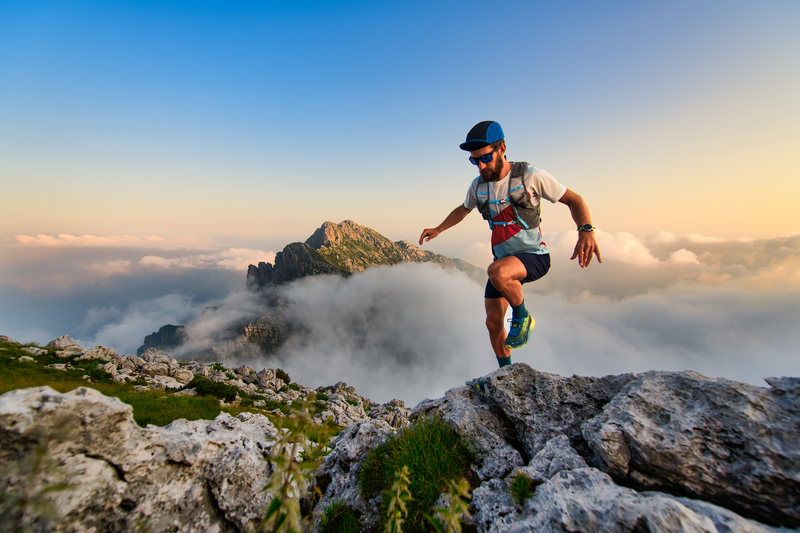
95% of researchers rate our articles as excellent or good
Learn more about the work of our research integrity team to safeguard the quality of each article we publish.
Find out more
ORIGINAL RESEARCH article
Front. Endocrinol.
Sec. Cardiovascular Endocrinology
Volume 16 - 2025 | doi: 10.3389/fendo.2025.1545462
This article is part of the Research Topic The Role of Metabolic Syndrome and Disorders in Cardiovascular Disease - Volume II View all 22 articles
The final, formatted version of the article will be published soon.
You have multiple emails registered with Frontiers:
Please enter your email address:
If you already have an account, please login
You don't have a Frontiers account ? You can register here
AbstractBackground: Upper gastrointestinal bleeding (UGIB) is a common complication in patients with non-ST-segment elevation myocardial infarction (NSTEMI) after percutaneous coronary intervention (PCI), and the aim of our study is to construct a nomogram for predicting the occurrence of UGIB within 1 years after PCI in NSTEMI patients.Methods: In this study, 784 patients with NSTEMI after PCI in the Affiliated Hospital of Xuzhou Medical University between September 1, 2017 and August 31, 2019 were included as the training group, and 336 patients from the East Affiliated Hospital of Xuzhou Medical University were included as the external validation group. Classical regression methods were combined with a machine learning model to identify the independent risk factors. These factors based on multivariate logistic regression analysis were then utilized to develop a nomogram. The performance of the nomogram was evaluated using the area under the receiver operating characteristic curve (AUC), calibration plots, and decision curve analysis (DCA).Results: The nomogram consisted of six independent predictors, including HASBLED, triglyceride glucose index, alcohol drinking, red blood cell count, use of proton pump inhibitor, and angiographic microvascular resistance of culprit vessel. Training and validation groups accurately predicted the occurrence of UGIB (AUC, 0.936 and 0.910). The calibration curves showed that the nomogram agreed with the actual observations and the DCA also demonstrated that the nomogram was applicable in the clinic.Conclusion: We developed a simple and effective nomogram for predicting the occurrence of UGIB within 1 years in NSTEMI patients after PCI based on angiographic microvascular resistance.
Keywords: Angiographic microvascular resistance of culprit vessel, upper gastrointestinal bleeding, Non-ST-segment elevation myocardial infarction, nomogram, Percutaneous Coronary Intervention
Received: 15 Dec 2024; Accepted: 28 Mar 2025.
Copyright: © 2025 Wang, Yang, Zhou, Li, Chen, Chen, LI, Li and Xu. This is an open-access article distributed under the terms of the Creative Commons Attribution License (CC BY). The use, distribution or reproduction in other forums is permitted, provided the original author(s) or licensor are credited and that the original publication in this journal is cited, in accordance with accepted academic practice. No use, distribution or reproduction is permitted which does not comply with these terms.
* Correspondence:
Tongda Xu, Department of Cardiology, The Affiliated Hospital of Xuzhou Medical University, Xuzhou, China
Disclaimer: All claims expressed in this article are solely those of the authors and do not necessarily represent those of their affiliated organizations, or those of the publisher, the editors and the reviewers. Any product that may be evaluated in this article or claim that may be made by its manufacturer is not guaranteed or endorsed by the publisher.
Research integrity at Frontiers
Learn more about the work of our research integrity team to safeguard the quality of each article we publish.