- 1Department of Computer Science, University of Thessaly, Lamia, Greece
- 2Department of Environmental Engineering, Western Macedonia University of Applied Sciences, Kozani, Greece
- 3Department of Electrical Engineering, University of Applied Sciences of Thessaly, Larissa, Greece
- 4Unit of Environmental Science & Technology, School of Chemical Engineering, National Technical University of Athens, Athens, Greece
Sustainable Waste Biorefinery Facilities (WBFs) represent multifactorial systems that necessitate the organization, cooperation and the acceptance of different social stakeholders. However, these attempts have become targets of environmental, social and legal oppositions despite their obvious economic benefits. The variety of ambivalent and heterogeneous external effects of such projects result in either local support or opposition to the facility, which in turn becomes a critical factor affecting facility location decisions, and subsequent success of a WBF. Research has shown that simple surveys do not sufficiently measure social acceptance of such endeavors, and in most cases, local community factors dominate other external valuable impacts. In the current study, a novel Fuzzy Cognitive Map (FCM) modeling approach is proposed in order to analyze the socio-economic implications and to overcome multiple uncertainties occurring in sustainable WBF development and implementation. The primary investigation relates to the factors that influence the development of organic or chemical treatment of waste by the local communities and the competent authorities. The determination of concepts involved in the FCM modeling depends on a hybrid approach where both experts' opinion and statistical results from questionnaires distributed to stakeholders participate in the concept circumscription, thus identifying the centrality of each node in the model. Several steady state and dynamic analysis scenarios show the influence of driver concepts to receiver concepts on the social aspect FCM constructed.
Introduction
Our society well-being remains heavily dependent on non-renewable fossil sources that provide heat, electricity, pharmaceuticals, transportation and food production. The major problem is though that our reliance on non-renewable energy sources has important negative environmental impacts, such acidification of oceans and global warming. To safeguard present livelihoods and offer development pathways for non-industrialized societies, energy sustainability is needed. Thus, the requirement to use renewable resources and to minimize waste becomes apparent. For that reason, an increasing number of individuals, companies, organizations and governments turn toward the development of biorefineries and biofuel production (Halder et al., 2012). This rapid growth is predominantly supported by major associations, such as central and local agencies whose primary goal is to promote policies, regulations and R&D initiatives that will lead to the increased production and use of sustainable biofuels. For the biorefinery industry the selection of the installation location is of great importance, as this is the major parameter impacting the initial capital of the project, the transportation costs for the raw materials and the produced fuel, which in turn become secondary factors affecting the retail price of the product. It must be noted here that a major role affecting the decision on the installation location is the acceptance of the local community at a first level and the approval of the local competent authorities at a second level. The immediate social acceptance drastically diminishes installation time delays and indirectly decreases the installation costs. On the other hand, companies believe that they are the ones that should decide where to locate the plants, arguing on the assumption that producers are economic free agents, evaluating sites as if all counties are contenders for their business, weighing the availability of feedstocks along with their infrastructure needs, operating without ties to localities, and being subject to enticement from policy incentives (Walter and Gutscher, 2011; Tigges and Noble, 2012; Fortenbery et al., 2013; Nizami et al., 2017a,b). Regardless of any minor disadvantages in relation to bioeconomy, the vast majority of researchers support the broad participation of various stakeholder groups and citizen associations, in order to “chart a strategic plan” to promote crucial importance of Waste Biorefinery Facility (WBF) growth. To be more specific, the following important impacts necessitate the urgency to open up the discussion about WBFs into the general public and include all affected stakeholders and major citizen groups (McGuire et al., 2017):
• The engagement of local citizens and stakeholders in relation to WBF growth are established at the regional level and mostly at the places where the establishments are meant to be built.
• The bigger the interaction with the major stakeholder groups, the bigger the increase of mutual understanding and agreement when dealing with collisions and conflicts of various social groups of citizens.
• The benefits of the local and regional rural economy are maximized when the WBF growth is “mainstreamed” and the vast majority of local citizens get advantage of it.
• The engagement of the general public and the major stakeholder groups makes better use of good practices in relation to the efficient use of waste-based resources.
• The mutual decision making process among all affected stakeholder groups, scientists, researchers, and non-governmental organizations ensures an integrated methodology resulting in a sustainable bioeconomy.
Certainly, there are also negative effects emanating by WBF growth. However, appropriate management of such inverse impacts ends up in promoting new sustainability standards and a better policy making framework than the existed one. On the other hand, high levels of public acceptance are essential if local authorities plan substantial increases in new renewable energy power plants for a certain area, as such projects may impose both positive and negative externalities on the local communities concerned. Apart from the increase of local jobs created and the stimulation of the local economy, such attempts positively affect local tax revenues, while at the same time purchases of local goods and services are also boosted. Nevertheless, the most common phenomenon occurring is a social syndrome usually referred as “Not In My Back Yard (NIMBY)” (Dear, 1992; van der Horst, 2007), reflecting residents' opposition to a proposed development in their local area. This characteristic phenomenon often carries the connotation that local residents are only opposing the development of waste biorefinery plants because they are close to them, and that they would tolerate or support such installations if they were built further away (Haddad et al., 2009; Lambert, 2009; CTV Kitchener, 2012; Lakioti et al., 2017). Characteristic cases are the ones mentioned in Selfa (2010), which investigate the opposition to biofuel facilities by local communities in three different locations in Kansas and Iowa. Lambert (2009) also mentions a good practice by a consulting company, which has helped tremendously various WBF corporations to address the NIMBY wars triggered in 135 locations. However, more and more researchers lately tend to discard the NIMBY-thesis due to the limitations imposed relatively to the human attitudes in obscuring the actual reasons for rejecting any kind of land use and particularly any WBF related land use (Devine-Wright, 2009; Wolsink, 2012).
Similar thesis relative to land use (Brion, 2015; Ciment, 2015) is the “Locally Unwanted Land Use” (LULU) syndrome. This basically argues that there is a land use creating externality costs on those citizens living within close proximity and these costs include potential health hazards, poor aesthetics, or reduction in home values. Therefore, the development of such facilities, with an increased level of hazards, need to be created for the greater benefits of the society as a whole, in order to get global acceptance and supportiveness.
The design and control of engineering systems such as waste biorefineries is a complex decision-making process in which multiple conflicting objectives of social, economic, and environmental nature must be taken into account. The key sustainability factors that drive the development and management of WBF or change the status quo of an existing waste management system can be summarized and categorized into: (a) environmental (climate change, land use, depletion of natural resources etc.), (b) social (local demographics, public resistance/resilience, NIMBY-LULU, public participation in making policies etc.), (c) competent authorities (institutional and administrative policies for WBF development, regional and municipal politics and legislation, structural development etc.), (d) economic (development funding, structural efficiency in installation minimizing costs, pricing models, secondary materials market etc.), and (e) technological (collection and transfer system, treatment technologies, waste stream composition and change) (Bovea and Powell, 2006; den Boer and Lager, 2007; Beigl et al., 2008; Graymore et al., 2008; Rehan et al., 2018).
The decision and policy making relatively to the development and sustainability of such large-scale waste biorefinery projects is done by stakeholders that influence these operations and sometimes their opinion is conflicting with others. Stakeholders include each of people or groups who are affected by technology developments or can affect that. Many of the barriers to access a successful performance of the projects could be the result of lack of acceptance of such projects by these social groups (Hosseini et al., 2018). From a socio-political point of view, acceptance of projects by core stakeholders and political actors has critical importance. When challenge is programming and increasing pub1ic participation incentive, cooperation with stakeholders and policy makers and also analysis of the acceptance level is important. For that reason, the decision-making model that will be adopted should provide quantifiable metric enabling systematic comparisons of the effect of different compromise solutions on the dissatisfaction of the stakeholders. With that model, it must be possible to make a thorough analysis relatively to the fairness of the decision taken and how decisions affect the opinions of specific stakeholder communities. Because of the diversity of stakeholder perceptions and attitudes, the model should also be able to analyze if a certain stakeholder or subset of stakeholders fully dictates the nature of a decision and therefore dissatisfaction of the rest of the stakeholders occurs. Stakeholder opinions along with polling procedures are the main tools that can be used for the development of such decision and policy making models, as they alleviate the conflicts created due to the way the information is presented and processed by the stakeholders (Cucek et al., 2013; Geraili and Romagnoli, 2015; Liew et al., 2015; Dowling et al., 2016).
Even though there is not an exact consensus on all environmental, economic and social impacts of building and managing WBFs, in the current paper we outline how an understanding of the processes of bridging cognitive and socio-political legitimacy can be used in the formation of a Fuzzy Cognitive Map (FCM) as a decision and policy making tool for the management of WBFs. Unlike all previous attempts of modeling such a problem, this study primarily depends on the local society stakeholder perceptions, opinions, attitudes and expectations to model the installation of new sites in rural and suburban areas.
The structure of the paper is as follows: In the next section, all the material and methods related to WBFs and FCMs are presented. More specifically, the integration of stakeholders in the WBF management decision process, the multi-criteria analysis and the scenario-based analysis methodologies are reviewed. Also, the characteristics of the FCM including the most popular activation and transformation functions as well as the algorithm of FCM construction from experts and the development of FCM from learning and training techniques are quoted. In section three, the application of FCMs in analyzing Social Acceptance for WBFs, developing a decision making FCM for a case study of WBF development in Thessaly, Greece, is discussed. Furthermore, due to the amount of concepts and the FCM complexity generated, the social part of the FCM is focused, by discussing its steady and dynamic state analysis and how the driver concepts influence the social acceptance. In the final section, conclusions and future challenges on the issue are highlighted.
Materials and Methods
Integration of Stakeholders in the WBF Development Decision Making Process
Analyzing waste biorefinery development and management decision making has been a challenge in the recent years along with other similar problems (Lin and Yang, 2018; Stadler et al., 2018). Most of the researchers initially based their analyses on science, consensus, structured and multi-criteria decision making approaches according to Gregory et al. (2012). With a focus on methods to involve citizens and other stakeholders in decision-making processes Van Asselt and Rijkens-Klomp (2002) identified eight different methods such as focus groups, scenario analysis, scientific stakeholder workshops, policy exercises, participatory modeling building, citizens' juries, consensus conferences, and participatory planning. However, when it comes to make decisions, the integration of stakeholders along with the opinion differences created between them and the experts in co-producing knowledge forces the analysts to use more widely approved methodologies. Among these methods, multi-criteria analysis, scenario analysis and rationality theory analysis are the ones to bring stakeholders and experts together in triggering learning processes that can make science more sensitive for societal problems (Mielke et al., 2016).
Multi-Criteria Analysis
The basic idea behind Multi-Criteria Analysis (MCA) is to set up measurable parameters that affect the process under study and to identify well established units for these impacts along with a clear ranking methodology. This formalized procedure allows decision makers to select the most desirable alternative among all available choices. Whenever there exist trade-offs between various alternatives, MCA clarifies values associated with decisions and evaluates these different alternatives including additional criteria. More specifically, the alternatives are further classified and clustered into criteria, which are weighted separately and then recombined back together onto the initial grading scale. Munda (2004, 2008). MCA methodologies utilize predominantly quantitative data. On the other hand, when the incorporate of stakeholders' perspectives into the decision process is attempted, their input usually consists of qualitative information making MCA methods insufficient to integrate this input. To overcome this problem, researchers synthesize traditional social research techniques (surveys, discourse based evaluation, narrative analysis, and value integration methods) with MCA for addressing the integration of stakeholders' perspectives. Thus, alternative MCA methodology variants have been developed to overcome the aforementioned challenge (Stirling, 2006; Yatsalo et al., 2007). For our case, the most important variant is the one that corresponds to Social Multi-Criteria Evaluation (SMCE), as emphasis is given mostly to stakeholder engagement in making decisions and also on the operational framework useful to answer the following question: how can the local society and the local competent authorities integrate a plurality of technical aspects and social views into its ex-ante impact assessment in a coherent and transparent manner for the development and management of WBFs. According to Scolobig and Lilliestam (2016) the phases of SMCE are: (a) institutional analysis, (b) alternatives and criteria, (c) assessment of the criteria, and d) identification of the most desirable alternative. Finally, the most critical parameter in this impact assessment technique is to produce a decision policy based on the following three main objectives:
1. Effectiveness: the degree to which the policy objectives are achieved (in terms of goals or levels of output) and the problems identified are solved,
2.Efficiency: making sure that the local society of experts and stakeholders has expressed its commitment to ensure that its proposals meet policy goals at minimum cost and/or taking into account an analysis of costs and benefits and their distribution among the stakeholders affected, and
3. Coherence: with other existing local society rules and policies.
Scenario-Based Decision Making
In general, the Scenario-Based methodology simply presents all the alternative scenarios evaluating the impacts they impose in decision making without the inclusion of a decision-making directive. After evaluating the impacts of each scenario, the decision maker should be able to make a decision related to the problem at hand, evaluating the less expensive alternative. A critical task in this process is the involvement of stakeholders in suggesting different scenarios for evaluation, or evaluating the existing ones, or setting scenario boundary conditions together with the decision makers (Kok et al., 2015; Trutnevyte et al., 2016). Furthermore, as we already explained, the difficulty stands on the fact that all the qualitative information coming from the stakeholders' input needs to be converted into quantitative. Therefore, it is useful to incorporate Fuzzy Logic techniques to achieve this defuzzificaton process. To summarize, the key steps of the engagement of the stakeholders in scenario-based approaches (Scolobig and Lilliestam, 2016) are: (a) the elicitation of stakeholders' perspectives, (b) quantification of inputs to feed the model parameters and (c) the scenario ranking and identification of actions to enact a particular scenario.
Plural Rationality Decision Making
In this case, decision and policy making is based on a solution as a result of a compromising process among multiple “voices” through extraction of multiple stakeholder perspectives. This methodology is based on the theory of plural rationality, which suggests that all stakeholder perspectives are organized and relate to five different issues, namely the hierarchical, the egalitarian, the individualistic, the fatalistic, and the autonomous. These categories share common attitudes and views of stakeholders. Thus, for this method, the key steps that need to be taken are: (a) the elicitation of stakeholder perspectives, (b) the creation of technical-policy alternatives, (c) the construction of focus groups to discuss these alternatives and prioritize the actions needed to be taken, and finally (d) a thorough discussion to reach a decision.
Experts and Stakeholders
The increasing number of decision making models that can incorporate both expert opinions and a diverse collection of stakeholder perspectives creates inevitably a confusion in selecting the best analytic-deliberative-adaptive methodology for developing and managing WBFs. For this reason, we choose to base our approach on the inclusive definition of all the concepts made primarily by experts. At the same time, the model scrutiny will also utilize systematic and transparent procedures including as wide a range of stakeholders as possible. However, it must be noted that priority must be given to the quality of the enquiry for those responsible for waste biorefinery management and policy formulation for this kind of projects. For such systems, the efficiency of decision-making depends largely on the ability of decision-makers to analyse the complex cause and effect relationships and take productive actions based on the analysis. More specifically, the dynamics of large scale complex WBF reveal different components which affect each other, and these cause and effect relations show system behavior. All aforementioned arguments guide us to use system conceptualization graphs to understand all of the system aspects, such as FCMs.
Fuzzy Cognitive Mapping (FCM)
FCM is a qualitative or rather semi-quantitative and dynamic method to structure expert knowledge that aims to capture a person's perception of a particular issue in a diagrammatic format. FCM graphs provide both the modeler and the interviewee with an informal structured process having the ability to give additional beliefs, insights and concepts about a certain domain. Furthermore, the interrelations and interdependencies of these concepts are also revealed, providing information about how the change of one issue can affect the others. The selection of the FCM methodology to investigate the development and management of WBF is due to following advantages according to Ozesmi and Ozesmi (2004): (a) FCMs are well structured to imprint the complexity and the reason for the waste biorefinery model, (b) FCMs are capable to represent the quantitative and qualitative information obtained from the stakeholders' opinion, overcoming the lack of quantitative reliability of data due to uncertainty, and (c) FCMs are suitable to illustrate the effects of factor changing for the whole systems even though they are not able to make quantitative predictions. Therefore, FCMs are allowed to predict the effects of the policy taken under the “what-if ” scenario, having assumed that, since the real world is complex, knowledge can be obtained from the perception of people involved into a certain issue (Kosko, 1986, 1987).
Basic Concepts for FCMs
More rigorously, an FCM is a graphical representation of a system used to illustrate the cause and effect relations between nodes, thus giving us the opportunity to describe its behavior in a simple and symbolic way. In FCM graphs, nodes represent concepts, and arcs represent the perceived relationships between these concepts (Axelrod, 1976). These relationships in a FCM are logically imposed by connecting concepts via semantic or otherwise meaningful directed linkages showing the causality between them (Novak and Cañas, 2008). According to Gray et al. (2014), this causality in representing cognition by weighted arcs in a structural map is derived from constructivist psychology, which suggests that individuals interactively and collectively construct experience/knowledge developing internal associative representations that help to catalog, interpret, and assign meaning to environmental stimuli and experiences (Raskin, 2002). Another view of the FCMs is from the Neural Networks and Fuzzy Logic point of view. For that reason, learning techniques and algorithms can be borrowed and utilized to train the FCMs and adjust the weights of the concept interconnections.
In order to ensure the operation of the system, FCMs embody the accumulated knowledge and experience from experts who acquaint how the system operates under different conditions. For experts to conclude on which concepts to integrate, stakeholders give firstly feedback prior to knowledge extraction. Especially for decision policies made, this knowledge extraction is succeeded transforming all linguistic variables into numeric values via a defuzzification process. This produces a set of concepts denoted as Ci (i = 1, 2, …n) (graph nodes) with their interrelations denoted as wi(graph directed edges). After the defuzzification, concepts are assigned a value within the range [0, 1] and weights are assigned values in the range [−1, 1] to capture negative and positive causality. A positive value of the weight wij indicates that an increase (decrease) in the value of concept Ci results to an increment (decrement) of the concept's value Cj. Similarly, a negative weight wij indicates that an increase (decrease) in the value of concept Ci results to a decrement (increment) of the concept's value Cj, while a zero weight denotes the absence of relationship between concepts Ci and Cj, respectively. Figure 1 depicts a typical FCM.
Taking into consideration the interrelations between the concepts of a FCM, the corresponding adjacency matrix can easily be formed. Every concept Ci in the graph has a value Ai that expresses the quantity of its corresponding physical value derived after the defuzzification described above. The value Ai of Ci is computed in each simulation step and it basically indicates the influence of all other concepts Cj to Ci (inference). The most popular inference rules are: (a) Kosko's inference, (b) Modified Kosko's inference, and (c) Rescale inference, as shown in the following three activation functions, respectively.
Also f(.) is the threshold (transformation) function that can be: (a) bivalent, (b) trivalent, (c) sigmoid or (d) hyperbolic, as shown in the following four equations, respectively.
where λ is a real positive number (λ>0) that determines the steepness of the continuous function f and x is the value Ai(k) on the equilibrium point. It should be noted that the sigmoid threshold function ensures that the calculated value of each concept will belong to the interval [0, 1]. When the values of concepts can be negative and their values belong to the interval [−1, 1], the hyperbolic tangent function can be used instead.
FCM Development Using Expert Knowledge
Experts can use their knowledge in the area under study to develop an FCM by firstly identifying the main concepts involved and secondly indicating the causal relationships among these concepts. The final step is the calculation of the causal relationships strengths using either crisp numeric values within the range [−1, 1] or using linguistic variables and values that at second stage are defuzzified into numeric. Furthermore, experts can improve an existed FCM by collectively analysing the key characteristics of the system under study and reevaluating the structure and the interconnections of the graph using fuzzy conditional statements or fuzzy rules. The algorithm used for the development of a FCM is depicted below (Groumpos and Anninou, 2017):
Step 1 : Experts select the concepts Ci that constitute the FCM graph.
Step 2 : Each expert defines the causal relationship between any two concepts, if there exists one (positive, negative, neutral).
Step 3 : Experts carefully determine the value of the relationship between the two concepts.
Step 4 : Experts describe initially the causal influence using linguistic variables, such as “low,” “medium,” “high” etc. The sign of each weight (+ or –) represents the type of influence between concepts. There are three types of interconnections between two concepts Ci and Cj:
° wij ≥ 0 means that an increase or decrease in concept Ci causes the same result in concept Cj.
° wij ≤ 0 means that an increase or decrease in concept Ci causes the opposite result in concept Cj.
° wij = 0 means that there is no relation between concepts Ci and Cj.
The degree of influence between the two concepts is indicated by the absolute value of wij. During the simulation, the value of each concept is calculated using the following rule:
Learning Process in a FCM
In general, the manual process for developing an FCM described in section FCM Development Using Expert Knowledge can be used only when there is at least one expert with expertise on the problem under study. However, in some situations, a FCM could not be constructed because of the following reasons:
• There is no expert or stakeholders with direct interest to define a FCM.
• The experts' knowledge is different or with each other and they draw drastically different FCMs or even FCMs with minimum overlap of concepts.
• There are large amount of concepts and connections between them, which could not be drawn without mistakes (it occurs in high complexity problems).
For such extreme cases there has been developed a systematic way of constructing the FCM using a learning process, i.e., a process that will automatically determine the weights of the FCM, which best fits the decision-making and prediction problems. Diverse learning methods have been proposed by many researchers and they are based on the same techniques that used to train neural networks. These techniques are of three basic types namely: (a) Hebbian-based, (b) Population-based, and (c) Hybrid. Hebbian oriented learning requires data to be used alomg with a learning formula that regulates the adjustment of the cognitive map weights. The initial map before FCM learning in this case is established using experts' knowledge. Population-based learning algorithms on the other hand use evolution strategies to learn FCMs from data such as particle swam optimization (Koulouriotis et al., 2001), real-coded genetic algorithms (Stach et al., 2005), and the well-known big bang-big crunch algorithm (Yesil and Dodurka, 2013). Finally, hybrid learning approaches make use of both the effectiveness of Hebbian learning and the global search capability of evolutionary-based algorithms. Even though the hybrid approaches can learn FCMs with high accuracy, these approaches still suffer from the problem of converging to undesired states for the output concept values (Zhu and Zhang, 2008).
In the case of unsupervised learning algorithms, Hebbian-based methods use available data and a learning formula that is based on several modifications of Hebbian law, to iteratively adjust FCM weights. Typical Hebbian-based methods have been reported in the literature (Dickerson and Kosko, 1994; Huerga, 2002; Papageorgiou et al., 2003, 2004; Konar and Chakraborty, 2005; Stach et al., 2007, 2008).
In the case of population-based algorithms, such as simulated annealing, evolution-based and particle swarm optimization, the basic idea is to use the available input datasets in order to discover models that mimic the input data. It should be noticed that this method uses an objective criterion or a function to be optimized, thus making the method computationally intensive (for the genotype development and management). The primary goal is to find a near-optimal weight matrix based on the functional characteristics of the FCMs. Typical population-based methods have been published by many researchers (Koulouriotis et al., 2001; Papageorgiou et al., 2004; Mateou et al., 2005; Petalas et al., 2005; Stach et al., 2005; Alizadeh et al., 2007).
Finally, the hybrid method is implemented combining the previous two learning techniques using the coupling of differential evolution algorithm and nonlinear Hebbian learning algorithm, by using both the global search capabilities of evolutionary strategies and the effectiveness of the NHL rule (Papageorgiou and Groumpos, 2005). This hybrid learning module was applied successfully in real-world problems. Moreover, through the experimental analysis, the results showed that this hybrid strategy was capable to train FCM effectively, thus leading the system to desired states and determining an appropriate weight matrix for each specific problem (Zhu and Zhang, 2008).
Application of FCMs in Analyzing Social Acceptance for WBFs –Steady and Dynamic State Analysis
Description of the Case Study
The knowledge relating to FCM was applied in a case study considering a hypothetical development of a WBF. This study was supposedly applied to the district of Thessaly, Greece. Thessaly region is located in the central part of Greece (Coordinates: 39.6103° N, 22.0476° E) and has one of the two largest plains in Greece covering a total area of 14,037 km2. Also, there exist four major cities generating a huge amount of municipal solid waste among other types of waste making the area an ideal candidate for developing a WBF. The hypothetical location chosen was near the existed one, i.e., near the Parapotamos of Tempi municipality. The reason the location chosen were nearby the real location was not to create any opposition and prejudice in answering questionnaires by the local stakeholders and residents. Furthermore, we assumed that the heat and cooling customer was a local community. The main goal for the study was to assess the community attitudes toward the development and management of a WBF, measuring the degree of this acceptability. Apart from the site selection we also tried to keep the other characteristics of the hypothetical study as close as possible to the real case and only altering the ones that deal with the social issues. That is, our polling methods avoided to deal with: (a) the selection of energy conversion technologies and processes, (b) the product management policies, (c) the technological improvement, and (d) the economic and environmental sustainability. Even though we included a plethora of concepts relating to environmental, health, governmental, economic and technological issues, we primarily concentrated on the social concepts affecting the acceptance proposition or opposition of WBFs.
In order to draw the FCM, a diverse team of stakeholders and experts were interviewed to express their perceptions toward the environmental, health, social, governmental, economic and technological aspects relating to the development and management of WBFs. The diversity of the group imposed the inclusion of stakeholders such as local citizens, consumers, competent authority's government employees, institution representatives, farmers etc. Two experts were also interviewed, one with socio-economic and the other with chemical process engineering expertise. The experts were showed similar research works on the subject such as of Lopolito et al. (2009) and Sacchelli (2014). This is the reason that some of the concepts involved in the creation of the FCM were similar to the ones used in these two works. The total number of stakeholders raised to 23 and complemented by the interviews of the two experts. In total, the experts have identified 43 concepts, as it is explained in the following subsection focusing on the social acceptance of WBF operations. We must say that the number 43 resulted after the merging of similar concepts that were practically the same but with different linguistic description/labeling. It should also be noted that some concepts were dominant for most of the stakeholders and experts, but others were depended of their area of interest and expertise. The age of the involved participants in the study was between 29 and 59 years. The weight of the answers was calculated differently for the stakeholders and the experts though. More specifically, 40% of the weighted average of the answers was due to experts and the rest of 60% to the stakeholders following a widely known practice in developing and drawing FCMs. Relatively to the interviewing process, at first we described to the stakeholders the most relevant concepts to WBF and also the causal relationships between concepts using mostly natural language, so they could understand, digest and share among themselves the new information. Once the participants were given an assessment example of a similar problem, they were able to assign negative or positive causality. The values assigned to causality (weights) were of the fuzzy range [very high, high, medium to high, medium, medium to low, low, very law] and similarly on the negative scale. After the collection of interviews, the results were defuzzified and entered in the online software “Mental Modeler” (2018)1 to draw the FCM, which is depicted in Figure 2, and calculate the following:
• Total number of components
• Total number of connections
• Indegree and Outdegree of each component
• Connections per component
• Type of component (driver, ordinary, receiver)
• Centrality: absolute value of either a) overall influence in the model (all + and –relationships indicated, for entire model) or b) influence of individual concepts as indicated by positive (+) or negative (-) values placed on connections between components; indicates a) the total influence (positive and negative) to be in the system or b) the conceptual weight/importance of individual concepts (Kosko, 1986, 1987). The higher the value, the greater is the importance of all concepts or the individual weight of a concept in the overall model2.
• C/N: number of connections divided by number of variables (concepts)
• Complexity: ratio of receiver variables to transmitter variables
• Density: connections number compared to all possible connections number.
FCM Steady State Analysis
Table 1 shows the general statistics of the FCM and Table 2 the concept individualized properties described above. The complexity of the resulting FCM comes from the large amount of connections, but this is due to the nature of the concepts identified. It should be noted that we categorized these concepts into five classes, namely: (a) Environmental-Health, (b) Social, (c) Government-Laws, (d) Economics, and (e) Technological. An apparent similarity and increased interdependence of the concepts belonging to the same class can be observed. However, there is also an increased number of interconnections between concepts of different classes. This is because of the correlation of environment and health with societal issues and how these affect the decisions of local citizens when it comes to altering their lives.
It must be noticed that experts and stakeholders from the local community (citizens, consumers) referred to the social issues (Risk perception, Public Trust, Well-being of citizens, Inclusiveness) as the most crucial factors influencing the operation and management of such facilities, and regarded the technological and economics issues as secondary. On the other hand, experts and stakeholders coming from competent authorities such as government employees, institution representatives and chemical engineers gave extensive weight to the potential consequences of using WBF products and by-products and also to the resulting economic benefits (new investment and business plans, profit analysis for the companies involved etc.). Respectively, the average number of connections was 158, ranging from 123 to 209 according to different participants. For the merged FCM shown in Figure 2, a density of 0.115725 is deduced, with average Connections per Component raised up to 4.860465.
Another structural measure of FCM is the Hierarchy Index which is defined by the following equation and depends on the out degree (od) of each concept (node) in an FCM of N concepts (MacDonald, 1983).
When h is equal to 1, the mapping is completely hierarchical, and when it is equal to 0, the mapping is completely democratic (Ozesmi and Ozesmi, 2004). The hierarchy index of the FCM was calculated to be 0.137, making it very close to 0 and therefore highly democratic. Of course there is diversification of the hierarchy index in the case of the individual FCMs as opposed to the merged one. Obviously, the collective case increases the number of nodes, as it affects the in-degree and out-degree of these nodes, making the FCM more democratic and its system's steady state more resistant to the alterations of individual concepts. The concept with the highest centrality, as expected, was the “Acceptance” with a very high score of 24.83. Furthermore, the most central concepts directly affecting the Acceptance concept were the following, in decreasing order of their complexity: WBF Product Certification 11.32, Risk perception 11.08, Equity of decision making processes 6.64, Uncertainty toward the settlement of the new industry 6.51 and Well-being of citizens 6.09. This depicts the direct association of the social issues (primarily) and the environmental issues (secondly) to affect this decision.
To the best of the authors' knowledge, there does not exist any relevant research that could establish the current state and values of the environmental, health, social, governmental, economic and technological oriented concepts involved in the creation of the FCM depicted in Figure 2. For this reason, we consider the steady state of the FCM model as the initial scenario to start our analysis. In order to make evaluations and draw conclusions, we examined the worst case and the best case scenario as compared to the steady state. The worst case scenario is set up with all driver concepts to have the value of 0.1. On the opposite side the best case scenario is set up with all driver concepts to have the value of 1. In Figure 3 we depict the analysis where in the worst case scenario a decrease of about 1% is shown in the “Social Acceptance” receiver concept when it is compared to the original steady state scenario which was set as the base for the analysis. In the same figure, we also observe the biggest decrease in the “WBF Product Certification” concept rising up to 18% with the “Intervention of non-local businessman” to follow having an overall 11% decrease. These two values show that the relation of the driver concepts for this FCM to the “WBF Product Certification” and the “Intervention of non-local businessman” concepts is driven by what we call negative causality. The meaning of this great decrease of concept C7 and C14, respectively is that a small increase of the driver concepts causes the highest irrelevance to them. Therefore, these two concepts are the ones that they are the least affected in positive changes of the driver concepts in the FCM. The values of 18 and 11% decrease, respectively are not significant but the fact of the greater negatively causality is of great importance. The highest increases appear on the “Uncertainty toward the settlement of the new industry” and “Equity in sharing WBF product profit” scoring a 9 and 2% increase, respectively.
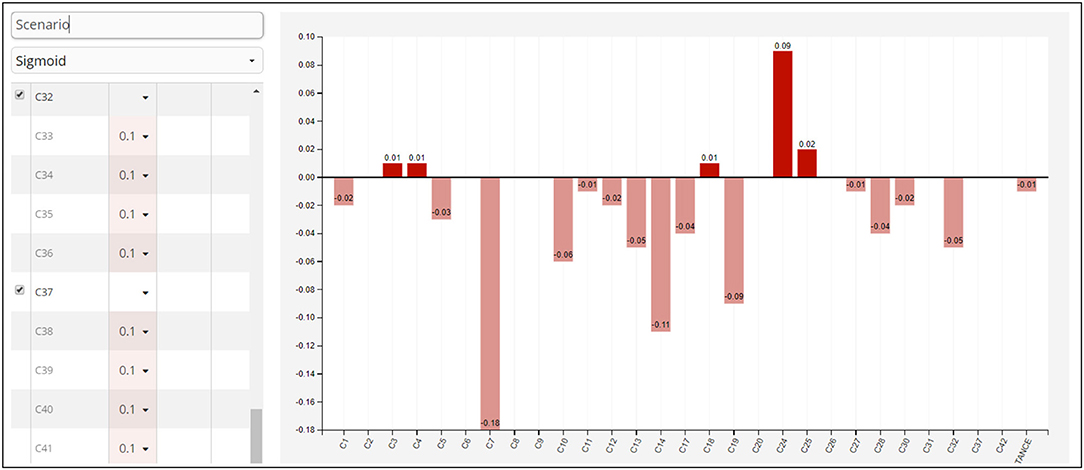
Figure 3. The effect of the driver concepts in the full FCM for the worst case scenario compared to the steady state.
On the opposite side, the best case scenario is set up with all driver concepts to have the value near the value of 1. That means that we set up our model so that driver concepts are the ones that primarily affect the FCM as opposed to the rest of the ordinary concepts. Similarly in this case and after setting up all the driver concepts in the steady analysis to the value of 1 we observe the difference on the ordinary and receiver concepts giving special priority on the final receiver concept of the “Social Acceptance” (see Figure 4). We observe a 10% increase in the “Social Acceptance” receiver concept when it is compared to the original steady state scenario which was set as the base for the analysis. Also several ordinary concepts behave in a similar way with representative the “Intervention of non-local businessman” with an increase of 10% and the “Consumer/citizen awareness” with an increase of 9%. In the inverse side concept C15 in Figure 4 which is the “Risk perception” is decreased as expected with a decrease of 12% and concept C24 (Uncertainty toward the settlement of the new industry) performs accordingly with a decrease of 7%. The significance of the highest decrease of concept C15 in this Figure is similar as in Figure 3 for the negative causality between the driver concepts and the concepts C15 and C24, respectively. The highest the increase of the driver concept makes the concepts C15 and C24 to be insignificant for the social acceptance receiver concept. The values of the decrease are as much important as the fact of signifying the least relevant ordinary concepts C15 and C24 in the model. For this case also, it was expected that the biggest increase of the driver concepts will decrease the risk (for example the bigger the Mass media publicity the smaller the risk reception and similarly this holds for the rest of the driver concepts).
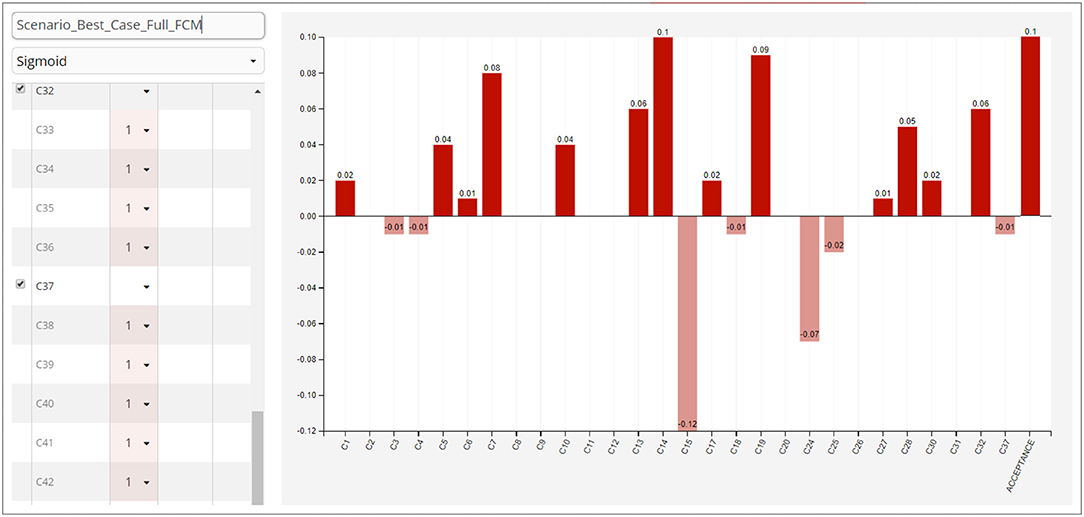
Figure 4. The effect of the driver concepts in the full FCM for the best case scenario compared to the steady state.
Dynamic Scenario Analysis of the Social Issues
The size and complexity of the FCM conducted in sections Description of the Case Study and FCM Steady State Analysis however make the dynamic simulations needed for scenario analysis of the model less efficient. Such simulations on the extreme case (all concepts used as variables with semi-continuity on the values assigned between 0 and 1) run for long time periods to converge. Furthermore, the combinations of fixed value and variable value concepts are of exponential nature in this case, thus making us unable to explore the dynamic interactions between all concepts in the merged FCM. For this reason, we developed a second FCM based only on the concepts related to the social issues, trying to discover how only these aspects affect the social acceptance of WBFs. The graph achieved is shown in Figure 5, and from now on, it will be denoted as Social_FCM.
For this case, the total number of concepts including “Social Acceptance” rises up to 18, with the total number of associations between concepts to be 60. For this case again, the only receiver concept is the “Social Acceptance,” with 7 Driver components and 10 Ordinary. The hierarchy index for this case was calculated to 0.0023, making the FCM slightly “more democratic” than the previous one. The general statistics of the Social_FCM are shown in Table 3 and the degrees and centralities of all the concepts in Table 4.
For the resulted FCM, we run a significant amount of simulations to explore the nature of the associations between the related concepts in the map and the importance of one concept (defuzzified concept value). We focused on the convergence of the FCM after a certain number of iterations, using the clamping process as stated by Kosko (1986). According to clamping, a subset of the concepts is selected to be studied, i.e., to change values as opposed to the rest, in order to understand their effect in reaching the equilibrium end states. We may use all the ordinary and driver concepts for this process, although the receiver concepts cannot be included. However, it is intuitively logical to clamp only the driver concepts, as they primarily participate on the influence of the social acceptance receiver concept. According to this process, each of the concepts is increased or decreased accordingly, and the resulting concept value vector is compared to the steady state vector of the FCM (Vasslides and Jensen, 2016). For our case, the changes are compared to the initial Social_FCM steady state. Figure 6 shows an indicative simulation of the Social_FCM, with all 18 concepts ranging from 0 to 1 along with their final converged values, and the corresponding curves of concept activation levels per each iteration. Simulations are run for all cases of activation functions available in combination to the available transformation functions, as described with the equations in section Basic Concepts for FCMs. Furthermore, any other combination and mixture of variable and ordinary concepts can easily produce individualized effects of specific concepts to Social Acceptance.
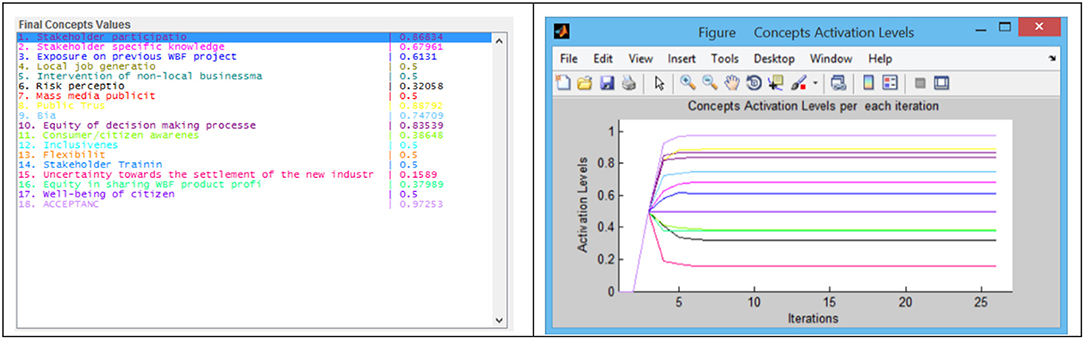
Figure 6. Indicative dynamic simulation of the Social_FCM with the converged values of the social concepts and the corresponding curves of concept activation levels per each iteration.
Similarly to the previous subsection, we consider the steady state of the Social_FCM model as the initial scenario to start our analysis. Again, in order to draw conclusions for the effect of the driver concepts to the receiver and ordinary concepts of the Social_FCM, we examined the worst case and the best case scenario as compared to the steady state. The worst case scenario is set up with all driver concepts to have the value of 0.1 as in the full FCM use case before. On the opposite side, the best case scenario is set up with all driver concepts to have the value of 1. In Figure 7, we depict the analysis where in the worst case scenario a decrease of about 3% is shown in the “Social Acceptance” receiver concept when it is compared to the original steady state scenario that was set as the base for the analysis. In the same figure, we also observe the biggest decrease in the “Public Trust” concept rising up to 8%, with the “Equity of decision making processes” to follow having an overall 16% decrease. The highest increases appear on the “Risk perception,” “Uncertainty toward the settlement of the new industry” and “Equity in sharing WBF product profit,” recording a 16, 8, and 8% increase, respectively.
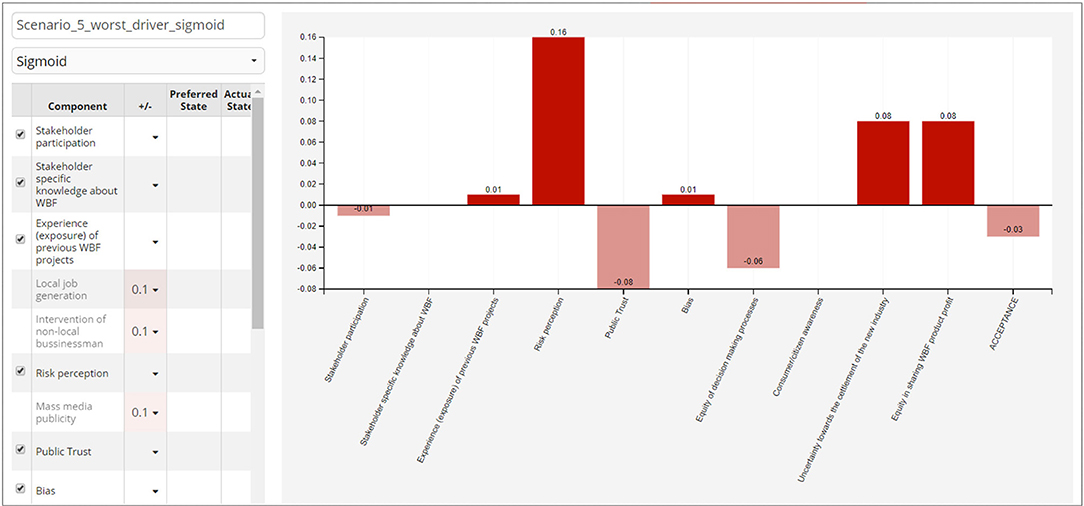
Figure 7. The effect of the driver concepts in the Social_FCM for the worst case scenario compared to the steady state.
It must be noted that Figure 7 does not depict absolute concept values, but only the increase or the decrease of these values relatively to the corresponding values of the steady state. For the case of the worst scenario explained previously, the concept values after convergence, as well as the curves of activation levels as they relate to the number of iterations, are shown in Figure 8.
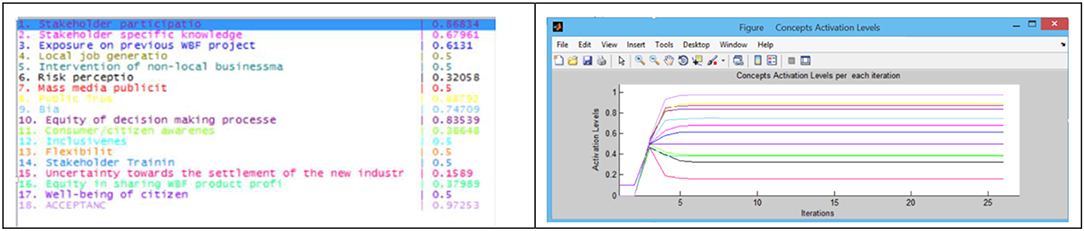
Figure 8. Converged concept values and activation curves for the worst case scenario of the Social_FCM.
On the opposite side, the best case scenario was run using the same activation function to be comparable with the previous scenario. For the best case, all seven driver concepts are set to value 1 and we study the decrease or increase of the ordinary and receiver concepts relatively to the steady state. It should be noted that, on Figure 9, we have: increase on the “Stakeholder participation,” decrease of the “Risk perception,” increase on “Public trust,” decrease on “Bias,” increase on “Equity of decision making processes,” decrease on “Uncertainty toward the settlement of the new industry,” decrease on the “Equity in sharing WBF product profit,” and finally an increase on the “Social acceptance.” It should also be noticed that these increases or decreases are relatively to the worst case scenario.
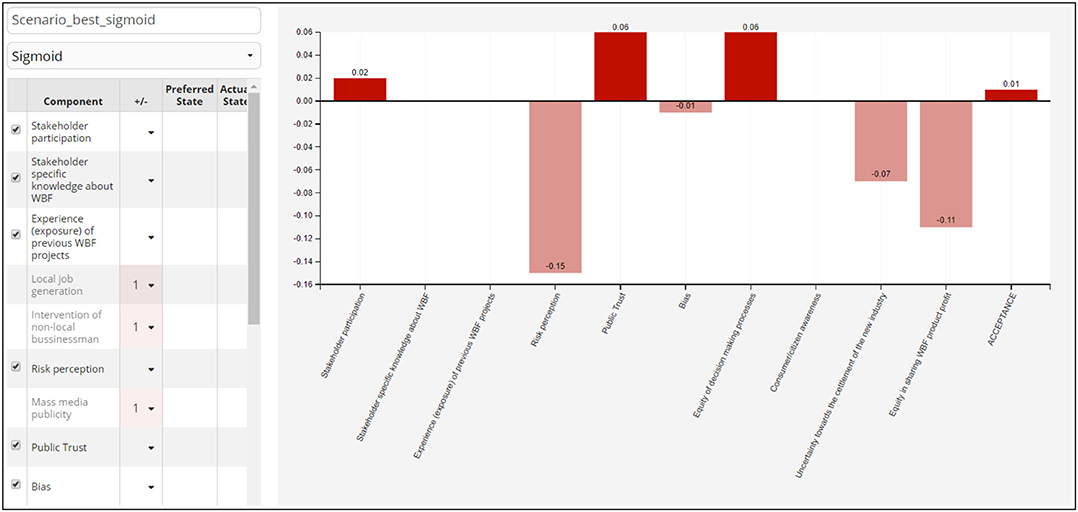
Figure 9. The effect of the driver concepts in the Social_FCM for the best case scenario compared to the steady state.
All increases and decreases are judged logical expect the concept of “Equity of decision making processes.” This and only misbehavior of the FCM is minor for two reasons: firstly the concept affected is ordinary and secondly the receiver concept is not affected. Similarly, on Figure 10 the curves of activation levels as they relate to the number of iterations are shown.
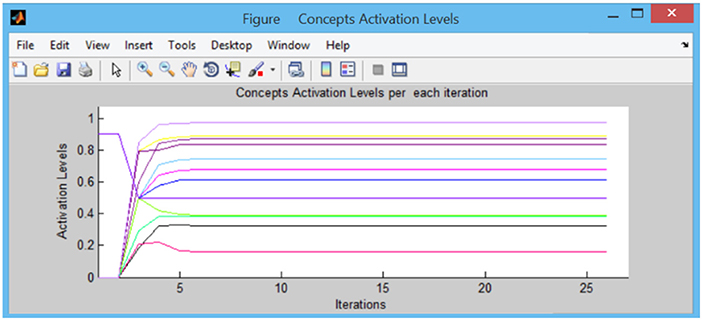
Figure 10. Concept activation curves per each iteration for the best case scenario of the Social_FCM.
Conclusions and Future Challenges
Waste biorefinery facilities (WBFs) development and sustainable waste management are still in infancy in the Southern Europe countries, due to limited allocated assets given, resulting in ischemic infrastructure and maintenance facilities. On the other hand, waste management is an enormously critical aspect because of the environmental, economical, technological and social consequences. For that reason, such large scale operations necessitate the coalition of large social bodies, stakeholders of diversified interest and competent authority policy makers.
Our methodology was two fold in relation to identifying the most important aspects that affect the social acceptance of WBF. Firstly, a Fuzzy Cognitive Map (FCM) was developed, which captures the interoperation of environmental, health, social, technological and economic concepts that influence the social acceptance of WBFs. Since the complicated structure of the resulted FCM did not allow any definite conclusion regarding the most influential concepts to the social acceptance, in the second phase we modeled the impacts of just the social aspects to “social acceptance” for WBFs. For this purpose, of smaller FCM was used, to show treads and general directions in identifying the most determinant factors affecting the public opinion. Our analysis was also twofold: a) Steady State, where the FCM resulted as an amalgamation of stakeholder and expert knowledge, identifying five major classes of issues and b) Dynamic scenario analysis, after extracting only the social components, resulting in a much smaller FCM. For the second case, we were able to highlight the effect of the driver concepts, comparing the worst case and best case scenarios with the steady state analysis results. Various simulations were tried also, with a combination of activation and transformation functions selected as well as a variety of value levels between the worst and best case scenarios for a subset of ordinary and driver components.
More specifically, the analysis revealed that concepts such as “Uncertainty toward the settlement of the new industry,” “Risk perception,” “Stakeholder participation,” “Consumer/Citizen awareness,” “Equity of decision making processes” are the most influential factors to “Social Acceptance.” This was what the dynamic scenario analysis has verified for the Social_FCM part of the FCM that was originally developed. However, there are some shortcomings to this study, which should be taken into account. First, the representation of the model and its dynamic behavior was focused only on the social aspects. This happened because the preliminary analysis of the full FCM resulted in a complicated structure increasing tremendously the state space of the FCM. For that reason, around 25 issues/concepts were cut from the original FCM overcoming the inability to provide dynamic scenario analysis with so many concepts. This concept reduction process also diminishes several indirect interrelations of the social concepts with the concepts of the other classes (environmental, economic, technological etc.). Thus, these interrelations and their impacts are not taken into consideration and their result in increase or decrease of the “Social Acceptance” cannot be revealed in the second version of the FCM. Secondly, the impact of the number of experts/stakeholders deployed to the developing task of the FCMs was not examined. There are studies that highlight the fact that, the greater the expert body the more stable the version of the FCM produced. Also, the improvement of the conceptual model describing the WBFs social acceptance is shown to be dependent of learning methodologies used.
Based on our discussion above and the input received from stakeholders, there are several recommended areas of focus as industry moves toward the optimization of WBF application. However, the most important issue is to design a competitive waste-biorefinery concept by systematic participation of all the factors that affect the decision and policy making in WBF application. The technology knowledge must be transmitted to various stakeholders in the wider society and the final decision must integrate not only the technological and economic advantages but also health and social acceptance. For that reason, social acceptance must always be present alongside life-cycle assessment and economic value and environmental impact analysis tools for the WBF application. The bottom line is that it is critical to engage local communities in the development and management of WBFs in order to elicit the view of lay people, as a means to amend burdens relating to social factors and to avoid the “NIMBY” phenomenon. We believe therefore that the enlargement of the present study with further investigation in this research area can provide a valuable tool for competent authorities and policy makers in developing WBFs.
Author Contributions
All authors listed have made a substantial, direct and intellectual contribution to the work, and approved it for publication.
Conflict of Interest Statement
The authors declare that the research was conducted in the absence of any commercial or financial relationships that could be construed as a potential conflict of interest.
Footnotes
1. ^www.mentamodeler.org (Accessed July 7, 2018).
References
Alizadeh, S., Ghazanfari, M., Jafari, M., and Hooshmand, S. (2007). Learning FCM by tabu search. Int. J. Comput. Sci. 2, 142–149.
Axelrod, R. (1976). “The analysis of cognitive maps,” in Structure of Decision: The Cognitive Maps of Political Elites, ed R. Axelrod (Princeton, NY: Princeton University Press), 55–73
Beigl, P., Lebersorger, S., and Salhofer, S. (2008). Modelling municipal solid waste generation: a review. J. Waste Manag. 28, 200–214. doi: 10.1016/j.wasman.2006.12.011
Bovea, M. D., and Powell, J. C. (2006). Alternative scenarios to meet the demands of sustainable waste management. J. Environ. Manage. 79, 115–132. doi: 10.1016/j.jenvman.2005.06.005
Brion, D. J. (2015). An essay on LULU, NIMBY, and the problem of distributive justice. Boston College Environ. Affairs Law Rev. 15, 1–68.
CTV Kitchener (2012). Group of Elmira Residents Protesting Biofuel Plant. Published Sunday, April 15, 2012 5:35PM EDT. Available online at: http://kitchener.ctvnews.ca/group-of-elmira-residents-protestingbiofuel-plant-1.796466 [Last accessed June 21st 2018].
Cucek, L., Klemes, J. J., Varbanov, P. S., and Kravanja, Z. (2013). Dealing with high-dimensionality of criteria in multi-objective optimization of biomass energy supply network. Ind. Eng. Chem. Res. 52, 7223–7239. doi: 10.1021/ie302599c
Dear, M. (1992). Understanding and overcoming the NIMBY syndrome. J. Am. Plann. Assoc. 58, 288–300. doi: 10.1080/01944369208975808
den Boer, E., and Lager, J. (2007). LCA-IWM: a decision support tool for sustainability assessment of waste management systems. J. Waste Manage. 27, 1032–1045. doi: 10.1016/j.wasman.2007.02.022
Devine-Wright, P. (2009). Rethinking NIMBYism: the role of place attachment and place identity in explaining place-protective action. J. Commun. Appl. Soc. Psychol. 19, 426–441. doi: 10.1002/casp.1004
Dickerson, J. A., and Kosko, B. (1994). Virtual worlds as fuzzy cognitive maps. Presence 3, 173–189. doi: 10.1162/pres.1994.3.2.173
Dowling, A. W., Ruiz-Mercado, G., and Zavala, V. M. (2016). A framework for multi-stakeholder decision-making and conflict resolution. Comp. Chem. Eng. 90, 136–150. doi: 10.1016/j.compchemeng.2016.03.034
Fortenbery, T., Randall, D., Steven, C., and Amiel, L. (2013). The location decisions of biodiesel refineries. Land Econ. 89, 118–136. doi: 10.3368/le.89.1.118
Geraili, A., and Romagnoli, J. A. (2015). A multi-objective optimization framework for design of integrated biorefineries under uncertainty. AIChE J. 61 3208–3222. doi: 10.1002/aic.14849
Gray, S. A., Zanre, E., and Gray, S. R. J. (2014). “Fuzzy cognitive maps as representations of mental models and group beliefs: theoretical and technical issues,” in Fuzzy Cognitive Maps for Applied Sciences and Engineering—From Fundamentals to Extensions and Learning Algorithms. Papageorgiou, E. I. ed (Heidelberg: Springer), 29–48.
Graymore, M. L. M., Sipe, N. G., and Rickson, R. E. (2008). Regional sustainability: how useful are current tools of sustainability assessment at the regional scale? J. Ecol. Econom. 67, 362–372. doi: 10.1016/j.ecolecon.2008.06.002
Gregory, R., Failing, L., Harstone, M., Long, G., McDaniels, T., and Ohlson, D. (2012). Structured Decision Making: A Practical Guide to Environmental Management Choices. (New York, NY: Wiley).
Groumpos, P., and Anninou, A. (2017). A critical overview of modeling methods and decision support systems for complex dynamic systems. Ann. Faculty Eng. Hunedoara 15, 17–26.
Haddad, M. A., Gary, T., and Francis, O. (2009). Locational choices of the ethanol industry in the Midwest Corn Belt. Econ. Dev. Q. 24, 74–86. doi: 10.1177/0891242409347722
Halder, P., Prokop, P., Chang, C.-Y., Usak, M., Pietarinen, J., Havu-Nuutinen, S., et al. (2012). International survey on bioenergy knowledge, perceptions, and attitudes among young citizens. Bioenergy Res. 5, 247–261. doi: 10.1007/s12155-011-9121-y
Hosseini, A., Zolfagharzadeh, M. M., Asghar Sadabadi, A., Aslani, A., and Jafari, H. (2018). Social acceptance of renewable energy in developing countries: challenges and opportunities. Distribut. Gener. Alternat. Energy J. 33, 31–48. doi: 10.1080/21563306.2018.11969264
Huerga, A. V. (2002). A Balanced Differential Learning Algorithm in Fuzzy Cognitive Maps. 16th International Workshop Qualitat. Reason., Catalonia, Spain.
Kok, K., Bärlund, I., Flörke, M., Holman, I., Gramberger, M., Sendzimir, J., et al. (2015). European participatory scenario development: strengthening the link between stories and models. Climate Change 128, 187–200. doi: 10.1007/s10584-014-1143-y
Konar, A., and Chakraborty, U. K. (2005). Reasoning and unsupervised learning in a fuzzy cognitive map. Inf. Sci. 170, 419–441. doi: 10.1016/j.ins.2004.03.012
Kosko, B. (1986). Fuzzy cognitive maps. Int. J. Man Mach. Stud. 24, 65–75. doi: 10.1016/S0020-7373(86)80040-2
Kosko, B. (1987). “Adaptive inference in fuzzy knowledge networks,” in Proceedings of the First IEEE International Conference on Neural Networks, vol. II, San Diego, California, 261–268.
Koulouriotis, D. E., Diakoulakis, I. E., and Emiris, D. M. (2001). “Learning fuzzy cognitive maps using evolution strategies: a novel schema for modeling and simulating high-level behavior,” in Proceedings IEEE Congress. Evoluation Computer, 364–371.
Lakioti, E. N., Moustakas, K. G., Komilis, D. P., Domopoulou, A. E., and Karayannis, V. G. (2017). Sustainable solid waste management: Socio-economic considerations. Chem. Eng. Trans. 56, 661–666. doi: 10.3303/CET1756111
Lambert, E. (2009). Nimby wars. Published Jan. 29th 2009. Available online at: http://www.forbes.com/forbes/2009/0216/098.html [Last accessed June 21st 2018].
Liew, W. H., Hassim, M. H., Ng, D. K. S., and Chemmangattuvalappil, N. (2015). Systematic framework for sustainability assessment of biodiesel production: preliminary engineering stage. Ind. Eng. Chem. Res. 54, 12615–12629. doi: 10.1021/acs.iecr.5b02894
Lin, Y., and Yang, W. (2018). Application of multi-objective genetic algorithm based simulation for cost-effective building energy efficiency design and thermal comfort improvement. Front. Energy Res. 6:25. doi: 10.3389/fenrg.2018.00025
Lopolito, A., Prosperi, M., and Sisto, R. (2009). Socio-Economic Implications of the Development of a Bio-Refinery: An Analysis with Fuzzy Cognitive Maps. Available online at: http://unifg.academia.edu/AntonioLopolito/Papers/1053338/Socio-Economic_Implications_Of_The_Development_Of_A_BioRefinery_An_Analysis_With_Fuzzy_Cognitive_Maps.
Mateou, N. H., Moiseos, M., and Andreou, A. S. (2005). “Multi-objective evolutionary fuzzy cognitive maps for decision support,” in Proceedings IEEE Congress Evoluation Computer vol. 1, Edinburgh 824–830. Mental Modeler Manual, 2018. Available online at: www.mentamodeler.org [Last accessed 23rd of June, 2018].
McGuire, J. B., Leahy, J. E., Marciano, J. A., Lilieholm, R. J., and Teisl, M. F. (2017). Social acceptability of establishing forest-based biorefineries in Maine, United States. Biomass Bioenergy 105, 155–163. doi: 10.1016/j.biombioe.2017.06.015
Mielke, J., Vermaßen, H., Ellenbeck, S., Fernandez Milan, B., and Jaeger, C. (2016). Stakeholder involvement in sustainability science—A critical view. Energy Res. Soc. Sci. 17, 71–81. doi: 10.1016/j.erss.2016.04.001
Munda, G. (2004). Social multi-criteria evaluation: methodological foundations and operational consequences. Eur. J. Oper. Res. 158, 662–677. doi: 10.1016/S0377-2217(03)00369-2
Nizami, A. S., Rehan, M., Waqas, M., Naqvi, M., Ouda, O. K. M., Shahzad, K., et al. (2017a). Waste biorefineries: enabling circular economies in developing countries. Bioresour. Technol. 241, 1101–1117. doi: 10.1016/j.biortech.2017.05.097
Nizami, A. S., Shahzad, K., Rehan, M., Ouda, O. K. M., Khan, M. Z., Ismail, I. M. I., et al. (2017b). Developing waste biorefinery in Makkah: a way forward to convert urban waste into renewable energy. Appl. Energy 186, 189–196. doi: 10.1016/j.apenergy.2016.04.116
Novak, J. D., and Cañas, A. J. (2008). The theory underlying concept maps and how to construct and use them. Technical Report IHMC CmapTools 2006-01 Rev 01-2008. Florida Institute for Human and Machine Cognition, Pensacola, Florida. Available online at: http://cmap.ihmc.us/publications/researchpapers/theoryunderlyingconceptmaps.pdf
Ozesmi, U., and Ozesmi, S. L. (2004). Ecological models based on people's knowledge: a multistep fuzzy cognitive mapping approach. Ecol. Modell. 176, 43–64. doi: 10.1016/j.ecolmodel.2003.10.027
Papageorgiou, E., Stylios, C. D., and Groumpos, P. P. (2003). “Fuzzy cognitive map learning based on nonlinear Hebbian rule,” in Proceedings Australasian Joint Conference on Artificial Intelligence (Perth, WA), 256–268.
Papageorgiou, E., Stylios, C. D., and Groumpos, P. P. (2004). Active Hebbian learning algorithm to train fuzzy cognitive maps. Int. J. Approx. Reason. 37, 219–249. doi: 10.1016/j.ijar.2004.01.001
Papageorgiou, E. I., and Groumpos, P. P. (2005). A weight adaptation method for fine-tuning fuzzy cognitive map causal links. Soft Comput. J. 9, 846–857. doi: 10.1007/s00500-004-0426-z
Petalas, Y. G., Papageorgiou, E. I., Parsopoulos, E. I., Groumpos, P. P., and Vrahatis, M. N. (2005). “Fuzzy cognitive maps learning using memetic algorithms,” in Proceedings Conference Computational Methods in Sciences and Engineering, Loutraki 1420–1423.
Raskin, J. D. (2002). “Constructivism in psychology: personal construct psychology, radical constructivism, and social constructionism,” in Studies in Meaning: Exploring Constructivist Psychology ed Raskin, J. D., and Bridges, S. K. (New York, NY: Pace University Press), 1–25.
Rehan, M., Gardy, J., Demirbas, A., Rashid, U., Budzianowski, W. M., Pant, D., et al. (2018). Waste to biodiesel: a preliminary assessment for Saudi Arabia. Bioresour. Technol. 250, 17–25. doi: 10.1016/j.biortech.2017.11.024
Sacchelli, S. (2014). Social acceptance optimization of biomass plants: a fuzzy cognitive map and evolutionary algorithm application. Chem. Eng. Trans. 37, 181–186. doi: 10.3303/CET1437031
Scolobig, A., and Lilliestam, J. (2016). Comparing approaches for the integration of stakeholder perspectives in environmental decision making. Resources 5:37. doi: 10.3390/resources5040037
Selfa, T. (2010). Global benefits, local burdens? The paradox of governing biofuels production in Kansas and Iowa. Renewable Agric. Food Syst. 25, 129–142. doi: 10.1017/S1742170510000153
Stach, W., Kurgan, L., and Pedrycz, W. (2007). “Parallel learning of large fuzzy cognitive maps,” in Proceedings International Joint Conference Neural Network (Orlando, FL), 1584–1589.
Stach, W., Kurgan, L., and Pedrycz, W. (2008). “Data driven nonlinear Hebbian learning method for fuzzy cognitive maps,” in Proceedings IEEE World Congress on Computational Intelligence, Hong Kong, 1975–1981.
Stach, W., Kurgan, L. A., Pedrycz, W., and Reformat, M. (2005). Genetic learning of fuzzy cognitive maps. Fuzzy Sets Syst. 153, 371–401. doi: 10.1016/j.fss.2005.01.009
Stadler, P., Girardin, L., Ashouri, A., and Maréchal, F. (2018). Contribution of model predictive control in the integration of renewable energy sources within the built environment. Front. Energy Res. 6:22. doi: 10.3389/fenrg.2018.00022
Stirling, A. (2006). Analysis, participation and power: justification and closure in participatory multi-criteria analysis. Land Use Policy 23, 95–107. doi: 10.1016/j.landusepol.2004.08.010
Tigges, L. M., and Noble, M. (2012). Getting to yes or bailing on no: the site selection process of ethanol plants in Wisconsin. Rural Sociol. 77, 547–568. doi: 10.1111/j.1549-0831.2012.00092.x
Trutnevyte, E., Guivarch, C., Lempert, R., and Strachan, N. (2016). Reinvigorating the scenario technique to expand uncertainty consideration. Climate Change 135, 373–379. doi: 10.1007/s10584-015-1585-x
Van Asselt, M., and Rijkens-Klomp, N. (2002). A look in the mirror: Reflection on participation in integrated assessment from a methodological perspective. Glob. Environ. Chang. 12, 167–184. doi: 10.1016/S0959-3780(02)00012-2
van der Horst, D. (2007). NIMBY or not? Exploring the relevance of locations and the politics of voiced opinions in renewable energy citing controversies. Energy Policy 35 2705–2714. doi: 10.1016/j.enpol.2006.12.012
Vasslides, J. M., and Jensen, O. P. (2016). Fuzzy Cognitive Mapping in support of integrated ecosystem assessments: developing a shared conceptual model among stakeholders. J. Environ. Manage. 166, 348–356. doi: 10.1016/j.jenvman.2015.10.038
Walter, G., and Gutscher, H. (2011). Public Acceptance of Wind Energy and Bioenergy Projects in the Framework of Distributive and Procedural Justice Theories: Insights from Germany, Austria and Switzerland. Zurich: The Advisory House.
Wolsink, M. (2012). “Wind power: basic challenge concerning social acceptance,” in Encyclopedia of Sustainability Science and Technology, ed Meyers, R.A. New York, NY: Springer, 12218–12254.
Yatsalo, B. I., Kiker, G. A., Kim, J., Bridges, T. S., Seager, T. P., Gardner, K., et al. (2007). Application of multi-criteria decision analysis tools to two contaminated sediment case studies. Integr. Environ. Assess. Manag. 3, 223–233. doi: 10.1897/IEAM_2006-036.1
Yesil, E., and Dodurka, M. F. (2013). “Goal-oriented decision support using big bang-big crunch learning based fuzzy cognitive map: An ERP management case study,” in Proceedings 2013 IEEE International Conference on Fuzzy Systems (FUZZ-IEEE), 1–8.
Keywords: waste biorefinery facility (WBF), social acceptance, uncertainty, fuzzy cognitive map (FCM), modeling
Citation: Kokkinos K, Lakioti E, Papageorgiou E, Moustakas K and Karayannis V (2018) Fuzzy Cognitive Map-Based Modeling of Social Acceptance to Overcome Uncertainties in Establishing Waste Biorefinery Facilities. Front. Energy Res. 6:112. doi: 10.3389/fenrg.2018.00112
Received: 07 August 2018; Accepted: 04 October 2018;
Published: 26 October 2018.
Edited by:
Abdul-Sattar Nizami, Centre of Excellence in Environmental Studies, King Abdulaziz University, Saudi ArabiaReviewed by:
Giuseppe Mancini, Università degli Studi di Catania, ItalyAlok Satlewal, Indian Oil Corporation, India
Ta Yeong Wu, Monash University Malaysia, Malaysia
Copyright © 2018 Kokkinos, Lakioti, Papageorgiou, Moustakas and Karayannis. This is an open-access article distributed under the terms of the Creative Commons Attribution License (CC BY). The use, distribution or reproduction in other forums is permitted, provided the original author(s) and the copyright owner(s) are credited and that the original publication in this journal is cited, in accordance with accepted academic practice. No use, distribution or reproduction is permitted which does not comply with these terms.
*Correspondence: Konstantinos Moustakas, a29ubW91c3RAY2VudHJhbC5udHVhLmdy