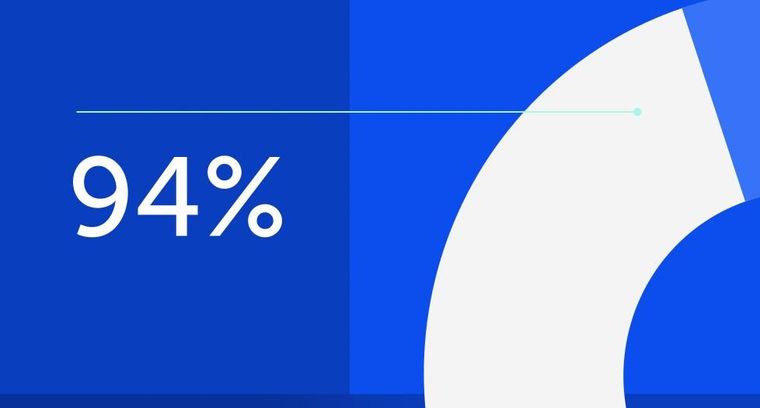
94% of researchers rate our articles as excellent or good
Learn more about the work of our research integrity team to safeguard the quality of each article we publish.
Find out more
ORIGINAL RESEARCH article
Front. Endocrinol.
Sec. Systems Endocrinology
Volume 16 - 2025 | doi: 10.3389/fendo.2025.1543185
This article is part of the Research TopicMechanistic, Machine Learning and Hybrid Models of the 'Other' Endocrine Regulatory Systems in Health and Disease: Volume IIView all 4 articles
The final, formatted version of the article will be published soon.
Select one of your emails
You have multiple emails registered with Frontiers:
Notify me on publication
Please enter your email address:
If you already have an account, please login
You don't have a Frontiers account ? You can register here
The intricate interplay between endocrine systems and EEG signals is pivotal for understanding and managing physiological and neurological health. Traditional mathematical models often fail to capture the nonlinear dynamics, feedback mechanisms, and cross-system interactions inherent in these processes, limiting their applicability in clinical and research settings. This study proposes a novel framework for modeling and analyzing the interaction between endocrine regulatory systems and EEG signals, leveraging advanced methodologies such as the Hormone Interaction Dynamics Network (HIDN) and the Adaptive Hormonal Regulation Strategy (AHRS). HIDN integrates graph-based neural architectures with recurrent dynamics to encapsulate the spatialtemporal interdependencies among endocrine glands, hormones, and EEG signal fluctuations. AHRS complements this by dynamically optimizing therapeutic interventions using real-time feedback and patient-specific parameters, ensuring adaptability to individual variability and external perturbations. The proposed model excels in scalability, precision, and robustness, addressing challenges like sparse clinical data, temporal resolution, and multi-hormonal regulation.Experimental validation demonstrates its efficacy in predicting hormone dynamics, EEG signal patterns, and therapeutic outcomes under varying conditions. This interdisciplinary approach bridges the gap between computational modeling and practical healthcare applications, advancing our understanding of endocrine-neurological interactions.
Keywords: Endocrine systems, EEG signals, Nonlinear Dynamics, Adaptive regulation, Hormonal Modeling
Received: 11 Dec 2024; Accepted: 21 Apr 2025.
Copyright: © 2025 Wei. This is an open-access article distributed under the terms of the Creative Commons Attribution License (CC BY). The use, distribution or reproduction in other forums is permitted, provided the original author(s) or licensor are credited and that the original publication in this journal is cited, in accordance with accepted academic practice. No use, distribution or reproduction is permitted which does not comply with these terms.
* Correspondence: Liu Wei, Sichuan Provincial Maternity and Child Health Care Hospital, Chengdu, China
Disclaimer: All claims expressed in this article are solely those of the authors and do not necessarily represent those of their affiliated organizations, or those of the publisher, the editors and the reviewers. Any product that may be evaluated in this article or claim that may be made by its manufacturer is not guaranteed or endorsed by the publisher.
Supplementary Material
Research integrity at Frontiers
Learn more about the work of our research integrity team to safeguard the quality of each article we publish.