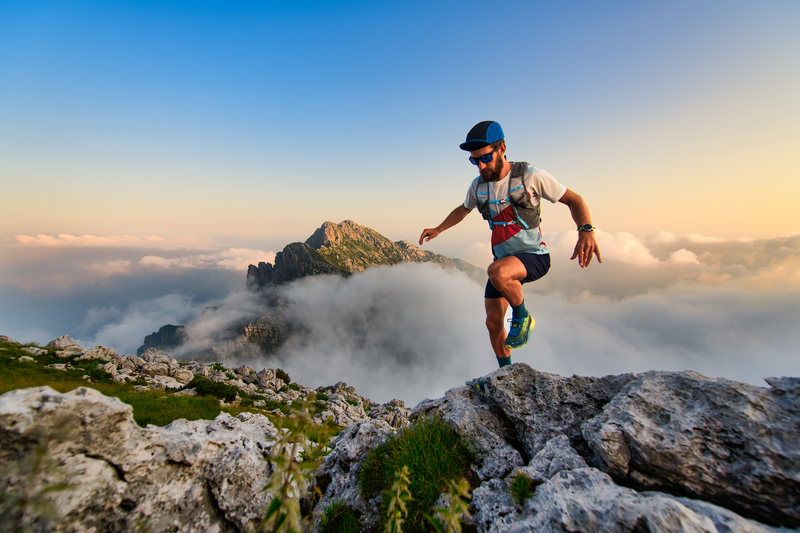
95% of researchers rate our articles as excellent or good
Learn more about the work of our research integrity team to safeguard the quality of each article we publish.
Find out more
ORIGINAL RESEARCH article
Front. Endocrinol.
Sec. Clinical Diabetes
Volume 16 - 2025 | doi: 10.3389/fendo.2025.1492082
This article is part of the Research Topic Endocrinology, Lipids, and Disease: Unraveling the Links View all 14 articles
The final, formatted version of the article will be published soon.
You have multiple emails registered with Frontiers:
Please enter your email address:
If you already have an account, please login
You don't have a Frontiers account ? You can register here
Objective: This study aims to explore the associations between various obesity and lipid-related indicators in patients with diabetes or prediabetes. Specifically, the indicators examined include the triglyceride-glucose index (TyG), along with its derived metrics: TyG-BMI, TyG-WHtR, TyG-WWI, TyG-WC, lipid accumulation product (LAP), visceral adiposity index (VAI), and abdominal obesity index (ABSI), resulting in a total of eight indicators. Methods: This study utilizes data from the NHANES conducted from 1999 to 2018, analyzing a cohort of 4,058 patients diagnosed with diabetes/prediabetes. We utilized multivariable Cox regression models to evaluate the impact of these indicators on both all-cause and cardiovascular mortality rates. Additionally, we compared the predictive performance of eight machine learning (ML) algorithms regarding mortality risk and used the SHAP method to clarify the significance of obesity and lipid-related indicators in mortality prediction.The results of the multivariable Cox regression analysis reveal significant associations between TyG, TyG-WWI, and ABSI with all-cause mortality among patients with diabetes/prediabetes. Compared to baseline levels, the HR for TyG in the fourth quartile (Q4) was 1.49, while for TyG-WWI (Q4), the HR was 1.52. Furthermore, ABSI was associated with increased all-cause mortality risk in groups Q3 and Q4, presenting risk ratios of 1.80 and 1.68, respectively. Notably, TyG (Q4) was also significantly associated with cardiovascular mortality risk, with an HR of 1.98. RCS analysis indicated a linear trend between TyG, TyG-WWI, and all-cause mortality, whereas ABSI displayed a non-linear trend. Among the ML algorithms evaluated, the XGBoost model exhibited the strongest predictive capability. The SHAP analysis indicated that the indicators with the greatest impact on all-cause mortality in patients with diabetes/prediabetes were ranked as follows: TyG > ABSI > TyG-WWI. Furthermore, sex-based subgroup analysis indicated that VAI was positively associated with cardiovascular mortality in male patients with diabetes/prediabetes, exhibiting a linear trend. Conclusion: TyG, TyG-WWI, ABSI, and VAI are closely linked to mortality risk in diabetes/prediabetes patients. Among these, TyG is significantly associated with both all-cause and cardiovascular mortality, showing superior predictive capability. We recommend long-term monitoring of these indicators and their inclusion in management strategies to effectively inform diabetes/prediabetes patients about their mortality risks.
Keywords: Obesity and lipid-related indicators, Triglyceride-glucose index, Abdominal obesity index, visceral adiposity index, Diabetes/Prediabetes, National Health and Nutrition Examination Survey; Machine Learning Algorithms
Received: 06 Sep 2024; Accepted: 07 Apr 2025.
Copyright: © 2025 Yan, Chang, Liu and Liu. This is an open-access article distributed under the terms of the Creative Commons Attribution License (CC BY). The use, distribution or reproduction in other forums is permitted, provided the original author(s) or licensor are credited and that the original publication in this journal is cited, in accordance with accepted academic practice. No use, distribution or reproduction is permitted which does not comply with these terms.
* Correspondence:
Zhiming Liu, Guang’anmen Hospital, China Academy of Chinese Medical Sciences, Beijing, China
Ruxiu Liu, Guang’anmen Hospital, China Academy of Chinese Medical Sciences, Beijing, China
Disclaimer: All claims expressed in this article are solely those of the authors and do not necessarily represent those of their affiliated organizations, or those of the publisher, the editors and the reviewers. Any product that may be evaluated in this article or claim that may be made by its manufacturer is not guaranteed or endorsed by the publisher.
Research integrity at Frontiers
Learn more about the work of our research integrity team to safeguard the quality of each article we publish.