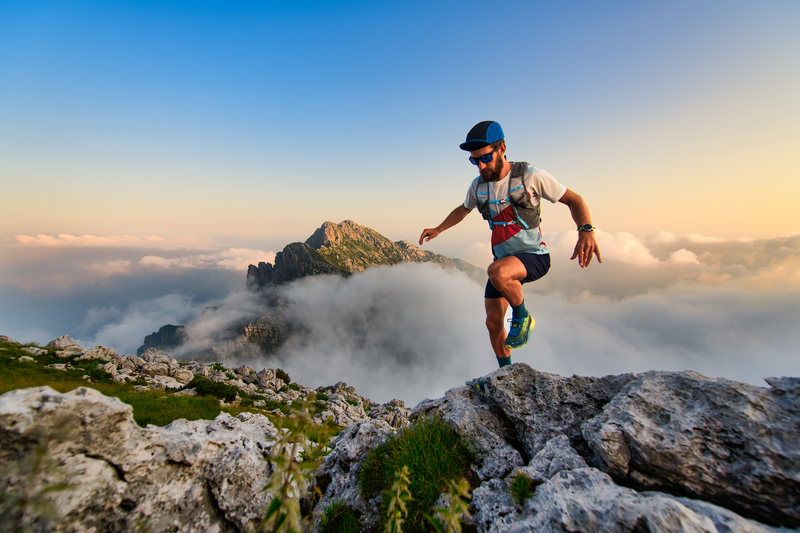
94% of researchers rate our articles as excellent or good
Learn more about the work of our research integrity team to safeguard the quality of each article we publish.
Find out more
PERSPECTIVE article
Front. Endocrinol.
Sec. Clinical Diabetes
Volume 16 - 2025 | doi: 10.3389/fendo.2025.1583227
This article is part of the Research Topic Future Horizons in Diabetes: Integrating Gut Microbiota, AI, and Personalized Care View all articles
The final, formatted version of the article will be published soon.
You have multiple emails registered with Frontiers:
Please enter your email address:
If you already have an account, please login
You don't have a Frontiers account ? You can register here
Diabetes is a global health crisis with rising incidence, mortality, and economic burden.Traditional markers like HbA1c are insufficient for capturing short-term glycemic fluctuations, leading to the need for more precise metrics such as Glucose Variability (GV) and Time in Range (TIR). Continuous Glucose Monitoring (CGM) and AI integration offer real-time data analytics and personalized treatment plans, enhancing glycemic control and reducing complications. The combination of transcutaneous auricular vagus nerve stimulation (taVNS) with artificial Intelligence (AI) further optimizes glucose regulation and addresses comorbidities. Empowering patients through AI-driven self-management and community support is crucial for sustainable improvements. Future horizons in diabetes care must focus on overcoming challenges in data privacy, algorithmic bias, device interoperability, and equity in AI-driven care while integrating these innovations into healthcare systems to improve patient outcomes and quality of life.
Keywords: diabetes blood glucose, Glucose variability, time in range, Continuous glucose monitoring, taVNS, artificial intelligence
Received: 25 Feb 2025; Accepted: 11 Mar 2025.
Copyright: © 2025 Zhang, Qi, Wang, Tian, Wang, Xu and Zhai. This is an open-access article distributed under the terms of the Creative Commons Attribution License (CC BY). The use, distribution or reproduction in other forums is permitted, provided the original author(s) or licensor are credited and that the original publication in this journal is cited, in accordance with accepted academic practice. No use, distribution or reproduction is permitted which does not comply with these terms.
* Correspondence:
Kaiqi Zhang, Wangjing Hospital, China Academy of Chinese Medical Sciences, Beijing, Beijing Municipality, China
Disclaimer: All claims expressed in this article are solely those of the authors and do not necessarily represent those of their affiliated organizations, or those of the publisher, the editors and the reviewers. Any product that may be evaluated in this article or claim that may be made by its manufacturer is not guaranteed or endorsed by the publisher.
Research integrity at Frontiers
Learn more about the work of our research integrity team to safeguard the quality of each article we publish.