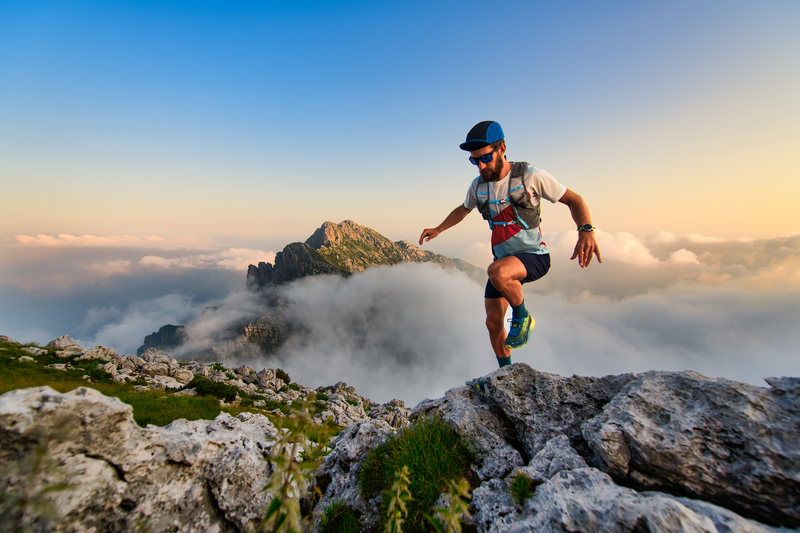
94% of researchers rate our articles as excellent or good
Learn more about the work of our research integrity team to safeguard the quality of each article we publish.
Find out more
ORIGINAL RESEARCH article
Front. Endocrinol.
Sec. Cancer Endocrinology
Volume 16 - 2025 | doi: 10.3389/fendo.2025.1521940
This article is part of the Research Topic Cancer, Metabolism and Kidney Injury: From Molecular Mechanisms to Therapy View all articles
The final, formatted version of the article will be published soon.
You have multiple emails registered with Frontiers:
Please enter your email address:
If you already have an account, please login
You don't have a Frontiers account ? You can register here
Background: Clear cell renal cell carcinoma is the most predominant type of renal malignancies, characterized by high aggressiveness and probability of distant metastasis. Renin angiotensin system (RAS) plays a crucial role in maintaining fluid balance within the human body, and its involvement in tumorigenesis is increasingly being uncovered, while its role in ccRCC remains unclear.Methods: WGCNA was used to identify RAS related genes. Machine learning was applied to screen hub genes for constructing risk model, E-MTAB-1980 dataset was used for external validation. Transwell and CCK8 assays were used to investigate the impact of SLC6A19 to ccRCC cells.Results: SLC6A19, SLC16A12 and SMIM24 were eventually screened to construct risk model and the predictive efficiency for prognosis was validated by internal and external cohorts. Moreover, the differences were found in pathway enrichment, immune cell infiltration, mutational landscapes and drug prediction between high and low risk groups. Experimental results indicated that SLC6A19 could inhibit invasion and proliferation of ccRCC cells and GSEA pinpointed that SLC6A19 was intimately correlated with fatty acid metabolism and CPT1A.The risk model based on the three RAS-related genes have a robust ability to predict the prognosis and drug sensitivity of ccRCC patients, further providing a valid instruction for clinical care.
Keywords: Risk model, ccRCC, renin angiotensin system, SLC6A19, CPT1a
Received: 03 Nov 2024; Accepted: 11 Feb 2025.
Copyright: © 2025 Chang, Zhao, Sun, Guo, Yang, Qiu, Zhang, Fan and Liu. This is an open-access article distributed under the terms of the Creative Commons Attribution License (CC BY). The use, distribution or reproduction in other forums is permitted, provided the original author(s) or licensor are credited and that the original publication in this journal is cited, in accordance with accepted academic practice. No use, distribution or reproduction is permitted which does not comply with these terms.
* Correspondence:
Yidong Fan, Qilu Hospital, Shandong University, Jinan, 250012, Shandong Province, China
Jikai Liu, Shandong University, Jinan, China
Disclaimer: All claims expressed in this article are solely those of the authors and do not necessarily represent those of their affiliated organizations, or those of the publisher, the editors and the reviewers. Any product that may be evaluated in this article or claim that may be made by its manufacturer is not guaranteed or endorsed by the publisher.
Research integrity at Frontiers
Learn more about the work of our research integrity team to safeguard the quality of each article we publish.