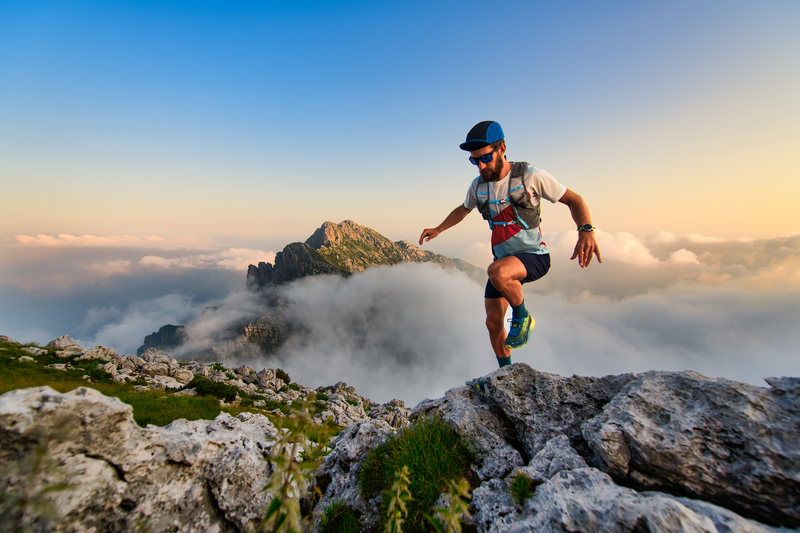
94% of researchers rate our articles as excellent or good
Learn more about the work of our research integrity team to safeguard the quality of each article we publish.
Find out more
ORIGINAL RESEARCH article
Front. Endocrinol.
Sec. Cardiovascular Endocrinology
Volume 16 - 2025 | doi: 10.3389/fendo.2025.1405833
The final, formatted version of the article will be published soon.
You have multiple emails registered with Frontiers:
Please enter your email address:
If you already have an account, please login
You don't have a Frontiers account ? You can register here
Introduction: This study investigates the subclasses of metabolic syndrome (Mets) and their relationship with non-alcoholic fatty liver (NAFLD) and the probable predictive role of serum vitamin D and CRP levels. Methods: This community-based, cross-sectional study was performed on adults in the framework of the Amol cohort prospective study (AmolCPS). Mets was defined as Adult Treatment Panel III criteria (ATP III) and ultrasound was used to diagnose NAFLD. Anthropometric and blood pressure measurements were conducted, and biochemical measurements were assessed after fasting. Data analysis included Latent class analysis, two-tailed χ2 statistics, one-way analysis of variance, and logistic regression using Mplus (version 7.4) and spss (version 26) softwares. Results: The study involved 2308 participants, with a mean age of 43.17±12.30 years. Mets prevalence was 25.64%, with three identified classes: Mets with Hypertension (HTN), Mets without HTN (Non-HTN), and Low Risk. Mets with HTN had a high probability of at least four components, particularly high SBP. Non-HTN had at least three high probable components, especially high TG and low HDL but not high SBP and DBP. The low-risk class had a low probability of all components except low HDL in women. Serum vitamin D and CRP levels did not significantly predict Mets classes in men, while CRP level significantly predicted the HTN class in women (OR:1.03, CI:1.004-1.067). Both HTN, and Non-HTN Mets classes significantly increased the odds of NAFLD compared to the low risk class, especially in women (HTN class OR: 4.20 vs 2.94; non-HTN class OR: 5.60 vs 3.12 in women and men respectively). Conclusion: The latent class analysis in northern Iran identified three Mets classes: HTN, Non-HTN, and low-risk, with hypertension playing a crucial role in determining these classes. These classes were stronger predictors of NAFLD in women. Serum CRP and vitamin D levels did not emerge as significant predictors of the classes, except for serum CRP in the HTN class among women.
Keywords: latent class analysis, metabolic syndrome, Iran, non-alcoholic fatty liver, Hypertension latent class analysis
Received: 06 Apr 2024; Accepted: 22 Jan 2025.
Copyright: © 2025 Amirkalali, Hassanzadeh, Sheikholmolooki, Gholizadeh, Doustmohammadian, Tameshkel, Motamed, Maadi, Sohrabi, Sobhrakhshankhah, Zamani and Ajdarkosh. This is an open-access article distributed under the terms of the Creative Commons Attribution License (CC BY). The use, distribution or reproduction in other forums is permitted, provided the original author(s) or licensor are credited and that the original publication in this journal is cited, in accordance with accepted academic practice. No use, distribution or reproduction is permitted which does not comply with these terms.
* Correspondence:
Farhad Zamani, Gastrointestinal and Liver Diseases Research Center, Iran University of Medical Sciences, Tehran, Iran
Hossein Ajdarkosh, Gastrointestinal and Liver Diseases Research Center, Iran University of Medical Sciences, Tehran, Iran
Disclaimer: All claims expressed in this article are solely those of the authors and do not necessarily represent those of their affiliated organizations, or those of the publisher, the editors and the reviewers. Any product that may be evaluated in this article or claim that may be made by its manufacturer is not guaranteed or endorsed by the publisher.
Research integrity at Frontiers
Learn more about the work of our research integrity team to safeguard the quality of each article we publish.