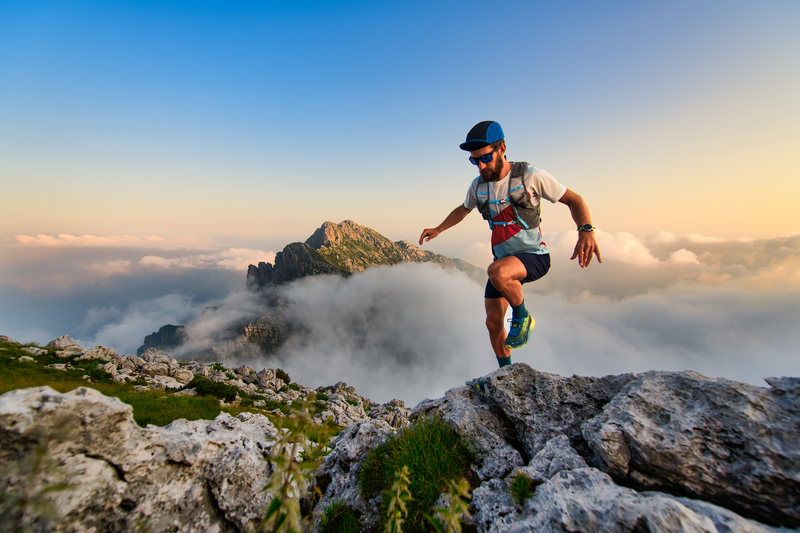
95% of researchers rate our articles as excellent or good
Learn more about the work of our research integrity team to safeguard the quality of each article we publish.
Find out more
ORIGINAL RESEARCH article
Front. Endocrinol. , 14 March 2025
Sec. Cardiovascular Endocrinology
Volume 16 - 2025 | https://doi.org/10.3389/fendo.2025.1540241
Background: Cardiovascular disease (CVD) is strongly correlated with plasma atherogenic index (AIP); however, there is limited literature exploring the association between trajectories of change in AIP and the risk of CVD. This study aimed to investigate whether changes in AIP are associated with CVD in individuals with cardiovascular-kidney-metabolic (CKM) syndrome stage 0-3.
Methods: Data were sourced from the China Health and Retirement Longitudinal Study (CHARLS), aimed to compile high-quality microdata on individuals and households aged 45 and older in China. Change in AIP from 2012 to 2015 were classified employing K-means clustering analysis. Logistic regressions were employed to assess the association between different AIP change clusters and cumulative AIP and CVD incidence. Additionally, restricted cubic spline (RCS) regression was conducted to further evaluate the underlying linear relationship between cumulative AIP and CVD. Subgroup analyses were applied to verify the influence of confounding variables on the relationship between AIP and CVD. Weighted quantile sum (WGS) regressions were utilized to offer a comprehensive assessment of the overall effect.
Results: Out of 4,525 participants, 578 (12.77%) ultimately developed CVD within three years. Compared to cluster 1, which served as the best control for AIP, the odds ratio (OR) was 1.29 (1.02-1.62) for cluster 2, 1.33 (1.04-1.71) for cluster 3 and 1.35 (0.98-1.85) for cluster 4 after adjusting for several confounding variables. Categorizing the cumulative AIP into quartiles revealed an ascending trend (P for trend = 0.014). RCS regression disclosed a linear relationship between cumulative AIP and CVD. Further subgroup analyses revealed variations in these correlations modified by gender and Hukou status. WQS regression analysis highlighted the significance of triglyceride in the pathogenesis of CVD.
Conclusions: Significant changes in AIP are independently associated with the elevated risk of CVD in adults aged > 45 with CKM syndrome stage 0-3. Monitoring long-term fluctuations in AIP may aid in the early identification of individuals at high risk for CVD.
Cardiovascular diseases (CVD), encompassing conditions such as heart disease and stroke, are major contributors to global morbidity and mortality (1). By 2021, the overall prevalence of CVD had reached 523 million, a significant rise from the 271 million reported in 1990 (2). The death rate of CVD has been on a steady decline over the past few decades; however, there has been an observably ascending trend in CVD mortality since the onset of the COVID-19 pandemic, which has exerted a persistent influence on the ongoing disease burden and its relevant strain on health systems (3).
Cardiovascular-kidney-metabolic (CKM) syndrome is a complex systemic condition manifested as interrelated pathophysiological mechanisms involving metabolic risk factors, chronic kidney disease (CKD) and cardiovascular dysfunction, resulting in multi-organ impairment and an elevated risk of adverse cardiovascular events (4). Epidemiological studies have demonstrated that progression through CKM syndrome stages is closely associated with an increase in both relative and absolute risks of CVD (5). Moreover, the clinical impact of CKM syndrome is incommensurately linked to CVD (6). Consequently, it is imperative that early identification and comprehensive management of early stage of CKM syndrome will contribute to the prevention of the onset and progression of CVD.
The atherogenic index of plasma (AIP) has emerged as a newly identified lipid-metabolism-associated biomarker for plasma atherosclerosis (7). Extensive studies have demonstrated that AIP is correlated with multiple hazard for CVD, including hypertension (8), obesity (9), diabetes mellitus (DM) (10, 11), and metabolic syndrome (MetS) (12). Increasing evidence supports that AIP is a reliable biomarker and a potentially valuable tool for clinicians in predicting the onset and prognosis of CVD (13–16).
A limited number of studies have probed the link between changes in the AIP and the incidence of CVD in the existing research (17–19). The inherent limitation of previous studies was that the AIP was typically appraised at a single time point, failing to capture the dynamic relationship between longitudinal fluctuations in AIP and CVD risk over time, which may not adequately reflect the impact of prolonged exposure. Longitudinal studies examining the effects of repeated AIP measurements on CVD risk remain limited. Thus, it remains uncertain whether controlling AIP further influences the presence of CVD in participants with CKM syndrome. The objective of our longitudinal study is to ascertain the association between AIP controlling level and the danger of CVD in individuals classified with CKM syndrome stage 0 to 3.
The China Health and Retirement Longitudinal Study (CHARLS) is the data source of this study, the first nationally representative longitudinal survey targeting Chinese middle-aged and elderly populations. The baseline survey (Wave 1) was carried out between 2011 and 2012, subsequent to Wave 2 in 2013, Wave 3 in 2015, and Wave 4 in 2018 and the multistage probability proportional to size sampling strategy was adopted (20).
Ethical approval for all the CHARLS waves was granted by the Institutional Review Board (IRB) at Peking University - main household survey (IRB00001052-11015) and biomarker collection (IRB00001052-11014).
The baseline survey collected a total of 17,705 participants at Wave 1, covering 150 counties/450 villages/10,000 households. Comprehensive data on demographics, health status, physical measurements, and laboratory parameters were collected. Ultimately, 4,525 participants were recruited for the final analysis after applying exclusion criteria (Supplementary Figure 1). The exclusion criteria were described below (1): lack of relevant information regarding the diagnosis of CKM syndrome (2); age under 45 years (3); absence of TG or HDL-C testing data at Wave 1 or Wave 3 (4); individuals who self-reported CVD incidents prior to 2015 (5); individuals who did not provide incomplete information on the CVD incidence during the three-year follow-up period.
The stages of CKM syndrome, ranging from 0 to 3, were categorized on the basis of the cardiovascular-kidney-metabolic health presidential advisory from the American Heart Association. The classification was as following: stage 0, no risk factors of CKM present; stage 1, excess or abnormal adiposity; stage 2, metabolic risk factors or moderate-risk to high-risk CKD; and stage 3, subclinical CVD overlapping with CKM risk factors (21). Within this framework, very high-risk CKD (stage G4 or G5) and advanced predicted 10-year risk of CVD based on the Framingham risk score were considered equivalent to those with subclinical CVD (22). The modified Modification of Diet in Renal Disease equation was applied to obtain estimated glomerular filtration ratio (eGFR) (23), which was divided into CKD stages according to Kidney Disease Improving Global Outcomes guidelines (24).
CVD was designated as the dominating outcome of the study, whereas heart disease and stroke were the secondary outcomes. The question “Have you been diagnosed with heart attack, coronary heart disease, angina, congestive heart failure, or other heart problems by a doctor?” identified whether participants were the incidence of heart disease; the question “Have you been diagnosed with stroke by a doctor?” indicated whether participants were the presence of stroke. The diagnosis of CVD was in the light of the participants’ self-reported medical histories concerning heart disease or stroke.
The TG and HDL-C levels were measured through the tests of blood sample conducted during Wave 1 and Wave 3, with both parameters expressed in mmol/L. AIP and cumulative AIP were gained using the following equations:
Demographic covariates included age, gender, marital status, Hukou status, educational level, smoking status and drinking status. Basic physical measurements comprised of systolic blood pressure, diastolic blood pressure, body mass index and waist circumference. Comorbidities and complications included hypertension, diabetes, dyslipidemia, MetS and kidney disease. Medication history included the use of antihypertensive, antidiabetic, and lipid-lowering drugs. Laboratory examination included white blood cell (WBC), hemoglobin (Hb), platelets, fasting blood glucose (FBG), glycated hemoglobin A1C, total cholesterol (TC), TG, HDL-C, low-density lipoprotein cholesterol (LDL-C), C-reactive protein (CRP), blood urea nitrogen, serum creatinine and eGFR.
K-means clustering analysis is a commonly used unsupervised learning algorithm that iteratively solves problems based on distance measurement (25). Using the elbow method, the dataset was categorized into four distinct clusters: Cluster 1, with AIP values ranging from -0.3467 to -0.2174, representing consistently low AIP levels; Cluster 2, with AIP values from 0.0409 to -0.0868, indicating moderate AIP levels that decrease over time; Cluster 3, with AIP values between 0.0576 and 0.2696, reflecting moderate AIP levels that increase over time; and Cluster 4, with AIP values from 0.5863 to 0.3866, indicating the highest AIP levels with a declining trend (Figure 1).
Figure 1. Clustering of the change in the AIP from 2012 to 2015. (a) The elbow method for determining the optimal number of clusters; (b) Data visualization for the clusters of the change in the AIP. AIP, atherogenic index of plasma.
The data distribution of all continuous variables was assessed employing the Shapiro-Wilk test, which indicated that all data were non-normally distributed. The continuous variables were presented as median and interquartile range (IQR). The categorical variables were expressed as counts and percentages. To compare statistical differences across the AIP clusters, either the Kruskal-Wallis test or Fisher’s exact test was used.
Multivariable logistic regression analyses were utilized to illuminate the relationships between AIP and CVD across four models; and all the results were summarized as odds ratio (OR) and 95% confidence interval (CI). The regression models were concluded as follows: Crude model I (unadjusted); adjusted model II (age and gender); adjusted model III (age, gender, marital status, Hukou status, educational level, smoking status, and drinking status); and adjusted model IV (age, gender, marital status, Hukou status, educational level, smoking status, drinking status, WBC, Hb, platelets and CRP). The potential dose–response relationships between cumulative AIP and CVD were inspected by restricted cubic spline (RCS) models, which were adjusting for same covariates as in Model IV. Additionally, subgroup analyses were performed to estimate the impact of confounding variables on the association between AIP and CVD. The weighted quantile sum (WQS) regression models were implemented to quantify the relative contributions of blood lipid and glucose levels to the overall effect.
Statistical analyses were performed using R software version 4.3.0; and statistical significance was set at p < 0.05.
In this study, a total of 4,525 participants diagnosed with CKM stage 0-3 were enrolled, with a median age of 58 years, and 2084 males (46.06%). The median AIP was -0.04 (-0.24, 0.19) in 2012 and -0.01 (-0.18, 0.20) in 2015, with a median cumulative AIP of -0.06 (-0.58, 0.52). Over the three-year follow-up period, the incidence of CVD, heart disease and stroke were observed to be 8.13%, 5.44% and 12.77%, respectively. On the basis of the clustering analysis, the baseline characteristics in each cluster are detailed in Table 1.
Four logistic regression models were developed to evaluate the associations between AIP and CVD incidence among populations across CKM syndrome stages 0-3 (Table 2). Compared to cluster 1, the OR (95% CI) for the prevalence of CVD were 1.29 (1.02-1.62) for cluster 2, 1.33 (1.04-1.71) for cluster 3 and 1.35 (0.98-1.85) for cluster 4 after adjusting for certain confounding covariates. Meanwhile, categorizing cumulative AIP into quartiles revealed that compared to the first quartile (Q1), the OR (95% CI) for Q2, Q3, and Q4 were 1.13 (0.87-1.48), 1.37 (1.05-1.77) and 1.33 (1.03-1.74). The trend for P value was 0.014, uncovering a significant association across the quartiles.
Table 2. Logistic regression analysis for the association between different classifications and CVD.
Supplementary Tables 1, 2 presented the associations between AIP and the incidence of the secondary outcomes. No significant relevance was observed between AIP and heart disease in the fully adjusted model (Model IV). Whereas, clusters 2 through 4 displayed a significantly elevated risk of stroke compared to cluster 1. Furthermore, a notable ascending trend in stroke incidence was observed from the first to the fourth quartile of cumulative AIP (P for trend <0.001).
There was a linearly incremental relationship between the cumulative AIP and the risk of CVD (P for association = 0.006, P for nonlinear = 0.18) and stroke (P for association < 0.001, P for nonlinear = 0.146) by the RCS model. However, the corresponding relationship between cumulative AIP and heart disease was not obvious (P for association = 0.146), consistent with the findings of the regression analysis (Figure 2).
Figure 2. The RCS curve of the association between cumulative AIP and CVD. (a) CVD; (b) heart disease; (c) stroke. RCS regression was adjusted for age, gender, marital status, Hukou status, educational level, smoking status, drinking status, white blood cell, hemoglobin, platelets and C-reactive protein. RCS, restricted cubic spline; AIP, atherogenic index of plasma; CVD, cardiovascular disease; OR, odds ratio; CI, confidence interval.
Tables 3, 4 showed the associations between the change in AIP and cumulative AIP with the presence of CVD, stratified by potential risk factors. After adjusting for demographic covariates, the significant interaction was demonstrated in subgroups based on Hukou status and gender.
Table 3. Associations of different clusters of change in the AIP with CVD stratified by different factors.
With respect to heart disease, no interaction was found between change in AIP clusters or cumulative AIP and subgroup variables (Supplementary Tables 3, 4). Except for Hukou status, no significant interactions were observed across subgroups for stroke (P for interaction >0.05) (Supplementary Tables 5, 6).
WQS regression models were utilized to thoroughly elucidate the risk factors for CVD. As illustrated in Supplementary Figure 2, TG accounted for a large proportion of the occurrence of both the primary and secondary outcomes.
To explore the association between AIP and CVD among older individuals with CKM syndrome stages 0–3, we performed a longitudinal study involving 4,525 participants from the CHARLS study. Our study confirmed that a chronically elevated AIP or a superior cumulative AIP was associated with a elevated incidence of CVD and stroke events, but no such association was observed with heart disease. Notably, TG was identified as the dominant contributing factor to CVD. The above results underscored that AIP level was a robust predictor of CVD risk among populations with CKM syndrome stages 0 to 3.
CKM syndrome typically arises from excess or dysfunctional adipose tissue (4), which accelerates atherosclerosis by exacerbating inflammation, lipid abnormalities, abnormal blood pressure, and insulin resistance (26). Thus, CKM syndrome represents the interconnected pathophysiological effects of metabolic risk factors of obesity, DM, CKD, and CVD. AIP was a composite parameter relevant to aberrant glucose and lipid metabolism (27), firstly proposed by Dobiásová and Frohlich (7). Zhu et al. affirmed that AIP was a positive and strong predictor of obesity (28). Besides, AIP has been linked to an increased risk of diabetes across various populations (10, 11), as well as prehypertension or hypertension (8, 29). A comprehensive review has further summarized that AIP may be associated with multiple cardiometabolic risk factors (30). Hu et al. indicated that AIP was positively correlated with CVD risk in Chinese individuals in CKM stages 0-3 (31). Zhang et al. demonstrated the prognostic values of the incidence of CVD by cumulative AIP and AIP control in individuals with CKM syndrome from stages 1 to 3 (32). Collectively, these studies have proved that AIP serves as a potent predictor of prognosis in CKM syndrome.
Min et al. revealed that constantly higher AIP may have a higher incidence of CVD in hyperglycemic individuals (17). In this study, the population was split into disparate clusters according to the dynamic changes of AIP levels from 2012 to 2015. Cluster 1 represented a consistently low AIP, as the control group; cluster 2 showed a moderate AIP with a descending trend; cluster 3 displayed a moderate AIP with a rising trend; and cluster 4 maintained the highest AIP despite a declining trend. The results indicated that when AIP remained within a high range - regardless of whether it increased or decreased - the risk of CVD events showed no improvement. In contrast, maintaining AIP at very low levels was related to a potentially dramatical reduction in the incidence of CVD. However, with regard to the secondary outcomes, no impact of AIP change on the cause of heart disease was observed, which was not consistent with findings from previous literature (19, 33, 34). A mass of studies primarily paid close attention to AIP measurements at a single time point, and failed to investigate the impact of dynamic fluctuations in AIP on the occurrence of CVD. This discrepancy highlights the need for further prospective trials to validate these divergent results in the future.
It has been reported that AIP was regarded as an independent predictor of CVD events (29), with the underlying mechanism attributed to its correlation with the size of lipoprotein particle (35). With the increase of AIP levels, the incidence of CVD presented a corresponding upward trend (35). Our study also throwed the latent relation between cumulative AIP and both primary and secondary outcomes. Specifically, there was a linear relationship between cumulative AIP and the risk of CVD and stroke. Thus, the interventions aimed at controlling AIP levels are crucial for reducing CVD risk.
The effect of Hukou status on the occurrence of CVD was showed in the subgroup analysis of this study. Our project confirmed that the availability of AIP in monitoring CVD was more potent in rural areas as a whole. Possible reasons may have contributed to this observation. First, there were shortage of medical institutions and healthcare professionals, making it difficult for rural residents to access timely diagnosis, treatment and preventive services. Second, the limited income of rural residents may hinder their ability to afford a healthy diet, regular medical check-ups, and necessary medications. Third, urbanization has contributed to the migration of young labors from rural areas, exacerbating the aging population in these regions.
At present, the most well-established risk factors for CVD events include lipoprotein and glucose metabolism disorders (36). In order to thoroughly investigate the contributions of these metabolic disorders to CVD, the WQS analyses were adapted to assess the relative proportion of TG, TC, HDL-C, LDL-C and FBG. Both the primary and secondary outcomes identified TG as a primary biochemical indicator contributing to CVD events.
Our study enhances the existing knowledge hierarchy by affording evidence in favor of the the utility of dynamic AIP changes as a valuable biologic-indicator for discerning individuals at high risk for CVD.
AIP, stemmed from the calculation of TG and HDL-C, can be conveniently measured from a single blood sample, offering a cost-effective and convenient alternative for risk assessment. Therefore, AIP holds significant promise as a promising potential marker for picking out patients at increased risk for CVD, particularly for stroke.
However, several limitations must be acknowledged in our study. First, our participants were exclusively drawn from the Chinese middle-aged and elderly populations, which may limit the applicability of our findings to other countries or age groups. Second, the participants self-reported CVD status, which may introduce over-reporting or under-reporting bias. Third, despite employing multivariate adjustments and conducting subgroup analyses, the study was a single-center research and some latent confounding covariates may have been overlooked.
In conclusion, we emphasized that significant changes in the AIP are independently related to the increased risk of CVD in Chinese individuals (age <45) with CKM syndrome stage 0-3. Consequently, supervising long-term fluctuations in AIP should be prioritized in CVD prevention strategies. Furthermore, our findings highlighted that TG was the dominating contributor to the gross effects. Moving forward, AIP holds promise as a precious appliance for assessing CVD risk in this group, but intensive studies are warranted to conduct in-depth analyses and explore its broader applicability.
The datasets presented in this study can be found in online repositories. The names of the repository/repositories and accession number(s) can be found below: https://charls.pku.edu.cn/.
The studies involving humans were approved by Peking University Biomedical Ethics Committee. The studies were conducted in accordance with the local legislation and institutional requirements. The participants provided their written informed consent to participate in this study.
YL: Conceptualization, Data curation, Formal Analysis, Methodology, Writing – original draft. XL: Formal Analysis, Visualization, Writing – original draft. CS: Formal Analysis, Resources, Writing – review & editing. TW: Data curation, Writing – review & editing. ZJ: Validation, Visualization, Writing – review & editing. CG: Conceptualization, Funding acquisition, Methodology, Project administration, Supervision, Writing – original draft. QJ: Writing – original draft.
The author(s) declare that financial support was received for the research and/or publication of this article. This work was supported by Science and Technology Project of Jiaxing (No. 2021AD30177), Qi Mingxing Project of First Hospital of Jiaxing (No. 2023-QMX-005), Key Construction Disciplines of Provincial and Municipal Co-construction of Zhejiang (No. 2023-SSGJ-002), Peak Discipline of Jiaxing First Hospital (No. 2021-GFXK-04), Medical and Health Science and Technology Research Program of Zhejiang Province (No. 2025KY1580) and Science and Technology Plan Projects of Jinhua City (No. 2022-4-106).
We appreciate the CHARLS research team, the organization and participants of the field survey, every respondent and all those who provided support for the visit.
The authors declare that the research was conducted in the absence of any commercial or financial relationships that could be construed as a potential conflict of interest.
The author(s) declare that no Generative AI was used in the creation of this manuscript.
All claims expressed in this article are solely those of the authors and do not necessarily represent those of their affiliated organizations, or those of the publisher, the editors and the reviewers. Any product that may be evaluated in this article, or claim that may be made by its manufacturer, is not guaranteed or endorsed by the publisher.
The Supplementary Material for this article can be found online at: https://www.frontiersin.org/articles/10.3389/fendo.2025.1540241/full#supplementary-material
AIP, Atherogenic index of plasma; CHARLS, China Health and Retirement Longitudinal Study; CI, Confidence interval; CKD, Chronic kidney disease; CKM, Cardiovascular-Kidney-Metabolic; CRP, C-reactive protein; CVD, Cardiovascular diseases; DM, Diabetes mellitus; eGFR, Estimated glomerular filtration ratio; FBG, Fasting blood glucose; Hb, Hemoglobin; HDL-C, High-density lipoprotein cholesterol; IQR, Interquartile range; IRB, Institutional Review Board; LDL-C, Low-density lipoprotein cholesterol; OR, Odds ratio; RCS, Restricted cubic spline; TC, Total cholesterol; TG, Triglyceride; WBC, White blood cell; WQS, Weighted quantile sum.
1. Diseases GBD, Injuries C. Global burden of 369 diseases and injuries in 204 countries and territories, 1990-2019: a systematic analysis for the Global Burden of Disease Study 2019. Lancet. (2020) 396:1204–22. doi: 10.1016/S0140-6736(20)30925-9
2. Diseases GBD, Injuries C. Global incidence, prevalence, years lived with disability (YLDs), disability-adjusted life-years (DALYs), and healthy life expectancy (HALE) for 371 diseases and injuries in 204 countries and territories and 811 subnational locations, 1990-2021: a systematic analysis for the Global Burden of Disease Study 2021. Lancet. (2024) 403:2133–61. doi: 10.1016/S0140-6736(24)00757-8
3. Woodruff RC, Tong X, Khan SS, Shah NS, Jackson SL, Loustalot F, et al. Trends in cardiovascular disease mortality rates and excess deaths, 2010-2022. Am J Prev Med. (2024) 66:582–9. doi: 10.1016/j.amepre.2023.11.009
4. Ndumele CE, Neeland IJ, Tuttle KR, Chow SL, Mathew RO, Khan SS, et al. A synopsis of the evidence for the science and clinical management of cardiovascular-kidney-metabolic (CKM) syndrome: A scientific statement from the American heart association. Circulation. (2023) 148:1636–64. doi: 10.1161/CIR.0000000000001186
5. Khan SS, Coresh J, Pencina MJ, Ndumele CE, Rangaswami J, Chow SL, et al. Novel prediction equations for absolute risk assessment of total cardiovascular disease incorporating cardiovascular-kidney-metabolic health: A scientific statement from the American heart association. Circulation. (2023) 148:1982–2004. doi: 10.1161/CIR.0000000000001191
6. Sebastian SA, Padda I, Johal G. Cardiovascular-Kidney-Metabolic (CKM) syndrome: A state-of-the-art review. Curr Probl Cardiol. (2024) 49:102344. doi: 10.1016/j.cpcardiol.2023.102344
7. Dobiasova M, Frohlich J. The plasma parameter log (TG/HDL-C) as an atherogenic index: correlation with lipoprotein particle size and esterification rate in apoB-lipoprotein-depleted plasma (FER(HDL)). Clin Biochem. (2001) 34:583–8. doi: 10.1016/s0009-9120(01)00263-6
8. Tan M, Zhang Y, Jin L, Wang Y, Cui W, Nasifu L, et al. Association between atherogenic index of plasma and prehypertension or hypertension among normoglycemia subjects in a Japan population: a cross-sectional study. Lipids Health Dis. (2023) 22:87. doi: 10.1186/s12944-023-01853-9
9. Shen SW, Lu Y, Li F, Yang CJ, Feng YB, Li HW, et al. Atherogenic index of plasma is an effective index for estimating abdominal obesity. Lipids Health Dis. (2018) 17:11. doi: 10.1186/s12944-018-0656-1
10. Zhang J, Suo Y, Wang L, Liu D, Jia Y, Fu Y, et al. Association between atherogenic index of plasma and gestational diabetes mellitus: a prospective cohort study based on the Korean population. Cardiovasc Diabetol. (2024) 23:237. doi: 10.1186/s12933-024-02341-9
11. Jiang L, Li L, Xu Z, Tang Y, Zhai Y, Fu X, et al. Non-linear associations of atherogenic index of plasma with prediabetes and type 2 diabetes mellitus among Chinese adults aged 45 years and above: a cross-sectional study from CHARLS. Front Endocrinol (Lausanne). (2024) 15:1360874. doi: 10.3389/fendo.2024.1360874
12. Li YW, Kao TW, Chang PK, Chen WL, Wu LW. Atherogenic index of plasma as predictors for metabolic syndrome, hypertension and diabetes mellitus in Taiwan citizens: a 9-year longitudinal study. Sci Rep. (2021) 11:9900. doi: 10.1038/s41598-021-89307-z
13. Zheng H, Wu K, Wu W, Chen G, Chen Z, Cai Z, et al. Relationship between the cumulative exposure to atherogenic index of plasma and ischemic stroke: a retrospective cohort study. Cardiovasc Diabetol. (2023) 22:313. doi: 10.1186/s12933-023-02044-7
14. Huang H, Yu X, Li L, Shi G, Li F, Xiao J, et al. Atherogenic index of plasma is related to coronary atherosclerotic disease in elderly individuals: a cross-sectional study. Lipids Health Dis. (2021) 20:68. doi: 10.1186/s12944-021-01496-8
15. Wu X, Qiu W, Yang H, Chen YJ, Liu J, Zhao G. Associations of the triglyceride-glucose index and atherogenic index of plasma with the severity of new-onset coronary artery disease in different glucose metabolic states. Cardiovasc Diabetol. (2024) 23:76. doi: 10.1186/s12933-024-02163-9
16. Kim JJ, Yoon J, Lee YJ, Park B, Jung DH. Predictive value of the atherogenic index of plasma (AIP) for the risk of incident ischemic heart disease among non-diabetic Koreans. Nutrients. (2021) 13:3231. doi: 10.3390/nu13093231
17. Min Q, Wu Z, Yao J, Wang S, Duan L, Liu S, et al. Association between atherogenic index of plasma control level and incident cardiovascular disease in middle-aged and elderly Chinese individuals with abnormal glucose metabolism. Cardiovasc Diabetol. (2024) 23:54. doi: 10.1186/s12933-024-02144-y
18. Zhang Y, Chen S, Tian X, Xu Q, Xia X, Zhang X, et al. Elevated atherogenic index of plasma associated with stroke risk in general Chinese. Endocrine. (2024) 84:934–42. doi: 10.1007/s12020-023-03677-0
19. Alifu J, Xiang L, Zhang W, Qi P, Chen H, Liu L, et al. Association between the atherogenic index of plasma and adverse long-term prognosis in patients diagnosed with chronic coronary syndrome. Cardiovasc Diabetol. (2023) 22:255. doi: 10.1186/s12933-023-01989-z
20. Zhao Y, Hu Y, Smith JP, Strauss J, Yang G. Cohort profile: the China health and retirement longitudinal study (CHARLS). Int J Epidemiol. (2014) 43:61–8. doi: 10.1093/ije/dys203
21. Ndumele CE, Rangaswami J, Chow SL, Neeland IJ, Tuttle KR, Khan SS, et al. Cardiovascular-kidney-metabolic health: A presidential advisory from the American heart association. Circulation. (2023) 148:1606–35. doi: 10.1161/CIR.0000000000001184
22. D’Agostino RB, Vasan RS, Pencina MJ, Wolf PA, Cobain M, Massaro JM, et al. General cardiovascular risk profile for use in primary care: the Framingham Heart Study. Circulation. (2008) 117:743–53. doi: 10.1161/CIRCULATIONAHA.107.699579
23. Ma YC, Zuo L, Chen JH, Luo Q, Yu XQ, Li Y, et al. Modified glomerular filtration rate estimating equation for Chinese patients with chronic kidney disease. J Am Soc Nephrol. (2006) 17:2937–44. doi: 10.1681/ASN.2006040368
24. Kidney Disease: Improving Global Outcomes CKDWG. KDIGO 2024 clinical practice guideline for the evaluation and management of chronic kidney disease. Kidney Int. (2024) 105:S117–314. doi: 10.1002/cbdv.202400200
25. Eckhardt CM, Madjarova SJ, Williams RJ, Ollivier M, Karlsson J, Pareek A, et al. Unsupervised machine learning methods and emerging applications in healthcare. Knee Surg Sports Traumatol Arthrosc. (2023) 31:376–81. doi: 10.1007/s00167-022-07233-7
26. Powell-Wiley TM, Poirier P, Burke LE, Despres JP, Gordon-Larsen P, Lavie CJ, et al. Obesity and cardiovascular disease: A scientific statement from the American heart association. Circulation. (2021) 143:e984–e1010. doi: 10.1161/CIR.0000000000000973
27. Qin Z, Zhou K, Li Y, Cheng W, Wang Z, Wang J, et al. The atherogenic index of plasma plays an important role in predicting the prognosis of type 2 diabetic subjects undergoing percutaneous coronary intervention: results from an observational cohort study in China. Cardiovasc Diabetol. (2020) 19:23. doi: 10.1186/s12933-020-0989-8
28. Zhu X, Yu L, Zhou H, Ma Q, Zhou X, Lei T, et al. Atherogenic index of plasma is a novel and better biomarker associated with obesity: a population-based cross-sectional study in China. Lipids Health Dis. (2018) 17:37. doi: 10.1186/s12944-018-0686-8
29. Hang F, Chen J, Wang Z, Zheng K, Wu Y. Association between the atherogenic index of plasma and major adverse cardiovascular events among non-diabetic hypertensive older adults. Lipids Health Dis. (2022) 21:62. doi: 10.1186/s12944-022-01670-6
30. Lioy B, Webb RJ, Amirabdollahian F. The association between the atherogenic index of plasma and cardiometabolic risk factors: A review. Healthcare (Basel). (2023) 11:966. doi: 10.3390/healthcare11070966
31. Hu Y, Liang Y, Li J, Li X, Yu M, Cui W. Correlation between atherogenic index of plasma and cardiovascular disease risk across Cardiovascular-kidney-metabolic syndrome stages 0-3: a nationwide prospective cohort study. Cardiovasc Diabetol. (2025) 24:40. doi: 10.1186/s12933-025-02593-z
32. Zhang Y, Song Y, Lu Y, Liu T, Yin P. Atherogenic index of plasma and cardiovascular disease risk in cardiovascular-kidneymetabolic syndrome stage 1 to 3: a longitudinal study. Front Endocrinol (Lausanne). (2025) 16:1517658. doi: 10.3389/fendo.2025.1517658
33. Won KB, Heo R, Park HB, Lee BK, Lin FY, Hadamitzky M, et al. Atherogenic index of plasma and the risk of rapid progression of coronary atherosclerosis beyond traditional risk factors. Atherosclerosis. (2021) 324:46–51. doi: 10.1016/j.atherosclerosis.2021.03.009
34. Ulloque-Badaracco JR, Hernandez-Bustamante EA, Alarcon-Braga EA, Mosquera-Rojas MD, Campos-Aspajo A, Salazar-Valdivia FE, et al. Atherogenic index of plasma and coronary artery disease: A systematic review. Open Med (Wars). (2022) 17:1915–26. doi: 10.1515/med-2022-0590
35. Dobiasova M, Frohlich J, Sedova M, Cheung MC, Brown BG. Cholesterol esterification and atherogenic index of plasma correlate with lipoprotein size and findings on coronary angiography. J Lipid Res. (2011) 52:566–71. doi: 10.1194/jlr.P011668
Keywords: atherogenic index of plasma, cardiovascular-kidney-metabolic syndrome, cardiovascular disease, CHARLS, K-mean clustering analysis
Citation: Lin Y, Lv X, Shi C, Wang T, Jin Z, Jin Q and Gu C (2025) Association between atherogenic index of plasma and future cardiovascular disease risk in middle-aged and elderly individuals with cardiovascular-kidney-metabolic syndrome stage 0-3. Front. Endocrinol. 16:1540241. doi: 10.3389/fendo.2025.1540241
Received: 05 December 2024; Accepted: 26 February 2025;
Published: 14 March 2025.
Edited by:
Taolin Chen, Sichuan University, ChinaReviewed by:
Ian James Martins, University of Western Australia, AustraliaCopyright © 2025 Lin, Lv, Shi, Wang, Jin, Jin and Gu. This is an open-access article distributed under the terms of the Creative Commons Attribution License (CC BY). The use, distribution or reproduction in other forums is permitted, provided the original author(s) and the copyright owner(s) are credited and that the original publication in this journal is cited, in accordance with accepted academic practice. No use, distribution or reproduction is permitted which does not comply with these terms.
*Correspondence: Chao Gu, Z3VjaGFvemp1QHpqdS5lZHUuY24=
†These authors have contributed equally to this work and share first authorship
Disclaimer: All claims expressed in this article are solely those of the authors and do not necessarily represent those of their affiliated organizations, or those of the publisher, the editors and the reviewers. Any product that may be evaluated in this article or claim that may be made by its manufacturer is not guaranteed or endorsed by the publisher.
Research integrity at Frontiers
Learn more about the work of our research integrity team to safeguard the quality of each article we publish.