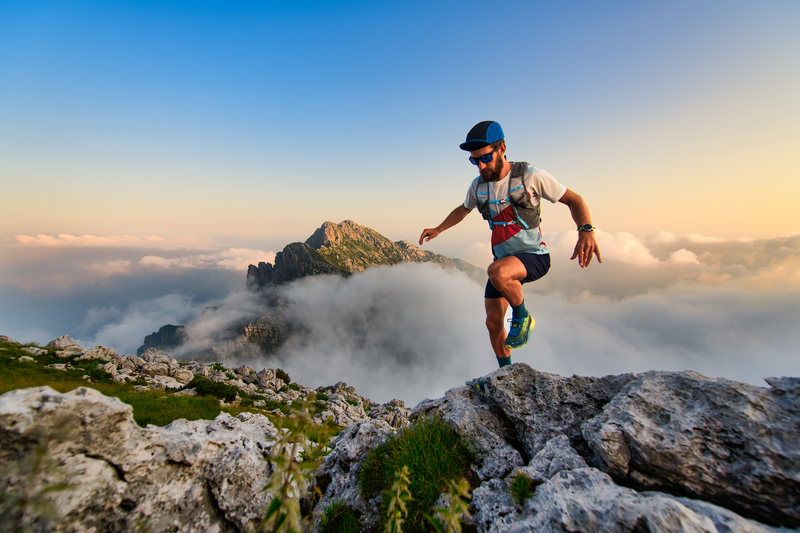
95% of researchers rate our articles as excellent or good
Learn more about the work of our research integrity team to safeguard the quality of each article we publish.
Find out more
ORIGINAL RESEARCH article
Front. Endocrinol. , 27 January 2025
Sec. Cardiovascular Endocrinology
Volume 16 - 2025 | https://doi.org/10.3389/fendo.2025.1529004
Background: The objective of this study was to explore the relationship between the triglyceride-glucose-body mass index (TyG-BMI) and all-cause mortality rate and to determine valuable predictive factors for the survival status of patients with cardiovascular disease (CVD).
Methods: Conduct a study on CVD patients in the NHANES database from 2007 to 2016. Patients were divided into four groups based on the weighted quartiles of TyG-BMI. Kaplan-Meier curves, Cox regression, and restricted cubic spline (RCS) were used to analyze the correlation between this index and all-cause mortality. Receiver operating characteristic (ROC) curves were used to evaluate its predictive ability, sensitivity, and specificity.
Results: This study included 1085 patients, and revealed significant differences in survival rates among patients with different TyG-BMI levels. Patients in the higher TyG-BMI group have a lower mortality risk, yet there is no evident non-linear relationship. The ROC curve indicates that this indicator can serve as a predictive value for mortality in CVD patients, demonstrating good sensitivity and specificity.
Conclusion: This study found a significant association between TyG-BMI index and all-cause mortality in patients with CVD. TyG-BMI can be used as a predictive indicator of all-cause mortality in CVD patients.
Cardiovascular disease(CVD), as a serious health problem, is the cause of death for one-third of the global population (1). Coronary heart disease(CHD) and heart failure(HF), as the most common cardiovascular diseases in clinical practice, can cause malignant arrhythmias, cardiogenic shock, and even sudden death. Its high incidence rate, disability and mortality rate pose a serious threat to the global health system (2, 3). Although the treatment of cardiovascular disease has made good progress, the mortality rate of patients has not been reduced to a satisfactory level. Therefore, early identification and intervention of risk factors that affect mortality are very important for the world’s public health system.
Insulin resistance (IR) is a condition in which the body’s sensitivity to insulin decreases, leading to ineffective uptake and utilization of blood glucose by target tissues and cells (4). In recent years, many studies have shown that IR is one of the important risk factors for cardiovascular, diabetes, kidney disease and liver disease (5–7). Triglyceride-glucose (TyG) and triglyceride glucose-body mass index (TyG-BMI), due to their ease of measurement and non-invasive nature, are replacing the high insulin normal glucose clamp test and Homeostasis Model Assessment of Insulin Resistance (HOMA-IR) as simple indicators for IR testing (8–10).
Studies have shown that there may be varying degrees of correlation between the TyG index and mortality rates among critically ill patients with acute myocardial infarction at different stages (11, 12). However, few studies have evaluated the relationship between the TyG-BMI index and all-cause mortality in patients with general cardiovascular diseases. Currently, there is still a lack of evidence to support the use of the TyG-BMI index as a long-term predictor of all-cause mortality risk in these patients. Therefore, this study aims to investigate the correlation and predictive value of the TyG-BMI index with all-cause mortality in CVD patients.
Data were obtained from the National Health and Nutrition Examination Survey (NHANES 2007–2016) and the National Death Index (NDI). This database collects the health and nutrition status, examination results, and follow-up outcomes of different populations in the United State. The NHANES protocol has obtained informed written consent from all participants in the study. Our study on the correlation between TyG-BMI index and all-cause mortality in cardiovascular disease patients used NHANES data from 2007 to 2016. The following patient groups were excluded from the investigation: (1) Participants under the age of 18; (2) Participants lacking fasting blood glucose, triglycerides, BMI, and mortality outcome data; (3) Participants who do not suffer from CVD (coronary heart disease, angina pectoris, myocardial infarction, and heart failure). The study ultimately included 1085 participants. The flowchart for research is shown in Figure 1:
The TyG-BMI index was designated as the primary variable of this investigation. Extract baseline data of patients from the database, Including demographic data [age, gender, race, education level, smoking], vital signs [BMI, systolic blood pressure SBP, diastolic blood pressure DBP], laboratory parameters [triglyceride, cholesterol, urinary creatinine, blood sugar, insulin], complications [diabetes, kidney disease]. To reduce potential bias, this study excluded variables with missing values exceeding 15%. Use the “mice” package in R software to handle missing data.
The TyG-BMI index was calculated using the following equation: In [TG (mg/dL) × FBG (mg/dL)/2] × BMI (11). CVD patients are those who have been informed by their doctors that they have coronary heart disease/angina pectoris/myocardial infarction/heart failure according to NHANES survey data. The primary endpoint of this study is all-cause mortality, The follow-up period starts from the date of the interview and ends on December 31, 2019.
Stratify population characteristics based on the weighted quartiles of the TyG-BMI index, with categorical variables being weighted percentages and continuous variables being weighted means and standard deviations. Compare the four groups using analysis of variance or Kruskal-Wallis test for continuous variables, and the chi-square test for categorical variables. To investigate the effect of different TyG-BMI index levels on all-cause mortality, Kaplan-Meier survival analysis was conducted and log-rank test was used for inter group comparison.
Evaluating the relationship between different TyG-BMI index and survival status using a combination of univariate and multivariate Cox proportional hazards models. We adjusted for different covariates and constructed three regression models. Model 1 is an unadjusted reference; Model 2 adjusted for age, gender, and race; Model 3, as a comprehensive adjustment, added smoking SBP, DBP, TC, insulin, covariates such as diabetes and kidney disease. Quantify the association between TyG BMI index and mortality rate by calculating the hazard ratios (HR) and 95% confidence intervals for different models. By establishing a restricted cubic spline RCS regression model, we explored the nonlinear relationships between variables (three nodes were selected in this study). Receiver operating characteristic (ROC) curves were used for diagnostic value analysis, and the area under the curve, as measured by the C-statistic, was computed to quantify the predictive power of TyG-BMI. The data analysis is conducted using R software (version 4.3.2). Use a P-value less than 0.05 to determine statistical significance.
As shown in Table 1, according to the weighted TyG-BMI index quartile level (Q1:<219.2; Q2:219.2~263.2; Q3:263.2~312.0;Q4:>312.0), Stratify the patients. The results showed that there were certain differences in age, gender, smoking, blood pressure, and comorbidities among the groups of patients. The patients in the group with the highest TyG-BMI index are younger, less male, have higher education levels, and have significantly higher BMI and serum insulin levels than the other three groups.
Figure 2 illustrates Kaplan-Meier survival analysis curves evaluating mortality rates between different TyG-BMI groups. The results showed that there was a significant difference in the survival rate within 160 months among the four groups of patients (p for log-rank test<0.05), and the survival rate in Q4 was significantly higher than the other three groups.
The correlation between TyG-BMI and all-cause mortality was analyzed using Cox proportional hazards regression, and the results are shown in Table 2. Using Q1 as the standard [Q1: HR=1], the Q4 group in Model 1 was significantly associated with lower all-cause mortality (HR 0.55, 95% CI 0.41-0.75). In Model 2, there were no significant differences among the groups. In Model 3, both the Q2 group (HR 0.75, 95% CI 0.57-0.98) and the Q4 group (HR 0.65, 95% CI 0.47-0.90) were associated with lower all-cause mortality rates. These results align with those obtained from the K-M survival curve.
As illustrated in Figure 3, the RCS curve shows the relationship between the TyG BMI index and all-cause mortality in patients with CVD. The results showed that there was no non-linear relationship between TyG BMI index and all-cause mortality rate (P for overall<0.05, P for nonlinear>0.05).
The ROC curve is shown in Figure 4, which is used to evaluate the sensitivity and specificity of TyG-BMI index as a diagnostic tool for all-cause mortality. Compared with the TyG index alone, TyG-BMI has a stronger predictive ability for CVD patients. TyG-BMI (AUC 0.548, 95%CI 0.513-0.583) vs. TyG (AUC 0.511, 95%CI 0.476-0.547). This study used Youden Index to determine the optimal critical point of TyG-BMI at 304.45, with a sensitivity of 79.0% and a specificity of 68.6%.
The objective of this study was to explore the relationship between the TyG-BMI index and all-cause mortality rate and to determine valuable predictive factors for the survival status of patients with CVD. Using Kaplan-Meier survival analysis, univariate and multivariate Cox proportional hazards regression models, RCS curve analysis, etc., it was determined that TyG-BMI index can be used as a predictive indicator for all-cause mortality in CVD patients, and compared with TyG, it has better predictive ability, higher sensitivity, and specificity. In addition, the survival analysis results indicate that patients with TyG-BMI>312 have the lowest all-cause mortality rate in both unadjusted and fully adjusted models. This means that non critical CVD patients may be able to achieve a lower risk of death by appropriately increasing their TyG-BMI. However, although the RCS curve results do not support a nonlinear relationship between the two (p=0.056), by fitting the curve trend in the graph, it can be seen that excessively high TyG-BMI will also increase the risk of all-cause mortality. Therefore, excessively increasing or decreasing TyG-BMI is not advisable behavior. This will help develop new guidelines for reducing the risk of death.
In recent years, TyG and its related indicators have been widely studied in various systemic diseases (13–15). TyG-BMI, as an effective alternative indicator of insulin resistance, is a key factor in evaluating type 2 diabetes, obesity, metabolic abnormalities, and dyslipidemia (16–19). However, the relationship between TyG and its related index and CVD is still inconclusive, and the existing research results are roughly the following two: the first conclusion is that there is a linear and positive correlation between TyG related index and the incidence, disease progression and mortality of CVD (20–23), which means that TyG and TyG-BMI are absolute risk factors for CVD (24, 25). Another conclusion is that the TyG related index has a nonlinear or U-shaped relationship with the occurrence and progression of CVD (26–28), which means that too high or too low TyG and TyG-BMI may lead to an increased risk of death in patients with CVD. This may be influenced by various factors, including the level of insulin resistance, inflammatory response, oxidative stress, and vascular endothelial function. Consequently, an excessively low TyG may also elevate the risk of cardiovascular mortality (29, 30). The findings of this study align with this conclusion. Furthermore, the RCS curve does not indicate the dispersion of the data. This could be attributed to the limited number of CVD patients, varying disease complexities, the configuration of RCS curve parameters, and model selection.
Both conclusions acknowledge the risk posed by a high TyG-related index to CVD. Prospective studies have indicated that a high TyG-BMI ratio can exacerbate the mortality risk among CVD patients (31), potentially due to insulin resistance-induced glucose metabolism disorders, lipotoxicity, and excessive inflammatory response (32). This study will not delve further into this matter, but will solely focus on the risk associated with an excessively low TyG-BMI index.
On the one hand, lower fasting blood glucose can significantly decrease TyG, and repeated hypoglycemia can cause serious irreversible damage to multiple organs, mainly the brain, leading to an increased risk of multifactorial cardiovascular and cerebrovascular death (33, 34). On the other hand, a low BMI may be associated with malnutrition, as individuals with lower BMI may have weaker immune function, metabolic capacity, and self-regulation compared to those with higher BMI within the normal range (35, 36). Therefore, this group of people may have a higher risk of death. In addition, in the baseline data of patients, we observed that those in the low TyG-BMI group had a higher average age and lower insulin levels. Previous studies have indicated that exogenous insulin can dilate and protect blood vessels, as well as alleviate myocardial ischemia-reperfusion injury (37, 38). Nevertheless, the association between endogenous high-level insulin and CVD requires further investigation.
Our research possesses several advantages. Firstly, we screened specific populations suffering from CVD, encompassing coronary heart disease, angina pectoris, myocardial infarction, and heart failure. CVD, being a common chronic illness and a leading cause of death posing significant harm, if its mortality risk can be predicted and intervened upon early, it will significantly alleviate the burden on the global public health system. Secondly, this study used the NHANES database, and the conclusions were representative to a certain extent. The influence of different covariates was fully adjusted in the statistical process. For missing data less than 15%, multiple imputation was used to supplement them, so that the interpolated data remained the same as the standard deviation of the mean to avoid bias caused by excessive deletion of missing data.
However, our research also has some limitations. Like other cross-sectional studies, this study was unable to establish a causal relationship between TyG-BMI index and all-cause mortality. In addition, although we have tried our best to adjust for potential confounding factors in the process of constructing statistical models, there are still many residual factors that have not been measured or included, which have a certain impact on the results. In addition, due to limitations in NHANES data, we were unable to obtain specific causes of death for some patients, thus preventing us from conducting in-depth analysis.
In summary, this study found a significant association between TyG-BMI index and all-cause mortality in patients with CVD. Compared with TyG, TyG-BMI can be used as a predictive indicator of all-cause mortality in CVD patients, and has good specificity and sensitivity.
The datasets presented in this study can be found in online repositories. The names of the repository/repositories and accession number(s) can be found below: https://www.cdc.gov/nchs/nhanes/.
The studies involving humans were approved by the NCHS Institutional Review Board (IRB), as detailed at: https://www.cdc.gov/nchs/nhanes/about/erb.html. As NHANES is a publicly available database with anonymized personal information, no additional ethical approval or informed consent was necessary. The studies were conducted in accordance with the local legislation and institutional requirements. Written informed consent for participation was not required from the participants or the participants’ legal guardians/next of kin in accordance with the national legislation and institutional requirements.
YS: Conceptualization, Methodology, Software, Visualization, Writing – original draft, Writing – review & editing. YH: Conceptualization, Funding acquisition, Supervision, Visualization, Writing – review & editing.
The author(s) declare financial support was received for the research, authorship, and/or publication of this article. Funded by Tianjin Key Medical Discipline (Specialty) Construction Project (TJYXZDXK-055B).
The authors declare that the research was conducted in the absence of any commercial or financial relationships that could be construed as a potential conflict of interest.
The author(s) declare that no Generative AI was used in the creation of this manuscript.
All claims expressed in this article are solely those of the authors and do not necessarily represent those of their affiliated organizations, or those of the publisher, the editors and the reviewers. Any product that may be evaluated in this article, or claim that may be made by its manufacturer, is not guaranteed or endorsed by the publisher.
1. WHO. Cardiovascular diseases factsheet. Available online at: https://www.who.int/news-room/fact-sheets/detail/cardiovascular-diseases-(cvds) (Accessed 7th March 2024).
2. Jentzer JC, Pöss J, Schaubroeck H, Morrow DA, Hollenberg SM, Mebazaa A. Advances in the management of cardiogenic shock. Crit Care Med. (2023) 51:1222–33. doi: 10.1097/CCM.0000000000005919
3. Redfern J, Gallagher R, Maiorana A, Candelaria D, Hollings M, Gauci S, et al. Cardiac rehabilitation and secondary prevention of CVD: time to think about cardiovascular health rather than rehabilitation. NPJ Cardiovasc Health. (2024) 1:22. doi: 10.1038/s44325-024-00017-7
4. Lee SH, Park SY, Choi CS. Insulin resistance: from mechanisms to therapeutic strategies. Diabetes Metab J. (2022) 46:15–37. doi: 10.4093/dmj.2021.0280
5. Valensi P. Evidence of a bi-directional relationship between heart failure and diabetes: a strategy for the detection of glucose abnormalities and diabetes prevention in patients with heart failure. Cardiovasc Diabetol. (2024) 23:354. doi: 10.1186/s12933-024-02436-3
6. Park D, Lim B, Lee O. Association between relative grip strength, insulin resistance, and nonalcoholic fatty liver disease among middle-aged and older adults: A prospective cohort study. Metab Syndr Relat Disord. (2024). doi: 10.1089/met.2024.0177
7. Lei M, Ling P, Zhou Y, Lv J, Ni Y, Deng H, et al. Correlation between triglyceride-glucose index and diabetic kidney disease risk in adults with type 1 diabetes mellitus. Diabetol Metab Syndr. (2024) 16:239. doi: 10.1186/s13098-024-01468-9
8. Tahapary DL, Pratisthita LB, Fitri NA, Marcella C, Wafa S, Kurniawan F, et al. Challenges in the diagnosis of insulin resistance: Focusing on the role of HOMA-IR and Tryglyceride/glucose index. Diabetes Metab Syndr. (2022) 16:102581. doi: 10.1016/j.dsx.2022.102581
9. Prystupa K, Renklint R, Chninou Y, Otten J, Fritsche L, Hoerber S, et al. Comprehensive validation of fasting-based and oral glucose tolerance test-based indices of insulin secretion against gold standard measures. BMJ Open Diabetes Res Care. (2022) 10:e002909. doi: 10.1136/bmjdrc-2022-002909
10. Lim J, Kim J, Koo SH, Kwon GC. Comparison of triglyceride glucose index, and related parameters to predict insulin resistance in Korean adults: An analysis of the 2007-2010 Korean National Health and Nutrition Examination Survey. PloS One. (2019) 14:e0212963. doi: 10.1371/journal.pone.0212963
11. Luo C, Li Q, Wang Z, Duan S, Ma Q. Association between triglyceride glucose-body mass index and all-cause mortality in critically ill patients with acute myocardial infarction: retrospective analysis of the MIMIC-IV database. Front Nutr. (2024) 11:1399969. doi: 10.3389/fnut.2024.1399969
12. Cheng Y, Fang Z, Zhang X, Wen Y, Lu J, He S, et al. Association between triglyceride glucose-body mass index and cardiovascular outcomes in patients undergoing percutaneous coronary intervention: a retrospective study. Cardiovasc Diabetol. (2023) 22:75. doi: 10.1186/s12933-023-01794-8
13. Ding L, Fu B, Zhang H, Dai C, Zhang A, Yu F, et al. The impact of triglyceride glucose-body mass index on all-cause and cardiovascular mortality in elderly patients with diabetes mellitus: evidence from NHANES 2007-2016. BMC Geriatr. (2024) 24:356. doi: 10.1186/s12877-024-04992-5
14. Zhan C, Peng Y, Ye H, Diao X, Yi C, Guo Q, et al. Triglyceride glucose-body mass index and cardiovascular mortality in patients undergoing peritoneal dialysis: a retrospective cohort study. Lipids Health Dis. (2023) 22:143. doi: 10.1186/s12944-023-01892-2
15. Park PG, Pyo JY, Ahn SS, Song JJ, Park YB, Huh JH, et al. New index using triglyceride glucose-body mass index for predicting mortality in patients with antineutrophil cytoplasmic antibody-associated vasculitis. Front Med (Lausanne). (2023) 10:1168016. doi: 10.3389/fmed.2023.1168016
16. Son DH, Lee HS, Lee YJ, Lee JH, Han JH. Comparison of triglyceride-glucose index and HOMA-IR for predicting prevalence and incidence of metabolic syndrome. Nutr Metab Cardiovasc Dis. (2022) 32:596–604. doi: 10.1016/j.numecd.2021.11.017
17. Wan H, Cao H, Ning P. Superiority of the triglyceride glucose index over the homeostasis model in predicting metabolic syndrome based on NHANES data analysis. Sci Rep. (2024) 14:15499. doi: 10.1038/s41598-024-66692-9
18. Ramdas Nayak VK, Satheesh P, Shenoy MT, Kalra S. Triglyceride Glucose (TyG) Index: A surrogate biomarker of insulin resistance. J Pak Med Assoc. (2022) 72:986–8. doi: 10.47391/JPMA.22-63
19. Ference BA, Graham I, Tokgozoglu L, Catapano AL. Impact of lipids on cardiovascular health: JACC health promotion series. J Am Coll Cardiol. (2018) 72:1141–56. doi: 10.1016/j.jacc.2018.06.046
20. Cui C, Liu L, Qi Y, Han N, Xu H, Wang Z, et al. Joint association of TyG index and high sensitivity C-reactive protein with cardiovascular disease: a national cohort study. Cardiovasc Diabetol. (2024) 23:156. doi: 10.1186/s12933-024-02244-9
21. Zhou Z, Liu Q, Zheng M, Zuo Z, Zhang G, Shi R, et al. Comparative study on the predictive value of TG/HDL-C, TyG and TyG-BMI indices for 5-year mortality in critically ill patients with chronic heart failure: a retrospective study. Cardiovasc Diabetol. (2024) 23:213. doi: 10.1186/s12933-024-02308-w
22. Huang R, Wang Z, Chen J, Bao X, Xu N, Guo S, et al. Prognostic value of triglyceride glucose (TyG) index in patients with acute decompensated heart failure. Cardiovasc Diabetol. (2022) 21:88. doi: 10.1186/s12933-022-01507-7
23. Huang YC, Huang JC, Lin C, Chien HH, Lin YY, Wang CL, et al. Comparison of innovative and traditional cardiometabolic indices in estimating atherosclerotic cardiovascular disease risk in adults. Diagnostics. (2021) 11:603. doi: 10.3390/diagnostics11040603
24. Ren Q, Huang Y, Liu Q, Chu T, Li G, Wu Z, et al. Association between triglyceride glucose-waist height ratio index and cardiovascular disease in middle-aged and older Chinese individuals: a nationwide cohort study. Cardiovasc Diabetol. (2024) 23:247. doi: 10.1186/s12933-024-02336-6
25. Lopez-Jaramillo P, Gomez-Arbelaez D, Martinez-Bello D, Abat MEM, Alhabib KF, Avezum A, et al. Association of the triglyceride glucose index as a measure of insulin resistance with mortality and cardiovascular disease in populations from five continents (PURE study): a prospective cohort study. Lancet Healthy Longev. (2023) 4:e23–33. doi: 10.1016/S2666-7568(22)00247-1
26. Hu B, Wang Y, Wang Y, Feng J, Fan Y, Hou L. Association between Triglyceride-Glucose Index and risk of all-cause and cardiovascular mortality in adults with prior cardiovascular disease: a cohort study using data from the US National Health and Nutrition Examination Survey, 2007-2018. BMJ Open. (2024) 14:e084549. doi: 10.1136/bmjopen-2024-084549
27. Dou J, Guo C, Wang Y, Peng Z, Wu R, Li Q, et al. Association between triglyceride glucose-body mass and one-year all-cause mortality of patients with heart failure: a retrospective study utilizing the MIMIC-IV database. Cardiovasc Diabetol. (2023) 22:309. doi: 10.1186/s12933-023-02047-4
28. Zhang Q, Xiao S, Jiao X, Shen Y. The triglyceride-glucose index is a predictor for cardiovascular and all-cause mortality in CVD patients with diabetes or pre-diabetes: evidence from NHANES 2001-2018. Cardiovasc Diabetol. (2023) 22:279. doi: 10.1186/s12933-023-02030-z
29. Kizer J, Arnold A, Jenny N, Cushman M, Strotmeyer E, Ives D, et al. Longitudinal changes in adiponectin and inflammatory markers and relation to survival in the oldest old: the cardiovascular health study all stars study. J Gerontol A Biol Sci Med Sci. (2011) 66:1100–7. doi: 10.1093/gerona/glr098
30. Ludin A, Gur-Cohen S, Golan K, Kaufmann K, Itkin T, Medaglia C, et al. Reactive oxygen species regulate hematopoietic stem cell self-renewal, migration and development, as well as their bone marrow microenvironment. Antioxid Redox Signal. (2014) 21:1605–19. doi: 10.1089/ars.2014.5941
31. Li F, Wang Y, Shi B, Sun S, Wang S, Pang S, et al. Association between the cumulative average triglyceride glucose-body mass index and cardiovascular disease incidence among the middle-aged and older population: a prospective nationwide cohort study in China. Cardiovasc Diabetol. (2024) 23:16. doi: 10.1186/s12933-023-02114-w
32. Szukiewicz D. Molecular mechanisms for the vicious cycle between insulin resistance and the inflammatory response in obesity. Int J Mol Sci. (2023) 24:9818. doi: 10.3390/ijms24129818
33. He J, Zhang Y, Cheng X, Tian Y, Hao P, Li T, et al. Association of postoperative hypoglycemia with mortality after elective craniotomy. Neurosurgery. (2024) 95:682–91. doi: 10.1227/neu.0000000000002938
34. Johnston LE, Kirby JL, Downs EA, LaPar DJ, Ghanta RK, Ailawadi G, et al. Postoperative hypoglycemia is associated with worse outcomes after cardiac operations. Ann Thorac Surg. (2017) 103:526–32. doi: 10.1016/j.athoracsur.2016.05.121
35. Cheong CY, Yap P, Yap KB, Ng TP. Associations of inflammatory, metabolic, malnutrition, and frailty indexes with multimorbidity incidence and progression, and mortality impact: Singapore longitudinal aging study. Gerontology. (2023) 69:416–27. doi: 10.1159/000527428
36. Chen T, Wan H, Luo Y, Chen L. Association of triglyceride-glucose-body mass index with all-cause and cardiovascular mortality among individuals with chronic kidney disease. Sci Rep. (2024) 14:20593. doi: 10.1038/s41598-024-71579-w
37. Yazar A, Polat G, Un I, Levent A, Kaygusuz A, Büyükafşar K, et al. Effects of glibenclamide, metformin and insulin on the incidence and latency of death by oubain-induced arrhythmias in mice. Pharmacol Res. (2002) 45:183–7. doi: 10.1006/phrs.2001.0944
Keywords: NHANES, TyG-BMI, cardiovascular disease, TyG index, CVD (cardio vascular disease)
Citation: Sun Y and Hu Y (2025) Association of triglyceride-glucose-body mass index with all-cause mortality among individuals with cardiovascular disease: results from NHANES. Front. Endocrinol. 16:1529004. doi: 10.3389/fendo.2025.1529004
Received: 15 November 2024; Accepted: 09 January 2025;
Published: 27 January 2025.
Edited by:
Gaetano Santulli, Albert Einstein College of Medicine, United StatesReviewed by:
Barbara Ciastek, University of Opole, PolandCopyright © 2025 Sun and Hu. This is an open-access article distributed under the terms of the Creative Commons Attribution License (CC BY). The use, distribution or reproduction in other forums is permitted, provided the original author(s) and the copyright owner(s) are credited and that the original publication in this journal is cited, in accordance with accepted academic practice. No use, distribution or reproduction is permitted which does not comply with these terms.
*Correspondence: Yuecheng Hu, SHdoaXRlY3JhbmVAMTYzLmNvbQ==
Disclaimer: All claims expressed in this article are solely those of the authors and do not necessarily represent those of their affiliated organizations, or those of the publisher, the editors and the reviewers. Any product that may be evaluated in this article or claim that may be made by its manufacturer is not guaranteed or endorsed by the publisher.
Research integrity at Frontiers
Learn more about the work of our research integrity team to safeguard the quality of each article we publish.