure (their critical temperature is only 66–69% of the maximum heat source temperature).
The first ranked pure fluid is RE347mcc, which can achieve an exergy efficiency of 85.28% (corresponding to an ORC net power target of 3.53 MW) with a subcritical regenerated ORC. It appears to be a promising candidate since it is non-flammable, its ODP is zero, and the GWP is not excessive (530). If flammable fluids are discarded because of safety issues, the most promising alternatives seem to be R1336mzz (GWP = 2) and R1233zde (GWP = 1). In all solutions, the regenerator of the ORC is used to compensate for the relative scarcity of available heat below 80°C.
The use of binary zeotropic mixtures with optimized composition leads to a gain in exergy efficiency of 2.8 percentage points (+3.3% of ORC net power target). The most efficient solution employs a mixture of isobutane/isopentane (0.66/0.34) with a supercritical regenerated ORC. The efficiency gain compared to the pure fluids is mainly due to the temperature glide occurring in condensation.
The technoeconomic optimization, performed for RE347mcc, R1336mzz, and the mixture of isobutane/isopentane, shows that RE347mcc is the best option for low electricity prices (in absence of incentives). Its optimized ORC maintains good efficiency (generating 88% of the target mass flow rate of ORC vapor) as the electricity revenues more than compensate for the equipment costs. As a comparison, the optimized cycle using R1336mzz has an appreciable reduction of ORC mass flow rate compared to the target value (it generates only 72% of the target mass flow rate). The main reason appears to be the pinch point at the evaporator (due to larger enthalpy of evaporation of R1336mzz compared to RE347mcc) that limits the mass flow rate. Another important result is the economic advantage of using the regenerator to preheat the liquid in place of the economizers (which would recover heat from H1 and H2). This is due to the higher heat transfer coefficient of organic fluids compared to stream H1 and H2 (air). For high electricity prices, the economic optimum becomes close to the thermodynamic optimum in terms of energy performance and working fluid mass flow rates. Differences between economic performance of the different fluids are mainly due to the cost of the heat exchangers, favoring the fluid (RE347mcc) with larger regenerator.
The mixture results showed to be economically advantageous only for high electricity prices, although the relative difference compared to RE347mcc is small. The gain can likely not compensate for the extra equipment costs related to fluid flammability (i.e., need of a thermal oil loop) and to prevent fluid leakages (important issue for zeotropic mixtures), not considered in this work.
As far as fluid selection criteria are concerned, this work has shown that the thermodynamic performance of ORCs mainly depend on the critical temperature of the fluids while the economic performance is influenced also by other parameters, such as the de-superheating heat (available for the regenerator) and the evaporation enthalpy (influencing the heat integration with the available hot streams and the raised mass flow rate of working fluid).
Author Contributions
All authors listed have made a substantial, direct and intellectual contribution to the work, and approved it for publication.
Conflict of Interest Statement
The authors declare that the research was conducted in the absence of any commercial or financial relationships that could be construed as a potential conflict of interest.
References
Astolfi, M., Martelli, E., and Pierobon, L. (2017). “Thermodynamic and technoeconomic optimization of organic rankine cycle systems,” in Organic Rankine Cycle (ORC) Power Systems, eds E. Macchi and M. Astolfi (Woodhead Publishing), 173–249. doi: 10.1016/B978-0-08-100510-1.00007-7
Azzolin, M., Berto, A., Bortolin, S., and Del Col, D. (2017). Heat transfer degradation during condensation of non-azeotropic mixtures. J. Phys. Conf. Ser. 923:012017. doi: 10.1088/1742-6596/923/1/012017
Azzolin, M., Bortolin, S., and Del Col, D. (2016). Predicting methods for flow boiling heat transfer of a non-azeotropic mixture inside a single microchannel. Heat Transfer Eng. 37, 1136–1147. doi: 10.1080/01457632.2015.1111114
Braimakis, K., Preißinger, M., Brüggemann, D., Karellas, S., and Panopoulos, K. (2015). Low grade waste heat recovery with subcritical and supercritical Organic Rankine Cycle based on natural refrigerants and their binary mixtures. Energy 88, 80–92. doi: 10.1016/j.energy.2015.03.092
Campana, F., Bianchi, M., Branchini, L., De Pascale, A., Peretto, A., Baresi, M., et al. (2013). ORC waste heat recovery in European energy intensive industries: energy and GHG savings. Energ. Convers. Manage. 76, 244–252. doi: 10.1016/j.enconman.2013.07.041
Cavallini, A. (2017). “Heat transfer and heat exchangers,” in Organic Rankine Cycle (ORC) Power Systems, eds E. Macchi and M. Astolfi (Woodhead Publishing), 397–470. doi: 10.1016/B978-0-08-100510-1.00013-2
Chen, C.-L., Li, P.-Y., and Le, S. N. T. (2016). Organic Rankine cycle for waste heat recovery in a refinery. Ind. Eng. Chem. Res. 55, 3262–3275. doi: 10.1021/acs.iecr.5b03381
Chys, M., van den Broek, M., Vanslambrouck, B., and De Paepe, M. (2012). Potential of zeotropic mixtures as working fluids in organic Rankine cycles. Energy 44, 623–632. doi: 10.1016/j.energy.2012.05.030
Desai, N. B., and Bandyopadhyay, S. (2009). Process integration of organic Rankine cycle. Energy, 1674–1686. doi: 10.1016/j.energy.2009.04.037
Duran, M. A., and Grossmann, I. E. (1986). Simultaneous optimization and heat integration of chemical processes. AICHE J. 32, 123–138. doi: 10.1002/aic.690320114
Elsido, C., Martelli, E., and Grossmann, I. E. (2018). A bilevel decomposition method for the simultaneous synthesis of utility systems, Rankine cycles and heat exchanger networks. Comput. Aided Chem. Eng. 43, 373–378. doi: 10.1016/B978-0-444-64235-6.50068-1
Elsido, C., Martelli, E., and Grossmann, I. E. (in press). A bilevel decomposition method for the simultaneous heat integration synthesis of steam/Organic Rankine Cycles. Comput. Chem. Eng. doi: 10.1016/j.compchemeng.2019.05.041
Elsido, C., Martelli, E., and Kreutz, T. (2019). Heat integration and heat recovery steam cycle optimization for a low-carbon lignite/biomass-to-jet fuel demonstration project. Appl. Energy 239, 1322–1342. doi: 10.1016/j.apenergy.2019.01.221
Elsido, C., Mian, A., Marechal, F., and Martelli, E. (2017b). A general superstructure for the optimal synthesis and design of power and inverse Rankine cycles. Comput. Aided Chem. Eng. 40, 2407–2412. doi: 10.1016/B978-0-444-63965-3.50403-7
Elsido, C., Mian, A., and Martelli, E. (2017a). A systematic methodology for the techno-economic optimization of Organic Rankine Cycles. Energy Procedia 129, 26–33. doi: 10.1016/j.egypro.2017.09.171
ENTSOE (2019). Market Reports: European Transmission Tariffs. Available online at: https://www.entsoe.eu/publications/market-reports/#european-transmission-tariffs (accessed August 4, 2019).
E. P. R. Institute (1993). Technical Assessment Guide (TAG) Electricity Supply, TR-102276-V1R7. Palo Alto, CA: EPRI.
Hipólito-Valencia, B. J., Rubio-Castro, E., Ponce-Ortega, J. M., Serna-González, M., Nápoles-Rivera, F., and El-Halwagi, M. M. (2013). Optimal integration of organic Rankine cycles with industrial processes. Energ. Convers. Manage. 73, 285–302. doi: 10.1016/j.enconman.2013.04.036
IEA and Nordicetp (2016). Nordic Energy Technology Perspective 2016, ODEC/IEA. Oslo: Nordic Energy Research.
Invernizzi, C. M. (2013). “Closed power cycle,” in Thermodynamic Fundamentals and Applications (Brescia: Springer). 109–111.
Invernizzi, C. M., Iora, P., Bonalumi, D., Macchi, E., Roberto, R., and Caldera, M. (2016). Titanium tetrachloride as novel working fluid for high temperature Rankine cycles: thermodynamic analysis and experimental assessment of the thermal stability. Appl. Therm. Eng. 107, 21–27. doi: 10.1016/j.applthermaleng.2016.06.136
Kalitventzeff, B., and Maréchal, F. (1999). Targetting the optimal integration of stram networks: mathematical tools and methodology. Comput. Chem. Eng. 23, S133–S136. doi: 10.1016/S0098-1354(99)80034-9
Karellas, S., Leontaritis, A. D., Panousis, G., Bellos, E., and Kakaras, E. (2013). Energetic and exergetic analysis of waste heat recovery systems in the cement industry. Energy 58, 147–156. doi: 10.1016/j.energy.2013.03.097
Kemp, I. C. (2006). Pinch Analysis and Process Integration, 2nd Edn. Abingdon: Butterworth-Heinemann, 15–36.
Kruse, H., and Rinne, F. (1992). “Performance and leakage investigations of refrigeration and airconditioning systems using refrigerant mixtures as working fluids,” in International Refrigeration and Air Conditioning Conference (West Lafayette, IN) 621–30.
Ladam, Y., Solheim, A., Segatz, M., and Lorentsen, O. (2011). Heat recovery from aluminium reduction cells. Light Metals 2011, 393–398. doi: 10.1002/9781118061992.ch70
Lampe, M., Stavrou, M., Bücker, M., Gross, J., and Bardow, A. (2014). Simultaneous optimization of working fluid and process for organic Rankine cycles (ORCs) using PC-SAFT. Ind. Eng. Chem. Res. 53, 8821–8830. doi: 10.1021/ie5006542
Lemmon, E. W., Huber, M. L., and McLinden, M. L. (2013). NIST Standard Reference Database 23: Reference Fluid Thermodynamic and Transport Properties-REFPROP. Version 9.1. National Institute of Standards and Technology.
Lemmon, E. W., and McLinden, M. O. (2001). Method for Estimating Mixture Equation of State Parameters. National Institute of Standards and Technology.
Linke, P., Papadopoulos, A., and Seferlis, P. (2015). Systematic methods for working fluid selection and the design, integration and control of organic Rankine cycles-A review. Energies 8, 4755–4801. doi: 10.3390/en8064755
Linnhoff, B. (1979). Themrodynamic analysis in the design of process networks, Ph.D. Thesis. Leeds: The University of Leedss.
Martelli, E., and Amaldi, E. (2013). PGS-COM: a hybrid method for constrained non-smooth black-box optimization problems. Brief review, novel algorithm and comparative evaluation. Comput. Chem. Eng. 63, 108–139. doi: 10.1016/j.compchemeng.2013.12.014
Martelli, E., Capra, F., and Consonni, S. (2015). Numerical optimization of combined heat and power Organic Rankine Cycles-Part A: design optimization. Energy 90, 310–328. doi: 10.1016/j.energy.2015.06.111
Martelli, E., Elsido, C., Mian, A., and Marechal, F. (2016). Synthesis of heat exchanger networks and utility systems: Sequential initialization procedure and simultaneous MINLP algorithm. Comput. Aided Chem. Eng. 38, 1450–1454. doi: 10.1016/B978-0-444-63428-3.50246-0
Martelli, E., Elsido, C., Mian, A., and Marechal, F. (2017). MINLP model and two-stage algorithm for the simultaneous synthesis of heat exchanger networks, utility systems and heat recovery cycles. Comput. Chem. Eng. 106, 663–689. doi: 10.1016/j.compchemeng.2017.01.043
Nordpool (2019). Day-Ahead Prices. Available online at: https://www.nordpoolgroup.com/Market-data1/Dayahead/Area-Prices/ALL1/Yearly/ (accessed August 4, 2019).
Palma-Flores, O., Flores-Tlacuahuac, A., and Canseco-Melchor, G. (2014). Optimal molecular design of working fluids for sustainable low-temperature energy recovery. Comput. Chem. Eng. 72, 334–339. doi: 10.1016/j.compchemeng.2014.04.009
Papadopoulos, A., Stijepovic, M., and Linke, P. (2010). On the systematic design and selection of optimal working fluids for organic Rankine cycles. Appl. Therm. Eng. 30, 760–769. doi: 10.1016/j.applthermaleng.2009.12.006
Sami, S. M. (2010). Energy and exergy analysis of new refrigerant mixtures in an organic Rankine cycle for low temperature power generation. Int. J. Ambient Energy. 31, 23–32. doi: 10.1080/01430750.2010.9675805
Scaccabarozzi, R., Tavano, M., Invernizzi, C. M., and Martelli, E. (2018). Comparison of working fluids and cycle optimization for heat recovery ORCs from large internal combustion enegines. Energy 158, 396–416. doi: 10.1016/j.energy.2018.06.017
Solheim, A. (2018). Inert anodes-The blind alley to environmental friendliness? in TMS 2018. Light Metals 2018, 1253–1260. doi: 10.1007/978-3-319-72284-9_164
Wang, E., Zhang, H., Fan, B., Ouyang, M., Zhao, Y., and Mu, Q. (2011). Study of working fluid selection of organic Rankine cycle (ORC) for engine waste heat recovery. Energy 36, 3406–3418. doi: 10.1016/j.energy.2011.03.041
World Aluminium Website of the International Aluminium Institute (2019). Available online at: http://www.world-aluminium.org/statistics/primary-aluminium-smelting-energy-intensity/#data (accessed January 03, 2019).
Yang, K., Zhang, H., Wang, E., Song, S., Bei, C., Chang, Y., et al. (2014). Study on mixed working fluids with different compositions in Organic Rankine Cycle (ORC) systems for vehicle diesel engines. Entropy 16, 4769–4787. doi: 10.3390/e16094769
Yee, T., and Grossmann, I. (1990). Simultaneous optimization models for heat integration-II. Heat exchanger network synthesis. Comput. Chem. Eng. 14, 1165–1184. doi: 10.1016/0098-1354(90)85010-8
Yu, H., Eason, J., Biegler, L. T., and Feng, X. (2017). Simultaneous heat integration and techno-economic optimization of Organic Rankine Cycle (ORC) for multiple waste heat stream recovery. Energy, 322–33. doi: 10.1016/j.energy.2016.12.061
Yu, M., Gudjonsdottir, M. S., Valdimarsson, P., and Saevarsdottir, G. (2018). Waste heat recovery from aluminum production. Miner. Met. Mater. Ser. F6, 165–178. doi: 10.1007/978-3-319-72362-4_14
Zhang, J., Zhang, H., Yang, K., Yang, F., Wang, Z., Zhao, G., et al. (2014). Performance analysis of regenerative organic Rankine cycle (RORC) the pure working fluid and the zeotropic mixture over the whole using operating range of a diesel engine. Energ. Convers. Manage. 84, 282–294. doi: 10.1016/j.enconman.2014.04.036
Zhang, L. H., Wu, L. J., Hu, H. R., and Gao, X. J. (2013). Comparison and optimization of low temperature flue gas power generation systems in iron and steel enterprises. Reneng Dongli Gongcheng/J. Eng. Therm. Energy Power 28, 257–261.
Appendix
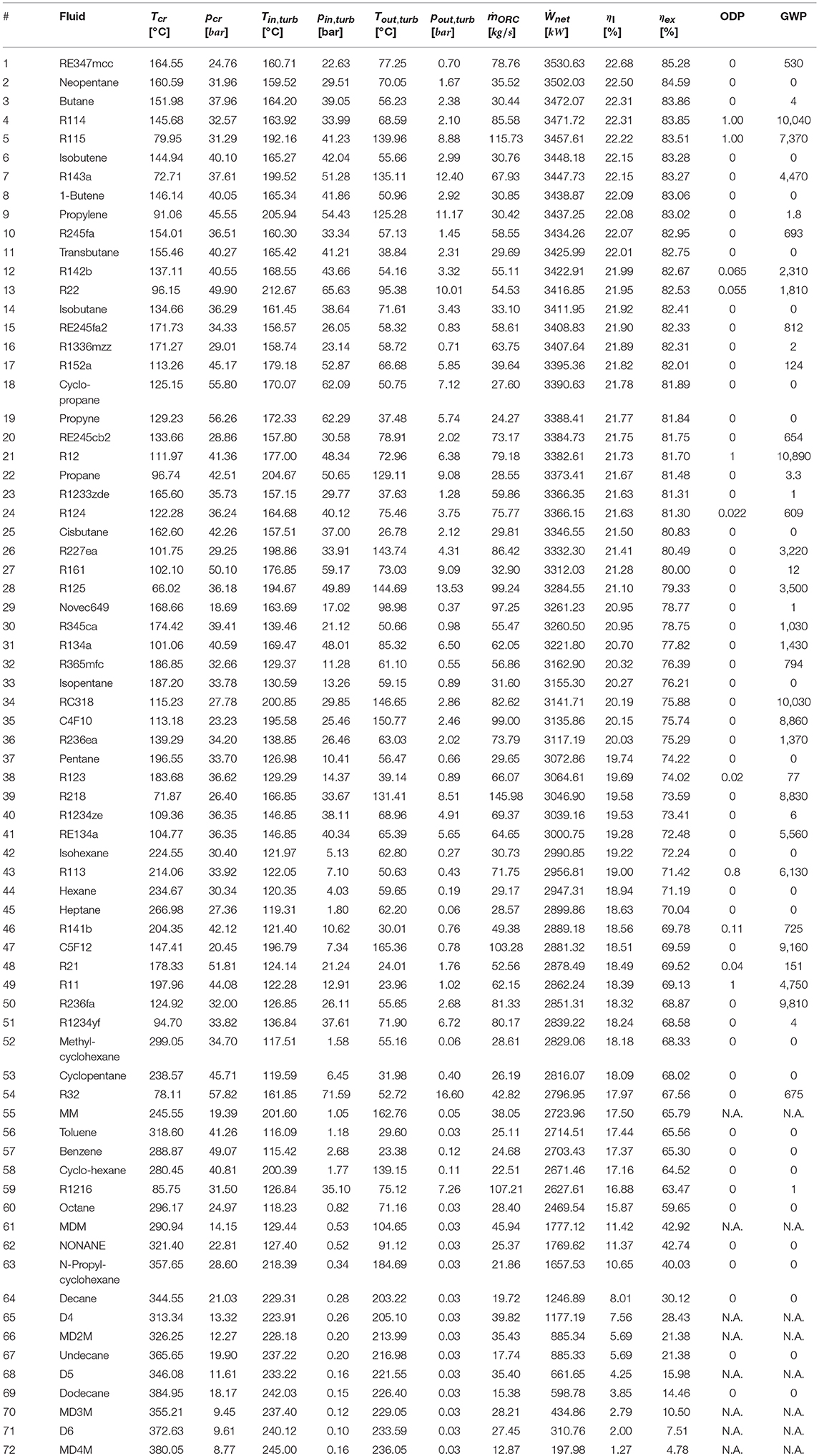
Table A1. Thermodynamic optimization results of all pure fluids ranked according to the exergy efficiency.
Keywords: organic rankine cycle (ORC), waste heat recovery, optimization, heat integration, zeotropic mixture, working fluid
Citation: Castelli AF, Elsido C, Scaccabarozzi R, Nord LO and Martelli E (2019) Optimization of Organic Rankine Cycles for Waste Heat Recovery From Aluminum Production Plants. Front. Energy Res. 7:44. doi: 10.3389/fenrg.2019.00044
Received: 19 January 2019; Accepted: 23 April 2019;
Published: 20 June 2019.
Edited by:
Vincent Lemort, University of Liège, BelgiumReviewed by:
Xiaofeng Guo, ESIEE Paris, FranceSantanu Bandyopadhyay, Indian Institute of Technology Bombay, India
Copyright © 2019 Castelli, Elsido, Scaccabarozzi, Nord and Martelli. This is an open-access article distributed under the terms of the Creative Commons Attribution License (CC BY). The use, distribution or reproduction in other forums is permitted, provided the original author(s) and the copyright owner(s) are credited and that the original publication in this journal is cited, in accordance with accepted academic practice. No use, distribution or reproduction is permitted which does not comply with these terms.
*Correspondence: Emanuele Martelli, ZW1hbnVlbGUubWFydGVsbGlAcG9saW1pLml0