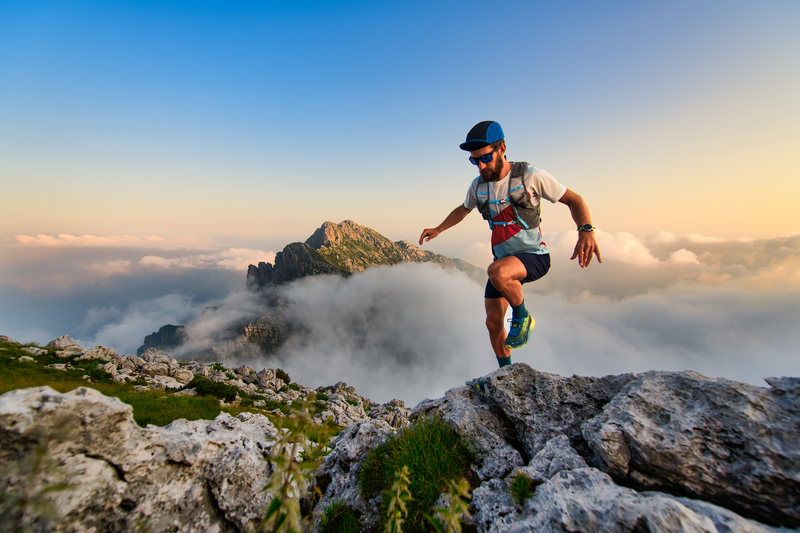
95% of researchers rate our articles as excellent or good
Learn more about the work of our research integrity team to safeguard the quality of each article we publish.
Find out more
ORIGINAL RESEARCH article
Front. Endocrinol. , 04 March 2025
Sec. Cardiovascular Endocrinology
Volume 16 - 2025 | https://doi.org/10.3389/fendo.2025.1467886
This article is part of the Research Topic Cardiovascular Diseases Related to Diabetes and Obesity - Volume V View all 22 articles
Background: While numerous epidemiological studies on body fat and diabetes already exist, there remains a scarcity of evidence regarding gender differences within hypertensive populations. The aim of this study was to examine gender-specific differences in the association of body fat percentage (BFP) with diabetes.
Methods and results: This cross-sectional study encompassed 14,228 hypertensive patients from the Chinese Hypertension Registry. An easily obtainable anthropometric parameter, Clínica University de Navarra-Body Adiposity Estimator (CUN-BAE) equation was used to calculate body fat percentage (BFP). Diabetes was defined as the self-report of a previous diagnosis of diabetes, fasting blood glucose ≥ 7.0mmol/l, and the use of antidiabetic agents. The average BFP was 24.5% in men and 37.0% in women. Multivariate logistic regression analysis revealed a dose-dependent relationship between BFP and the risk of diabetes in men (odds ratio [OR] 1.09, 95% CI 1.07, 1.11) and women (OR 1.06, 95% CI 1.04, 1.07) while considering BFP as a continuous variable. After taking BFP as the quartile across different genders, compared with Q1 group, the risk of diabetes in Q4 group increased 176% (OR 2.76, 95% CI 2.15, 3.55) in men and 66% (OR 1.66, 95% CI 1.36, 2.03) in women. Furthermore, the positive association was found to be more significant in men, whether BFP was considered a continuous variable (P for interaction = 0.016) or a categorical variable in quartiles (P for interaction = 0.008). In addition, the positive association between BFP and diabetes remained consistent across various subgroups.
Conclusion: BFP is positively associated with the increased risk of diabetes in hypertensive population, especially in men.
Approximately 11.9% of Chinese adults are affected by diabetes, and this number is steadily increasing. However, in China, only 38.0% of diabetes cases are properly recognized, with 34.1% receiving treatment and 33.1% having their blood glucose levels effectively controlled (1). Hyperglycemia is recognized for triggering various vascular complications and increasing oxidative stress through multiple pathways (2). Atherosclerosis, a leading cause of mortality in diabetes patients, is primarily associated with natural and modified lipids (3–5). Furthermore, obesity, characterized by an excessive proportion of body fat, is closely linked to the risk of diabetes (6, 7). Research conducted about 20 years ago underscored body mass index (BMI) as an independent predictor of diabetes (8). Bariatric surgery has been identified as a promising method for achieving diabetes remission (9). In conclusion, obesity not only poses a significant risk for diabetes and cardiovascular disease but also contributes to premature mortality and disability in the population at large (10–12).
To accurately assess the complexity of obesity, there is a pressing need for more precise indicators. Although the BMI is widely utilized and easily accessible, its simplicity hinders its ability to capture the multifaceted nature of obesity. BMI relies solely on height and weight measurements, failing to differentiate between fat and muscle mass or account for the distribution of body fat. Recognizing the limitations of BMI, recent findings have challenged its effectiveness in predicting health risks, such as diabetes (13, 14). Research by Lu et al., involving 5860 Chinese participants, revealed that increased BMI alone, without abdominal obesity, did not significantly correlate with the risk of type 2 diabetes. Conversely, individuals with abdominal obesity experienced a 55% increase in the incidence of type 2 diabetes (15). Such limitations have prompted the exploration of alternative obesity indicators. Given the crucial role of fat accumulation in the pathogenesis of diabetes, it is imperative to explore a new fat index. Notably, body fat percentage (BFP) has demonstrated greater accuracy than BMI in measuring obesity, particularly central obesity, and has shown improved predictive capabilities for certain diseases (16, 17).
Previous studies have explored the relationship between BFP and diabetes risk under different backgrounds (18, 19). However, whether the relationship between them in patients with hypertension is the same as that of other populations is still rarely explored. Given the significant differences in BFP levels between men and women due to variations in sex hormones and body fat distribution, the association between BFP and diabetes has seldom been investigated across different genders. Therefore, we conducted a cross-sectional study to explore the association between BFP and diabetes risk and the gender differences in hypertensive population.
The protocol was obtained from the China H-type Hypertension Registry Study (registration number: ChiCTR1800017274). The trial design and methods have been previously introduced. This is a real-world observational study that was registered in August 2018 in China. Eligible subjects were hypertensive patients aged 18 and over. The exclusion criteria for this study include the following: (1) Inability to provide informed consent due to psychological or nervous system damage. (2) Inability to comply with the study’s follow-up protocol or planning to relocate in the near future. (3) Patients evaluated by the investigator who were deemed unsuitable for inclusion or long-term follow-up. All participants provided written informed consent. But since our investigation is mainly conducted in rural areas, the majority of participants are aged between 50-70 years old (Table 1).
Table 1. Clinical characteristics of the study population according to body fat percentage among men and women.
This study was conducted in accordance with the Declaration of Helsinki and approved by the Ethics Committee of the Institute of Biomedical Sciences, Anhui Medical University (Ethics NO. CH1059), and the Second Affiliated Hospital of Nanchang University (Ethics NO. 2018019). All patients provided signed informed consent before participating in this study.
A total of 14234 patients with hypertension met the inclusion and exclusion criteria. After excluding patients with missing BFP data (n=5) and abnormal BMI values (n=1), 14228 subjects were included in the final analysis (Figure 1)
Demographic characteristics (gender and age), lifestyle variances (smoking status, drinking status, and physical activity), and medical history (diabetes, heart failure, hyperlipidemia, hypertension, and medication use) were gathered by trained specialized researchers. Blood pressure (BP) was measured using automatic electronic equipment (Omron; Dalian, China). Following a 10-minute rest, both systolic blood pressure (SBP) and diastolic blood pressure (DBP) were recorded, and the average values from three blood pressure measurements were documented.
Fasting venous blood samples were collected from all subjects. Subsequently, the blood samples were frozen and dispatched to the Shenzhen Biaojia Biotechnology Laboratory for analysis. Fasting blood glucose (FPG) and fasting blood lipids, including total cholesterol (TC), high-density lipoprotein cholesterol (HDL-C), low-density lipoprotein cholesterol (LDL-C), and triglycerides (TG), were analyzed using the Beckman Coulter automatic clinical analyzer. The homocysteine (Hcy) was measured using automatic clinical analyzers (Beckman Coulter, USA). The glomerular filtration rate (eGFR) was estimated using the Chronic Kidney Disease Epidemiology Collaboration (CKD-EPI) equation.
The height, weight, and waist circumference (WC) of the study subjects were measured by trained researchers using standardized equipment. BMI was calculated by dividing the weight (in kilograms) by the square of the height (in meters). BFP was estimated using the Clínica University de Navarra-Body Adiposity Estimator (CUN-BAE) equation: BFP = -44.988 + (0.503 × age) + (10.689 × sex) + (3.172 × BMI) - (0.026 × BMI2) + (0.181 × BMI × sex) - (0.02 × BMI × age) - (0.005 × BMI2 × sex) + (0.00021 × BMI2 × age) (20). In this equation, a male gender is represented as 0, while a female gender is represented as 1. This formula has been utilized in various clinical research studies.
Since the recommended reference range of BFP for the Chinese population is currently unavailable, we will categorize the research subjects based on gender. They were divided into four groups according to BFP quartiles for each gender. For men, the BFP quartiles were defined as follows: below 21.7% (quartile 1), 21.7% - 24.5% (quartile 2), 24.5% - 27.2% (quartile 3), and 27.2% and above (quartile 4). In women, the BFP quartiles were: below 34.3% (quartile 1), 34.3% - 37.0% (quartile 2), 37.0% - 39.9% (quartile 3), and 39.9% and above (quartile 4)
We used the Beckman Coulter automatic clinical analyzer to measure the subjects’ fasting blood glucose level. Diabetes in this study was defined as self-reported previous diagnosis of diabetes or fasting blood glucose ≥ 7.0 mmol/L and use of glucose-lowering drugs.
For normally distributed continuous variables, data are typically presented as mean ± standard deviation (SD). In contrast, for continuous variables with a skewed distribution, data are usually presented as median (25th-75th percentile), while categorical variables are often depicted as count (%). In this study, a descriptive analysis was conducted based on the quartiles of BFP. The differences between gender groups were compared using either an ANOVA test or a chi-square test. The dose-response relationship between BFP and the risk of diabetes was assessed using a generalized additive model (GAM) and adjusted penalized spline method. A Multivariate logistic regression model, including odds ratio (OR) and 95%CI confidence interval, was utilized to control for the main covariates across three models and establish an independent association between BFP and diabetes risk. The models were as follows: Model 1: Crude model; Model 2: adjusted for age, physical activity, current smoking, and current drinking; Model 3: adjusted for age, SBP, DBP, current smoking, current drinking, physical activity, Hcy, TG, HDL-C, LDL-C, and eGFR, stroke, coronary heart disease (CHD), antihypertensive drugs, lipoprotein-lowering drugs. Furthermore, the potential interaction between BFP and diabetes was examined through stratified analysis and an interaction test.
All data analyses were conducted using the statistical package R (http://www.r-project.org) and Empower(R) (www.empowerstats.com; X&Y Solutions, Inc., Boston, MA). A two-tailed P < 0.05 was considered statistically significant.
A total of 6,719 male and 7,509 female hypertensive patients were included in the final analysis. The findings presented in Table 1 reveal that men with higher BFP tend to be younger, non-smokers, have elevated levels of DBP, TC, TG, LDL-C, a higher prevalence of diabetes, and lower levels of HDL-C. Conversely, there was no significant statistical difference observed in current drinking habits and levels of SBP and eGFR. On the other hand, among women, the results slightly differ, as individuals with higher BFP are older and have lower levels of eGFR.
As shown in Figure 2, BFP was significantly positively associated with the risk of diabetes for both men and women. Table 2 indicates that there is a dose-dependent relationship between BFP and diabetes risk. Regardless of whether the confounding factors were adjusted for, BFP of different genders was positively associated with the risk of diabetes (P <0.001). With BFP as the continuous variable, the risk of diabetes increased by 9% in the fully adjusted model in men (OR 1.09, 95% CI 1.07, 1.11), and the risk increased by 6% (OR 1.06, 95% CI 1.04, 1.07) in women. BFP was further divided into quartiles, in the men fully adjusted model, compared with Q1 (<21.7%), the risk of diabetes increased by 51% (OR 1.51, 95% CI 1.17, 1.94) in Q2 (21.7% -24.5%), 94% (OR 1.94, 95% CI 1.51, 2.49) in Q3 (24.5% -27.2%) and 176% (OR 2.76, 95% CI 2.15, 3.55) in Q4 (≥ 27.2%). In the fully adjusted women quartile model, compared with Q1 (<34.3%), there was no significant difference in the risk of diabetes in Q2 (34.3% -37.0%) group (OR 1.20, 95% CI 0.98, 1.47), while the risk in Q3 (37.0% -39.9%) increased by 57% (OR 1.57, 95% CI 1.29, 1.91), and 66% (OR 1.66, 95% CI 1.36, 2.03) in Q4 (≥ 39.9%). Although the positive association between BFP and diabetes is stable, there is still gender difference. Whether using BFP as a continuous variable (P = 0.016 for interaction) or a categorical variable in the quartile (P = 0.008 for interaction).
Figure 2. Dose-response relationship between body fat percentage and diabetes among men (A) and women (B). Models were adjusted for age, SBP, DBP, current smoking, current drinking, physical activity, Hcy, TG, HDL-C, LDL-C, and eGFR. stroke, CHD, antihypertensive agents, lipid-lowering agents.
We conducted an analysis to investigate the relationship between BFP and diabetes within various subgroups (Figure 3), including age, BMI, SBP, current smoking, current drinking, LDL-C, Hcy, and eGFR. Our findings revealed a consistent positive association between BFP and diabetes across all stratified subgroups among women (with interaction P value > 0.05). Although the positive association between BFP and diabetes risk remained constant in men, it was more pronounced in individuals with lower LDL-C (OR 1.14, 95% CI 1.10, 1.17) levels compared to those with higher LDL-C (OR 1.07, 95% CI 1.05, 1.10) levels (P for interaction = 0.004).
Figure 3. Subgroup analyses of the effect of BFP on diabetes among men (A) and women (B). Each subgroup analysis adjusted, if not stratified, for age, SBP, DBP, current smoking, current drinking, physical activity, Hcy, TG, HDL-C, LDL-C, and eGFR. stroke, CHD, antihypertensive agents, lipid-lowering agents.
This extensive cross-sectional study, encompassing a substantial sample size of 14,228 participants from the hypertensive population in southern China, revealed there is a positive association between BFP and diabetes. Even after adjusting for potential confounding variables, the association remained statistically significant. Notably, in men, this positive association was more pronounced. Additionally, the positive association between BFP and diabetes was consistent across all subgroups of women, and there is a more significant positive association between the two in men with LDL-C<2.6mmol/L.
There have been numerous speculations and reports on the pathogenesis of obesity and diabetes. As early as 2001, researchers discovered that adipocytes secrete resistin, which initially shed light on the link between obesity and insulin resistance (21). This discovery has since catalyzed the emergence of various research directions and disciplines, delving into mechanisms that lead to adipose tissue inflammation. These investigations include examining the role of adaptive immune cells and the activation and phenotype transformation of macrophages, all of which may contribute to insulin resistance (22).
The accumulation of fat is an important link in the physical damage caused by obesity. BFP has been preliminarily explored as an emerging indicator for evaluating body fat. Previous studies have investigated the connection between BFP and diabetes in various populations. Chen et al.’s study on 3367 Chinese subjects demonstrated a positive association between BFP and diabetes, highlighting BFP as an independent risk factor and a valuable diagnostic indicator for type 2 diabetes (23). Similarly, Peña J et al. found in a study involving 1920 Mexican participants that BFP is a key risk factor for type 2 diabetes, surpassing BMI in predictive value (24). Moreover, a prospective study with 1532 participants revealed a significant positive association between male trunk fat percentage and diabetes incidence, while the association with female trunk fat percentage, although positive, was not statistically significant (25). But as is well known that hypertension is an independent risk factor for diabetes often co-existing with the condition (26), is linked to insulin resistance in hypertensive individuals and serves as a confounding variable associated with overweight and obesity (27–29). High blood pressure can increase the risk of vascular complications in diabetic patients that emphasizing the importance of identifying high-risk hypertensive individuals with diabetes for prevention and management (30, 31). While previous studies have explored various other anthropometric indices for predicting diabetes in hypertensive populations (32), but our study uniquely focuses on the relationship between BFP and diabetes among hypertensive patients and the gender differences within it.
In the elderly population, a distinct gender-specific pattern in BFP distribution is observed. Younger male individuals tend to exhibit higher BFP values, whereas older female individuals demonstrate an opposite trend. This phenomenon can be attributed to age-related hormonal changes: in aging males, declining testosterone levels contribute to alterations in fat distribution patterns and progressive loss of muscle mass, consequently leading to reduced body fat percentage. Conversely, postmenopausal women experience significant estrogen depletion, which promotes visceral fat accumulation in abdominal regions, ultimately resulting in increased BFP. Our research also indicates a stronger positive association between BFP and diabetes in men compared to women. This disparity may stem from differences in male and female hormones or variations in fat distribution (33). Men are more prone to abdominal obesity and higher levels of visceral fat, while women typically accumulate fat in the buttocks and thighs, with a greater proportion of subcutaneous fat (34). Consequently, weight gain in men often results from increased visceral fat, which may significantly elevate the risk of diabetes. Research has found that fat in gluteus–femoral area in overweight and obese women has a protective effect on glucose and lipid related cardiac metabolic risks. Compared with subjects with widespread distribution of visceral fat, hip and thigh fat are associated with a beneficial fat factor profile and fewer pro-inflammatory molecules (35, 36). This distribution may offer protective effects against glucose and fat-related cardiac metabolic diseases (37). The study also confirmed that people with type 2 diabetes respond less to obesity treatment than those without type 2 diabetes, especially males. Women respond better to treatment than men (38). This could explain the heightened association between BFP and diabetes in men. Our findings also suggest that in men with lower LDL-C levels, the positive association between BFP and diabetes is more pronounced. Traditional treatments often involve lowering LDL-C to less than 2.6 mmol/L, or even lower, particularly for patients with known cardiovascular diseases or at high risk (39). However, intensive statin therapy may elevate the risk of developing diabetes (40, 41). Studies propose that patients with lower LDL-C levels may become complacent, adopt poorer lifestyles, gain weight, and subsequently develop diabetes. Additionally, impaired insulin secretion and intensified insulin resistance may contribute to this phenomenon (42).
This cross-sectional study, offers insights into the gender differences within the association between BFP and diabetes among hypertensive patients that less-explored. The study’s emphasis on a homogeneous population of hypertensive patients enhances result reliability, and detailed subgroup analyses contribute to result stability. However, this article also has certain limitations. Dual energy X-ray absorption or bioelectrical impedance analysis is considered the gold standard for measuring BFP (43). The formula we use to calculate BFP has been applied in multiple articles and is easier to obtain in clinical practice. But it still cannot achieve the same status as the gold standard (44). And the hypertensive participants enrolled in our study were not stratified according to the etiology of hypertension, including both primary and secondary hypertension cases. Secondly, this study is a cross-sectional study, which means that the results could not establish a causal relationship between BFP and diabetes. Despite performing multivariate corrections, it remains challenging to eliminate potential confounding factors. And we did not gather more detailed information about subjects, such as dietary structure. As a result, we cannot ascertain whether these factors have a regulatory effect on the association between BFP and the risk of diabetes. Lastly, this study focuses solely on the hypertensive population in southern China, raising uncertainties about the generalizability of the conclusions to other populations.
BFP shows a positive association with the risk of diabetes among hypertensive patients in southern China. This association is notably stronger in men compared to women. It is recommended that men should pay more attention to changes in BFP than women. Further longitudinal studies are required to unveil the underlying mechanisms and establish a causal relationship between the two variables.
The raw data supporting the conclusions of this article will be made available by the authors, without undue reservation.
The studies involving humans were approved by Ethics Committee of the Institute of Biomedical Sciences, Anhui Medical University (Ethics NO. CH1059), and the Second Affiliated Hospital of Nanchang University (Ethics NO. 2018019). The studies were conducted in accordance with the local legislation and institutional requirements. The participants provided their written informed consent to participate in this study.
JR: Conceptualization, Investigation, Software, Writing – original draft, Writing – review & editing. CD: Methodology, Writing – review & editing. YS: Writing – original draft. WZ: Data curation, Methodology, Software, Supervision, Writing – review & editing. CY: Data curation, Methodology, Software, Supervision, Writing – review & editing. TW: Data curation, Methodology, Software, Supervision, Writing – review & editing. LZ: Data curation, Methodology, Supervision, Writing – review & editing. XH: Data curation, Methodology, Software, Supervision, Writing – review & editing. HB: Data curation, Funding acquisition, Methodology, Project administration, Supervision, Writing – review & editing. XC: Data curation, Funding acquisition, Methodology, Project administration, Supervision, Writing – review & editing.
The author(s) declare financial support was received for the research, authorship, and/or publication of this article. This work was supported by the Cultivation of backup projects for National Science and Technology Awards (20223AEI91007), Jiangxi Science and Technology Innovation Base Plan -Jiangxi Clinical Medical Research Center (20223BCG74012, 20224BAB206090), Science and Technology Innovation Base Construction Project (20221ZDG02010), Fund project of the Second Affiliated Hospital of Nanchang University (2021efyA01, 2023efyA05).
We appreciate the contributions of all the staff involved in this study, as well as the research participants who have dedicated their time to collaborate with us.
The authors declare that the research was conducted in the absence of any commercial or financial relationships that could be construed as a potential conflict of interest.
All claims expressed in this article are solely those of the authors and do not necessarily represent those of their affiliated organizations, or those of the publisher, the editors and the reviewers. Any product that may be evaluated in this article, or claim that may be made by its manufacturer, is not guaranteed or endorsed by the publisher.
1. Chinese Diabetes Society, National Office for Primary Diabetes Care. National guidelines for the prevention and control of diabetes in primary care (2022). Zhonghua Nei Ke Za Zhi. (2022) 61:249–62. doi: 10.3760/cma.j.cn112138-20220120-000063
2. Mazzone T, Chait A, Plutzky J. Cardiovascular disease risk in type 2 diabetes mellitus: insights from mechanistic studies. Lancet. (2008) 371:1800–9. doi: 10.1016/S0140-6736(08)60768-0
3. Langberg R. Diabetes Mellitus Mortality in the United States, 1950-67. Vital Health Stat. (1971) 20(10):1–48.
4. Balodimos MC. Joslin’s Diabetes Mellitus. 11th ed. Marble A, White P, Bradley RF, Krall LP, editors. Philadelohia: Lea & Febiaer (1971).
5. Lee CK, Liao CW, Meng SW, Wu WK, Chiang JY, Wu MS. Lipids and lipoproteins in health and disease: focus on targeting atherosclerosis. Biomedicines. (2021) 9:985. doi: 10.3390/biomedicines9080985
6. Maggio CA, Pi-Sunyer FX. Obesity and type 2 diabetes. Endocrinol Metab Clin North Am. (2003) 32:805–22. doi: 10.1016/s0889-8529(03)00071-9
7. Klein S, Gastaldelli A, Yki-Järvinen H, Scherer PE. Why does obesity cause diabetes? Cell Metab. (2022) 34:11–20. doi: 10.1016/j.cmet.2021.12.012
8. Weinstein AR, Sesso HD, Lee IM, Cook NR, Manson JE, Buring JE, et al. Relationship of physical activity vs body mass index with type 2 diabetes in women. JAMA. (2004) 292:1188–94. doi: 10.1001/jama.292.10.1188
9. Ding L, Fan Y, Li H, Zhang Y, Qi D, Tang S, et al. Comparative effectiveness of bariatric surgeries in patients with obesity and type 2 diabetes mellitus: A network meta-analysis of randomized controlled trials. Obes Rev. (2020) 21:e13030. doi: 10.1111/obr.13030
10. World Health Organization. Noncommunicable diseases progress monitor, 2017. Geneva: WHO (2017). Available at: https://www.who.int/nmh/publications/ncd-progress-monitor-2017/en/.
11. Bray GA, Kim KK, Wilding JPH, World Obesity Federation. Obesity: a chronic relapsing progressive disease process. A position statement of the World Obesity Federation. Obes Rev. (2017) 18:715–23. doi: 10.1111/obr.12551
12. Blüher M. Obesity: global epidemiology and pathogenesis. Nat Rev Endocrinol. (2019) 15:288–98. doi: 10.1038/s41574-019-0176-8
13. Sturm R, An R. Obesity and economic environments. CA: Cancer J Clin. (2014) 64:337–50. doi: 10.3322/caac.21237
14. Expert Panel on Detection, Evaluation, and Treatment of High Blood Cholesterol in Adults. Executive summary of the third report of the national cholesterol education program (NCEP) expert panel on detection, evaluation, and treatment of high blood cholesterol in adults (Adult treatment panel III). JAMA. (2001) 285:2486–97. doi: 10.1001/jama.285.19.2486
15. Lu Y, Yang H, Xu Z, Tang X. Association between different obesity patterns and the risk of developing type 2 diabetes mellitus among adults in eastern China: A cross-sectional study. Diabetes Metab Syndr Obes. (2021) 14:2631–9. doi: 10.2147/DMSO.S309400
16. Lavie CJ, De Schutter A, Patel DA, Romero-Corral A, Artham SM, Milani RV. Body composition and survival in stable coronary heart disease: impact of lean mass index and body fat in the “obesity paradox. J Am Coll Cardiol. (2012) 60:1374–80. doi: 10.1016/j.jacc.2012.05.037
17. Hayes AJ, Larkin J. BMI and outcomes in melanoma: more evidence for the obesity paradox. Lancet Oncol. (2018) 19:269–70. doi: 10.1016/S1470-2045(18)30077-9
18. Kang N, Chen G, Tu R, Liao W, Liu X, Dong X, et al. Adverse associations of different obesity measures and the interactions with long-term exposure to air pollutants with prevalent type 2 diabetes mellitus: The Henan Rural Cohort study. Environ Res. (2022) 207:112640. doi: 10.1016/j.envres.2021.112640
19. Compeán-Ortiz LG, Trujillo-Olivera LE, Valles-Medina AM, Reséndiz-González E, García-Solano B, Pérez BDA. Obesity, physical activity and prediabetes in adult children of people with diabetes. Rev Lat Am Enfermagem. (2018) 25:e2981. doi: 10.1590/1518-8345.2102.2981
20. Xiong Y, Wangsheng F, Wang S, Zhou W, Huang X, Bao H, et al. Positive association between body fat percentage and hyperuricemia in patients with hypertension: The China H-type hypertension registry study. Nutr Metab Cardiovasc Dis. (2021) 31:3076–84. doi: 10.1016/j.numecd.2021.07.002
21. Steppan CM, Bailey ST, Bhat S, Brown EJ, Banerjee RR, Wright CM, et al. The hormone resistin links obesity to diabetes. Nature. (2001) 409:307–12. doi: 10.1038/35053000
22. Sell H, Habich C, Eckel J. Adaptive immunity in obesity and insulin resistance. Nat Rev Endocrinol. (2012) 8:709–16. doi: 10.1038/nrendo.2012.114
23. Chen Y, He D, Yang T, Zhou H, Xiang S, Shen L, et al. Relationship between body composition indicators and risk of type 2 diabetes mellitus in Chinese adults. BMC Public Health. (2020) 20:452. doi: 10.1186/s12889-020-08552-5
24. Escobedo-de-la-Peña J, Ramírez-Hernández JA, Fernández-Ramos MT, González-Figueroa E, Champagne B. Body fat percentage rather than body mass index related to the high occurrence of type 2 diabetes. Arch Med Res. (2020) 51:564–71. doi: 10.1016/j.arcmed.2020.05.010
25. Tatsukawa Y, Misumi M, Kim YM, Yamada M, Ohishi W, Fujiwara S, et al. Body composition and development of diabetes: a 15-year follow-up study in a Japanese population. Eur J Clin Nutr. (2018) 72:374–80. doi: 10.1038/s41430-017-0077-7
26. Bress AP, King JB, Kreider KE, Beddhu S, Simmons DL, Cheung AK, et al. Effect of intensive versus standard blood pressure treatment according to baseline prediabetes status: a post hoc analysis of a randomized trial. Diabetes Care. (2017) 40:1401–8. doi: 10.2337/dc17-0885
27. Petrie JR, Guzik TJ, Touyz RM. Diabetes, hypertension, and cardiovascular disease: clinical insights and vascular mechanisms. Can J Cardiol. (2018) 34:575–84. doi: 10.1016/j.cjca.2017.12.005
28. Wang S, Ma W, Yuan Z, Wang SM, Yi X, Jia H, et al. Association between obesity indices and type 2 diabetes mellitus among middle-aged and elderly people in Jinan, China: a cross-sectional study. BMJ Open. (2016) 6:e012742. doi: 10.1136/bmjopen-2016-012742
29. Mokdad AH, Ford ES, Bowman BA, Dietz WH, Vinicor F, Bales VS, et al. Prevalence of obesity, diabetes, and obesity-related health risk factors, 2001. JAMA. (2003) 289:76–9. doi: 10.1001/jama.289.1.76
30. Salman RA, Al-Rubeaan KA. Incidence and risk factors of hypertension among Saudi type 2 diabetes adult patients: an 11-year prospective randomized study. J Diabetes Complications. (2009) 23:95–101. doi: 10.1016/j.jdiacomp.2007.10.004
31. Anderson RJ, Bahn GD, Moritz TE, Kaufman D, Abraira C, Duckworth W. Blood pressure and cardiovascular disease risk in the veterans affairs diabetes trial. Diabetes Care. (2011) 34:34–8. doi: 10.2337/dc10-1420
32. Liu Y, Liu X, Guan H, Zhang S, Zhu Q, Fu X, et al. Body roundness index is a superior obesity index in predicting diabetes risk among hypertensive patients: A prospective cohort study in China. Front Cardiovasc Med. (2021) 8:736073. doi: 10.3389/fcvm.2021.736073
33. Gado M, Tsaousidou E, Bornstein SR, Perakakis N. Sex-based differences in insulin resistance. J Endocrinol. (2024) 261(1). doi: 10.1530/JOE-23-0245
34. Miljkovic-Gacic I, Wang X, Kammerer CM, Bunker CH, Patrick AL, Wheeler VW, et al. Sex and genetic effects on upper and lower body fat and associations with diabetes in multigenerational families of African heritage. Metabolism. (2008) 57:819–23. doi: 10.1016/j.metabol.2008.01.022
35. Manolopoulos KN, Karpe F, Frayn KN. Gluteofemoral body fat as a determinant of metabolic health. Int J Obes. (2010) 34:949–59. doi: 10.1038/ijo.2009.286
36. Borges MC, Oliveira IO, Freitas DF, Horta BL, Ong KK, Gigante DP, et al. Obesity-induced hypoadiponectinaemia: the opposite influences of central and peripheral fat compartments. Int J Epidemiol. (2017) 46:2044–55. doi: 10.1093/ije/dyx022
37. Karastergiou K, Smith SR, Greenberg AS, Fried SK. Sex differences in human adipose tissues - the biology of pear shape. Biol Sex Differences. (2012) 3:13. doi: 10.1186/2042-6410-3-13
38. Jensterle M, Rizzo M, Haluzik M, Janez A. Efficacy of GLP-1 RA approved for weight management in patients with or without diabetes: A narrative review. Adv Ther. (2022) 39:2452–67. doi: 10.1007/s12325-022-02153-x
39. Grundy SM, Cleeman JI, Merz CN, Brewer HB Jr., Clark LT, Hunninghake DB, et al. Implications of recent clinical trials for the National Cholesterol Education Program Adult Treatment Panel III Guidelines. J Am Coll Cardiol. (2004) 44:720–32. doi: 10.1016/j.jacc.2004.07.001
40. Preiss D, Seshasai SR, Welsh P, Murphy SA, Ho JE, Waters DD, et al. Risk of incident diabetes with intensive-dose compared with moderate-dose statin therapy: a meta-analysis. JAMA: J Am Med Assoc. (2011) 305:2556–64. doi: 10.1001/jama.2011.860
41. Cholesterol Treatment Trialists’ (CTT) Collaboration, Cholesterol Treatment Trialists’ (CTT) Collaboration. Effects of statin therapy on diagnoses of new-onset diabetes and worsening glycaemia in large-scale randomised blinded statin trials: an individual participant data meta-analysis. Lancet Diabetes Endocrinol. (2024) 305(24):2556–64. doi: 10.1016/S2213-8587(24)00040-8
42. Cai R, Yuan Y, Zhou Y, Xia W, Wang P, Sun H, et al. Lower intensified target LDL-c level of statin therapy results in a higher risk of incident diabetes: a meta-analysis. PloS One. (2014) 9:e104922. doi: 10.1371/journal.pone.0104922
43. Gallagher D, Heymsfield SB, Heo M, Jebb SA, Murgatroyd PR, Sakamoto Y. Healthy percentage body fat ranges: an approach for developing guidelines based on body mass index. Am J Clin Nutr. (2000) 72:694–701. doi: 10.1093/ajcn/72.3.694
Keywords: body fat percentage, diabetes, gender difference, hypertension, cohort study
Citation: Rao J, Ding C, Shi Y, Zhou W, Yu C, Wang T, Zhu L, Huang X, Bao H and Cheng X (2025) Association of body fat percentage with diabetes in hypertensive adults of different genders: a cross-sectional study. Front. Endocrinol. 16:1467886. doi: 10.3389/fendo.2025.1467886
Received: 21 July 2024; Accepted: 10 February 2025;
Published: 04 March 2025.
Edited by:
Gaetano Santulli, Albert Einstein College of Medicine, United StatesReviewed by:
Aleksandra Zdrojowy-Wełna, Wroclaw Medical University, PolandCopyright © 2025 Rao, Ding, Shi, Zhou, Yu, Wang, Zhu, Huang, Bao and Cheng. This is an open-access article distributed under the terms of the Creative Commons Attribution License (CC BY). The use, distribution or reproduction in other forums is permitted, provided the original author(s) and the copyright owner(s) are credited and that the original publication in this journal is cited, in accordance with accepted academic practice. No use, distribution or reproduction is permitted which does not comply with these terms.
*Correspondence: Xiaoshu Cheng, eGlhb3NodW1lbmZhbjEyNkAxNjMuY29t; Huihui Bao, aHVpaHVpX2Jhbzc3QDEyNi5jb20=
Disclaimer: All claims expressed in this article are solely those of the authors and do not necessarily represent those of their affiliated organizations, or those of the publisher, the editors and the reviewers. Any product that may be evaluated in this article or claim that may be made by its manufacturer is not guaranteed or endorsed by the publisher.
Research integrity at Frontiers
Learn more about the work of our research integrity team to safeguard the quality of each article we publish.