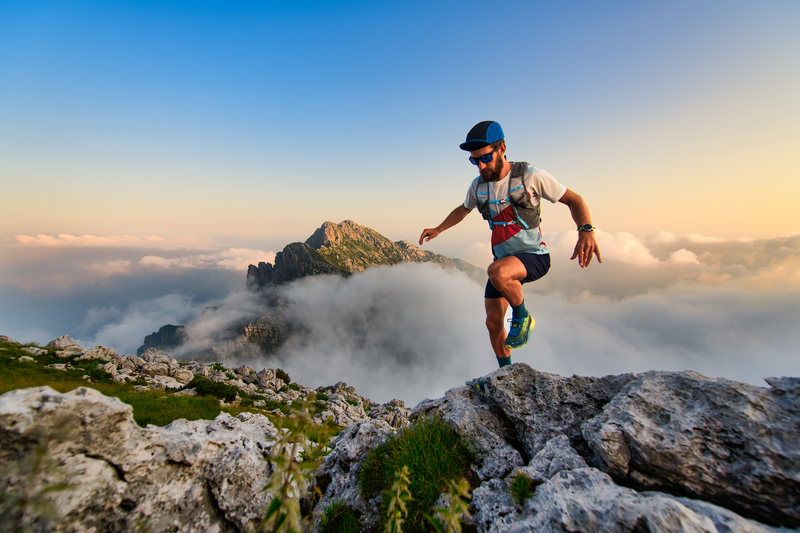
95% of researchers rate our articles as excellent or good
Learn more about the work of our research integrity team to safeguard the quality of each article we publish.
Find out more
ORIGINAL RESEARCH article
Front. Endocrinol.
Sec. Cardiovascular Endocrinology
Volume 16 - 2025 | doi: 10.3389/fendo.2025.1515559
The final, formatted version of the article will be published soon.
You have multiple emails registered with Frontiers:
Please enter your email address:
If you already have an account, please login
You don't have a Frontiers account ? You can register here
This study compares the association of eight insulin resistance (IR)-related markers (triglyceride-glucose index (TyG), TyG-body mass index (TyG-BMI), TyG-waist circumference (TyG-WC), TyG-waist-to-height ratio (TyG-WHtR), triglycerides-to-high-density lipoprotein cholesterol ratio (TG/HDL), lipid accumulation product (LAP), visceral adiposity index (VAI), and estimated glucose disposal rate (eGDR)) with cardiovascular disease (CVD).Methods: Spearman's coefficients were used for correlations between IR-related markers. Predictive capacities were evaluated using receiver operating characteristic (ROC) curve analysis, Akaike Information Criterion, and Bayesian Information Criterion were calculated. Multivariable-adjusted Cox regression models and restricted cubic spline (RCS) analysis were performed to explore associations between IR-related markers and CVD.In Pearson correlation analysis, TyG-WC and TyG-WHtR had a correlation coefficient of 0.95, while TG/HDL ratio and VAI had a correlation coefficient of 0.97.Regarding predictive capacity across different glycemic states, eGDR showed the best performance among the 8 IR-related markers, particularly in predicting stroke.According to Cox regression analysis, with each unit increase in TyG, TyG-BMI, TyG-WC, and TyG-WHtR, the risk of heart disease increased by 24.1%, 0.4%, 0.1%, and 17.56%, respectively; and the risk of stroke increased by 69.3%, 0.6%, 0.2%, and 36.5%, respectively. Additionally, TG/HDL ratio, VAI, and LAP exhibited nonlinear associations with heart disease and stroke risk. For each unit increase in eGDR, the risks of heart disease and stroke decreased by 21% and 14.2%, respectively Conclusion: eGDR is the most effective marker for predicting CVD, especially stroke, across all glycemic states. Modified TyG indices provide better predictive value than TyG alone.
Keywords: Stroke, Heart disease, Insulin Resistance, cardiovascular, Glucose
Received: 23 Oct 2024; Accepted: 02 Apr 2025.
Copyright: © 2025 Zhang and Tan. This is an open-access article distributed under the terms of the Creative Commons Attribution License (CC BY). The use, distribution or reproduction in other forums is permitted, provided the original author(s) or licensor are credited and that the original publication in this journal is cited, in accordance with accepted academic practice. No use, distribution or reproduction is permitted which does not comply with these terms.
* Correspondence:
Lan Tan, Qingdao Municipal Hospital, Qingdao University Medical College, Qingdao, China
Disclaimer: All claims expressed in this article are solely those of the authors and do not necessarily represent those of their affiliated organizations, or those of the publisher, the editors and the reviewers. Any product that may be evaluated in this article or claim that may be made by its manufacturer is not guaranteed or endorsed by the publisher.
Research integrity at Frontiers
Learn more about the work of our research integrity team to safeguard the quality of each article we publish.