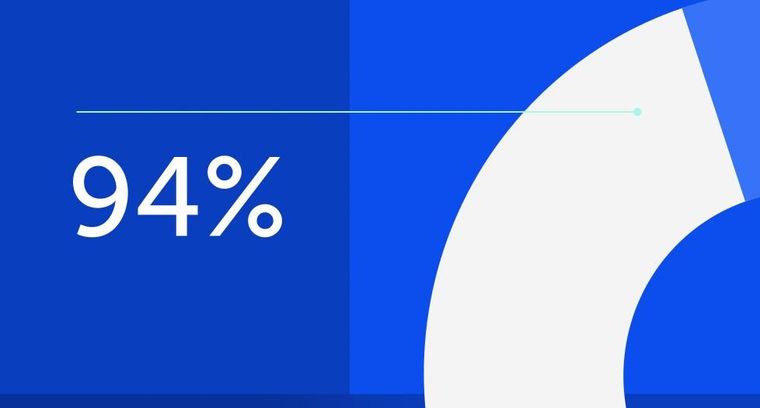
94% of researchers rate our articles as excellent or good
Learn more about the work of our research integrity team to safeguard the quality of each article we publish.
Find out more
SYSTEMATIC REVIEW article
Front. Endocrinol.
Sec. Thyroid Endocrinology
Volume 16 - 2025 | doi: 10.3389/fendo.2025.1506729
The final, formatted version of the article will be published soon.
Select one of your emails
You have multiple emails registered with Frontiers:
Notify me on publication
Please enter your email address:
If you already have an account, please login
You don't have a Frontiers account ? You can register here
In recent years, artificial intelligence (AI) tools have become widely studied for thyroid ultrasonography (USG) classification. The real-world applicability of these developed tools as pre-operative diagnostic aids is limited due to model overfitting, clinician trust and a lack of gold standard surgical histology as ground truth class label. The ongoing dilemma within clinical thyroidology is the surgical decision making for indeterminate thyroid nodules (ITN).Genomic sequencing classifiers (GSC) have been utilised for this purpose however costs and availability preclude universal adoption, creating an inequity gap. We conducted this review to analyse the current evidence of AI in ITN diagnosis without the use of GSC. English language articles evaluating the diagnostic accuracy of AI for ITNs were identified. A systematic search of PubMed, Google Scholar and Scopus from inception to February 18th 2025 was performed using comprehensive search strategies incorporating MeSH headings and key words relating to AI, indeterminate thyroid nodules and pre-operative diagnosis. This systematic review and meta-analysis was conducted in accordance with methods recommended by the Cochrane Collaboration (PROSPERO ID CRD42023438011).The search strategy yielded 134 records after the removal of duplicates. A total of 20 models were presented in the seven studies included; five of which were radiological driven, one utilised natural language processing and one focused on cytology. The pooled meta-analysis incorporated 16 area under the curve (AUC) results derived from 15 models across three studies, yielding a combined estimate of 0.82(95% CI: 0.81-0.84), indicating moderate to good classification performance across machine learning (ML) and deep learning (DL) architectures. However, substantial heterogeneity was observed, particularly among DL models (I²=99.7%, pooled AUC = 0.85,95% CI: 0.85-0.86). Minimal heterogeneity was observed among ML models (I²=0.7%), with a pooled AUC of 0.75(95% CI: 0.70-0.81). Meta-regression performed suggests potential publication bias or systematic differences in model architectures, dataset composition and validation methodologies.This review demonstrated the burgeoning potential of AI to be of clinical value in surgical decision making for ITNs however, studies developed models were unsuitable for clinical implementation based on performance alone at their current states or lacked robust independent external validation. There is substantial capacity for further development in this field.
Keywords: artificial intelligence, Thyroid cancer, Thyroid nodule - diagnosis, Meta - analysis, machine learning
Received: 06 Oct 2024; Accepted: 09 Apr 2025.
Copyright: © 2025 Jassal, Edwards, Koohestani, Brown, Serpell and Lee. This is an open-access article distributed under the terms of the Creative Commons Attribution License (CC BY). The use, distribution or reproduction in other forums is permitted, provided the original author(s) or licensor are credited and that the original publication in this journal is cited, in accordance with accepted academic practice. No use, distribution or reproduction is permitted which does not comply with these terms.
* Correspondence: Karishma Jassal, Monash University Endocrine Surgery Unit, Melbourne, Australia
Disclaimer: All claims expressed in this article are solely those of the authors and do not necessarily represent those of their affiliated organizations, or those of the publisher, the editors and the reviewers. Any product that may be evaluated in this article or claim that may be made by its manufacturer is not guaranteed or endorsed by the publisher.
Supplementary Material
Research integrity at Frontiers
Learn more about the work of our research integrity team to safeguard the quality of each article we publish.