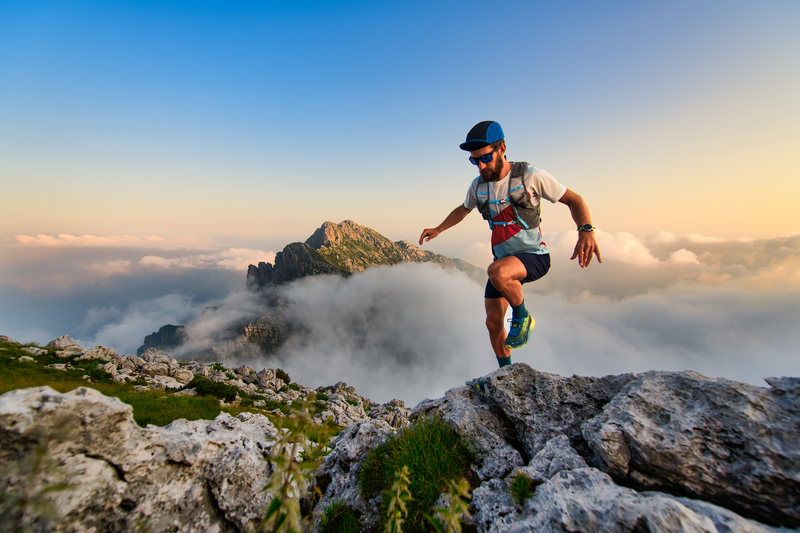
95% of researchers rate our articles as excellent or good
Learn more about the work of our research integrity team to safeguard the quality of each article we publish.
Find out more
ORIGINAL RESEARCH article
Front. Endocrinol. , 08 November 2024
Sec. Clinical Diabetes
Volume 15 - 2024 | https://doi.org/10.3389/fendo.2024.1501690
Background: We aimed to assess temporal trends in type 2 diabetes mellitus (T2DM)-related deaths and disability-adjusted life years (DALYs) at global and cross-social demographic index (SDI) levels, using data from the Global Burden of Disease (GBD) in 2021.
Methods: We used geospatial mapping to visualize the global distribution of T2DM-related mortality and DALYs in 2021. Joinpoint regression assessed annual and average percent changes in DALYs and deaths from 1990 to 2021 across SDI regions. Age-period-cohort modeling examined the effects of age, period, and cohort on trends. Decomposition analysis evaluated the impact of population growth, aging, and epidemiological changes on DALY trends. A stratified projection forecasted future T2DM burden by age and sex from 2020 to 2044.
Results: T2DM-related mortality and DALYs were highest in low-SDI regions. Globally, T2DM-related deaths and DALYs have increased, with the most rapid rise in low and low-middle SDI regions, driven by population growth and epidemiological shifts. High-SDI countries showed a slower increase in DALYs, influenced more by aging. Age-period-cohort analysis indicated higher DALY rates in later birth cohorts and recent periods, especially in high-SDI regions. Future projections show a significant increase in the 70-74 age group and a gradual rise in other age groups.
Conclusion: The burden of T2DM is projected to continue increasing, especially in low-SDI and low-middle SDI regions, where population growth and epidemiological shifts are the main contributors. This underscores the need for targeted, region-specific healthcare policies, preventive strategies, and age-specific interventions to address the increasing T2DM burden globally.
Type 2 diabetes mellitus (T2DM) has emerged as one of the most prevalent chronic diseases worldwide, with significant implications for global public health (1). Characterized by insulin resistance (2) and relative insulin deficiency (3), T2DM leads to numerous long-term complications, including cardiovascular disease (4), kidney failure (5), neuropathy (6), and retinopathy (7). The global rise in T2DM incidence has been closely associated with rapid lifestyle changes, population growth, and increased longevity, particularly in developing countries undergoing urbanization and industrialization (8, 9).
The burden of T2DM is often measured through mortality and disability-adjusted life years (DALYs) (10), which reflect both premature mortality and the years lived with disability (11). DALYs provide a comprehensive metric to assess the total impact of T2DM, making them critical for the formulation of healthcare policies and intervention strategies. Global trends indicate substantial variations in T2DM-related mortality and DALYs, largely driven by socio-economic and demographic factors (10). The socio-demographic index (SDI), which combines income, education, and fertility rates, has become a key framework for understanding disease burden across countries at different stages of development (12, 13).
In the context of global health transitions, there has been a marked shift from infectious diseases to non-communicable diseases (NCDs) like T2DM, especially as populations age (14). This epidemiological transition is altering the landscape of global health, with non-communicable diseases becoming the leading cause of morbidity and mortality (15). Despite the increasing recognition of this transition, comprehensive assessments of how population dynamics, aging, and epidemiological changes influence T2DM-related deaths and DALYs across different SDI levels remain limited. Understanding the relative contributions of age, period, and birth cohort effects to these trends is critical for projecting future disease burden and shaping effective public health interventions.
To address this gap, we conducted a detailed analysis of the temporal trends in T2DM-related DALYs and mortality from 1990 to 2021, with a focus on global and regional patterns across different SDI levels. We applied advanced epidemiological techniques, including Joinpoint regression analysis to evaluate changes in T2DM burden over time, and age-period-cohort modeling to disentangle the effects of aging, period, and cohort dynamics. Additionally, a decomposition analysis was performed to quantify the contributions of population growth, aging, and epidemiological shifts to the observed trends in DALYs.
This comprehensive approach allows us to identify the key drivers of the rising global T2DM burden, offering insights that are crucial for the development of tailored healthcare policies. By understanding the differential impact of population aging in high-SDI regions and rapid population growth in low- and middle-SDI regions, our study provides a framework for region-specific interventions. These interventions can help mitigate the future burden of T2DM and its associated complications, especially in the most vulnerable populations.
In this study, we utilized data from the Global Burden of Disease (GBD) study, managed by the Institute for Health Metrics and Evaluation (IHME). The GBD database is an annually updated global health database that encompasses a wide array of diseases and associated health metrics (10, 16).
Our primary data on T2DM-related mortality and DALYs were extracted from the Global Burden of Disease Collaborative Network and the Global Burden of Disease Study 2021 results. These sources provide comprehensive, age-standardized rates of T2DM mortality and DALYs, which are cataloged by age, sex, year, and SDI region, and are publicly accessible through IHME’s various visualization tools (https://vizhub.healthdata.org/gbd-results/). We specifically analyzed data spanning from 1990 to 2021, focusing on global trends and projections up to 2044, which are also derived from these datasets. To provide a more thorough explanation of the steps we took to ensure data accuracy and reliability, we have included additional details in Supplementary Material 1.
To evaluate the temporal trends and forecast the future burden of T2DM, we employed several statistical and modeling techniques:
This method was used to analyze changes in T2DM DALYs and mortality rates over time. It identifies points where significant changes in trend occur and estimates the annual percent change and average annual percent change (AAPC).
To disentangle the effects of age, period, and cohort on T2DM trends, Age-period-cohort modeling was applied. This model helps in understanding how different cohorts (birth years), periods (calendar years), and ages influence the rates of T2DM.
This approach quantifies the contributions of population growth, aging, and epidemiological changes to the observed trends in DALYs. This method helps in understanding the relative contributions of these factors to the changing burden of T2DM.
The reasons for our choice of age-period-cohort modeling are presented in Supplementary Material 2.
The SDI is a composite indicator that measures a region’s socio-economic status based on income, education, and fertility rates. For this study, countries and regions were categorized into quintiles based on their SDI values: low (SDI <20th percentile), low-middle (20-39th), middle (40-59th), high-middle (60-79th), and high (SDI ≥80th percentile). This categorization allows for the analysis of T2DM trends across different socio-economic contexts.
Projections of T2DM burden up to 2044 were conducted using Nordpred prediction model, as implemented in the GBD study. This method utilizes historical data to predict future trends, taking into account current epidemiological and demographic changes.
To address the inherent variability in DALY rates and model predictions, we implemented a robust uncertainty analysis using bootstrap resampling techniques. This approach helps quantify the uncertainty surrounding our estimates of DALY increases and mortality rates, providing more reliable results for interpretation. We assumed that the DALY rates followed log-normal distributions based on the nature of the data, where values are positive and may exhibit right-skewness. The log-normal assumption is appropriate here as it allows for the modeling of variables that cannot be negative and where the underlying processes that generate the data are multiplicative rather than additive. Specifically, this assumption accommodates the variability often observed in epidemiological data, which can reflect a combination of different risk factors influencing health outcomes. To generate uncertainty intervals (UIs), we applied bootstrap resampling techniques, which involved drawing 1,000 samples with replacement from the original dataset. For each bootstrap sample, we recalculated the DALY and mortality estimates, thereby generating a distribution of estimates. From this distribution, we computed the 2.5th and 97.5th percentiles to establish 95% uncertainty intervals. This method allows us to capture the variability in our estimates due to sampling uncertainty, which is particularly important when working with complex health data. All statistical analyses were conducted using Python version 3.7.3, with results deemed statistically significant at p < 0.05.
Details of all of the above methods can be found in Supplementary Material 3.
In 2021, the global distribution of type 2 diabetes-related mortality showed significant regional variations (Figure 1A). Countries were classified into five categories based on their mortality rates, ranging from less than 54.09 to as high as 1176.73 deaths per 100,000 people. The lowest mortality rates (<54.09 per 100,000) were found in regions such as East Asia (China, Japan, South Korea), Nordic countries (Sweden, Norway, Finland), and Canada. These areas generally benefit from strong healthcare systems and effective diabetes management programs. Countries with slightly higher, but still relatively low, mortality rates (54.09 to 86.38 per 100,000) included parts of Western Europe (France, Germany), the United States, and several regions in South America (Argentina), Southeast Asia (Malaysia), and Australia. The moderate mortality category (86.38 to 131.52 per 100,000) encompassed regions like Russia, parts of Brazil, and several African countries (Somalia, Kenya, Morocco). Higher mortality rates (131.52 to 255.81 per 100,000) were observed in countries such as Saudi Arabia, Iraq, Pakistan, and Nigeria, while the highest diabetes-related mortality rates (255.81 to 1176.73 per 100,000) were concentrated in South Africa, Egypt, Oman, and Mexico.
Figure 1. Global burden of T2DM in 2021: Distribution of deaths and DALYs. (A) Global distribution of T2DM-related deaths per 100,000 persons. (B) Global distribution of T2DM-related DALYs per 100,000 persons.
Similarly, the distribution of disability-adjusted life years (DALYs) in 2021, which reflects both mortality and morbidity, shows a clear disparity across regions (Figure 1B). Countries with the lowest DALYs (<1510.28 per 100,000) included Sweden, Norway, Finland, Western Europe (France, Germany), Australia, and China, which are better equipped to manage the disease and its complications. Moderately low DALYs (1510.28 to 2283.42 per 100,000) were seen in Canada, Russia, Kazakhstan, and India, while moderate DALYs (2283.42 to 3294.8 per 100,000) were found in countries such as Brazil, Argentina, the United States, Algeria, and Myanmar. The category of moderately high DALYs (3294.8 to 5910.14 per 100,000) included Bolivia, Paraguay, Iran, and Saudi Arabia. The highest DALYs (5910.14 to 22,824.56 per 100,000) were concentrated in South Africa, Mexico, Egypt, and Oman, highlighting regions where diabetes is not only a leading cause of death but also contributes heavily to disability and long-term health complications.
Globally, the burden of DALYs for diabetes mellitus type 2 steadily increased from 1990 to 2021, reflecting broader global health trends. The overall global increase (AAPC 1.6%) demonstrates periods of both acceleration and deceleration, with significant growth observed between 1990-1994 (annual percent change 2.21%) and a slower rise from 2003 to 2012 (annual percent change 0.87%), before picking up again in recent years (annual percent change 1.93% from 2012 to 2018). This general upward trend underscores the expanding global burden of diabetes as a public health issue (Figure 2A).
Figure 2. Trends in T2DM burden from 1990 to 2021 by SDI levels: Age-standardized DALYs. (A) Global trend of age-standardized DALYs per 100,000 persons from 1990 to 2021. (B) Trend of age-standardized DALYs in Low SDI regions from 1990 to 2021. (C) Trend of age-standardized DALYs in Low-middle SDI regions from 1990 to 2021. (D) Trend of age-standardized DALYs in Middle SDI regions from 1990 to 2021. (E) Trend of age-standardized DALYs in High-middle SDI regions from 1990 to 2021. (F) Trend of age-standardized DALYs in High SDI regions from 1990 to 2021.
Across SDI regions, the rise in DALYs is marked by varying intensities. Low and low-middle SDI regions showed the steepest increases. In low SDI regions (AAPC 1.8%), a consistent rise in DALYs occurred, with noticeable accelerations during the 2010-2014 period (annual percent change 2.72%), likely reflecting rapid demographic shifts and increased prevalence of risk factors in these areas (Figure 2B). Low-middle SDI regions, with the highest AAPC of 2.72%, experienced a significant surge in DALYs from 2000 to 2009 (annual percent change 3.35%), followed by a gradual deceleration. These regions face an escalating diabetes burden driven by rising urbanization, changes in lifestyle, and limited healthcare resources (Figure 2C).
Middle SDI regions (AAPC 1.83%) also experienced a notable rise in DALYs, with a strong early growth between 1990 and 1996 (annual percent change 2.74%), followed by more gradual increases in the following decades. This trend reflects the transition of these regions through stages of socioeconomic development, where improvements in healthcare may lag behind rising diabetes risk (Figure 2D).
In contrast, high-middle and high SDI regions displayed more stable trends. High-middle SDI regions showed moderate growth (AAPC 1.35%), with brief accelerations such as between 2012 and 2017 (annual percent change 2.44%), followed by slower growth in the subsequent years (Figure 2E). High SDI regions exhibited the lowest overall AAPC (1.05%) and more variability over time, including a brief period of decline from 2005 to 2011 (annual percent change -1.08%). However, DALYs in these regions rose again significantly from 2014 to 2021 (annual percent change 2.04%) as lifestyle-related factors outweighed earlier preventive measures (Figure 2F). The specific information of AAPC in Supplementary Material 4. The specific information of annual percent change in Supplementary Material 5. A bar chart of the AAPC data is included in Supplementary Material 6.
In 2021, global trends in T2DM showed notable variations in net drift across different SDI levels and between genders. For males worldwide, the net drift in DALYs related to T2DM was 1.74% (95% CI, 1.69 to 1.79), while for females, it was 1.27% (95% CI, 1.18 to 1.37), indicating that the increase in DALYs was slightly higher among males than females. Among the different SDI levels, males in the low-middle SDI regions exhibited the highest annual increase rate at 3.37% (95% CI, 3.24 to 3.50), whereas males in high SDI regions showed a lower trend with an annual increase of 1.04% (95% CI, 1.00 to 1.09). For females, the low-middle SDI regions also displayed the highest growth rate at 2.72% (95% CI, 2.60 to 2.84), while in high SDI regions, the increase was minimal at 0.34% (95% CI, 0.27 to 0.40). Overall, the combined annual increase rate for both sexes in low-middle SDI regions was 3.02% (95% CI, 2.91 to 3.13), while in high SDI regions, it was 0.64% (95% CI, 0.59 to 0.70) (Figure 3A).
Figure 3. Changes in T2DM incidence across age and SDI levels: Net Drift and Age effects. (A) Net drift of annual percentage change in T2DM incidence rates across age groups, shown globally and across different SDI levels for males, females, and both sexes combined. (B) Age effect on T2DM incidence rates per 100,000 persons across different age groups, shown globally and across different SDI levels for males, females, and both sexes combined.
The age effect refers to the impact of aging on the risk of developing or dying from T2DM. As observed (Figure 3B), both the incidence and mortality rates of T2DM generally increase with age across all SDI regions and globally, but there is a decline in the 75-84 age group. This trend is consistent across genders (male, female, and combined). After age 84, both incidence and mortality rates rise sharply, highlighting the physiological vulnerability of the elderly to the onset of diabetes and its complications.
The cohort effect (Figure 4A) captures the impact of birth cohorts on the incidence and mortality rates of T2DM, reflecting how the risk evolves for individuals born within a specific time frame. We observed that individuals born after 1942-1951 generally showed a higher risk of developing and dying from type 2 diabetes compared to older people born before that. This trend is consistent across all SDI regions, with the most pronounced increase observed in Low-middle SDI regions. This cohort effect may be explained by generational shifts in lifestyle, particularly the transition from traditional diets to more processed and calorie-dense foods, decreased physical activity, and greater exposure to risk factors for obesity and diabetes. For those born later, these environmental shifts occur early in life, contributing to increased lifetime exposure to risk factors, and subsequently, a higher lifetime risk of diabetes. For mortality, the cohort effect likely reflects improvements in healthcare and management of diabetes over time, as older cohorts experienced fewer of these advancements during their earlier years. Despite healthcare improvements, the rising incidence of diabetes in younger cohorts poses a significant challenge for public health systems globally.
Figure 4. Birth cohort and time period impacts on T2DM incidence across SDI levels. (A) Cohort effect on T2DM incidence shown as risk ratios across birth cohorts, globally and across different SDI levels for males, females, and both sexes combined. (B) Period effect on T2DM incidence shown as risk ratios across different time periods, globally and across different SDI levels for males, females, and both sexes combined.
The period effect (Figure 4B) reflects the impact of environmental, medical, and social factors on the incidence and mortality rates of T2DM during specific time periods. The trend in risk ratios from 1990 to 2021 shows a significant increase across all SDI regions, particularly in the Low-middle SDI region. Over time, significant advancements in medical technology, diagnostics, and awareness may have influenced these period trends by improving case detection or altering mortality rates. The consistent rise in risk ratios globally and across all SDI levels indicates that the factors driving the diabetes epidemic are not confined to any particular region or group. However, differences in the magnitude of the rise suggest that high SDI regions may have benefited more from advancements in medical care, reflected by a slower increase in mortality compared to low-SDI regions, where healthcare access remains a significant barrier.
The global burden of T2DM from 1990 to 2021 is primarily driven by population growth, which is especially significant in younger populations and regions experiencing rapid demographic expansion. The increasing number of people susceptible to diabetes globally reflects the direct impact of population growth on the prevalence of the disease. Additionally, epidemiological changes, such as shifts in lifestyle, marked by greater urbanization, increasing rates of obesity, and more sedentary behaviors, play a crucial role in fueling the diabetes epidemic worldwide. However, aging contributes less significantly to the global T2DM burden, indicating that while an older population is more prone to diabetes, its impact is relatively smaller at a global scale (Figure 5).
Figure 5. Key drivers of T2DM burden across SDI levels: Aging, population growth, and epidemiological change. The bar chart shows the relative contributions of aging, population growth, and epidemiological change to the overall T2DM burden globally and across different SDI levels.
Projections for T2DM from 1990 to 2044 show a significant rise in both the number of cases and incidence rates among all age groups over 70. After 2020, the number of T2DM cases is expected to increase sharply, with the most noticeable growth in the 70-74 age group. Although smaller, the number of cases in the elderly population (those over 95) is also predicted to rise substantially. This sharp upward trend indicates that population aging will be a key driver of the future T2DM burden. Both men and women are expected to follow similar trends, although women will be slightly more affected in terms of case numbers (Figure 6A). Incidence rates are also expected to increase steadily, with the highest rates projected for the oldest age group (over 95). The continued growth across all age groups suggests that the risk of developing T2DM will increase as the population ages. The projections highlight that healthcare systems must prepare for the significant increase in diabetes cases after 2020, particularly among the elderly (Figure 6B). These findings emphasize the urgent need for public health interventions focused on the aging population. Strategies should prioritize prevention, lifestyle modifications, and improved diabetes management to address the rising burden, especially among older adults, whose cases and incidence rates are predicted to increase substantially in the coming decades.
Figure 6. Future projections of T2DM cases and incidence rates by age group from 1990 to 2044. (A) Projected number of T2DM cases from 1990 to 2044, shown for both sexes combined, males, and females, stratified by age groups (70-74, 75-79, 80-84, 85-89, 90-94, and 95+ years). (B) Projected incidence rates of T2DM per 100,000 persons from 1990 to 2044, shown for both sexes combined, males, and females, stratified by age groups (70-74, 75-79, 80-84, 85-89, 90-94, and 95+ years). Observed (solid lines) and predicted (dashed lines) incidence rates are provided.
The results of this study provide significant insights into the global and regional trends in T2DM from 1990 to 2021, with projections up to 2044. The increasing burden of T2DM, particularly in low and low-middle SDI regions, highlights the role of population growth and epidemiological changes as key drivers of the disease. These findings align with global health trends, where rapid urbanization, changes in lifestyle, and limited access to healthcare exacerbate the prevalence of diabetes in less developed regions (17, 18). These findings underscore the pressing need for targeted public health interventions and highlight disparities in T2DM management across socio-demographic contexts.
The sharp increase in T2DM incidence and mortality in low and low-middle SDI regions is primarily driven by population growth and urbanization. As these regions undergo economic transitions (19), shifting dietary patterns (towards processed and calorie-dense foods) (20, 21), coupled with reduced physical activity (22), are driving rising obesity rates, a critical risk factor for T2DM (23–25). The lack of robust healthcare systems in these regions exacerbates the challenge, as access to early diagnosis, preventive care, and treatment is often limited (8). Consequently, as demonstrated by the results, T2DM incidence is expected to grow disproportionately in these regions, straining healthcare systems. This emphasizes the urgent need for large-scale public health initiatives that focus on early intervention, education, and improving access to healthcare services.
In high and high-middle SDI regions, the increase in T2DM burden is more closely tied to aging populations. The results of this study confirm that aging is a major driver of the disease burden in these regions, as older age groups are at significantly higher risk for developing T2DM and its complications (26, 27). While healthcare systems in high-SDI regions are generally better equipped to manage chronic conditions like diabetes (28), the continuous rise in cases driven by aging populations points to the need for healthcare systems to evolve, placing a greater emphasis on managing chronic diseases in elderly populations (29, 30). Preventive strategies focusing on healthy aging, physical activity, and controlling risk factors such as obesity and hypertension in older adults should be prioritized moving forward.
The age-period-cohort analysis provides further insights into the dynamics of T2DM across different regions. The age effect, which highlights the increased vulnerability of older populations, is consistent across SDI levels but is more pronounced in high-SDI regions where life expectancy is higher. The period effect, reflecting societal changes over time, underscores how advancements in healthcare and increased awareness have altered T2DM trends, particularly in high-SDI regions where improved diabetes management has helped mitigate mortality. However, the cohort effect is concerning, later birth cohorts, especially in low-SDI regions, show a higher lifetime risk of developing T2DM, driven by early exposure to poor lifestyle factors (31, 32). This suggests that without timely interventions targeting younger populations, the T2DM epidemic will continue to worsen.
In high-SDI regions, where aging is the dominant factor, healthcare systems must prioritize managing chronic diseases in older populations. Integrating diabetes management into broader aging and health frameworks could help reduce the long-term T2DM burden. Policymakers and healthcare providers should focus on preventive measures that encourage healthy aging, including interventions aimed at controlling obesity, hypertension, and maintaining physical activity levels in older adults. In low- and middle-SDI regions, where population growth and epidemiological shifts drive the rise of T2DM, multifaceted public health strategies are essential. Key interventions should include promoting healthier diets through public health campaigns that encourage the consumption of whole foods while reducing processed foods high in sugar and unhealthy fats (33). Additionally, increasing physical activity is vital (34, 35); local governments can invest in safe walking and biking paths, while schools implement mandatory physical education and after-school sports programs. Community-based exercise initiatives, like walking clubs, can foster social support. Finally, raising awareness about diabetes risks through comprehensive educational campaigns using various media platforms, along with tailored materials for different literacy levels, can ensure that vital information reaches all populations (36).
While this study utilizes the SDI as an effective tool to categorize regions based on socio-economic factors, it is important to recognize its limitations in capturing the full complexity of socioeconomic diversity within countries. Large, heterogeneous nations like China, India, and United States exhibit significant disparities in healthcare access, public health infrastructure, and economic conditions across different regions (37, 38). These variations can result in notable differences in the incidence and management of T2DM. For instance, rural areas in these countries often face higher diabetes complications and poorer healthcare access compared to urban areas (39–41). As such, while SDI serves as a useful metric for broad analysis, a more granular approach (such as sub-national studies) would provide deeper insights into these within-country disparities and better inform targeted public health interventions.
The projections provided in this study are based on historical data and trends, yet it is crucial to consider the uncertainties inherent in these forecasts. Future advancements in diabetes treatment and management, healthcare reforms, and global public health initiatives may significantly alter the predicted trajectory of T2DM. For example, innovations such as improved pharmacological therapies (42) and novel prevention strategies (43) could potentially reduce the global burden of diabetes. Additionally, large-scale public health initiatives aimed at improving healthcare infrastructure in low- and middle-SDI regions could mitigate the projected rise in diabetes cases (44). Therefore, these forecasts should be interpreted with caution, and policymakers should consider them as a flexible framework rather than a fixed outcome.
In addition to the epidemiological and demographic factors emphasized in this study, other critical contributors to T2DM, such as genetics and environmental exposures, also warrant attention. Genetic predispositions, including insulin resistance and beta-cell dysfunction, can significantly impact an individual’s risk of developing diabetes (45). Moreover, environmental factors, such as exposure to pollutants (46), endocrine-disrupting chemicals (47), and socio-environmental stressors (31), may further exacerbate diabetes risk, particularly in vulnerable populations. Although these aspects were not the primary focus of this analysis, acknowledging their influence is essential for a more comprehensive understanding of global T2DM trends. Future research should explore these factors in greater depth to better elucidate their roles in diabetes prevalence and outcomes.
Overall, the study highlights the importance of early prevention and region-specific strategies to mitigate the future burden of T2DM. By addressing the distinct drivers of T2DM in different SDI contexts, policymakers and public health professionals can develop more effective interventions, helping to curb the rising global diabetes epidemic.
In summary, this study highlights the global and regional trends in T2DM from 1990 to 2021, with projections showing continued case and incidence increases through 2044. Population growth, aging, and epidemiological changes are the primary drivers of this trend, with low- and middle-SDI regions experiencing the most significant increases. While high-SDI regions also face growing burdens, particularly due to aging populations, their more robust healthcare systems provide some mitigation. These findings underscore the urgent need for region-specific interventions targeting lifestyle risk factors and improving healthcare access to curb the rising T2DM burden. Addressing these challenges will be critical to reducing future morbidity and mortality associated with T2DM globally.
The original contributions presented in the study are included in the article/Supplementary Material. Further inquiries can be directed to the corresponding authors.
KH: Conceptualization, Data curation, Formal analysis, Funding acquisition, Software, Supervision, Validation, Visualization, Writing – original draft, Writing – review & editing. HW: Data curation, Validation, Writing – original draft. JX: Supervision, Validation, Writing – original draft. GG: Visualization, Writing – original draft. XL: Data curation, Formal analysis, Writing – review & editing. HG: Formal analysis, Funding acquisition, Writing – review & editing.
The author(s) declare financial support was received for the research, authorship, and/or publication of this article. This project was funded by RCBS20231211090824039/Shenzhen Science and Technology Program, 82304939/National Natural Science Foundation of China, 2022JD223/Bao’an District Science and Technology Innovation Bureau Research Program and 2023K117/Quzhou Municipal Science and Technology Bureau.
The authors declare that the research was conducted in the absence of any commercial or financial relationships that could be construed as a potential conflict of interest.
The authors declare that no Gen AI was used in the creation of this manuscript.
All claims expressed in this article are solely those of the authors and do not necessarily represent those of their affiliated organizations, or those of the publisher, the editors and the reviewers. Any product that may be evaluated in this article, or claim that may be made by its manufacturer, is not guaranteed or endorsed by the publisher.
The Supplementary Material for this article can be found online at: https://www.frontiersin.org/articles/10.3389/fendo.2024.1501690/full#supplementary-material
Supplementary Material 1 | More information on the validity and reliability of the GBD 2021 dataset is provided.
Supplementary Material 2 | The reasons for our choice of age-period-cohort modeling are presented.
Supplementary Material 3 | Details of all of the above methods can be found.
Supplementary Material 4 | The specific information of AAPC.
Supplementary Material 5 | The specific information of annual percent change.
Supplementary Material 6 | A bar chart of the AAPC data is included.
1. Hassan S, Gujral UP, Quarells RC, Rhodes EC, Shah MK, Obi J, et al. Disparities in diabetes prevalence and management by race and ethnicity in the USA: defining a path forward. Lancet Diabetes Endocrinol. (2023) 11:509–24. doi: 10.1016/S2213-8587(23)00129-8
2. Lee SH, Park SY, Choi CS. Insulin resistance: from mechanisms to therapeutic strategies. Diabetes Metab J. (2022) 46:15–37. doi: 10.4093/dmj.2021.0280
3. Wigger L, Barovic M, Brunner AD, Marzetta F, Schöniger E, Mehl F, et al. Multi-omics profiling of living human pancreatic islet donors reveals heterogeneous beta cell trajectories towards type 2 diabetes. Nat Metab. (2021) 3:1017–31. doi: 10.1038/s42255-021-00420-9
4. Einarson TR, Acs A, Ludwig C, Panton UH. Prevalence of cardiovascular disease in type 2 diabetes: a systematic literature review of scientific evidence from across the world in 2007-2017. Cardiovasc Diabetol. (2018) 17:83. doi: 10.1186/s12933-018-0728-6
5. de Boer IH, Khunti K, Sadusky T, Tuttle KR, Neumiller JJ, Rhee CM, et al. Diabetes management in chronic kidney disease: A consensus report by the American diabetes association (ADA) and kidney disease: improving global outcomes (KDIGO). Diabetes Care. (2022) 45:3075–90. doi: 10.2337/dci22-0027
6. Elafros MA, Andersen H, Bennett DL, Savelieff MG, Viswanathan V, Callaghan BC, et al. Towards prevention of diabetic peripheral neuropathy: clinical presentation, pathogenesis, and new treatments. Lancet Neurol. (2022) 21:922–36. doi: 10.1016/S1474-4422(22)00188-0
7. Cioana M, Deng J, Nadarajah A, Hou M, Qiu Y, Chen SSJ, et al. Global prevalence of diabetic retinopathy in pediatric type 2 diabetes: A systematic review and meta-analysis. JAMA Network Open. (2023) 6:e231887. doi: 10.1001/jamanetworkopen.2023.1887
8. Flood D, Seiglie JA, Dunn M, Tschida S, Theilmann M, Marcus ME, et al. The state of diabetes treatment coverage in 55 low-income and middle-income countries: a cross-sectional study of nationally representative, individual-level data in 680 102 adults. Lancet Healthy Longevity. (2021) 2:e340–e51. doi: 10.1016/S2666-7568(21)00089-1
9. Hill-Briggs F, Adler NE, Berkowitz SA, Chin MH, Gary-Webb TL, Navas-Acien A, et al. Social determinants of health and diabetes: A scientific review. Diabetes Care. (2020) 44:258–79. doi: 10.2337/dci20-0053
10. GBD 2021 Diabetes Collaborators. Global, regional, and national burden of diabetes from 1990 to 2021, with projections of prevalence to 2050: a systematic analysis for the Global Burden of Disease Study 2021. Lancet (London England). (2023) 402:203–34. doi: 10.1016/S0140-6736(23)01301-6
11. Murray CJ, Ezzati M, Flaxman AD, Lim S, Lozano R, Michaud C, et al. GBD 2010: design, definitions, and metrics. Lancet (London England). (2012) 380:2063–6. doi: 10.1016/S0140-6736(12)61899-6
12. Chew NWS, Ng CH, Tan DJH, Kong G, Lin C, Chin YH, et al. The global burden of metabolic disease: Data from 2000 to 2019. Cell Metab. (2023) 35:414–28.e3. doi: 10.1016/j.cmet.2023.02.003
13. Foreman KJ, Marquez N, Dolgert A, Fukutaki K, Fullman N, McGaughey M, et al. Forecasting life expectancy, years of life lost, and all-cause and cause-specific mortality for 250 causes of death: reference and alternative scenarios for 2016-40 for 195 countries and territories. Lancet (London England). (2018) 392:2052–90. doi: 10.1016/S0140-6736(18)31694-5
14. Chen X, Giles J, Yao Y, Yip W, Meng Q, Berkman L, et al. The path to healthy ageing in China: a Peking University-Lancet Commission. Lancet (London England). (2022) 400:1967–2006. doi: 10.1016/S0140-6736(22)01546-X
15. Wang H, Song Y, Ma J, Ma S, Shen L, Huang Y, et al. Burden of non-communicable diseases among adolescents and young adults aged 10-24 years in the South-East Asia and Western Pacific regions, 1990-2019: a systematic analysis for the Global Burden of Disease Study 2019. Lancet Child Adolesc Health. (2023) 7:621–35. doi: 10.1016/S2352-4642(23)00148-7
16. Zhang H, Zhou XD, Shapiro MD, Lip GYH, Tilg H, Valenti L, et al. Global burden of metabolic diseases, 1990-2021. Metab Clin Exp. (2024) 160:155999. doi: 10.1016/j.metabol.2024.155999
17. Ye J, Wu Y, Yang S, Zhu D, Chen F, Chen J, et al. The global, regional and national burden of type 2 diabetes mellitus in the past, present and future: a systematic analysis of the Global Burden of Disease Study 2019. Front Endocrinol. (2023) 14:1192629. doi: 10.3389/fendo.2023.1192629
18. Cao Y, Jin C, Zhang J, Sun H, Ma B, Yang X, et al. Diabetes burden, trends, and inequalities in western pacific region, 1990-2019: A population-based study. Diabetes Metab syndrome. (2023) 17:102852. doi: 10.1016/j.dsx.2023.102852
19. Zhang N, Du SM, Ma GS. Current lifestyle factors that increase risk of T2DM in China. Eur J Clin Nutr. (2017) 71:832–8. doi: 10.1038/ejcn.2017.41
20. Colombet Z, Leroy P, Soler LG, Méjean C. Shifts in dietary patterns and risk of type-2 diabetes in a Caribbean adult population: ways to address diabetes burden. Eur J Nutr. (2023) 62:2233–43. doi: 10.1007/s00394-023-03144-6
21. Delpino FM, Figueiredo LM, Bielemann RM, da Silva BGC, Dos Santos FS, Mintem GC, et al. Ultra-processed food and risk of type 2 diabetes: a systematic review and meta-analysis of longitudinal studies. Int J Epidemiol. (2022) 51:1120–41. doi: 10.1093/ije/dyab247
22. Zang J, Ng SW. Age, period and cohort effects on adult physical activity levels from 1991 to 2011 in China. Int J Behav Nutr Phys Activity. (2016) 13:40. doi: 10.1186/s12966-016-0364-z
23. Yan Y, Wu T, Zhang M, Li C, Liu Q, Li F. Prevalence, awareness and control of type 2 diabetes mellitus and risk factors in Chinese elderly population. BMC Public Health. (2022) 22:1382. doi: 10.1186/s12889-022-13759-9
24. Yuan S, Larsson SC. An atlas on risk factors for type 2 diabetes: a wide-angled Mendelian randomisation study. Diabetologia. (2020) 63:2359–71. doi: 10.1007/s00125-020-05253-x
25. Teufel F, Seiglie JA, Geldsetzer P, Theilmann M, Marcus ME, Ebert C, et al. Body-mass index and diabetes risk in 57 low-income and middle-income countries: a cross-sectional study of nationally representative, individual-level data in 685 616 adults. Lancet (London England). (2021) 398:238–48. doi: 10.1016/S0140-6736(21)00844-8
26. Kyrou I, Tsigos C, Mavrogianni C, Cardon G, Van Stappen V, Latomme J, et al. Sociodemographic and lifestyle-related risk factors for identifying vulnerable groups for type 2 diabetes: a narrative review with emphasis on data from Europe. BMC Endocrine Disord. (2020) 20:134. doi: 10.1186/s12902-019-0463-3
27. Li Y, Guo C, Cao Y. Secular incidence trends and effect of population aging on mortality due to type 1 and type 2 diabetes mellitus in China from 1990 to 2019: findings from the Global Burden of Disease Study 2019. BMJ Open Diabetes Res Care. (2021) 9. doi: 10.1136/bmjdrc-2021-002529
28. Silva-Tinoco R, Cuatecontzi-Xochitiotzi T, de la Torre-Saldaña V, León-García E, Serna-Alvarado J, Guzmán-Olvera E, et al. Role of social and other determinants of health in the effect of a multicomponent integrated care strategy on type 2 diabetes mellitus. Int J Equity Health. (2020) 19:75. doi: 10.1186/s12939-020-01188-2
29. Wu N, Xie X, Cai M, Han Y, Wu S. Trends in health service needs, utilization, and non-communicable chronic diseases burden of older adults in China: evidence from the 1993 to 2018 National Health Service Survey. Int J Equity Health. (2023) 22:169. doi: 10.1186/s12939-023-01983-7
30. Xu Y, Koh XH, Chua YTS, Tan CGI, Aloweni FAB, Yap BEJ, et al. The impact of community nursing program on healthcare utilization: A program evaluation. Geriatric Nurs (New York NY). (2022) 46:69–79. doi: 10.1016/j.gerinurse.2022.04.024
31. Bjornstad P, Chao LC, Cree-Green M, Dart AB, King M, Looker HC, et al. Youth-onset type 2 diabetes mellitus: an urgent challenge. Nat Rev Nephrol. (2023) 19:168–84. doi: 10.1038/s41581-022-00645-1
32. Li C, Lumey LH. Early-life exposure to the Chinese famine of 1959-1961 and type 2 diabetes in adulthood: A systematic review and meta-analysis. Nutrients. (2022) 14. doi: 10.3390/nu14142855
33. Dening J, Mohebbi M, Abbott G, George ES, Ball K, Islam SMS. A web-based low carbohydrate diet intervention significantly improves glycaemic control in adults with type 2 diabetes: results of the T2Diet Study randomised controlled trial. Nutr Diabetes. (2023) 13:12. doi: 10.1038/s41387-023-00240-8
34. Carbone S, Del Buono MG, Ozemek C, Lavie CJ. Obesity, risk of diabetes and role of physical activity, exercise training and cardiorespiratory fitness. Prog Cardiovasc Dis. (2019) 62:327–33. doi: 10.1016/j.pcad.2019.08.004
35. Hemmingsen B, Gimenez-Perez G, Mauricio D, Roqué IFM, Metzendorf MI, Richter B. Diet, physical activity or both for prevention or delay of type 2 diabetes mellitus and its associated complications in people at increased risk of developing type 2 diabetes mellitus. Cochrane Database Systematic Rev. (2017) 12:Cd003054. doi: 10.1002/14651858.CD003054.pub4
36. Shirmohammadi N, Soltanian AR, Borzouei S. Public awareness of early and late complications of type 2 diabetes - application of latent profile analysis in determining questionnaire cut-off points. Osong Public Health Res perspectives. (2018) 9:261–8. doi: 10.24171/j.phrp.2018.9.5.08
37. Atre S, Deshmukh S, Kulkarni M. Prevalence of type 2 diabetes mellitus (T2DM) in India: A systematic review (1994-2018). Diabetes Metab Syndrome. (2020) 14:897–906. doi: 10.1016/j.dsx.2020.05.040
38. Luo H, Basu R, Bell RA, Rafferty AP, Zeng X, Qu H, et al. Rural-urban differences in uptake of diabetes self-management education among Medicare beneficiaries: Results from the 2016 to 2018 Medicare Current Beneficiary Survey. J Rural Health. (2022) 38:986–93. doi: 10.1111/jrh.12588
39. Kang N, Liu X, Liao W, Tu R, Sang S, Zhai Z, et al. Health-related quality of life among rural adults with type 2 diabetes mellitus: a cross-sectional study. Eur J Public Health. (2021) 31:547–53. doi: 10.1093/eurpub/ckaa247
40. Lin K, Yao M, Andrew L, Li R, Chen Y, Oosthuizen J, et al. Exploring treatment burden in people with type 2 diabetes mellitus: a thematic analysis in China's primary care settings. BMC Primary Care. (2024) 25:88. doi: 10.1186/s12875-024-02301-y
41. Dugani SB, Mielke MM, Vella A. Burden and management of type 2 diabetes in rural United States. Diabetes/metabolism Res Rev. (2021) 37:e3410. doi: 10.1002/dmrr.v37.5
42. Choi Y, Kwon HK, Park S. Polygenic variants linked to oxidative stress and the antioxidant system are associated with type 2 diabetes risk and interact with lifestyle factors. Antioxidants (Basel Switzerland). (2023) 12. doi: 10.3390/antiox12061280
43. Liu L, Zhang J, Cheng Y, Zhu M, Xiao Z, Ruan G, et al. Gut microbiota: A new target for T2DM prevention and treatment. Front Endocrinol. (2022) 13:958218. doi: 10.3389/fendo.2022.958218
44. Alareeki A, Awad SF, Critchley JA, El-Nahas KG, Al-Hamaq AO, Alyafei SA, et al. Epidemiological impact of public health interventions against diabetes in Qatar: mathematical modeling analyses. Front Public Health. (2023) 11:1167807. doi: 10.3389/fpubh.2023.1167807
45. Hivert MF, Vassy JL, Meigs JB. Susceptibility to type 2 diabetes mellitus–from genes to prevention. Nat Rev Endocrinol. (2014) 10:198–205. doi: 10.1038/nrendo.2014.11
46. Misra BB, Misra A. The chemical exposome of type 2 diabetes mellitus: Opportunities and challenges in the omics era. Diabetes Metab Syndrome. (2020) 14:23–38. doi: 10.1016/j.dsx.2019.12.001
Keywords: type 2 diabetes mellitus, disability-adjusted life years, mortality, age-period-cohort analysis, epidemiological transition, socio-demographic index
Citation: He K-J, Wang H, Xu J, Gong G, Liu X and Guan H (2024) Global burden of type 2 diabetes mellitus from 1990 to 2021, with projections of prevalence to 2044: a systematic analysis across SDI levels for the global burden of disease study 2021. Front. Endocrinol. 15:1501690. doi: 10.3389/fendo.2024.1501690
Received: 25 September 2024; Accepted: 24 October 2024;
Published: 08 November 2024.
Edited by:
Yun Shen, Pennington Biomedical Research Center, United StatesReviewed by:
Jiahe Li, University of Pittsburgh, United StatesCopyright © 2024 He, Wang, Xu, Gong, Liu and Guan. This is an open-access article distributed under the terms of the Creative Commons Attribution License (CC BY). The use, distribution or reproduction in other forums is permitted, provided the original author(s) and the copyright owner(s) are credited and that the original publication in this journal is cited, in accordance with accepted academic practice. No use, distribution or reproduction is permitted which does not comply with these terms.
*Correspondence: Ke-Jie He, aGVrZWppZUBzdHUueG11LmVkdS5jbg==; Huiting Guan, aHNzZ3k4NzVAb3V0bG9vay5jb20=; Xu Liu, eHVfbGl1MjNAMTYzLmNvbQ==
†These authors have contributed equally to this work and share first authorship
Disclaimer: All claims expressed in this article are solely those of the authors and do not necessarily represent those of their affiliated organizations, or those of the publisher, the editors and the reviewers. Any product that may be evaluated in this article or claim that may be made by its manufacturer is not guaranteed or endorsed by the publisher.
Research integrity at Frontiers
Learn more about the work of our research integrity team to safeguard the quality of each article we publish.