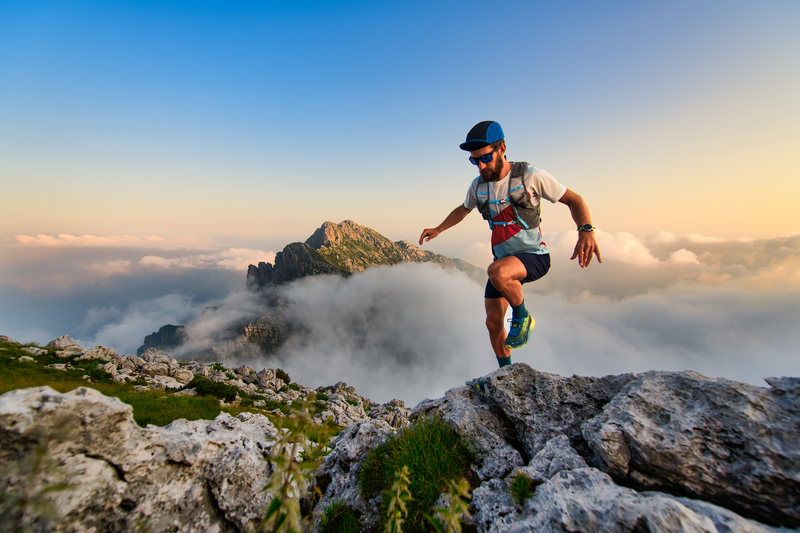
94% of researchers rate our articles as excellent or good
Learn more about the work of our research integrity team to safeguard the quality of each article we publish.
Find out more
ORIGINAL RESEARCH article
Front. Endocrinol.
Sec. Clinical Diabetes
Volume 16 - 2025 | doi: 10.3389/fendo.2025.1518060
This article is part of the Research Topic Advances in Diabetes and Hypertension Research View all 8 articles
The final, formatted version of the article will be published soon.
You have multiple emails registered with Frontiers:
Please enter your email address:
If you already have an account, please login
You don't have a Frontiers account ? You can register here
The Atherogenic Index of Plasma (AIP) is recognized as a surrogate marker for dyslipidemia. It has been well-established that the AIP is significantly associated with diabetes, and obesity is a known risk factor for both dyslipidemia and diabetes. However, the relationship between obesity and diabetes, as well as the potential role of AIP in hypertensive minority populations, remains unclear. This study aimed to assess the association between obesity index and diabetes in hypertensive people.This cross-sectional study included 9, 446 participants from the China Multi-Ethnicity Cohort (CMEC) Study. Our study suggested that obesity indices were significant higher in diabetic patients compared to those without. Moreover, logistic regression analysis suggested that higher quartiles of obesity indices were associated with an increased risk of diabetes whether in crude or adjusted models (P< 0.05). Mediation analysis revealed that the association between obesity and the risk diabetes, mediated by body mass index (BMI), waist-to-hip ratio (WHR), waist-to-height ratio (WHtR), and body adiposity index (BAI) through AIP being 17.2%, 15.3%, 15.8%, and 17.219.2%, respectively. Additionally, restricted cubic spline analysis revealed a non-linear relationship between obesity indices and diabetes.In summary, obesity is significantly associated with diabetes in hypertensive minority Chinese, with the AIP partially mediating this relationship.
Keywords: Atherogenic index of plasma, Obesity, diabetes, Hypertension, minority
Received: 27 Oct 2024; Accepted: 14 Feb 2025.
Copyright: © 2025 Zhou and Hong. This is an open-access article distributed under the terms of the Creative Commons Attribution License (CC BY). The use, distribution or reproduction in other forums is permitted, provided the original author(s) or licensor are credited and that the original publication in this journal is cited, in accordance with accepted academic practice. No use, distribution or reproduction is permitted which does not comply with these terms.
* Correspondence:
Feng Hong, Guizhou Medical University, Guiyang, China
Disclaimer: All claims expressed in this article are solely those of the authors and do not necessarily represent those of their affiliated organizations, or those of the publisher, the editors and the reviewers. Any product that may be evaluated in this article or claim that may be made by its manufacturer is not guaranteed or endorsed by the publisher.
Research integrity at Frontiers
Learn more about the work of our research integrity team to safeguard the quality of each article we publish.