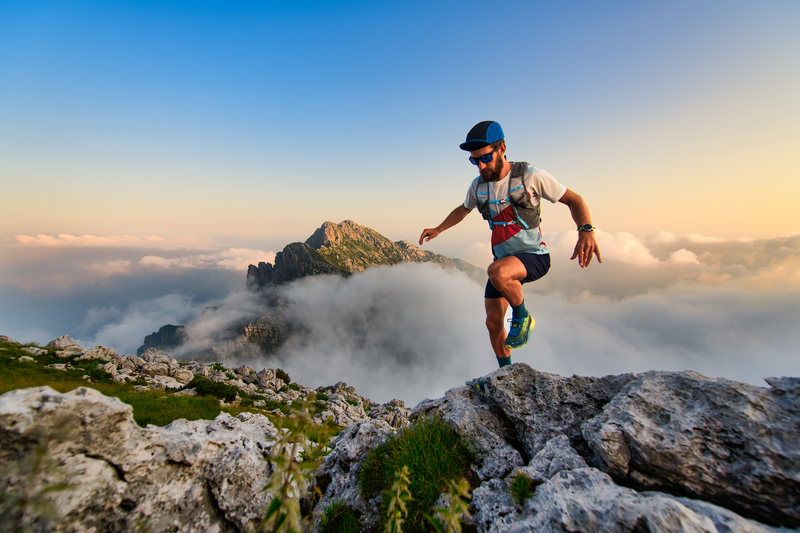
95% of researchers rate our articles as excellent or good
Learn more about the work of our research integrity team to safeguard the quality of each article we publish.
Find out more
ORIGINAL RESEARCH article
Front. Endocrinol. , 18 March 2025
Sec. Systems Endocrinology
Volume 16 - 2025 | https://doi.org/10.3389/fendo.2025.1416993
Objective: Skin fibrosis is a dermal lesion associated with inflammatory factors. However, the exact causal relationship between circulating inflammatory proteins (CIPs) and skin fibrosis remains unclear. To investigate this potential association and mediated effect, Mendelian randomization (MR) and two-step MR were used.
Methods: Summary statistics from genome-wide association studies (GWAS) were extracted from the GWAS Catalog for CIPs, blood metabolites (BMs), and skin fibrosis. Two-sample MR and reverse MR were conducted to determine the effect of CIPs on skin fibrosis. Two-step MR was then performed to investigate the role of BMs in mediating the effect of CIPs on skin fibrosis. Reverse MR analysis was performed to confirm the unidirectional causality between CIPs and BMs, as well as between BMs and skin fibrosis.
Results: Bidirectional Mendelian randomization revealed negative associations between skin fibrosis and the levels of T-cell surface glycoprotein CD6 isoform (odds ratio [OR] 0.670 [95% confidence interval [CI] 0.472, 0.951], p = 0.025), Delta and Notch-like epidermal growth factor-related receptor (OR 0.779 [95% CI 0.609, 0.998], p = 0.048), and Interleukin-10 receptor subunit beta (OR 0.541 [95% CI 0.332, 0.884], p = 0.014). There was a positive association between skin fibrosis and levels of Fibroblast growth factor 21 (OR 2.276 [95% CI 1.064, 4.870], p = 0.034). Two-step MR showed that Retinol (Vitamin A) to the linoleoyl-arachidonoyl-glycerol ratio (βM 0.108 [95% CI 0.006, 0.210], p = 0.004) and the Cholesterol to linoleoyl-arachidonoyl-glycerol ratio (βM 0.238 [95% CI 0.002, 0.474], p = 0.048) were identified as mediators, which showed evidence of the mediated effect of the levels of Fibroblast growth factor 21 on Keloid through these mediators.
Conclusion: The study presented credible evidence of a causal association between CIPs and skin fibrosis, with BMs potentially acting as a mediator in this association. These findings offer new insights into early screening and prevention of skin fibrosis.
Skin fibrosis refers to a group of skin conditions where there is an excessive deposition of connective tissue components in the dermis. It can be caused by damage from burns, surgery, or trauma, which disrupts the balance between extracellular matrix synthesis and degradation, leading to disease. Skin fibrosis, including keloid and hypertrophic scars, can cause a loss of physiological architecture and skin malfunction, resulting in physical and psychological distress for patients (1, 2). Keloid is a refractory skin fibrotic disease with a recurrence rate of up to 45%. It is commonly considered a benign skin tumor due to its aggressive proliferation, which can result in limited movement or disfigurement (3, 4). Early assessment and accurate treatment of diseases are crucial. However, our current understanding of diseases is imperfect, and efficient and rapid diagnostic methods are lacking. Therefore, it is necessary to deepen our knowledge and explore new diagnosis, prevention, and intervention methods.
Circulating inflammatory proteins (CIPs) plays an important role in many diseases. For example, IL-10 has been demonstrated to be implicated in cerebral microcirculatory defects and cognitive impairment associated with type 1 diabetes (5). High plasma levels of MIP-1β and TNF-α were positively related to atherosclerotic plaques with high inflammatory activity (6). Investigating the correlation between CIPs and diseases is essential for clarifying disease mechanisms and developing disease prevention and treatment strategies (7). TGFB is known to play crucial roles in the occurrence and development of skin fibrosis, as evidenced by their increased content in fibrotic skin tissue (8). However, there are no significant differences in serum levels of TGFB between patients with skin fibrosis and healthy individuals (9). The causal relationship between CIPs and skin fibrosis is still unclear.
Blood metabolite (BMs) is a small molecule of metabolic reaction. Serum BMs levels are influenced by various factors, such as genetics and diseases. Furthermore, they can affect diseases and serve as a therapeutic target (10, 11). For example, branched-chain amino acids were identified as insulin analogues. The high levels of branched-chain amino acids could eventually lead to insulin resistance and diabetes (12). Currently, researchers have found that metabolites might be associated with skin fibrosis. For example, butyrate is shown to improve skin fibrosis in mouse models (13). Furthermore, a decrease in L-tryptophan was identified in patients suffering from systemic sclerosis. The level of L-tryptophan in these patients exhibited a negative correlation with inflammatory markers, such as IL-6 (14). However, the causal relationship remains unclear. Determining the causal role of BMs in skin fibrosis can identify effective intervention points for therapies.
Genome-wide association studies (GWAS) contribute significantly to our understanding of skin fibrosis in genetics (7). With single nucleotide polymorphisms (SNPs) of GWAS, we can perform Mendelian randomization (MR) analysis to infer a credible causal relationship (15). As a widely used analytical method, MR can help reduce bias and eliminate reverse causality. This is because genetic variations are randomly assigned during meiosis and are independent of environmental and other acquired factors (16). Compared to traditional observational approaches, MR analysis does not require unmeasured confounding between exposure and outcome. Mediation analysis shares these strengths (17). MR studies revealed a causal effect of CIPs on diseases, such as osteoarthritis and colorectal cancer (18, 19). Currently, there is no MR evidence to establish a causal association or mediated effect between CIPs and skin fibrosis.
GWAS summary data from the GWAS Catalog were utilized to perform MR and reverse MR analyses to examine the causal relationship between CIPs and skin fibrosis. In addition, a two-step MR (also referred to as network MR) analysis was conducted to investigate the causal role of BMs in linking the effect of CIPs on skin fibrosis. These findings may guide the exploration of mechanisms and generate new ideas for reducing the risk of skin fibrosis.
Two-sample MR and inverse MR analyses were performed on datasets from the GWAS catalog to determine the causal relationship between CIPs (exposure), BMs (mediator), and skin fibrosis (outcome) (Figure 1A). In step 1 of two-step of MR model, we established the causal effect of CIPs (exposure) on BMs (mediator) (Figure 1B). Then in step 2 of two-step MR model, we established the causal effect of BMs (exposure) on skin fibrosis (outcome) (Figure 1C). In the end, the mediated effect was calculated to determine the role of BMs in mediating the effect of CIPs on skin fibrosis (20, 21).
Figure 1. The design of the study. (A) The question is whether there is a causal role of BMs in mediating the effect of CIPs (exposure) on skin fibrosis (outcome). The data on CIPs was extracted from the GWAS Catalog (GCST90274758–GCST90274848), the data on BMs was extracted from the GWAS Catalog (GCST90199621–GCST90201020) and the data on skin fibrosis was extracted from the GWAS Catalog (GCST90044522 & GCST90044521). (B) Step 1 of the two-step of MR model: establishing the causal effect of CIPs (exposure) on BMs (mediator). (C) Step 2 of the two-step of MR model: establishing the causal effect of BMs (mediator) on skin fibrosis (outcome).
The GWAS Catalog (GCST90274758–GCST90274848) was used to extract summary statistics of CIPs traits, which encompass 91 inflammation-related plasma proteins. All CIPs were listed in the “CIPs list” (Supplementary Table 1). The data comprises 11 cohorts with a total of 14,824 participants of European ancestry (7). Summary statistics of BMs traits, including 1,091 blood metabolites and 309 metabolite ratios, were extracted from the GWAS catalog (GCST90199621-GCST90201020). The sample size consists of 8,299 individuals of European ancestry (10). All BMs were listed in the “BMs list” (Supplementary Table 1). The GWAS summary statistics for skin fibrosis traits from the GWAS Catalog (GCST90044522 & GCST90044521) include keloid scar and Scar conditions and fibrosis of skin (Figure 1A). GCST90044522 comprises 201 cases of European ancestry and 456,147 controls of European ancestry, while GCST90044521 comprises 1,887 cases of European ancestry and 454,461 controls of European ancestry (22). All skin fibrosis traits were listed in the “Skin fibrosis list” (Supplementary Table 1). A list of CIPs, BMs, and skin fibrosis markers used for data extraction from GWAS in the study is also presented in Supplementary Table 1.
For each trait, only SNPs that showed a strong association (p < 5 × 10−7) were considered as instrumental variables (IVs). To avoid weak IV bias, SNPs with an F-statistic < 10 were not defined as IVs. Additionally, clumping was performed with the EUR population reference (r2 < 0.01 and clump distance > 10000 kb) to eliminate linkage disequilibrium (LD). SNPs related to confounders were also excluded based on PhenoScanner V2. Finally, palindromic SNPs were either harmonized or excluded using ‘TwoSampleMR’ R package. After selection, the remaining SNPs were considered as IVs for MR and reverse MR analyses (23–25).
MR and reverse MR analyses between exposure and outcome, between exposure and mediator, and between mediator and outcome were performed by R (version 4.3.2) and R package TwoSampleMR package (version 0.5.10).
Inverse variance-weighted (IVW), MR-Egger, weighted median, simple mode, and weighted mode methods were used to determine the causal association. P-value > 5 × 10−2 showed statistically significant. IVW was considered the primary method because of providing a more robust estimation (17). MR-Pleiotropy Residual Sum and Outlier (MR-PRESSO) was utilized to remove outliers (26). In sensitivity analysis, Cochran’s test was performed to assess heterogeneity while Q statistic P-value > 5 × 10−2 showed no heterogeneity. MR-Egger test was performed to assess horizontal pleiotropy while the P-value > 5 × 10−2 showed no pleiotropy (27, 28). We assessed the horizontal pleiotropy by a leave-one-out analysis (23).
First, we performed MR and reverse MR analyses between CIPs and skin fibrosis to determine the causal effect of CIPs on skin fibrosis, referred to as the total effect (β) (17, 21). The CIPs traits with a causal association with skin fibrosis were used in step 1 of two-step of MR model.
Due to the significant BMs traits, we conducted a two-sample MR analysis to identify the BMs traits that may be related to skin fibrosis. These BMs traits were utilized in step 1 of two-step of MR model.
Then in step 1 of two-step of MR model, we established the causal effect of CIPs (exposure) on BMs (mediator) by MR and reverse MR analyses (20, 21). The study referred to the effect as the β1 effect. In step 2 of the two-step MR model, we used the BMs traits that have a causal association with CIPs. We performed MR and reverse MR analyses to determine the causal effect of BMs (mediator) on skin fibrosis (outcome), which is referred to as the β2 effect.
In the end, the mediated effect (βM) was calculated by the product of the coefficients method. The mediated effect (βM) = β – (β1 × β2).
Due to a lack of SNPs (p < 5 × 10−8) associated with skin fibrosis, we opted to extract SNPs with a P-value < 5 × 10−7 for further analysis (Supplementary Table 2). A circular manhattan plot was generated to display the chromosome positions and P-values of SNPs associated with the Keloid scar trait. The manhattan plot displayed the chromosome positions and P-value of SNPs associated with Scar conditions and fibrosis of skin trait. The red line represents the threshold line (p = 5 × 10−7) (Figures 2A, B).
Figure 2. The causal association between CIPs and skin fibrosis. (A) The circle manhattan plot of the Keloid scar trait (GCST90044522) (threshold = 5 × 10−7). (B) The manhattan plot of the Scar conditions and fibrosis of skin trait (GCST90044521) (threshold = 5 × 10−7). (C) Forest plot of casual effect between CIPs and skin fibrosis.
The results of MR and reverse MR analyses indicated that T-cell surface glycoprotein CD6 isoform (CD6) levels were negatively associated with Keloid (odds ratio [OR] 0.670 [95% confidence interval [CI] 0.472, 0.951], p = 0.025). Fibroblast growth factor 21 (FGF21) levels were positively associated with Keloid (OR 2.276 [95% CI 1.064, 4.870], p = 0.034). Delta and Notch-like epidermal growth factor-related receptor (DNER) levels were negatively associated with Scar conditions and fibrosis of skin (OR 0.779 [95% CI 0.609, 0.998], p = 0.048). Interleukin-10 receptor subunit beta (IL1RB) levels were negatively associated with Scar conditions and fibrosis of skin (OR 0.541 [95% CI 0.332, 0.884], p = 0.014) (Figure 2C). More details could be found in Supplementary Table 3. A leave-one-out analysis, forest plot, and scatter plot were performed to verify the credibility of the results (Supplementary Figures S1-4).
There were 30 BMs potentially associated with keloid (IVW p < 5 × 10−2) and 19 BMs potentially associated with Scar conditions and fibrosis of skin (IVW p < 5 × 10−2) (Figures 3A, B). More details could be found in Supplementary Table 4. In step 1 of two-step of MR model, we found that CD6 levels were negatively related to Carotenoid levels (cryptoxanthin) (OR 0.942 [95% CI 0.892, 0.994]). FGF21 levels were positively related with Indolebutyrate levels (OR 1.358 [95% CI 1.121, 1.645]), 3-methylglutaconate levels (OR 1.278 [95% CI 1.113, 1.468]) and Cis-3,4-methyleneheptanoylglycine levels (OR 1.259 [95% CI 1.093, 1.450]). FGF21 levels were negatively related with Carotene diol (1) levels (OR 0.730 [95% CI 0.635, 0.840]), Retinol (Vitamin A) to linoleoyl-arachidonoyl-glycerol ratio (OR 0.818 [95% CI 0.706, 0.948]) and Cholesterol to linoleoyl-arachidonoyl-glycerol ratio (OR 0.720 [95% CI 0.603, 0.861]). All the IVW P-values were < 5 × 10−2 (Figure 4A). DNER levels were negatively related to 4-hydroxyphenylacetoylcarnitine levels (OR 0.878 [95% CI 0.779, 0.989]). IL10RB levels were positively related to X-23659 levels (OR 1.315 [95% CI 1.068, 1.619]) and N-acetylasparagine levels (OR 1.372 [95% CI 1.095, 1.719]). IL10RB levels were negatively related to N-delta-acetylornithine levels (OR 0.692 [95% CI 0.555, 0.863]). All the IVW P-values were < 5 × 10−2 (Figure 4B). More details could be found in Supplementary Table 5.
Figure 3. BMs potentially associated with skin fibrosis. (A) BMs related to keloid. (B) BMs related to Scar conditions and fibrosis of skin.
Figure 4. The causal association between CIPs and BMs. (A) Forest plot of casual effect between Keloid scar related CIPs and BMs. (B) Forest plot of casual effect between Scar conditions and fibrosis of skin related CIPs and BMs.
In step 2 of two-step of MR model, Retinol (Vitamin A) to linoleoyl-arachidonoyl-glycerol ratio was negatively related to Keloid (OR 0.583 [95% CI 0.349, 0.976]). Cholesterol to linoleoyl-arachidonoyl-glycerol ratio was negatively related to Keloid (OR 0.483 [95% CI 0.243, 0.960]) (Figure 5A). 4-hydroxyphenylacetoylcarnitine levels were negatively related to Scar conditions and fibrosis of skin (OR 0.774 [95% CI 0.613, 0.976]). X-23659 levels were positively related to Scar conditions and fibrosis of skin (OR 1.283 [95% CI 1.007, 1.635]). N-acetylasparagine levels were positively related to Scar conditions and fibrosis of skin (OR 1.114 [95% CI 1.024, 1.212]). All P-values were < 5 × 10−2 (Figure 5B). More details could be found in Supplementary Table 6.
Figure 5. The causal association between BMs and skin fibrosis. (A) Forest plot of casual effect between Keloid scar related BMs and Keloid scar. (B) Forest plot of casual effect between Scar conditions and fibrosis of skin related BMs and Scar conditions and fibrosis of skin.
Using the product of coefficients method, we calculated the indirect mediation effect based on the results of the two-step MR model. FGF21 levels were positively associated with Keloid (OR 2.276 [95% CI 1.064, 4.870]). It was found that there is a negative association between FGF21 levels and Retinol (Vitamin A) to linoleoyl-arachidonoyl-glycerol ratio (OR 0.818 [95% CI 0.706, 0.948]). Retinol (Vitamin A) to linoleoyl-arachidonoyl-glycerol ratio was negatively related to Keloid (OR 0.583 [95% CI 0.349, 0.976]) (Figure 6A). It was found that there is a negative association between FGF21 levels and Cholesterol to linoleoyl-arachidonoyl-glycerol ratio (OR 0.720 [95% CI 0.603, 0.861]). Cholesterol to linoleoyl-arachidonoyl-glycerol ratio was negatively related to Keloid (OR 0.483 [95% CI 0.243, 0.960]) (Figure 6B). Then mediated effect analysis showed evidence of the mediated effect of FGF21 on Keloid through Retinol (Vitamin A) to linoleoyl-arachidonoyl-glycerol ratio (βM 0.108 [95% CI 0.006, 0.210], p = 0.004) and Cholesterol to linoleoyl-arachidonoyl-glycerol ratio (βM 0.238 [95% CI 0.002, 0.474], p = 0.048) with a mediated proportion of 13.1% and 29% of the total effect, respectively (Figures 6C, D).
Figure 6. The causal association among CIPs, BMs and skin fibrosis. (A, B) Forest plot of casual effect among CIPs, BMs and keloid scar. (C, D) The effect and causal pathways among CIPs, BMs and keloid scar.
Frost plots were also exhibited for the other traits, but their credible mediated effect and proportion could not be calculated (Supplementary Figure S5).
The results of MR analyses revealed a causal association between CIPs and skin fibrosis. The levels of CD6, DNER, and IL10RB were negatively related to skin fibrosis while FGF21 was associated with an increased risk of skin fibrosis. According to mediation analysis, we found the evidence of mediated effect of FGF21 on skin fibrosis through Retinol (Vitamin A) to linoleoyl-arachidonoyl-glycerol ratio and Cholesterol to linoleoyl-arachidonoyl-glycerol ratio.
The role of inflammatory factor is crucial in the development of many diseases and is often targeted for therapeutic intervention. For example, the presence of ILR supports the maintenance of a CD8+ T cell population that sustains anti-tumor immunity (29). CD6 was identified as a therapeutic target of lupus nephritis (30). Changes in the expression levels of inflammatory factors, such as TGFB, can be detected in skin fibrotic tissues (8). Early diagnosis and treatment help prevent irreversible sequelae, such as cutaneous and subcutaneous atrophy. However, obtaining information for prevention and early diagnosis without tissue sampling or resection can be challenging. While CCL18 has been reported as a biomarker of skin fibrosis as an inflammation-related plasma protein, the investigation of CIPs in this area is still ongoing (9). There is a lack of causal association between CIPs and skin fibrosis. It is unclear whether CIPs is solely an index of skin fibrosis, or it can be considered as a target for diagnosis and treatment. For example, c-reactive protein (CRP) was considered as a biomarker of sepsis and COVID-19 disease progression (31, 32). However, it may not be appropriate to consider CRP as a therapeutic target for these diseases. In the present study, it was demonstrated that CD6, DNER, FGF21 and IL10RB exhibited a casual association with skin fibrosis. This finding suggests that these CIPs could be considered as potential therapeutic targets as well as biomarker. The CD6 lymphocyte receptor has been implicated in psoriasis, a chronic inflammatory skin disease (33). Targeting CD6 is an effective and well-tolerated novel biological therapy in moderate to severe psoriasis (34). In addition, CD6 was reported to be able to delay skin senescence induced by ultraviolet radiation b radiation (35). As we know, skin fibrosis is a typical aging-related pathological process (36). Modulating cellular senescence can inhibit fibrosis (37, 38). Further exploration of CD6 function provides insights into novel targets for addressing skin fibrosis. FGF21 was reported to be positively related to senescent cell accumulation at systemic and cellular levels (39). Besides, FGF21 is upregulated in tissue and can promote migration and differentiation of epidermal cells during wound healing (40). The disorder of plasma FGF21 may have an influence in disturbance of the tissue repair mechanism, which can ultimately result in skin fibrosis. What’s more, IL10RB has been demonstrated to limit liver fibrosis by inducing stellate cell senescence upon binding to IL22 (41). IL10 inhibits autophagy in hypertrophic scar fibroblasts via IL10-IL10R-STAT3 pathway which is helpful for treating skin fibrosis (42). The function of DNER is still unclear in fibrosis. These CIPs could be potential therapeutic targets for preventing and diagnosing skin fibrosis early. Improving our understanding of CIPs is expected to improve patients’ quality of life and provide insights into novel targets for addressing skin fibrosis.
Targeting CIPs may be a difficult task. We can focus on substances that are easy to regulate to intervene in the effect of CIPs on skin fibrosis. BMs is currently a focus and hotspot of research as it plays a crucial role in both tumor and non-tumor diseases (11, 43). BMs levels can be regulated by many factors, such as diet and lifestyle (10). Given the reported correlation between BMs and CIPs, regulating BMs may be a viable strategy (13, 14). There is evidence of the mediated effect of CIPs on skin fibrosis through BMs in our study. According to mediation analysis, both Retinol (Vitamin A) to linoleoyl-arachidonoyl-glycerol ratio and Cholesterol to linoleoyl-arachidonoyl-glycerol ratio mediated the effect of CIPs on skin fibrosis. The mediated effect of Retinol (Vitamin A) to linoleoyl-arachidonoyl-glycerol ratio was 0.108, and the mediated proportion was13.1%. In comparison, the mediated effect of Cholesterol to linoleoyl-arachidonoyl-glycerol ratio was 0.238 and the mediated proportion was 29%. Retinol has been shown to promote various anti-aging benefits for the skin (44, 45). In addition, it has been documented that retinol has an influence on the specification and differentiation of fibroblasts, indicating an anti-fibrotic effect (46). Cholesterol is a vital skin barrier lipid that plays a crucial role in maintaining skin homeostasis. The impairment of the skin barrier and disruption of skin homeostasis, which can lead to leaky epithelia and disease (47, 48). A cholesterol deficiency can lead to skin damage which may cause skin fibrosis (2, 49). It is important to maintain adequate levels of cholesterol for healthy skin. However, excessive intake of cholesterol can harm organs such as the liver and cardiovascular system (50). Compared with cholesterol, it is a better choice to promote the intake of retinol when intervening in the effect of FGF21 on skin fibrosis through retinol. Concurrently, it is also necessary to maintain the equilibrium of cholesterol levels within the plasma. Moreover, a more profound understanding of the interactions between the CIPs and BMs may facilitate the development of more efficacious treatments.
However, there are some limitations in our study. Caution should be exercised when interpreting these results as the study was analyzed at the genetic level. As the study individuals were predominantly of European ancestry, it is worth investigating whether these results apply to other ethnic groups, despite the large sample size (20). Furthermore, in the absence of a sufficiently extensive CIP dataset, it becomes challenging to conduct analyses encompassing all categories of CIPs such as CD8, CCL18 and CRP. Consequently, the present study is unable to explore the casual association between these CIPs and skin fibrosis, as well as whether BMs act as a mediating role in it. Large MR analyses were conducted on 1400 BMs traits, 91 CIPs traits, and two skin fibrosis traits. It is difficult to perform Bonferroni correction to obtain statistically significant results. Therefore, caution should be exercised when interpreting results with IVW-derived P values less than 5 × 10−2.
Skin fibrosis is a chronic dermatological condition characterized by a disruption of skin homeostasis. Early clinical manifestations of skin fibrosis are characterized by the expansion of sclerotic lesions. Early screening and treatment can prevent extensive skin involvement and avoid irreversible sequelae such as contractures and severe atrophy. MR analyses were performed to ascertain the causal association between the CIPs, CD6, FGF21, IL1RB, and DNER, and skin fibrosis. The results suggested that CIPs could be a potential target for skin fibrosis. Mediated effect analysis showed evidence of the mediated effect of FGF21 on the skin fibrosis through Retinol (Vitamin A) to linoleoyl-arachidonoyl-glycerol ratio and Cholesterol to linoleoyl-arachidonoyl-glycerol ratio. The analysis showed that BMs mediated the effect of CIPs on skin fibrosis.
It is essential to investigate the role of specific CIPs in dermatological conditions for precise and personalized treatments. Compare with tissue sampling or resection, the level of inflammation-related plasma protein could be detected in a rapid and efficient manner for early screening and diagnosis. Besides, the targeting of both specific CIPs and BMs may provide novel therapeutic strategies for patients. By detecting the level of the specific CIPs, it is possible to evaluate the effect of treatment and modify the therapeutic strategy. Improving the understanding of the impact of CIPs on skin fibrosis has the potential to improve patients’ quality of life and facilitate the development of innovative strategies for skin fibrosis.
The original contributions presented in the study are included in the article/Supplementary Material. Further inquiries can be directed to the corresponding authors.
ZZ: Conceptualization, Data curation, Formal analysis, Investigation, Methodology, Software, Validation, Visualization, Writing – original draft, Writing – review & editing. DL: Conceptualization, Data curation, Formal analysis, Investigation, Methodology, Software, Validation, Visualization, Writing – review & editing. RZ: Conceptualization, Data curation, Formal analysis, Investigation, Methodology, Software, Validation, Visualization, Writing – review & editing. YR: Writing – review & editing. ZX: Writing – review & editing. RY: Writing – review & editing, Funding acquisition, Resources. ZH: Writing – review & editing. XC: Supervision, Writing – review & editing. BT: Funding acquisition, Resources, Supervision, Writing – review & editing.
The author(s) declare that financial support was received for the research and/or publication of this article. This study was supported by the National Natural Science Foundation of China (No. 82072181, No. 82103706, and No. 82272273), and the Sun Yat-sen University Clinical Research 5010 Program (No. 2018003).
The authors express their gratitude to the participants and investigators GWAS Catalog and PhenoScanner V2.
The authors declare that the research was conducted in the absence of any commercial or financial relationships that could be construed as a potential conflict of interest.
All claims expressed in this article are solely those of the authors and do not necessarily represent those of their affiliated organizations, or those of the publisher, the editors and the reviewers. Any product that may be evaluated in this article, or claim that may be made by its manufacturer, is not guaranteed or endorsed by the publisher.
The Supplementary Material for this article can be found online at: https://www.frontiersin.org/articles/10.3389/fendo.2025.1416993/full#supplementary-material
Supplementary Figure 1 | Leave-one-out plot, forest plot, funnel plot, and scatter plot for the causal association between CD6 levels and keloid scar.
Supplementary Figure 2 | Leave-one-out plot, forest plot, funnel plot, and scatter plot for the causal association between FGF21 levels isoform levels and keloid scar.
Supplementary Figure 3 | Leave-one-out plot, forest plot, funnel plot, and scatter plot for the causal association between DNER levels and Scar conditions and fibrosis of skin.
Supplementary Figure 4 | Leave-one-out plot, forest plot, funnel plot, and scatter plot for the causal association between IL10RB levels and Scar conditions and fibrosis of skin.
Supplementary Figure 5 | Forest plot of casual effect among CIPs, BMs and Scar conditions and fibrosis of skin.
Supplementary Table 1 | The CIPs list and overview of the source of data.
Supplementary Table 2 | SNPs associated with skin fibrosis (5 × 10−8).
Supplementary Table 3 | MR and reverse MR analyses between 91 CIPs and skin fibrosis.
Supplementary Table 4 | MR analysis between 1400 BMs and skin fibrosis.
Supplementary Table 5 | MR and reverse MR analyses between CIPs and BMs.
Supplementary Table 6 | MR and reverse MR analyses between BMs and skin fibrosis.
1. Condorelli AG, El Hachem M, Zambruno G, Nystrom A, Candi E, Castiglia D. Notch-ing up knowledge on molecular mechanisms of skin fibrosis: focus on the multifaceted notch signalling pathway. J BioMed Sci. (2021) 28:36. doi: 10.1186/s12929-021-00732-8
2. Griffin MF, Huber J, Evan FJ, Quarto N, Longaker MT. The role of Wnt signaling in skin fibrosis. Med Res Rev. (2022) 42:615–28. doi: 10.1002/med.21853
3. Frech FS, Hernandez L, Urbonas R, Zaken GA, Dreyfuss I, Nouri K. Hypertrophic scars and keloids: advances in treatment and review of established therapies. Am J Clin Dermatol. (2023) 24:225–45. doi: 10.1007/s40257-022-00744-6
4. Lv W, Ren Y, Hou K, Hu W, Yi Y, Xiong M, et al. Epigenetic modification mechanisms involved in keloid: current status and prospect. Clin Epigenet. (2020) 12:183. doi: 10.1186/s13148-020-00981-8
5. Sharma S, Cheema M, Reeson PL, Narayana K, Boghozian R, Cota AP, et al. A pathogenic role for Il-10 signalling in capillary stalling and cognitive impairment in type 1 diabetes. Nat Metab. (2024) 6:2082–99. doi: 10.1038/s42255-024-01159-9
6. Edsfeldt A, Grufman H, Asciutto G, Nitulescu M, Persson A, Nilsson M, et al. Circulating cytokines reflect the expression of pro-inflammatory cytokines in atherosclerotic plaques. Atherosclerosis. (2015) 241:443–9. doi: 10.1016/j.atherosclerosis.2015.05.019
7. Zhao JH, Stacey D, Eriksson N, Macdonald-Dunlop E, Hedman AK, Kalnapenkis A, et al. Genetics of circulating inflammatory proteins identifies drivers of immune-mediated disease risk and therapeutic targets. Nat Immunol. (2023) 24:1540–51. doi: 10.1038/s41590-023-01588-w
8. Distler JHW, Gyorfi AH, Ramanujam M, Whitfield ML, Konigshoff M, Lafyatis R. Shared and distinct mechanisms of fibrosis. Nat Rev Rheumatol. (2019) 15:705–30. doi: 10.1038/s41584-019-0322-7
9. Mertens JS, de Jong E, van den Hoogen LL, Wienke J, Thurlings RM, Seyger MMB, et al. The identification of ccl18 as biomarker of disease activity in localized scleroderma. J Autoimmun. (2019) 101:86–93. doi: 10.1016/j.jaut.2019.04.008
10. Chen Y, Lu T, Pettersson-Kymmer U, Stewart ID, Butler-Laporte G, Nakanishi T, et al. Genomic atlas of the plasma metabolome prioritizes metabolites implicated in human diseases. Nat Genet. (2023) 55:44–53. doi: 10.1038/s41588-022-01270-1
11. Pietzner M, Stewart ID, Raffler J, Khaw KT, Michelotti GA, Kastenmuller G, et al. Plasma metabolites to profile pathways in noncommunicable disease multimorbidity. Nat Med. (2021) 27:471–9. doi: 10.1038/s41591-021-01266-0
12. Wishart DS. Emerging applications of metabolomics in drug discovery and precision medicine. Nat Rev Drug Discovery. (2016) 15:473–84. doi: 10.1038/nrd.2016.32
13. Park HJ, Jeong OY, Chun SH, Cheon YH, Kim M, Kim S, et al. Butyrate improves skin/lung fibrosis and intestinal dysbiosis in bleomycin-induced mouse models. Int J Mol Sci. (2021) 22:2765. doi: 10.3390/ijms22052765
14. Guo M, Liu D, Jiang Y, Chen W, Zhao L, Bao D, et al. Serum metabolomic profiling reveals potential biomarkers in systemic sclerosis. Metabolism. (2023) 144:155587. doi: 10.1016/j.metabol.2023.155587
15. Bowden J, Holmes MV. Meta-analysis and mendelian randomization: A review. Res Synth Methods. (2019) 10:486–96. doi: 10.1002/jrsm.1346
16. Sekula P, Del Greco MF, Pattaro C, Kottgen A. Mendelian randomization as an approach to assess causality using observational data. J Am Soc Nephrol. (2016) 27:3253–65. doi: 10.1681/ASN.2016010098
17. Zhao SS, Holmes MV, Zheng J, Sanderson E, Carter AR. The impact of education inequality on rheumatoid arthritis risk is mediated by smoking and body mass index: Mendelian randomization study. Rheumatol (Oxford). (2022) 61:2167–75. doi: 10.1093/rheumatology/keab654
18. Jiang Z, Cai X, Yao X, Zhang S, Lan W, Jin Z, et al. The causal effect of cytokine cycling levels on osteoarthritis: A bidirectional Mendelian randomized study. Front Immunol. (2023) 14:1334361. doi: 10.3389/fimmu.2023.1334361
19. Meng C, Sun L, Shi J, Li Y, Gao J, Liu Y, et al. Exploring causal correlations between circulating levels of cytokines and colorectal cancer risk: A Mendelian randomization analysis. Int J Cancer. (2024) 155:159–71. doi: 10.1002/ijc.34891
20. Xu M, Zheng J, Hou T, Lin H, Wang T, Wang S, et al. Sglt2 inhibition, choline metabolites, and cardiometabolic diseases: A mediation Mendelian randomization study. Diabetes Care. (2022) 45:2718–28. doi: 10.2337/dc22-0323
21. Carter AR, Sanderson E, Hammerton G, Richmond RC, Davey Smith G, Heron J, et al. Mendelian randomisation for mediation analysis: current methods and challenges for implementation. Eur J Epidemiol. (2021) 36:465–78. doi: 10.1007/s10654-021-00757-1
22. Jiang L, Zheng Z, Fang H, Yang J. A generalized linear mixed model association tool for biobank-scale data. Nat Genet. (2021) 53:1616–21. doi: 10.1038/s41588-021-00954-4
23. Meng C, Deng P, Miao R, Tang H, Li Y, Wang J, et al. Gut microbiome and risk of ischaemic stroke: A comprehensive mendelian randomization study. Eur J Prev Cardiol. (2023) 30:613–20. doi: 10.1093/eurjpc/zwad052
24. Li P, Wang H, Guo L, Gou X, Chen G, Lin D, et al. Association between gut microbiota and preeclampsia-eclampsia: A two-sample Mendelian randomization study. BMC Med. (2022) 20:443. doi: 10.1186/s12916-022-02657-x
25. Kamat MA, Blackshaw JA, Young R, Surendran P, Burgess S, Danesh J, et al. Phenoscanner V2: an expanded tool for searching human genotype-phenotype associations. Bioinformatics. (2019) 35:4851–3. doi: 10.1093/bioinformatics/btz469
26. Verbanck M, Chen CY, Neale B, Do R. Detection of widespread horizontal pleiotropy in causal relationships inferred from Mendelian randomization between complex traits and diseases. Nat Genet. (2018) 50:693–8. doi: 10.1038/s41588-018-0099-7
27. Greco MF, Minelli C, Sheehan NA, Thompson JR. Detecting pleiotropy in Mendelian randomisation studies with summary data and a continuous outcome. Stat Med. (2015) 34:2926–40. doi: 10.1002/sim.6522
28. Sanderson E, Davey Smith G, Windmeijer F, Bowden J. An examination of multivariable Mendelian randomization in the single-sample and two-sample summary data settings. Int J Epidemiol. (2019) 48:713–27. doi: 10.1093/ije/dyy262
29. Hanna BS, Llao-Cid L, Iskar M, Roessner PM, Klett LC, Wong JKL, et al. Interleukin-10 receptor signaling promotes the maintenance of a pd-1(Int) Tcf-1(+) Cd8(+) T cell population that sustains anti-tumor immunity. Immunity. (2021) 54:2825–41.e10. doi: 10.1016/j.immuni.2021.11.004
30. Phillips R. Cd6 is a therapeutic target for Ln. Nat Rev Rheumatol. (2022) 18:125. doi: 10.1038/s41584-022-00756-w
31. Pierrakos C, Velissaris D, Bisdorff M, Marshall JC, Vincent JL. Biomarkers of sepsis: time for a reappraisal. Crit Care. (2020) 24:287. doi: 10.1186/s13054-020-02993-5
32. Ponti G, Maccaferri M, Ruini C, Tomasi A, Ozben T. Biomarkers associated with Covid-19 disease progression. Crit Rev Clin Lab Sci. (2020) 57:389–99. doi: 10.1080/10408363.2020.1770685
33. Consuegra-Fernandez M, Julia M, Martinez-Florensa M, Aranda F, Catala C, Armiger-Borras N, et al. Genetic and experimental evidence for the involvement of the Cd6 lymphocyte receptor in psoriasis. Cell Mol Immunol. (2018) 15:898–906. doi: 10.1038/cmi.2017.119
34. Krupashankar DS, Dogra S, Kura M, Saraswat A, Budamakuntla L, Sumathy TK, et al. Efficacy and safety of itolizumab, a novel anti-Cd6 monoclonal antibody, in patients with moderate to severe chronic plaque psoriasis: results of a double-blind, randomized, placebo-controlled, phase-iii study. J Am Acad Dermatol. (2014) 71:484–92. doi: 10.1016/j.jaad.2014.01.897
35. Kim S. Lncrna-mirna-Mrna regulatory networks in skin aging and therapeutic potentials. Front Physiol. (2023) 14:1303151. doi: 10.3389/fphys.2023.1303151
36. Wei W, Li T, Chen J, Fan Z, Gao F, Yu Z, et al. Sirt3/6: an amazing challenge and opportunity in the fight against fibrosis and aging. Cell Mol Life Sci. (2024) 81:69. doi: 10.1007/s00018-023-05093-z
37. Guan R, Yuan L, Li J, Wang J, Li Z, Cai Z, et al. Bone morphogenetic protein 4 inhibits pulmonary fibrosis by modulating cellular senescence and mitophagy in lung fibroblasts. Eur Respir J. (2022) 60:2102307. doi: 10.1183/13993003.02307-2021
38. Zhang J, Yu H, Man MQ, Hu L. Aging in the dermis: fibroblast senescence and its significance. Aging Cell. (2024) 23:e14054. doi: 10.1111/acel.14054
39. Sorokina AG, Orlova YA, Grigorieva OA, Novoseletskaya ES, Basalova NA, Alexandrushkina NA, et al. Correlations between biomarkers of senescent cell accumulation at the systemic, tissue and cellular levels in elderly patients. Exp Gerontol. (2023) 177:112176. doi: 10.1016/j.exger.2023.112176
40. Chen X, Tong G, Fan J, Shen Y, Wang N, Gong W, et al. Fgf21 promotes migration and differentiation of epidermal cells during wound healing via Sirt1-dependent autophagy. Br J Pharmacol. (2022) 179:1102–21. doi: 10.1111/bph.15701
41. Kong X, Feng D, Wang H, Hong F, Bertola A, Wang FS, et al. Interleukin-22 induces hepatic stellate cell senescence and restricts liver fibrosis in mice. Hepatology. (2012) 56:1150–9. doi: 10.1002/hep.25744
42. Shi J, Wang H, Guan H, Shi S, Li Y, Wu X, et al. Il10 inhibits starvation-induced autophagy in hypertrophic scar fibroblasts via cross talk between the Il10-Il10r-Stat3 and Il10-Akt-Mtor pathways. Cell Death Dis. (2016) 7:e2133. doi: 10.1038/cddis.2016.44
43. Watson MJ, Vignali PDA, Mullett SJ, Overacre-Delgoffe AE, Peralta RM, Grebinoski S, et al. Metabolic support of tumour-infiltrating regulatory T cells by lactic acid. Nature. (2021) 591:645–51. doi: 10.1038/s41586-020-03045-2
44. Quan T. Human skin aging and the anti-aging properties of retinol. Biomolecules. (2023) 13:1614. doi: 10.3390/biom13111614
45. Gudas LJ. Synthetic retinoids beyond cancer therapy. Annu Rev Pharmacol Toxicol. (2022) 62:155–75. doi: 10.1146/annurev-pharmtox-052120-104428
46. Wang S, Yu J, Kane MA, Moise AR. Modulation of retinoid signaling: therapeutic opportunities in organ fibrosis and repair. Pharmacol Ther. (2020) 205:107415. doi: 10.1016/j.pharmthera.2019.107415
47. Akdis CA. Does the epithelial barrier hypothesis explain the increase in allergy, autoimmunity and other chronic conditions? Nat Rev Immunol. (2021) 21:739–51. doi: 10.1038/s41577-021-00538-7
48. Li W, Wang Z, Cao J, Dong Y, Chen Y. Melatonin improves skin barrier damage caused by sleep restriction through gut microbiota. J Pineal Res. (2023) 75:e12874. doi: 10.1111/jpi.12874
49. Sochorova M, Audrlicka P, Cervena M, Kovacik A, Kopecna M, Opalka L, et al. Permeability and microstructure of cholesterol-depleted skin lipid membranes and human stratum corneum. J Colloid Interface Sci. (2019) 535:227–38. doi: 10.1016/j.jcis.2018.09.104
Keywords: circulating inflammatory protein, skin fibrosis, bidirectional Mendelian randomization, mediation analysis, blood metabolite
Citation: Zhao Z, Lv D, Zeng R, Rong Y, Xu Z, Yin R, Hu Z, Cao X and Tang B (2025) Association and mediation between circulating inflammatory proteins and skin fibrosis. Front. Endocrinol. 16:1416993. doi: 10.3389/fendo.2025.1416993
Received: 17 April 2024; Accepted: 26 February 2025;
Published: 18 March 2025.
Edited by:
Darko Stefanovski, University of Pennsylvania, United StatesReviewed by:
Muhammad Nadeem Aslam, The University of Michigan Medical School, United StatesCopyright © 2025 Zhao, Lv, Zeng, Rong, Xu, Yin, Hu, Cao and Tang. This is an open-access article distributed under the terms of the Creative Commons Attribution License (CC BY). The use, distribution or reproduction in other forums is permitted, provided the original author(s) and the copyright owner(s) are credited and that the original publication in this journal is cited, in accordance with accepted academic practice. No use, distribution or reproduction is permitted which does not comply with these terms.
*Correspondence: Xiaoling Cao, Caoxling3@mail.sysu.edu.cn; Bing Tang, tangbing@mail.sysu.edu.cn
†These authors have contributed equally to this work
Disclaimer: All claims expressed in this article are solely those of the authors and do not necessarily represent those of their affiliated organizations, or those of the publisher, the editors and the reviewers. Any product that may be evaluated in this article or claim that may be made by its manufacturer is not guaranteed or endorsed by the publisher.
Research integrity at Frontiers
Learn more about the work of our research integrity team to safeguard the quality of each article we publish.