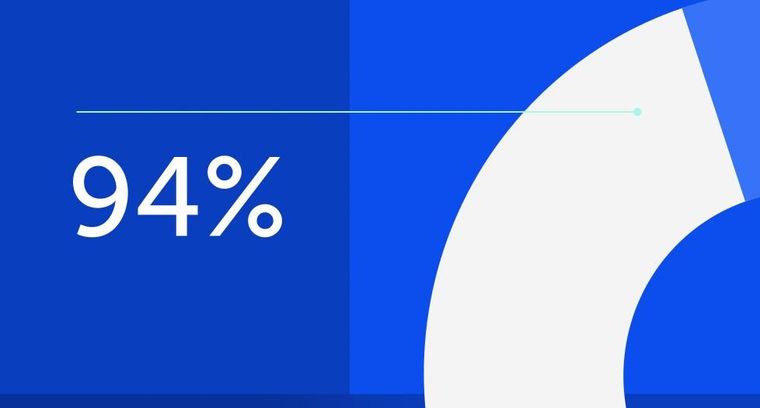
94% of researchers rate our articles as excellent or good
Learn more about the work of our research integrity team to safeguard the quality of each article we publish.
Find out more
ORIGINAL RESEARCH article
Front. Endocrinol.
Sec. Thyroid Endocrinology
Volume 16 - 2025 | doi: 10.3389/fendo.2025.1537386
The final, formatted version of the article will be published soon.
Select one of your emails
You have multiple emails registered with Frontiers:
Notify me on publication
Please enter your email address:
If you already have an account, please login
You don't have a Frontiers account ? You can register here
Objective: To develop and validate an interpretable machine learning (ML) model for the preoperative prediction of central lymph node metastasis (CLNM) in papillary thyroid microcarcinoma (PTMC).From December 2016 to December 2023, we retrospectively analyzed 710 PTMC patients who underwent thyroidectomies. Feature selection was conducted using the least absolute shrinkage and selection operator (LASSO) regression method, alongside the Support Vector Machine-Recursive Feature Elimination (SVM-RFE) algorithm in conjunction with multivariate logistic regression. Eight ML algorithms, namely Decision Tree, Random Forest (RF), K-nearest neighbors, Support vector machine, Extreme Gradient Boosting, Naive Bayes, Logistic regression, and Light Gradient Boosting machine, were developed for the prediction of CLNM. The performance of these models was evaluated using area under the receiver operating characteristic curve (AUC), decision curve analysis (DCA), sensitivity, specificity, accuracy, positive predictive value (PPV), negative predictive value (NPV), and F1 scores. Additionally, the Shapley Additive Explanation (SHAP) algorithm was utilized to clarify the results of the optimal ML model.Results:The results indicated that 32.95% of the patients (234/710) presented with CLNM. Tumor diameter, multifocality, lymph nodes identified via ultrasound (US-LN), and extrathyroidal extension (ETE) were identified as independent predictors of CLNM.The RF model achieved the highest performance in the validation set with an AUC of 0.893(95%CI: 0.846-0.940), accuracy of 0.832, sensitivity of 0.764, specificity of 0.866, PPV of 0.743, NPV of 0.879, and F1-score of 0.753. Furthermore, the DCA demonstrated that the RF model exhibited a superior clinical net benefit.Our model predicted the risk of CLNM in PTMC patients with high accuracy preoperatively.
Keywords: machine learning, papillary thyroid microcarcinoma, central lymph node metastasis, Diagnostic Imaging, SHapley Additive exPlanation
Received: 30 Nov 2024; Accepted: 17 Apr 2025.
Copyright: © 2025 Zhou, Lijuan, Hao, Wu, Liu, Zheng, Xia and Liu. This is an open-access article distributed under the terms of the Creative Commons Attribution License (CC BY). The use, distribution or reproduction in other forums is permitted, provided the original author(s) or licensor are credited and that the original publication in this journal is cited, in accordance with accepted academic practice. No use, distribution or reproduction is permitted which does not comply with these terms.
* Correspondence: Yong Liu, Department of Ultrasound, Beijing Shijitan Hospital, Capital Medical University, Beijing, China
Disclaimer: All claims expressed in this article are solely those of the authors and do not necessarily represent those of their affiliated organizations, or those of the publisher, the editors and the reviewers. Any product that may be evaluated in this article or claim that may be made by its manufacturer is not guaranteed or endorsed by the publisher.
Supplementary Material
Research integrity at Frontiers
Learn more about the work of our research integrity team to safeguard the quality of each article we publish.