- 1Graduate School, Heilongjiang University of Chinese Medicine, Harbin, China
- 2First Affiliated Hospital, Heilongjiang University of Chinese Medicine, Harbin, China
Objective: This study aimed to explore the role of the waist-to-height ratio (WHtR) in assessing insulin resistance (IR) in patients with polycystic ovary syndrome (PCOS).
Materials and methods: We enrolled 882 PCOS-afflicted women in a cross-sectional analysis to evaluate the association of the WHtR with IR. Their demographic characteristics, anthropometric parameters, and fasting blood samples were collected and measured. Moreover, IR was evaluated by homeostatic model assessment of insulin resistance (HOMA-IR). We estimated the relationship between the WHtR and IR and the cut-off thresholds of the WHtR for IR using multivariable linear regression and logistic regression models, respectively.
Result(s): The prevalence rate of IR was 51.9%. The patients with PCOS and IR displayed significantly increased values for body mass index (BMI), waist circumference (WC), WHtR, systolic blood pressure (SBP), diastolic blood pressure (DBP), free androgen index (FAI), HOMA-IR, total cholesterol (TC), triglyceride (TG), low-density lipoprotein cholesterol (LDL-C), and apolipoprotein B (ApoB). However, the patients with PCOS and IR showed a reduction in estradiol (E2), luteinizing hormone (LH), LH/FSH ratio, sex hormone binding globulin (SHBG), and high-density lipoprotein (HDL-C) values than those without IR. Moreover, BMI (log-transformed), WC, and HOMA-IR (log-transformed) were positively correlated with the WHtR. When adjusting for potential confounding variables, the WHtR was significantly associated with HOMA-IR (log-transformed), with a standardized regression coefficient of 0.271. Furthermore, the WHtR was significantly associated with an increased risk of IR, with the adjusted odds ratio (OR) of 3.15 (WHtR multiplied by 10). Additionally, the WHtR helped to identify IR in women with PCOS with an optimal cut-off point of 0.519 (Youden index = 0.433).
Conclusion(s): The WHtR had a positive association with IR in women with PCOS. Hence, we suggest that the WHtR, as a simple, practical, and reliable anthropometric measure, can be used to predict the risk of IR in patients with PCOS.
1 Introduction
With a global prevalence ranging from 4% to 21%, polycystic ovary syndrome (PCOS) is one of the most frequent endocrine conditions affecting women of childbearing age (1). PCOS is also significantly associated with several endocrine and metabolic abnormalities, including insulin resistance (IR), cardiovascular risks, and androgen excess (2–4). Since IR has been identified as a pivotal pathogenic component of many metabolic diseases, early detection of IR in women with PCOS is crucial (5).
IR refers to an inadequate cellular response to insulin action, which upsets glucose homeostasis and leads to compensatory hyperinsulinemia, impaired fasting glucose, impaired glucose tolerance, or type 2 diabetes mellitus (6). Furthermore, IR is a disordered physiological response to insulin stimulation through interference in different molecular pathways in target tissues (7). For women with PCOS, IR could also selectively affect certain tissues’ metabolic or mitotic pathways, including their ovaries (8). IR is prevalent in PCOS-afflicted women and affects approximately 12% to 60% of them (9). Thus, IR could be a beneficial measure for managing PCOS and its associated complications (10). Since the hyperinsulinemic-euglycemic clamp, the “gold standard” detection method for IR, is complicated, additional methods and techniques to detect IR are now being explored (11). Common clinical detection techniques include direct and indirect methods such as the hyperinsulinemic-euglycemic clamp technique, the Quantitative Insulin Sensitivity Check Index (QUICKI), and the homeostasis model assessment of insulin resistance (HOMA-IR). However, all these methods require specialized equipment or trained professionals, which might make it more difficult to identify IR early and manage PCOS-related metabolic conditions.
Various anthropometric measurements, such as body mass index (BMI), waist-to-height ratio (WHtR), and waist circumference (WC), can help identify types or the status of abnormal metabolism (12–14). Moreover, patients with PCOS displayed more severe IR and sex-hormone disorders; negative correlations were observed between body fat and sex hormones in patients with PCOS compared with those without PCOS (15). This suggested that body fat assessment should be more specific in PCOS management. A simple and time-saving anthropometric measurement, the WHtR refers to central obesity status (16). It has been identified as a cardiovascular disease predictor (17, 18). A population-based cross-sectional study revealed that the WHtR is closely related to diabetes, especially in women (19). Another WHtR analysis suggested that it could be a better index of prevalent cardiac events than BMI, waist-to-hip ratio (WHR), and WC (20, 21). However, there are an insufficient number of studies on WHtR and PCOS to date. A 2016 study involving a cohort of 704 eumenorrheic non-hirsute subjects and 50 women with PCOS indicated that the WHtR can reliably predict IR and metabolic syndrome in PCOS-afflicted women. This finding suggests that the WHtR might become a promising PCOS screening tool (22). Recently, the WHtR has been suggested as a representative marker for metabolic syndrome assessment in PCOS cases (23). Additionally, a cross-sectional study on 66 Indian patients with PCOS suggested that the WHtR might be a beneficial screening tool for detecting IR early in the PCOS population (24).
Hence, we performed a cross-sectional study based on a large-sample randomized controlled trial and aimed to explore the relationship between the WHtR and IR in patients with PCOS by examining the association between the WHtR and HOMA-IR. We also sought to test the accuracy and reliability of the WHtR as a marker for IR in patients with PCOS.
2 Materials and methods
2.1 Participants
This was an observational study on the association between the WHtR and metabolic dysfunction in PCOS-afflicted women. We collected data from the Polycystic Ovary Syndrome Acupuncture plus Clomiphene Trial (PCOSAct), a large-sample, randomized controlled trial in China (25). The trial was registered on ClinicalTrials.gov (NCT01573858) and chictr.org.cn (ChiCTR-TRC-12002081). The key findings (25) and protocol (25) have been published.
The inclusion criteria were: Women with PCOS who were anovulatory and were trying ovulation induction. PCOS was defined by the modified Rotterdam criteria (26). The exclusion criteria were: Women with other endocrine disorders [including Hyperprolactinemia (defined as two prolactin levels that were at least one week apart >=25 ng/mL); those with FSH levels > 15 mIU/mL; uncorrected thyroid disease patients (defined as TSH <0.2 mIU/mL or >5.5 mIU/mL); those with poorly controlled Type I or Type II diabetes (HbA1c level > 7.0%) or patients on antidiabetic medications such as metformin, insulin, thiazolidinediones, acarbose, or sulfonylureas; and those with suspected Cushing syndrome]; Patients who used hormonal or other medications including Chinese herbal prescriptions in the past 2 months; those with a miscarriage or who had given birth within 6 weeks or who were breastfeeding within the last 6 months; and those without glucose or lipid measurements were excluded. Thus, 882 PCOSAct participants were eligible for the secondary analysis. All procedures followed the ethical standards of the institutional and/or national research committees and the principles of the Declaration of Helsinki and its subsequent amendments or comparable ethical standards.
2.2 Anthropometric and laboratory measurements
The evaluation included collecting patients’ demographic profiles, anthropometric parameters, and fasting blood samples. The demographic status refers to patients’ ages. The anthropometric measurements included height, weight, blood pressure, and WC. BMI was calculated as weight (kg) divided by the square of height (m) and the WHtR was calculated as WC (cm)/height (cm). WHR was calculated as WC (cm)/hip circumference (cm). We used patients from 21 PCOSAct centers. All research assistants underwent unified training at the project’s initial stage, covering measurement techniques for height, weight, WC, hip circumference, systolic blood pressure (SBP), and diastolic blood pressure (DBP), as well as blood sample collection and storage techniques.
The specific measurement methods were as follows. (1) Height (cm) was measured with the patient barefoot, eyes looking straight ahead, arms at their sides, and their back touching a ruler. Subsequently, a horizontal plate was pressed against the head to obtain an accurate reading to the nearest 0.1 cm measurement. (2) Weight (kg) was measured after the patients removed their hats, emptied their bladders and bowels, and wore lightweight clothing. Its accuracy was ensured to the nearest 0.1 kg measurement. (3) WC (cm) was measured with an empty bladder and bowels, with arms at their sides, and normal breathing. We used a tape measure around the navel, keeping it at the central point, and measuring to the nearest 0.1 cm. (4) Hip circumference (cm) was measured with an empty bladder and bowels with arms sideways and normal breathing. A tape measure was used to measure the horizontal circumference at the widest point of the hips to the nearest 0.1 cm. (5) Blood pressure (mmHg) was measured using a mercury sphygmomanometer. After resting quietly for approximately 10 minutes, the patients sat with their upper arms exposed and their elbows positioned at the same level as their hearts. The cuff was wrapped around the upper arm and positioned 2-3 cm above the antecubital fossa. A stethoscope was placed over the brachial artery. Both SBP and DBP were measured to the nearest 1 mmHg level.
The blood collection and storage standards were as follows. We collected 20 ml of whole blood from each subject at baseline. Of this, 10 ml were placed into an EDTA anticoagulant tube and aliquoted into cryovials. We placed the other 10 ml into a clot-activating tube, followed by serum separation and aliquoting into Eppendorf (EP) tubes in a refrigerator at -20°C or -80°C. Each subject’s blood samples were independently placed in a self-sealing bag, with a blood sample storage registration card indicating the specimen information, subject’s identification number, and sampling time. Subsequently, the samples were sent to the central laboratory in batches through a cold chain company for storage at -80°C. All biomarker tests were uniformly conducted at the Radioimmunoassay Special Examination Department of Heilongjiang Provincial Hospital and the Clinical Laboratory of the First Affiliated Hospital of Heilongjiang University of Chinese Medicine to minimize potential instrument errors.
Baseline fasting circulating glucose, lipid metabolism, sex steroids, and gonadotropin levels were measured at the core laboratory in the Heilongjiang University of Chinese Medicine. This included fasting glucose, total fasting insulin, total cholesterol (TC), triglyceride (TG), lipoprotein A, high-density lipoprotein cholesterol (HDL-C), low-density lipoprotein cholesterol (LDL-C), apolipoprotein A1 (ApoA1), apolipoprotein B (ApoB), total testosterone (TT), free testosterone (FT), sex hormone binding globulin (SHBG), estradiol (E2), progesterone (P), luteinizing hormone (LH), and follicle-stimulating hormone (FSH) levels. Moreover, glucose and lipid profiles were measured by enzymatic methods using a Hitachi Biochemical Analyzer (LABOSPE CT008). All sex hormones were analyzed using a Roche Cobas 6000-E601, an electrochemiluminescent immunoassay, except FT, which was measured using a radioimmunoassay (RIA). All intra-and inter-assay coefficient variation assessments were <5% and ≤10%, respectively. The HOMA-IR levels (fasting insulin[mIU/mL]×fasting glucose[mmol/L])/22.5), free androgen index (FAI = TT (nmol/L)/[SHBG(nmol/L)]×100), and LH to FSH (LH/FSH) ratio were calculated. Participants were considered to have IR when their HOMA-IR index was R≥2.6×10-6 mol×U/L2.
2.3 Statistical analyses
All statistical analyses were done using SPSS version 25.0 software (IBM Corporation). Data were described as the mean ± standard deviation or as the median (interquartile range), or number and percentage for continuous and categorical variables, respectively. Shapiro-Wilk tests suggested that BMI, WHR, SBP, DBP, E2, P, LH, LH/FSH ratio, FAI, SHBG, fasting glucose, fasting insulin, HOMA-IR, TG, and lipoprotein A were not normally distributed. Differences among the two groups were analyzed for continuous variables using Student’s t-test and the Mann-Whitney U test for those with normal and skewed distributions, respectively. Moreover, BMI and HOMA-IR were log-transformed before Pearson’s correlation analysis was performed. Pearson’s correlation analysis helped us to assess the correlation between the WHtR and BMI (log-transformed), WC, WHR, and HOMA-IR (log-transformed). Univariate linear regression analysis determined the association between HOMA-IR (log-transformed) and the WHtR. Variables with a p<0.05 were included in the multivariate linear regression and multivariable logistic regression analyses, respectively. We used the multivariable linear regression to explore the association of WHtR with HOMA-IR (log-transformed). Furthermore, multivariable logistic regression analysis helped us to calculate the adjusted odds ratios (OR) and 95% confidence interval (CI) of WHtR for IR in different models after potential confounder adjustment. Due to the excessively large OR value observed for the WHtR in the IR logistic regression analysis, the WHtR values were multiplied by 10 to achieve precise and interpretable OR values. In the multivariable linear regression and logistic regression analyses, we did not adjust the variables in model 1. However, age, SBP, and DBP were adjusted for in model 2, and E2, LH, LH/FSH ratio, FAI, SHBG, HDL-C, LDL-C, cholesterol, and ApoB levels were adjusted for in model 3. A receiver operating characteristic (ROC) analysis was conducted for the WHtR to evaluate whether it can precisely discriminate for IR in patients with PCOS. All p-values were two-sided, and p<0.05 was considered statistically significant.
3 Results
We included 882 PCOSAct participants, of which 458 (51.9%) were diagnosed with IR. The mean ages (± standard deviation) were 28.05 (± 3.39) years and 27.84 (± 3.23) years for patients with IR and without IR, respectively (p=0.341).
3.1 Anthropometric and biochemical characteristics of the patients with PCOS
Table 1 shows the anthropometric and biochemical characteristics of the patients with PCOS categorized by the existence of IR.
The patients with PCOS and IR exhibited significantly higher anthropometric and metabolic parameters than those without IR. They displayed increased BMI (p<0.001), WC (p<0.001), WHtR (p<0.001), WHR (p<0.001), SBP (p<0.001), DBP (p<0.001), FAI (p<0.001), fasting glucose (p<0.001), fasting insulin (p<0.001), HOMA-IR (p<0.001), TC (p<0.001), TG (p<0.001), LDL-C (p<0.001), and ApoB (P<0.001) values but decreased E2 (p=0.015), LH (p<0.001), LH/FSH ratio (p<0.001), SHBG (p<0.001), and HDL-C (p<0.001) levels, respectively.
However, no significant differences were observed between the two groups in P (1.72[1.25] vs. 1.76[1.17]; p=0.207), FSH (5.96 ± 1.70 vs. 6.17 ± 1.61; p=0.058), TT (1.67 ± 0.67 vs. 1.65 ± 0.61; p=0.572), FT (2.32 ± 0.79 vs. 2.23 ± 0.88; p=0.130), lipoprotein A (102.05[87.0] vs. 100.70[83.70]; p=0.888), and ApoA1 (1.51 ± 0.31 vs. 1.51 ± 0.32; p=0.857) levels, respectively.
3.2 Correlations of the WHtR with the anthropometric and HOMA-IR data
Pearson’s correlation analysis helped us to assess the correlations between the WHtR and BMI (log-transformed), WC, WHR (log-transformed), and HOMA-IR (log-transformed), as shown in Figure 1. The WHtR in women with PCOS was positively correlated with BMI (r=0.812; p<0.001), WC (r=0.970; p<0.001), WHR (r=0.776; p<0.001), and HOMA-IR (r=0.484; p<0.001).
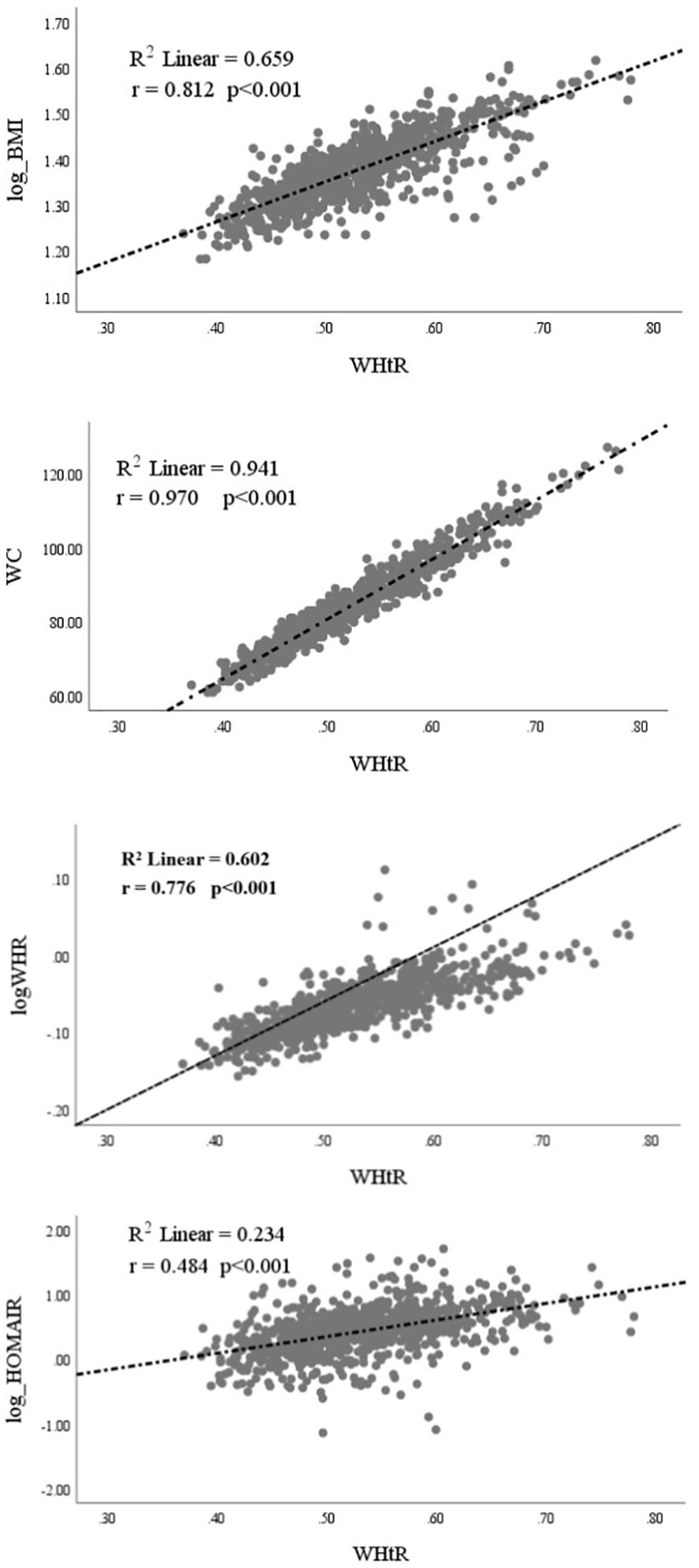
Figure 1. Correlation of Waist-to-Height Ratio (WHtR) with Log[Body Mass Index (BMI)], Waist Circumference (WC), Log[Waist-to-Hip Ratio (WHR)], and Log(HOMA-IR) for Insulin Resistance.
In order to further explore the associations between the WHtR and HOMA-IR (log-transformed), multivariable linear regression analysis was used with potential confounding factor adjustments (Table 2). In model 1, without any adjustment, the WHtR was significantly associated with HOMA-IR, and the coefficient (95% CI) was 2.57 (2.26-2.88). In model 2 (with the adjustments for age, SBP, and DBP) and model 3 (with additional adjustments for E2, LH, LH/FSH ratio, FAI, SHBG, TC, TG, LDL-c, HDL-C, and ApoB), the WHtR was significantly associated with HOMA-IR. The resultant coefficients (95% CI) were 2.45 (2.13-2.77) and 1.98 (1.49-2.46), respectively.
3.3 Association of the WHtR with IR
Additionally, multivariate logistic regression analysis helped us to identify the association between the WHtR and IR. As an excessively large OR value (>999.999) was observed for the WHtR in the IR logistic regression analysis, we multiplied the WHtR values by 10 to achieve precise and interpretable OR values. In model 1, without any confounding factor adjustment, the OR (95% CI) was 5.97 (4.50-7.92; p<0.001). In models 2 and 3, with the same adjustments as those in multivariate linear regression analysis, the association between WHtR (×10) and IR was statistically significant: the ORs (95% CI) were 5.69 (4.27-7.60; p<0.001) and 3.15 (2.27-4.37; p<0.001), respectively. This suggests that after adjusting for biomarkers relevant to IR, the risk of IR increased by 3.15 for every 0.1 unit increase in the WHtR.
ROC analysis helped us to determine the suggested WHtR cut-off values for IR. Figure 2 shows that the WHtR performed significantly well in classifying women with PCOS as having IR. With an area under the ROC curve (AUC, 95% CI) of 0.690 (0.655-0.725; p<0.001), the WHtR outperformed the WHR. The AUC [95% CI] for the WHtR was 0.777 (0.747-0.807; p<0.001), comparable to BMI and WC, which had AUCs (95% CI) of 0.799 (0.771-0.828; p<0.001) and 0.786 (0.756-0.816; p<0.001), respectively. The best cut-off point for the WHtR in the patients with PCOS was 0.519 (Youden index = 0.433). The sensitivity, specificity, and cut-off values of the WHtR, BMI, WC, and WHR for IR prediction are listed in Figure 2.
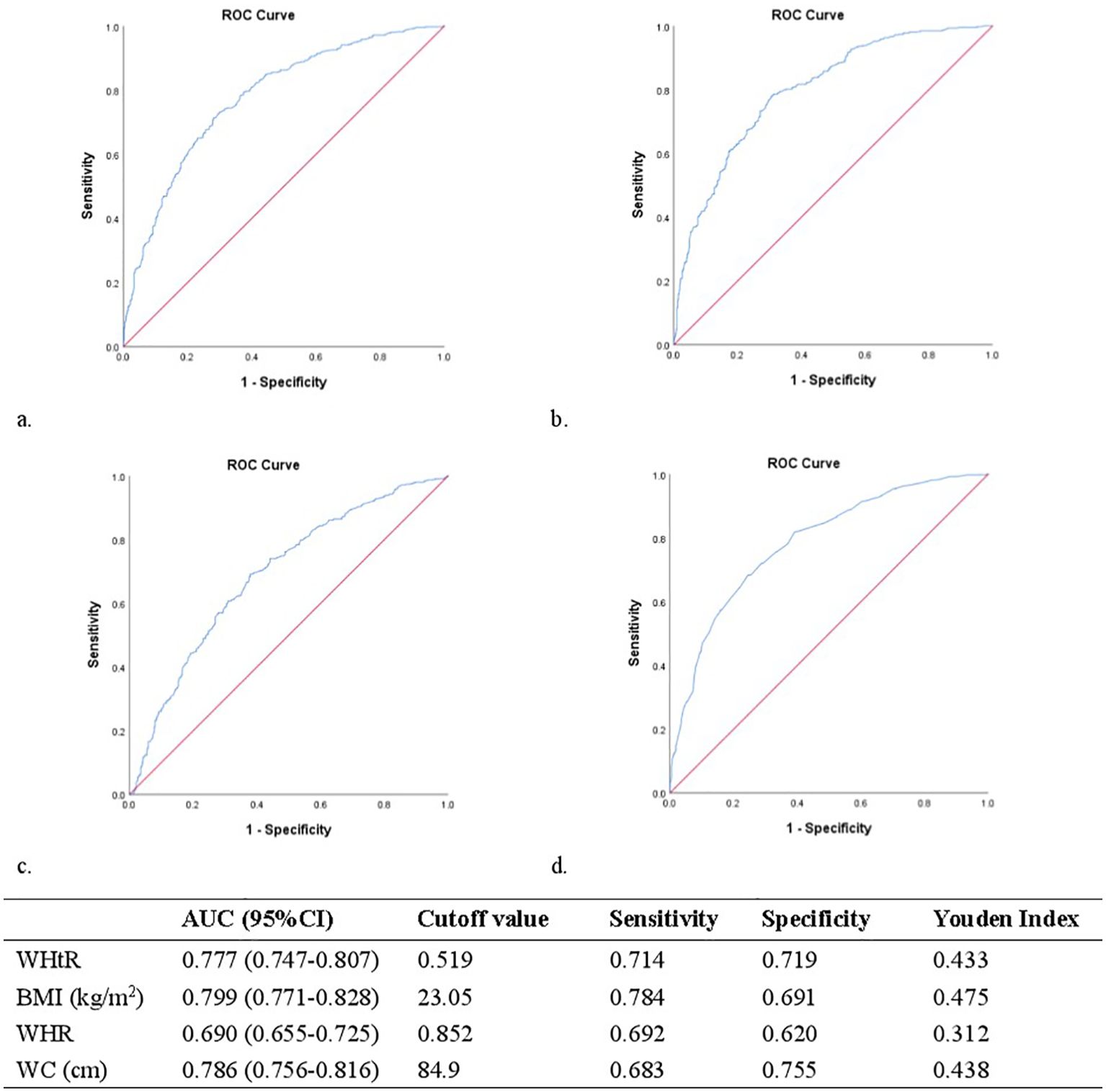
Figure 2. (A) Receiver operating characteristic curves and area under receiver operating characteristic curves for the detection of IR using WHtR. (B) Receiver operating characteristic curves and area under receiver operating characteristic curves for the detection of IR using BMI. (C) Receiver operating characteristic curves and area under receiver operating characteristic curves for the detection of IR using WHR. (D) Receiver operating characteristic curves and area under receiver operating characteristic curves for the detection of IR using WC.
Pearson’s correlation helped us to explore the association between several insulin resistance indexes, including fasting glucose, fasting insulin, and HOMA-IR, as well as anthropometric markers, including the WHtR, WC, and WHR (Table 3). Our results indicated that the WHtR exhibited similar correlations with fasting glucose, fasting insulin, and HOMA-IR, as well as WC and the WHR. Notably, the WHtR performed similarly to BMI and WC whereas the WHR was poorly associated when compared to the WHtR and WC. In the IR logistic regression analysis, the WHtR displayed a higher OR than BMI, WC, and WHR, suggesting that WHtR modifications have a greater impact on IR.
3.4 Comparison of IR condition based on the WHtR of patients with PCOS with a normal BMI, normal WC, or concurrent normal BMI and WC
As a previous study demonstrated that various IR predictors may vary among Chinese women with PCOS based on their body type (27), we conducted a comparison of IR conditions based on differing WHtRs in patients with PCOS with a normal BMI, or normal WC, or concurrent normal BMI and WC.
For patients with PCOS with a normal BMI, patients with IR were more likely to have a higher WHtR than those without IR (35.1% vs. 17.7%; p<0.001), and similar results were also found in patients with PCOS with a normal WC (16.6% vs. 7.5%; p=0.003) and patients with PCOS with concurrent normal BMI and WC (12.3% vs. 5.9%; p=0.043) (Table 4).
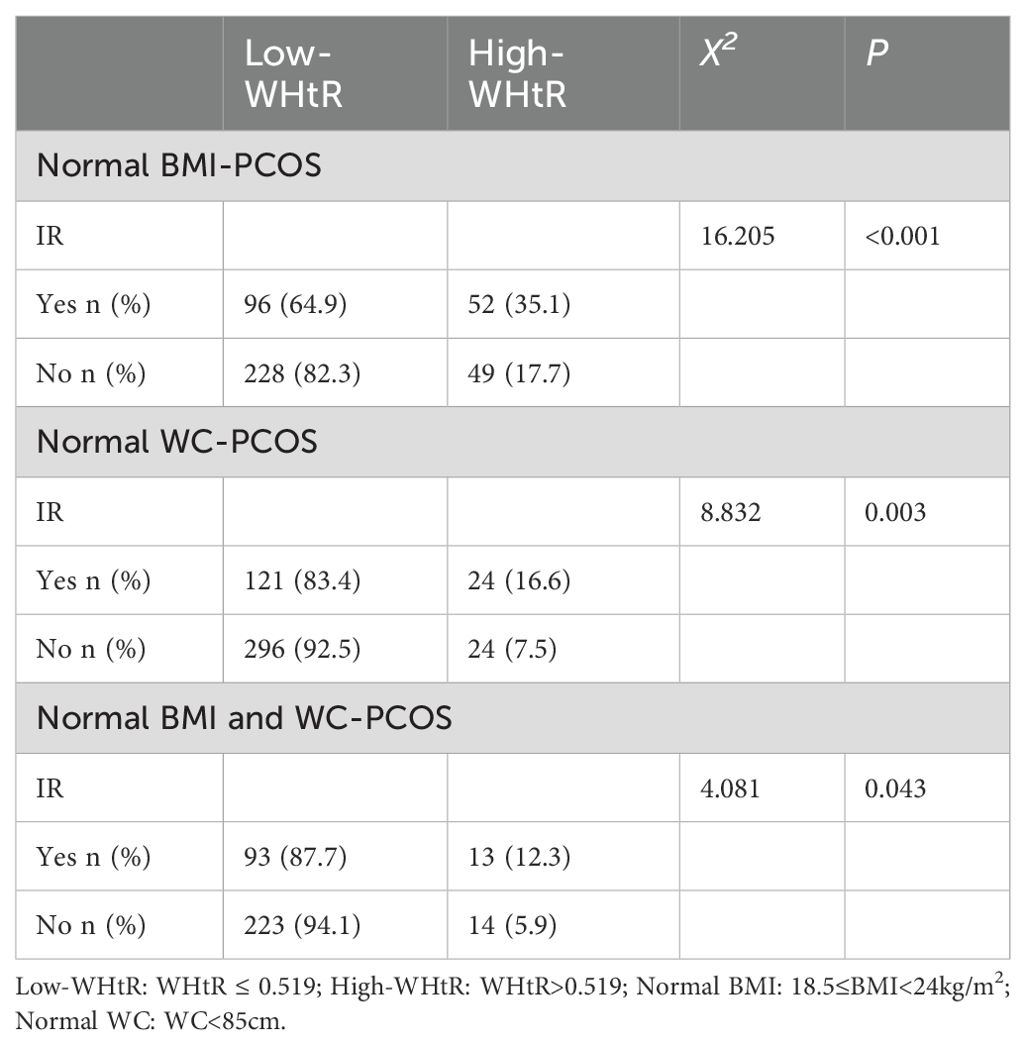
Table 4. Comparison of IR condition with different WHtRs in patients with PCOS with a normal BMI, normal WC, or concurrent normal BMI and WC.
4 Discussion
We showed that the WHtR was independently associated with IR in patients with PCOS. In our study, we found that the prevalence rate of IR diagnosed using the HOMA-IR >2.6 was 51.9% in the patients with PCOS; this value was smaller than 74.9%, the prevalence assessed by hyperinsulinemic-euglycemic clamp (28). A 2013 study showed that the IR prevalence was lower in Chinese patients with PCOS (29).
The IR group’s levels of fasting glucose, fasting insulin, SBP, DBP, TC, TG, LDL-C, ApoB, and anthropometric indexes (BMI, WHtR, WHR, and WC) were significantly higher than the patients with PCOS but without IR. Moreover, a positive correlation was also observed between BMI, WHR, WC, HOMA-IR, and the WHtR. This was consistent with previous studies (30, 31). Furthermore, the WHtR was significantly associated with IR after potential confounding factor adjustment in multivariable linear regression and logistic regression analyses, respectively. Finally, ROC analysis demonstrated that when the WHtR became 0.519, it was very effective as an IR marker in patients with PCOS. Though IR develops independently from PCOS, it was found to induce the endocrine and reproductive traits of PCOS (32); this result was similar to our findings. In this study, women with PCOS and IR had lower values of E2 and SHBG as well as higher FAI levels. High androgen and low estrogen levels in PCOS are linked to infertility (33). However, we observed that patients with PCOS and IR had lower LH and LH/FSH ratios than those without IR. This is consistent with another observational Chinese study (34). Matthew et al. found a “metabolic” subtype of PCOS that was characterized by enhanced BMI, glucose, and insulin levels with lower SHBG and LH levels in an unsupervised, phenotypic clustering analysis with similar features as our IR-PCOS patients (35). Moreover, decreased LH concentration and LH/FSH ratio in overweight PCOS cases were more prevalent compared with non-overweight patients with PCOS. Thus, obesity can reduce LH pulse amplitude (36).
IR is significantly associated with all-cause and cardiovascular mortalities and is an important factor in regulating metabolic syndrome and diabetes (37, 38). Thus, its early identification is crucial for cardiovascular disease management in patients with PCOS. Currently, the most commonly used parameter for IR detection is the Homeostatic Model Assessment (HOMA). Other laboratory indexes include QUICKI, the Matsuda Index, and the Insulin Secretion-Sensitivity Index-2 (ISSI-2). All these have different values that vary for different ages, sexes, populations, and ethics (39). However, all these examinations require specialized equipment and trained professionals. In recent studies, anthropometric measurements such as BMI and WHR have been shown to be cost-effective tools for IR prediction and detection (40, 41). A novel anthropometric measurement, the WHtR, is an effective and precise anthropometric index to predict IR (42). Additionally, the WHtR was significantly associated with IR among anthropometric indicators in obese individuals (43). Another cross-sectional analysis of 2,231 participants with normal or impaired fasting glucose levels or type 2 diabetes indicated that the WHtR performed well in IR prediction, including in the subgroups that had impaired fasting glucose levels or type 2 diabetes, respectively (44). In our study, the WHtR was a powerful indicator for detecting IR in women with PCOS; the optimal cut-off point was 0.519 for patients with PCOS of reproductive age. Within the normal BMI populations, i.e., normal WC or normal BMI and WC, increased WHtR was significantly associated with IR, suggesting that the WHtR might identify IR earlier than BMI and WC, thereby enabling patients to adopt proactive preventive measures (Table 4). Unlike the BMI, the WHtR does not involve unit conversion and WC and height can be measured in the same unit. This makes the value more accurate. Furthermore, the WHtR varies between the sexes and among sexual maturation stages (45). However, studies on the WHtR and PCOS are inadequate, and additional studies are needed to prove the relationship in patients with PCOS and determine the exact cut-off points in different phenotypes. Notably, future comparisons between obese women with PCOS and obese women without PCOS could better illustrate the utility of WHtR in the PCOS population. Since the majority of our study participants were Han Chinese, variations in the height among individuals from diverse racial and ethnic backgrounds may limit our results’ applicability. Thus, future research endeavors should include extensive and diverse participants from various racial and ethnic backgrounds.
Our study had a few limitations. First, we diagnosed IR based on HOMA-IR instead of hyperinsulinemic-euglycemic clamp due to its complicated procedures, even though hyperinsulinemic-euglycemic clamp is the “gold standard” for IR assessment. Second, we could not provide an interpretation of the causality of associations due to our cross-sectional design. Third, a few social or lifestyle factors that might have influenced IR were not included as this was a secondary analysis based on PCOSAct. Finally, excluding the body composition analysis limited our results, thereby allowing the potential for further refinement.
5 Conclusion
In summary, early identification of IR is helpful for patients with PCOS to manage endocrine and metabolic disorders as well as reduce long-term health risks. This study showed that the WHtR was positively correlated with IR in PCOS-afflicted women. Thus, the WHtR could become a simple, practical, and reliable measure for identifying IR early in patients with PCOS.
Data availability statement
The original contributions presented in the study are included in the article/supplementary material. Further inquiries can be directed to the corresponding author.
Ethics statement
The studies involving humans were approved by Ethics committee, First Affiliated Hospital, Heilongjiang University of Chinese Medicine. The studies were conducted in accordance with the local legislation and institutional requirements. The participants provided their written informed consent to participate in this study.
Author contributions
MZ: Methodology, Writing – original draft. KW: Methodology, Writing – original draft. JF: Supervision, Writing – original draft. YL: Methodology, Supervision, Writing – original draft. MG: Methodology, Supervision, Writing – review & editing. YW: Methodology, Supervision, Writing – review & editing. XW: Supervision, Writing – review & editing.
Funding
The author(s) declare financial support was received for the research, authorship, and/or publication of this article. This work is supported by (1) the Project of Heilongjiang Province Innovation Team “TouYan” (2); the Heilongjiang Provincial Clinical Research Centre for Ovary Diseases; and (3) the Key discipline construction fund on Gynecology of Traditional Chinese Medicine from the Department of Education of Heilongjiang Province.
Acknowledgments
The Steering Committee members included Tai-Xiang Wu, Elisabet Stener-Victorin and Heping Zhang, and Richard S. Legro (Chair). The Data and Safety Monitoring Board (DSMB) members included Esther Eisenberg, Wei-Liang Weng, Su-Lun Sun, Wei Zou and Zi-Dan Chen, and Robert Rebar (Chair). Principal investigators participated in patient recruitment at local sites. Other personnel with administrative resource supports included Song-Jiang Liu, Gui-Yuan Wang, Yan-Qiu Du, Yang Xia, Shu-Lai Li, Ke-Qiu Zhang and Jian-Hua Shen. Yan Li, Wen-Juan Shen, Wei Li and Jing Cong were involved in protocol preparation and blood sample management in Harbin office and core laboratory.
Conflict of interest
The authors declare that the research was conducted in the absence of any commercial or financial relationships that could be construed as a potential conflict of interest.
Generative AI statement
The author(s) declare that no Generative AI was used in the creation of this manuscript.
Publisher’s note
All claims expressed in this article are solely those of the authors and do not necessarily represent those of their affiliated organizations, or those of the publisher, the editors and the reviewers. Any product that may be evaluated in this article, or claim that may be made by its manufacturer, is not guaranteed or endorsed by the publisher.
References
1. Lizneva D, Suturina L, Walker W, Brakta S, Gavrilova-Jordan L, Azziz R. Criteria, prevalence, and phenotypes of polycystic ovary syndrome. Fertil Steril. (2016) 106:6–15. doi: 10.1016/j.fertnstert.2016.05.003
2. Armanini D, Boscaro M, Bordin L, Sabbadin C. Controversies in the pathogenesis, diagnosis and treatment of PCOS: focus on insulin resistance, inflammation, and hyperandrogenism. Int J Mol Sci. (2022) 23:4110. doi: 10.3390/ijms23084110
3. Osibogun O, Ogunmoroti O, Michos ED. Polycystic ovary syndrome and cardiometabolic risk: Opportunities for cardiovascular disease prevention. Trends Cardiovasc Med. (2020) 30:399–404. doi: 10.1016/j.tcm.2019.08.010
4. Sanchez-Garrido MA, Tena-Sempere M. Metabolic dysfunction in polycystic ovary syndrome: Pathogenic role of androgen excess and potential therapeutic strategies. Mol Metab. (2020) 35:100937. doi: 10.1016/j.molmet.2020.01.001
5. Lee S-H, Park S-Y, Choi CS. Insulin resistance: from mechanisms to therapeutic strategies. Diabetes Metab J. (2022) 46:15–37. doi: 10.4093/dmj.2021.0280
6. Greenwood EA, Huddleston HG. Insulin resistance in polycystic ovary syndrome: concept versus cutoff. Fertil Steril. (2019) 112:827–8. doi: 10.1016/j.fertnstert.2019.08.100
7. Li M, Chi X, Wang Y, Setrerrahmane S, Xie W, Xu H. Trends in insulin resistance: insights into mechanisms and therapeutic strategy. Signal Transduct Target Ther. (2022) 7:216. doi: 10.1038/s41392-022-01073-0
8. Zhao H, Zhang J, Cheng X, Nie X, He B. Insulin resistance in polycystic ovary syndrome across various tissues: an updated review of pathogenesis, evaluation, and treatment. J Ovarian Res. (2023) 16:9. doi: 10.1186/s13048-022-01091-0
9. Chen Y, Zheng X, Ma D, Zheng S, Han Y, Su W, et al. Neck circumference is a good predictor for insulin resistance in women with polycystic ovary syndrome. Fertil Steril. (2021) 115:753–60. doi: 10.1016/j.fertnstert.2020.07.027
10. Rahmatnezhad L, Moghaddam-Banaem L, Behroozi-Lak T, Shiva A, Rasouli J. Association of insulin resistance with polycystic ovary syndrome phenotypes and patients’ characteristics: a cross-sectional study in Iran. Reprod Biol Endocrinol. (2023) 21:113. doi: 10.1186/s12958-023-01160-z
11. Polak K, Czyzyk A, Simoncini T, Meczekalski B. New markers of insulin resistance in polycystic ovary syndrome. J Endocrinol Invest. (2017) 40:1–8. doi: 10.1007/s40618-016-0523-8
12. Wu L, Zhu W, Qiao Q, Huang L, Li Y, Chen L. Novel and traditional anthropometric indices for identifying metabolic syndrome in non-overweight/obese adults. Nutr Metab (Lond). (2021) 18:3. doi: 10.1186/s12986-020-00536-x
13. Zaid M, Ameer F, Munir R, Rashid R, Farooq N, Hasnain S, et al. Anthropometric and metabolic indices in assessment of type and severity of dyslipidemia. J Physiol Anthropol. (2017) 36:19. doi: 10.1186/s40101-017-0134-x
14. Perona JS, Schmidt Rio-Valle J, Ramírez-Vélez R, Correa-Rodríguez M, Fernández-Aparicio Á, González-Jiménez E. Waist circumference and abdominal volume index are the strongest anthropometric discriminators of metabolic syndrome in Spanish adolescents. Eur J Clin Invest. (2019) 49:e13060. doi: 10.1111/eci.13060
15. Zhang H, Wang W, Zhao J, Jiao P, Zeng L, Zhang H, et al. Relationship between body composition, insulin resistance, and hormonal profiles in women with polycystic ovary syndrome. Front Endocrinol (Lausanne). (2022) 13:1085656. doi: 10.3389/fendo.2022.1085656
16. Abdi Dezfouli R, Mohammadian Khonsari N, Hosseinpour A, Asadi S, Ejtahed H-S, Qorbani M. Waist to height ratio as a simple tool for predicting mortality: a systematic review and meta-analysis. Int J Obes (Lond). (2023) 47:1286–301. doi: 10.1038/s41366-023-01388-0
17. Choi JR, Koh SB, Choi E. Waist-to-height ratio index for predicting incidences of hypertension: the ARIRANG study. BMC Public Health. (2018) 18:767. doi: 10.1186/s12889-018-5662-8
18. Feng Q, Bešević J, Conroy M, Omiyale W, Woodward M, Lacey B, et al. Waist-to-height ratio and body fat percentage as risk factors for ischemic cardiovascular disease: a prospective cohort study from UK Biobank. Am J Clin Nutr. (2024) 119:1386–96. doi: 10.1016/j.ajcnut.2024.03.018
19. Zhang F-L, Ren J-X, Zhang P, Jin H, Qu Y, Yu Y, et al. Strong association of waist circumference (WC), body mass index (BMI), waist-to-height ratio (WHtR), and waist-to-hip ratio (WHR) with diabetes: A population-based cross-sectional study in jilin province, China. J Diabetes Res. (2021) 2021:8812431. doi: 10.1155/2021/8812431
20. Pasdar Y, Moradi S, Moludi J, Saiedi S, Moradinazar M, Hamzeh B, et al. Waist-to-height ratio is a better discriminator of cardiovascular disease than other anthropometric indicators in Kurdish adults. Sci Rep. (2020) 10:16228. doi: 10.1038/s41598-020-73224-8
21. Lu P, Zhu L, Hu L, Bao H, Huang X, Zhou W, et al. Association of waist-to-height ratio with hypertension and its subtypes in southern China. J Hum Hypertens. (2022) 36:775–80. doi: 10.1038/s41371-021-00566-9
22. Behboudi-Gandevani S, Ramezani Tehrani F, Cheraghi L, Azizi F. Could “a body shape index” and “waist to height ratio” predict insulin resistance and metabolic syndrome in polycystic ovary syndrome? Eur J Obstet Gynecol Reprod Biol. (2016) 205:110–4. doi: 10.1016/j.ejogrb.2016.08.011
23. Kałużna M, Czlapka-Matyasik M, Kompf P, Moczko J, Wachowiak-Ochmańska K, Janicki A, et al. Lipid ratios and obesity indices are effective predictors of metabolic syndrome in women with polycystic ovary syndrome. Ther Adv Endocrinol Metab. (2022) 13:20420188211066699. doi: 10.1177/20420188211066699
24. Bhattacharya K, Sengupta P, Dutta S, Chaudhuri P, Das Mukhopadhyay L, Syamal AK. Waist-to-height ratio and BMI as predictive markers for insulin resistance in women with PCOS in Kolkata, India. Endocrine. (2021) 72:86–95. doi: 10.1007/s12020-020-02555-3
25. Kuang H, Li Y, Wu X, Hou L, Wu T, Liu J, et al. Acupuncture and clomiphene citrate for live birth in polycystic ovary syndrome : study design of a randomized controlled trial. Evid Based Complement Alternat Med. (2013) 2013. doi: 10.1155/2013/527303
26. Rotterdam ESHRE/ASRM-Sponsored PCOS consensus workshop group. Revised 2003 consensus on diagnostic criteria and long-term health risks related to polycystic ovary syndrome (PCOS). Hum Reprod. (2004) 19:41–7. doi: 10.1093/humrep/deh098
27. Huang X, Wang Q, Liu T, Pei T, Liu D, Zhu H, et al. Body fat indices as effective predictors of insulin resistance in obese/non-obese polycystic ovary syndrome women in the Southwest of China. Endocrine. (2019) 65:81–5. doi: 10.1007/s12020-019-01912-1
28. Muscogiuri G, Barrea L, Caprio M, Ceriani F, Chavez AO, El Ghoch M, et al. Nutritional guidelines for the management of insulin resistance. Crit Rev Food Sci Nutr. (2022) 62:6947–60. doi: 10.1080/10408398.2021.1908223
29. Zhang HY, Guo CX, Zhu FF, Qu PP, Lin WJ, Xiong J. Clinical characteristics, metabolic features, and phenotype of Chinese women with polycystic ovary syndrome: a large-scale case-control study. Arch Gynecol Obstet. (2013) 287:525–31. doi: 10.1007/s00404-012-2568-z
30. Nevill AM, Stewart AD, Olds T, Duncan MJ. A new waist-to-height ratio predicts abdominal adiposity in adults. Res Sports Med. (2020) 28:15–26. doi: 10.1080/15438627.2018.1502183
31. Lo K, Wong M, Khalechelvam P, Tam W. Waist-to-height ratio, body mass index and waist circumference for screening paediatric cardio-metabolic risk factors: a meta-analysis. Obes Rev. (2016) 17:1258–75. doi: 10.1111/obr.12456
32. Moghetti P, Tosi F. Insulin resistance and PCOS: chicken or egg? J Endocrinol Invest. (2021) 44:233–44. doi: 10.1007/s40618-020-01351-0
33. Yang J, Chen C. Hormonal changes in PCOS. J Endocrinol. (2024) 261:e230342. doi: 10.1530/JOE-23-0342
34. Wang S. Characteristics and influence factors of LH and LH/FSH in patients with phlegm-dampness type/non phlegm-dampness type polycystic ovary syndrome. Clin J Chin Med. (2021) 13:21–5.
35. Dapas M, Lin FTJ, Nadkarni GN, Sisk R, Legro RS, Urbanek M, et al. Distinct subtypes of polycystic ovary syndrome with novel genetic associations: An unsupervised, phenotypic clustering analysis. PloS Med. (2020) 17:e1003132. doi: 10.1371/journal.pmed.1003132
36. Yu J, Zhou Y, Ding J, Zhang D, Yu C, Huang H. Characteristics and possible mechanisms of metabolic disorder in overweight women with polycystic ovary syndrome. Front Endocrinol (Lausanne). (2022) 13:970733. doi: 10.3389/fendo.2022.970733
37. Duan M, Zhao X, Li S, Miao G, Bai L, Zhang Q, et al. Metabolic score for insulin resistance (METS-IR) predicts all-cause and cardiovascular mortality in the general population: evidence from NHANES 2001-2018. Cardiovasc Diabetol. (2024) 23:243. doi: 10.1186/s12933-024-02334-8
38. Yaribeygi H, Farrokhi FR, Butler AE, Sahebkar A. Insulin resistance: Review of the underlying molecular mechanisms. J Cell Physiol. (2019) 234:8152–61. doi: 10.1002/jcp.27603
39. Placzkowska S, Pawlik-Sobecka L, Kokot I, Piwowar A. Indirect insulin resistance detection: Current clinical trends and laboratory limitations. BioMed Pap Med Fac Univ Palacky Olomouc Czech Repub. (2019) 163:187–99. doi: 10.5507/bp.2019.021
40. Alptekin H, Çizmecioğlu A, Işık H, Cengiz T, Yildiz M, Iyisoy MS. Predicting gestational diabetes mellitus during the first trimester using anthropometric measurements and HOMA-IR. J Endocrinol Invest. (2016) 39:577–83. doi: 10.1007/s40618-015-0427-z
41. Severeyn E, Wong S, Herrera H, Altuve M. Anthropometric measurements for assessing insulin sensitivity on patients with metabolic syndrome, sedentaries and marathoners. Annu Int Conf IEEE Eng Med Biol Soc. (2015) 2015:4423–6. doi: 10.1109/EMBC.2015.7319376
42. Silva MIB, Lemos CC da S, Torres MRSG, Bregman R. Waist-to-height ratio: an accurate anthropometric index of abdominal adiposity and a predictor of high HOMA-IR values in nondialyzed chronic kidney disease patients. Nutrition. (2014) 30:279–85. doi: 10.1016/j.nut.2013.08.004
43. Jamar G, de Almeida FR, Gagliardi A, Sobral MR, Ping CT, Sperandio E, et al. Evaluation of waist-to-height ratio as a predictor of insulin resistance in non-diabetic obese individuals. A cross-sectional study Sao Paulo Med J. (2017) 135:462–8. doi: 10.1590/1516-3180.2016.0358280417
44. Lechner K, Lechner B, Crispin A, Schwarz PEH, von Bibra H. Waist-to-height ratio and metabolic phenotype compared to the Matsuda index for the prediction of insulin resistance. Sci Rep. (2021) 11:8224. doi: 10.1038/s41598-021-87266-z
Keywords: anthropometric measurements, insulin resistance, polycystic ovary syndrome, prediction, waist-to-height ratio
Citation: Zhu M, Wang K, Feng J, Liu Y, Guan M, Wang Y and Wu X (2024) The waist-to-height ratio is a good predictor for insulin resistance in women with polycystic ovary syndrome. Front. Endocrinol. 15:1502321. doi: 10.3389/fendo.2024.1502321
Received: 26 September 2024; Accepted: 18 November 2024;
Published: 09 December 2024.
Edited by:
Zhiqin Bu, Zhengzhou University, ChinaReviewed by:
Skand Shekhar, National Institutes of Health (NIH), United StatesHui He, First Affiliated Hospital of Zhengzhou University, China
Copyright © 2024 Zhu, Wang, Feng, Liu, Guan, Wang and Wu. This is an open-access article distributed under the terms of the Creative Commons Attribution License (CC BY). The use, distribution or reproduction in other forums is permitted, provided the original author(s) and the copyright owner(s) are credited and that the original publication in this journal is cited, in accordance with accepted academic practice. No use, distribution or reproduction is permitted which does not comply with these terms.
*Correspondence: Xiaoke Wu, eGlhb2tld3UyMDAyQHZpcC5zaW5hLmNvbQ==