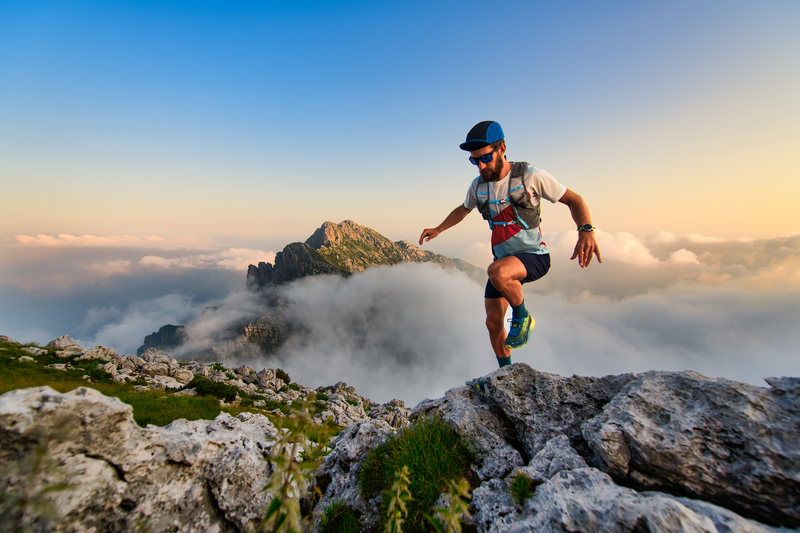
94% of researchers rate our articles as excellent or good
Learn more about the work of our research integrity team to safeguard the quality of each article we publish.
Find out more
ORIGINAL RESEARCH article
Front. Endocrinol.
Sec. Bone Research
Volume 16 - 2025 | doi: 10.3389/fendo.2025.1506841
The final, formatted version of the article will be published soon.
You have multiple emails registered with Frontiers:
Please enter your email address:
If you already have an account, please login
You don't have a Frontiers account ? You can register here
Introduction: Despite accumulating evidence on central obesity and osteoporosis, the role of a body shape index (ABSI), a nonlinear index quantifying body shape via body mass index (BMI), waist circumference (WC), and height, remains controversial and underexplored. Although recent meta-analyses suggest central obesity may modulate fracture risk bidirectionally, no research has comprehensively compared ABSI with traditional adiposity metrics, such as BMI, WC, and waist-to-height ratio (WHtR), to predict site-specific changes in bone mineral density (BMD) across anatomical regions. Methods: This study utilized National Health and Nutrition Examination Survey (NHANES) data from 2005 to 2018, involving 12,421 participants. ABSI was computed using the formula: ABSI = WC / (BMI²/³ × Height¹/²). BMD was assessed at four sites—the total femur (TF), femoral neck (FN), trochanter (TR), and intertrochanter (IN) regions—via dual-energy X-ray absorptiometry (DXA). The association between ABSI and BMD was analyzed via multiple regression models and a generalized additive model (GAM). To compare ABSI's predictive efficacy with conventional adiposity indices, regression analyses juxtaposed ABSI against BMI, WC, and WHtR in assessing correlations with site-specific BMD.Results: After full covariate adjustment, a significant negative association was observed between ABSI and BMD in four femoral regions (P < 0.01). Smoothed curve fitting revealed a significant nonlinear relationship and threshold effect between ABSI and BMD among middle-aged and older individuals. Additionally, an inverted J-shaped curve was observed between ABSI and BMD in all four femoral regions. Meanwhile, ABSI showed significant negative associations with BMD across all femoral sites (β = -0.27 to -0.31, p-trend < 0.000001), whereas BMI, WC, and WHtR exhibited positive correlations (WHtR showing the strongest effect: β = 0.41–0.69). This highlights ABSI’s ability to detect central adiposity-related bone loss obscured by conventional obesity metrics.Conclusion: ABSI’s robust inverse associations with femoral BMD (β = -0.27 to -0.31), persisting across nonlinear threshold analyses, establish it as a novel biomarker of central adiposity-related skeletal fragility. Unlike conventional indices reflecting mechanical loading benefits (BMI β = 0.008–0.012; WC β = 0.003–0.005; WHtR β = 0.41–0.69), ABSI specifically captures visceral fat-driven metabolic disorder—a critical pathway for osteoporosis risk stratification in normal-weight and obese populations.
Keywords: bone mineral density1, osteoporosis2, Abdominal Obesity3, A body shape index4, NHANES5
Received: 06 Oct 2024; Accepted: 20 Mar 2025.
Copyright: © 2025 Wang, Ma, Xu, Chen, Yang, Qin, Duan, Feng, Yin, Sun and Zhu. This is an open-access article distributed under the terms of the Creative Commons Attribution License (CC BY). The use, distribution or reproduction in other forums is permitted, provided the original author(s) or licensor are credited and that the original publication in this journal is cited, in accordance with accepted academic practice. No use, distribution or reproduction is permitted which does not comply with these terms.
* Correspondence:
He Yin, Department of Spine, Wangjing Hospital, China Academy of Chinese Medical Sciences, Beijing, China
Kai Sun, Department of Spine, Wangjing Hospital, China Academy of Chinese Medical Sciences, Beijing, China
Li Guo Zhu, Department of Spine, Wangjing Hospital, China Academy of Chinese Medical Sciences, Beijing, China
Disclaimer: All claims expressed in this article are solely those of the authors and do not necessarily represent those of their affiliated organizations, or those of the publisher, the editors and the reviewers. Any product that may be evaluated in this article or claim that may be made by its manufacturer is not guaranteed or endorsed by the publisher.
Research integrity at Frontiers
Learn more about the work of our research integrity team to safeguard the quality of each article we publish.