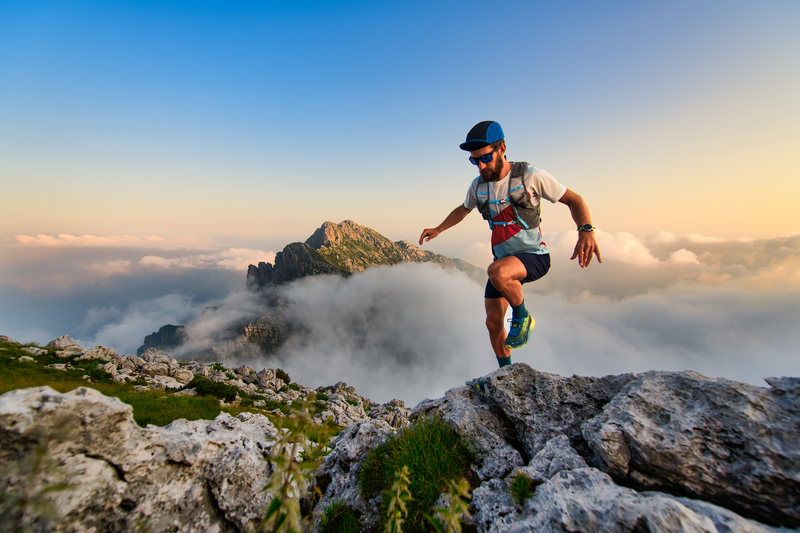
94% of researchers rate our articles as excellent or good
Learn more about the work of our research integrity team to safeguard the quality of each article we publish.
Find out more
ORIGINAL RESEARCH article
Front. Endocrinol.
Sec. Renal Endocrinology
Volume 16 - 2025 | doi: 10.3389/fendo.2025.1485477
The final, formatted version of the article will be published soon.
You have multiple emails registered with Frontiers:
Please enter your email address:
If you already have an account, please login
You don't have a Frontiers account ? You can register here
Background: Kidney stones are a prevalent disorder that is linked to a range of metabolic variables. The cardiometabolic index (CMI) is a newly developed combined measure used to evaluate the state of cardiometabolic health. Nevertheless, the correlation between CMI and kidney stone remains little investigated.In this study, cross-sectional analysis was done on NHANES data from 2007 to 2018. The relationship between the prevalence of kidney stones and the CMI was investigated using a logistic regression analysis. To look into the nonlinear link between them, we used restricted cubic spline (RCS) analysis. The study was made more reliable and accurate by using sensitivity analysis and subgroup analysis to look for factors that may have contributed to the different results.Results: A significant association was seen between increased CMI and the occurrence of kidney stones (OR = 1.19, 95% CI: 1.06-1.32). The RCS analysis revealed crucial CMI values that exhibited a robust association within a certain range. Subgroup studies revealed that this link was particularly prominent among those below the age of 50, females, obese, CKD and diabetes patients. The dependability of the study's conclusions was further established using sensitivity analysis.This study established a notable association between CMI and a higher occurrence of kidney stones, emphasizing the significance of CMI as a comprehensive measure for evaluating metabolic risk. Furthermore, it suggests that monitoring CMI levels could be beneficial in identifying populations with a high prevalence of kidney stones.
Keywords: CMI, Kidney stone, metabolic syndrome, Cross-sectional study, NHANES
Received: 02 Oct 2024; Accepted: 24 Feb 2025.
Copyright: © 2025 Zhong, Li, Tian, Jiang, Chen, Wang and Diao. This is an open-access article distributed under the terms of the Creative Commons Attribution License (CC BY). The use, distribution or reproduction in other forums is permitted, provided the original author(s) or licensor are credited and that the original publication in this journal is cited, in accordance with accepted academic practice. No use, distribution or reproduction is permitted which does not comply with these terms.
* Correspondence:
Pengfei Diao, Yuebei People's Hospital, Shaoguan, China
Disclaimer: All claims expressed in this article are solely those of the authors and do not necessarily represent those of their affiliated organizations, or those of the publisher, the editors and the reviewers. Any product that may be evaluated in this article or claim that may be made by its manufacturer is not guaranteed or endorsed by the publisher.
Research integrity at Frontiers
Learn more about the work of our research integrity team to safeguard the quality of each article we publish.