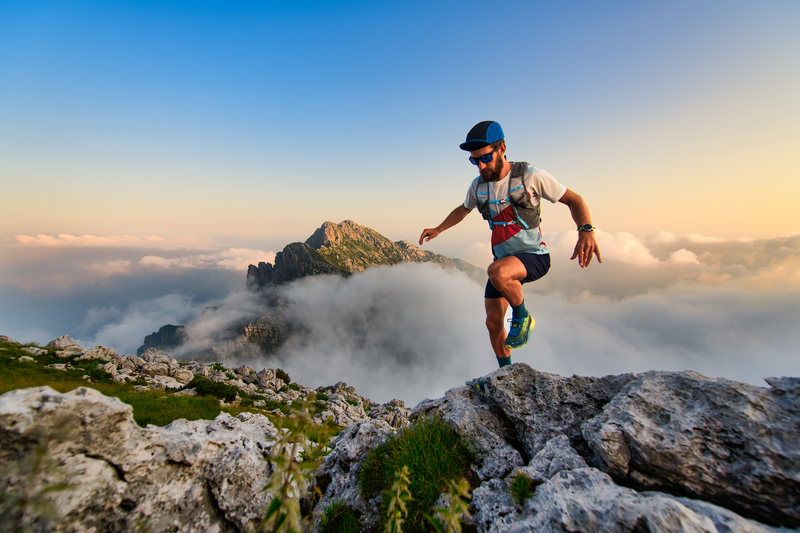
94% of researchers rate our articles as excellent or good
Learn more about the work of our research integrity team to safeguard the quality of each article we publish.
Find out more
ORIGINAL RESEARCH article
Front. Endocrinol.
Sec. Reproduction
Volume 16 - 2025 | doi: 10.3389/fendo.2025.1473228
The final, formatted version of the article will be published soon.
You have multiple emails registered with Frontiers:
Please enter your email address:
If you already have an account, please login
You don't have a Frontiers account ? You can register here
Background: A newly developed technique, Atherogenic Index of Plasma (AIP), is linked to numerous metabolic disorders. Prior researches have indicated strong correlation between AIP and waist circumference (WC), as well as between WC and infertility. Yet no investigation has examined link involving the AIP and infertility, as well as the potential mediating role of WC in this relationship.Methods: The study included 1,322 women from the 2013–2018 NHANES. Infertility was the outcome variable. Moreover, mediation analysis explored the mediating role of WC in the above relationships.Results: There were 1,163 controls and 159 infertile participants among the 1,322 participants. The study demonstrated increased WC and elevated AIP among infertile women. Also, the AIP demonstrated an independent correlation with a higher likelihood of infertility, regardless of adjustments for confounding factors. Subgroup analysis indicated the AIP was related to the prevalence of infertility even among women aged 35 years or younger with no history of cardiovascular disease (CVD), pelvic infections, or use of female hormones. Finally, WC had a substantial mediating effect on correlation between AIP and infertility, accounting for 54.49% of the association. Yet, it appears that the various IR surrogates did not demonstrate variability in their predictive ability for infertility [AIP: 0.642 (95% CI: 0.599, 0.683) vs. WC 0.658 (95% CI: 0.618, 0.705) vs. HOMA-IR 0.637 (95% CI: 0.593, 0.686)].Conclusion: A notable positive correlation exists between AIP and female infertility. It provides the first evidence to demonstrate the mediating role of WC in the above relationship. Managing abdominal obesity and monitoring AIP levels may contribute to reduce the likelihood of infertility.
Keywords: Atherogenic index of plasma1, infertility2, NHANES3, Waist circumference 4, crosssectional study5
Received: 30 Jul 2024; Accepted: 03 Mar 2025.
Copyright: © 2025 Wei and Dan Dan. This is an open-access article distributed under the terms of the Creative Commons Attribution License (CC BY). The use, distribution or reproduction in other forums is permitted, provided the original author(s) or licensor are credited and that the original publication in this journal is cited, in accordance with accepted academic practice. No use, distribution or reproduction is permitted which does not comply with these terms.
* Correspondence:
Liu Dan Dan, The Eighth Affiliated Hospital, Sun Yat-sen University, Shenzhen, 518033, China
Disclaimer: All claims expressed in this article are solely those of the authors and do not necessarily represent those of their affiliated organizations, or those of the publisher, the editors and the reviewers. Any product that may be evaluated in this article or claim that may be made by its manufacturer is not guaranteed or endorsed by the publisher.
Research integrity at Frontiers
Learn more about the work of our research integrity team to safeguard the quality of each article we publish.