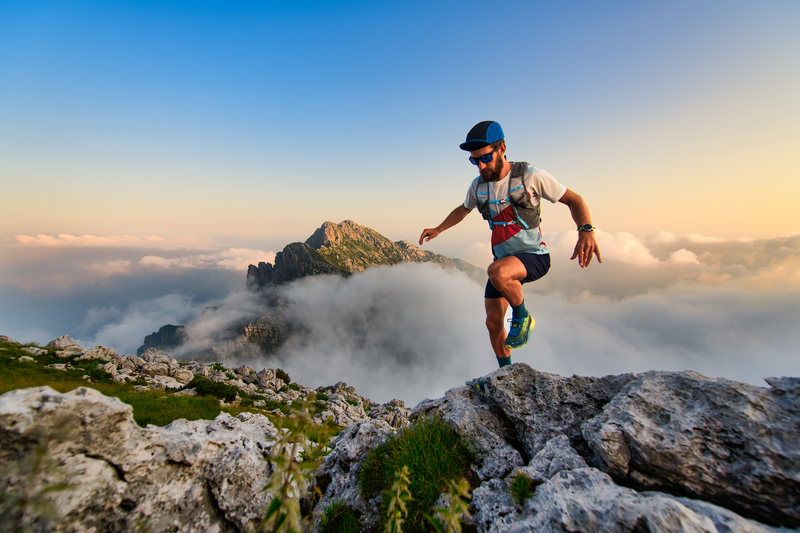
94% of researchers rate our articles as excellent or good
Learn more about the work of our research integrity team to safeguard the quality of each article we publish.
Find out more
ORIGINAL RESEARCH article
Front. Endocrinol. , 06 August 2024
Sec. Obesity
Volume 15 - 2024 | https://doi.org/10.3389/fendo.2024.1460652
This article is part of the Research Topic Advances in the Research of Diabetic Nephropathy Volume III View all 4 articles
A retraction of this article was approved in:
Retraction: Mendelian randomization based on immune cells in diabetic nephropathy
Background: DKD, a leading cause of chronic kidney and end-stage renal disease, lacks robust immunological research. Recent GWAS utilizing SNPs and CNVs has shed light on immune mechanisms of kidney diseases. However, DKD’s immunological basis remains elusive. Our goal is to unravel cause-effect relationships between immune cells and DKD using Mendelian randomization.
Methodology: We analyzed FinnGen data (1032 DKD cases, 451,248 controls) with 731 immunocyte GWAS summaries (MP=32, MFI=389, AC=118, RC=192). We employed forward and reverse Mendelian randomization to explore causal links between immune cell traits and DKD. Sensitivity analysis ensured robustness, heterogeneity checks, and FDR correction minimized false positives.
Results: Our study explored the causal link between diabetic nephropathy (DKD) and immunophenotypes using two-sample Mendelian Randomization (MR) with IVW. Nine immunophenotypes were significantly associated with DKD at p<0.05 after FDR correction. Elevated CD24, CD3 in Treg subsets, CD39+ CD4+, and CD33− HLA DR− AC correlated positively with DKD risk, while CD27 in B cells and SSC−A in CD4+ inversely correlated. Notably, while none showed significant protection, further research on immune cells’ role in DKD may provide valuable insights.
Conclusion: The results of this study show that the immune cells are closely related to DKD, which may be helpful in the future clinical study.
Diabetes is a major public health problem. Diabetes Renal Disease (DKD), which is a more general diagnosis than DN, is the leading cause of CKD and end stage renal disease in the United States (1). About 40% of people with diabetes develop kidney disease (DKD). DKD is characterized by hyperfiltration of glomerular, progressive albuminuria, reduced glomerular filtration rate (GFR), and eventually end-stage renal disease (ESRD). Diabetes-Related metabolic changes result in glomerulonephritis, glomerulonephritis, tubulointerstitial inflammation and fibrosis (2). Prevention and treatment of chronic renal disease (CKD) in patients with diabetes is now the main goal of their overall treatment. Intensified treatment in patients with diabetes involves controlling blood glucose and blood pressure and blocking the renin-angiotensin-aldosterone system; this will reduce the occurrence and delay the development of renal disease (DKD).
In fact, the significant decline in the incidence of DKD and improvement in patient outcomes over the past 30 years are mainly attributed to improvements in diabetes care. However, the need for innovative treatment strategies to prevent, halt, treat, and reverse DKD remains unmet (3). It significantly impacts an individual’s overall health and daily functioning, which is also the focus of our research.
Recent studies have uncovered a complicated link between the immune system and renal disease in diabetes. Evidence from both clinical and experimental studies suggests that several innate immune pathways may be involved in the development and progression of DM (DKD). Toll-like receptors detect endogenous danger-associated molecular patterns that are produced in diabetic patients and induce sterile tubulointerstitial inflammation via NF-κB signaling (4). The NLRP3 inflammasome is associated with the induction of IL 1β and IL-18 in diabetic renal metabolism and the activation of proinflammatory cascades (5). In DKD, the kallikrein-kinin system promotes inflammation by producing bradykinin and activating bradykinin receptors, whereas thrombin, which activates protease-activated receptors on kidney cells, is involved in inflammation and fibrosis in the kidney (6). However, to date, only a few risk loci for diabetic kidney disease have been identified. In the past decade, genome-wide association studies (GWAS) have emerged as a powerful tool for identifying genetic risk factors for diabetic kidney disease (DKD). In recent years, GWAS have gained access to larger numbers of participants, thus enhancing the statistical power to detect more genetic risk factors (7). The objective of the research is also to find safer and more effective methods by means of Pharmacogenetics (PGP) to deal with the high non-response or partial response to existing medicines in patients with DM (8). Genome-Wide Association Studies (GWAS) are essential for the analysis of genetic variations across the whole genome in large groups, to identify promising genetic sites and pathways, and to improve our understanding of the complex genetic factors that underlie possible diseases (9). This will also help us to explore the relationship between susceptibility, inflammation, and genetics in patients with diabetes mellitus. We have conducted profound discussions on the relationship between immunity and diabetic kidney disease (DKD), and found that studies have revealed the critical role of Th17/Treg cell imbalance in DKD. Dapagliflozin (Dap) reverses this imbalance by inhibiting SGK1, leading to a decrease in Th17 cells and an increase in Treg cells. Consequently, it improves DKD symptoms such as proteinuria and fibrosis independently of glycemic control, indicating the potential of Dap in the prevention of DKD (10). Additional research has comprehensively analyzed the macrophage transcriptomic profile in the early stages of DKD, revealing an increase in renal resident and infiltrating macrophage subsets, as well as a subgroup-specific enhancement in the expression of proinflammatory and anti-inflammatory genes. The changes in macrophage polarization status are consistent with the continuity of activation and differentiation states, tending towards an undifferentiated phenotype but with an increase in the M1-like inflammatory phenotype over time. Validation of the mouse studies through RNAseq and immunostaining further elucidates the dynamic changes in macrophage phenotypes in DKD, emphasizing their crucial role in disease progression (11). Additional research has comprehensively analyzed the macrophage transcriptomic profile in the early stages of DKD, revealing an increase in renal resident and infiltrating macrophage subsets, as well as a subgroup-specific enhancement in the expression of proinflammatory and anti-inflammatory genes. The changes in macrophage polarization status are consistent with the continuity of activation and differentiation states, tending towards an undifferentiated phenotype but with an increase in the M1-like inflammatory phenotype over time. Validation of the mouse studies through RNAseq and immunostaining further elucidates the dynamic changes in macrophage phenotypes in DKD, emphasizing their crucial role in disease progression (12).
Mendelian randomization (MR) is a statistical approach primarily used to infer epidemiological causality based on Mendelian genetic principles (13). In the MR method, ensuring the logical order of causality is crucial. Previous observational studies have shown some association between immune cell properties and DM, which supports the hypothesis that they are related (14). DKD and immune system interactions are multifaceted, with potential reciprocal influences. For instance, DKD can induce immune responses that further exacerbate kidney damage, while immune dysregulation can drive the initiation and progression of DKD. To address these complexities between them, we selected bidirectional Mendelian Randomization tests rather than conventional or reverse MR approaches to investigate the cause and effect of immune cell characteristics and renal disease in DM (15). Conventional MR focuses solely on the causal effect of an exposure on an outcome, while reverse MR examines the effect of the outcome on the exposure. Bidirectional MR, on the other hand, allows us to simultaneously explore both directions, providing a clearer picture of the causal pathways involved. This is particularly important for conditions like DKD, where causality can flow in both directions. Furthermore, bidirectional MR ensures that we are not overlooking important causal effects in either direction, which could lead to incomplete or misleading conclusions.
In this paper, we analyzed the cause and effect of 731 immunocytes and diabetic nephropathy by means of Mendelian randomization (MR). MR utilizes genetic variations as proxies for risk and requires validating instrumental variables (IVs) that satisfy three key assumptions for causal inference: (1) exposure has a direct relationship with genetic variation; (2) there is no genetic association between exposure and outcome, which is a potential confounder; (3) no genetic effect on the outcome is produced by non-exposure pathways (show in Figure 1).
All immunological characteristics in the GWAS catalogue, ranging from GCST0001391 to GCST0002121, are easily available (16). This GWAS included 3 757 European persons who did not overlap. About 22 million single nucleotide polymorphisms (SNPs) were estimated and statistically analyzed by means of a high density array based on a Sardinian sequence reference panel, taking into account the covariates of age, age, and gender. A total of 731 immunological phenotypes were examined, including 192 subtypes of RC, 32 subtypes of morphology (MP), 118 subtypes of absolute cell count (AC), and median fluorescence intensity (MFI) representing 389 surface antigen levels. In particular, the MP characteristics included CDC and TBNK panels, whereas the characteristics of MFI, RC and AC included B-cells, CDC, T-cell maturation, myeloid cells, monocyte, and TBNK (T-cells, B-cells, and naturally killer proteins).
Aggregated Diabetic Kidney Disease GWAS (DKD) statistics are available from FinnGen (https://www.finngen.fi/en). A total of 452280 samples were included in this study (Ncase = 1032, Ncontrol = 451,248), and more than 500,000 DKD phenotypes were included in the GWAS analysis, which identified over 20 million single nucleotide polymorphisms (SNPs).
We employed a selectively chosen tool variable (IVs), with a linkage disequilibrium (LD) r2 threshold of less than 0.1 and a distance of 500 kb, to refine these SNPs, aiming to reduce collinearity and confounding bias, and thereby improve the accuracy and reliability of causal inference analysis. The 1000 Genomes Project served as the reference panel for calculating LD r2. A significance threshold of 5×10-8 was adopted for the new DKD analysis, which conformed to the accepted standards in genetic research. The intravenous injection intensity was evaluated to mitigate potential weak instrument bias. The F-statistic was calculated to assess the strength of the instrumental variables. The length of the instrument variables (IVs) for the immune phenotypes ranged from 3 to 1643, with an average explanatory power of 0.137% (ranging from 0.009% to 0.995%) for the differences in relevant immune characteristics.
We used R Version 4.4 (http://www.Rproject.org) in all of our studies. In order to specifically assess the causal association of 731 immunophenotypes with Diabetic Kidney Disease (DKD), we used the clumping procedure in PLINK package (version v1.90) to prune these SNPs and screening instrumental variables (17). Mendelian Randomization package (version 0.4.3) was used to perform median weighted analysis (18), pattern-weighted analysis (19) and Inverse Variance Weighted Analysis (IVW) (20). The Cochran Q statistic and its p-value (IV) were used for the assessment of the instrument heterogeneity among the variables, and the MR-Egger method was incorporated for the detection of horizontal pleiotropy, represented by a significant intercept term (21). In addition, using the MR-PRESSO package (22), we used MR-PRESSO, a robust MR Pleiotropy Residual Sum and Outlier (MR-PRESSO) technique to detect and remove horizontal pleiotropy outliers that may significantly affect the estimation results. After these SNPs were deleted (19), the IVW analysis was re-performed. In addition, we searched the Phenoscanner V2 web site for SNPs showing suggestive associations (P < 10-5) (http://www.phenoscanner.medschl.cam.ac.uk/) (23). Finally, funnel plots were used to test the correlation and heterogeneity, scatter plots were used to detect the outliers.
In exploring the causal effects of diabetic nephropathy on immunophenotypes, we employed the IVW (inverse variance weighted) method as the primary approach in a two-sample Mendelian Randomization (MR) analysis. Despite applying multiple testing corrections via the False Discovery Rate (FDR) method, we detected nine suggestive immunophenotypes at a significance level of 0.05: four in the CD3 on CM CD4+ panel, three in the CD27 on memory B cell panel, three in the CD3 on secreting Treg panel, three in the SSC−A on CD4+ panel, two in the CD3 on CD39+ CD4+ panel, one in the CD28+ CD45RA+ CD8br %T cell panel, one in the CD33− HLA DR− AC panel, one in the CD3 on activated & secreting Treg panel, and one in the CD24 on switched memory panel.
Our findings suggest that the pathogenesis of diabetic kidney disease (DKD) is associated with increased levels of CD24 (OR=1.09, 95% CI=1.03-1.16, p=0.005), CD3 on activated & secreting Treg (OR=1.12, 95% CI=1.03-1.22, p=0.010), CD3 on CD39+ CD4+ (OR=1.11, 95% CI=1.03-1.19, p=0.006), CD3 on CM CD4+ (OR=1.11, 95% CI=1.03-1.20, p=0.007), CD3 on secreting Treg (OR=1.14, 95% CI=1.06-1.23, p<0.001), and CD33− HLA DR− AC (OR=1.16, 95% CI=1.06-1.28, p=0.002). Our study also revealed that diabetic kidney disease (DKD) is associated with decreased levels of CD27 on memory B cells (OR=0.91, 95% CI=0.86-0.97, p=0.006) and SSC−A on CD4+ cells (OR=0.81, 95% CI=0.73-0.91, p<0.001) (Figure 2). Finally, The scatter plots showed minimal influence of outliers on the data, but the funnel plots showed high correlation and no heterogeneity.
After FDR correction (FDR<0.05), we found that nine immunophenotypes did not exhibit significant protective effects against diabetic kidney disease (DKD). Among them CD24 (OR=1.02, 95% CI=0.96-1.09, p=0.446), CD3 on activated & secreting Treg (OR=0.97, 95% CI=0.91-1.02, p=0.231), CD3 on CD39+ CD4+ (OR=0.98, 95% CI=0.92-1.04, p=0.446), CD3 on CM CD4+ (OR=1.00, 95% CI=0.94-1.06, p=0.961), CD3 on secreting Treg (OR=0.97, 95% CI=0.92-1.03, p=0.332), CD33− HLA DR− AC (OR=1.03 95% CI=0.95-1.12, p=0.482), CD27 on memory B cells (OR=1.03, 95% CI=0.97-1.09, p=0.317) and SSC−A on CD4+ cells (OR=1.01, 95% CI=0.95-1.07, p=0.726). In addition, the MR-Egger intercept and the overall MR-PRESSO test ruled out the possibility of horizontal pleiotropic effects. The sensitivity analysis provided complete data confirming the strength of the established causality (Figure 3).Finally, The scatter plots showed minimal influence of outliers on the data, but the funnel plots showed high correlation and no heterogeneity.
Figure 3 Forest plots showed the causal associations between immune cell traits and DKD by using different methods.
Based on a large number of publicly available genetic data, we examined the cause and effect of the disease in 731 immune cells. This is, so far, the only Mendelian randomization study that has investigated a causal relationship between multiple immune phenotypes and DM. Four classes of immunity (MFI, RC, AC and MP) were included in the study. Among them, 9 of the immunological phenotypes demonstrated a causal effect by Mendelian randomization, and one of them showed a strong causal relationship with DM.
Our research indicates a correlation between an increased percentage of CD24 cells and a heightened risk of diabetic kidney disease (DKD). CD24, a small glycosylphosphatidylinositol (GPI)-anchored glycoprotein, is broadly expressed in various cell types. Because of the differences in glycosylation, CD24 on the cell surface has been shown to interact with a variety of receptors to mediate multiple physiological functions (24). In particular, the inactivation of CD24-Siglec-E pathway may worsen the condition, whereas CD24Fc therapy may alleviate metabolic disturbances caused by diet, including obesity, dyslipidemia, insulin resistance, and nonalcoholic steatohepatitis (NASH).Mechanistically, Siglec-E’s sialic acid-dependent recognition of CD24 induces the recruitment of SHP-1, which in turn suppresses metabolic inflammation and prevents metabolic syndrome (25). While the use of CD24 as a target of immune checkpoint for cancer immunotherapy is still in its infancy, clinical trials have shown promising results. Monoclonal antibodies targeting CD24 have been found to possess excellent tolerability and safety profiles. In addition, preclinical research is exploring the use of CAR T cells, antibody drug conjugates, and gene therapy to target CD24 and strengthen the immune response to cancer (26).
Immunological memory can protect the human body from reinfection with previously recognized pathogens. This memory includes the maintenance of durable serum antibody titers provided by long-lived plasma cells, as well as memory T and B cells, which are supported by other cells. Memory B cells are the primary precursor cells for new plasma cells during secondary infections. CD27 is one of the most commonly used markers to define human memory B cells (27). Costimulation of CD8 T cells by CD27 in mice may promote immune activation and enhance primary, secondary, memory, and recall responses to viral infection (28). These research findings have provided new insights for our study on the association between diabetic kidney disease and CD27 (29).
Previous research has shown that there is a significant causal relationship between several immunological mediators and GDM (GDM). After FDR detection, CD127 on CD28 + CD45RA + CD8br and CD19 on PB/PC have been demonstrated to reduce the effects of GDM (30). However, the role of CD28+ CD45RA+ CD8br %T cells in diabetic kidney disease (DKD) has not yet been reported in research. Previous studies have found that CD3 is involved in the pathogenesis of diabetic chronic kidney disease (CKD) (31). However, research on the specific mechanism between diabetic nephropathy and CD3 has yet to be found.
The association between HLA-DR (human leukocyte antigen-DR) and diabetic nephropathy has attracted considerable attention. However, according to an early study, there is no direct correlation between HLA-DR and diabetic nephropathy (32). However, there are also studies indicating that diabetic end-stage renal disease (DESRD) in young AB subjects with type 2 diabetes mellitus (T2DM) has a genetic basis related to HLA, which aligns with our research findings (33). The identification of specific cell subsets is particularly crucial for immune profiling analysis, as abnormal DNA methylation in peripheral immune cells contributes to the progression of diabetic kidney disease (DKD) (34).
In this study, we used a two-sample Mendelian randomization analysis, using data from a large population of about 452280 individuals, ensuring significant statistical power. The results of the study were based on the genetic instrumental variables, and a variety of robust Mendelian randomization techniques were used to analyze the causal relationship between the two groups. In addition, to control for false positive results in multi-hypothesis testing, we used the FDR (False Discovery Rate) to control the statistical bias caused by multiple comparisons.
However, this study indeed has some shortcomings. Firstly, even after several sensitivity studies, a comprehensive assessment of horizontal pleiotropy remains difficult to achieve. Second, the lack of data at the individual level made it impossible to conduct a stratified population analysis. Third, reliance on European databases limits the generalization of the results to other nationalities. Finally, the flexible criteria used in this study might have resulted in a higher rate of false positives, but they also allowed for a more comprehensive assessment of the strong link between immunity and DM. In general, the next step of the study will be to conduct randomized controlled trials in DM to minimize the potential effect of confounding factors and achieve a higher degree of causality.
In summary, our comprehensive bidirectional MR analysis has revealed the causal links between various immunophenotypes and diabetic kidney disease (DKD), shedding light on the intricate web of relationships between DKD and the immune system. Furthermore, our study has successfully mitigated the impact of reverse causality, other variables, and other inevitable confounding factors, offering researchers a fresh perspective to delve into the biological underpinnings of DKD and potentially paving the way for early intervention and treatment strategies.
The original contributions presented in the study are included in the article/supplementary material. Further inquiries can be directed to the corresponding author/s.
YZ: Writing – original draft. HZ: Data curation, Writing – review & editing. HY: Conceptualization, Writing – review & editing. CH: Methodology, Resources, Software, Writing – original draft. YL: Software, Writing – original draft.
The author(s) declare that no financial support was received for the research, authorship, and/or publication of this article.
The authors declare that the research was conducted in the absence of any commercial or financial relationships that could be construed as a potential conflict of interest.
All claims expressed in this article are solely those of the authors and do not necessarily represent those of their affiliated organizations, or those of the publisher, the editors and the reviewers. Any product that may be evaluated in this article, or claim that may be made by its manufacturer, is not guaranteed or endorsed by the publisher.
1. Gupta S, Dominguez M, Golestaneh L. Diabetic kidney disease: An Update. Med Clin North Am. (2023) 107:689–705. doi: 10.1016/j.mcna.2023.03.004
2. Alicic RZ, Rooney MT, Tuttle KR. Diabetic kidney disease: Challenges, Progress, and possibilities. Clin J Am Soc Nephrol. (2017) 12:2032–45. doi: 10.2215/CJN.11491116
3. Thomas MC, Brownlee M, Susztak K, Sharma K, Jandeleit-Dahm KA, Zoungas S, et al. Diabetic kidney disease. Nat Rev Dis Primers. (2015) 1:15018. doi: 10.1038/nrdp.2015.18
4. Panchapakesan U, Pollock C. The role of toll-like receptors in diabetic kidney disease. Curr Opin Nephrol Hypertens. (2018) 27:30–4. doi: 10.1097/MNH.0000000000000377
5. Shahzad K, Fatima S, Khawaja H, Elwakiel A, Gadi I, Ambreen S, et al. Podocyte-specific Nlrp3 inflammasome activation promotes diabetic kidney disease. Kidney Int. (2022) 102:766–79. doi: 10.1016/j.kint.2022.06.010
6. Liu W, Stanton RC, Zhang Z. The kallikrein-kinin system in diabetic kidney disease. Curr Opin Nephrol Hypertens. (2017) 26:351–7. doi: 10.1097/MNH.0000000000000344
7. Sandholm N, Dahlström EH, Groop PH. Genetic and epigenetic background of diabetic kidney disease. Front Endocrinol (Lausanne). (2023) 14:1163001. doi: 10.3389/fendo.2023.1163001
8. Salem RM, Todd JN, Sandholm N, Cole JB, Chen WM, Andrews D, et al. Genome-wide association study of diabetic kidney disease highlights biology involved in glomerular basement membrane collagen. J Am Soc Nephrol. (2019) 30:2000–16. doi: 10.1681/ASN.2019030218
9. Hayes B. Overview of statistical methods for genome-wide association studies (GWAS). Methods Mol Biol. (2013) 1019:149–69. doi: 10.1007/978-1-62703-447-0_6
10. Wang D, Zhang Z, Si Z, Yang Y, Li S, Xue Y. Dapagliflozin reverses the imbalance of T helper 17 and T regulatory cells by inhibiting SGK1 in a mouse model of diabetic kidney disease. FEBS Open Bio. (2021) 11:1395–405. doi: 10.1002/2211-5463.13147
11. Fu J, Sun Z, Wang X, Zhang T, Yuan W, Salem F, et al. The single-cell landscape of kidney immune cells reveals transcriptional heterogeneity in early diabetic kidney disease. Kidney Int. (2022) 102:1291–304. doi: 10.1016/j.kint.2022.08.026
12. Tanase DM, Gosav EM, Anton MI, Floria M, Seritean Isac PN, Hurjui LL, et al. Oxidative stress and NRF2/KEAP1/ARE pathway in diabetic kidney disease (DKD): New perspectives. Biomolecules. (2022) 12:1227. doi: 10.3390/biom12091227
13. Bowden J, Holmes MV. Meta-analysis and Mendelian randomization: A review. Res Synth Methods. (2019) 10:486–96. doi: 10.1002/jrsm.1346
14. Ference BA, Holmes MV, Smith GD. Using mendelian randomization to improve the design of randomized trials. Cold Spring Harb Perspect Med. (2021) 11:a040980. doi: 10.1101/cshperspect.a040980
15. Bonacina F, Baragetti A, Catapano AL, Norata GD. The interconnection between immuno-metabolism, diabetes, and CKD. Curr Diabetes Rep. (2019) 19:21. doi: 10.1007/s11892-019-1143-4
16. Visscher PM, Brown MA, McCarthy MI, Yang J. Five years of GWAS discovery. Am J Hum Genet. (2012) 90:7–24. doi: 10.1016/j.ajhg.2011.11.029
17. 1000 Genomes Project Consortium, Auton A, Brooks LD, Durbin RM, Garrison EP, Kang HM, et al. A global reference for human genetic variation. Nature. (2015) 526:68–74. doi: 10.1038/nature15393
18. Ren F, Jin Q, Liu T, Ren X, Zhan Y. Causal effects between gut microbiota and IgA nephropathy: a bidirectional Mendelian randomization study. Front Cell Infect Microbiol. (2023) 13:1171517. doi: 10.3389/fcimb.2023.1171517
19. Bowden J, Davey Smith G, Haycock PC, Burgess S. Consistent estimation in mendelian randomization with some invalid instruments using a weighted median estimator. Genet Epidemiol. (2016) 40:304–14. doi: 10.1002/gepi.21965
20. Hartwig FP, Davey Smith G, Bowden J. Robust inference in summary data Mendelian randomization via the zero modal pleiotropy assumption. Int J Epidemiol. (2017) 46:1985–98. doi: 10.1093/ije/dyx102
21. Patel A, Ye T, Xue H, Lin Z, Xu S, Woolf B, et al. MendelianRandomization v0.9.0: updates to an R package for performing Mendelian randomization analyses using summarized data. Wellcome Open Res. (2023) 8:449. doi: 10.12688/wellcomeopenres.19995.1
22. Cho Y, Haycock PC, Sanderson E, Gaunt TR, Zheng J, Morris AP, et al. Exploiting horizontal pleiotropy to search for causal pathways within a Mendelian randomization framework. Nat Commun. (2020) 11:1010. doi: 10.1038/s41467-020-14452-4
23. Verbanck M, Chen CY, Neale B, Do R. Publisher Correction: Detection of widespread horizontal pleiotropy in causal relationships inferred from Mendelian randomization between complex traits and diseases. Nat Genet. (2018) 50:1196. doi: 10.1038/s41588-018-0164-2
24. Liu Y, Zheng P. CD24-Siglec interactions in inflammatory diseases. Front Immunol. (2023) 14:1174789. doi: 10.3389/fimmu.2023.1174789
25. Wang X, Liu M, Zhang J, Brown NK, Zhang P, Zhang Y, et al. CD24-Siglec axis is an innate immune checkpoint against metaflammation and metabolic disorder. Cell Metab. (2022) 34:1088–1103.e6. doi: 10.1016/j.cmet.2022.07.005
26. Huang S, Zhang X, Wei Y, Xiao Y. Checkpoint CD24 function on tumor and immunotherapy. Front Immunol. (2024) 15:1367959. doi: 10.3389/fimmu.2024.1367959
27. Grimsholm O. CD27 on human memory B cells-more than just a surface marker. Clin Exp Immunol. (2023) 213:164–72. doi: 10.1093/cei/uxac114
28. Zuo MH, Tang J, Xiang MM, Long Q, Dai JP, Yu GD, et al. Clinical observation of the reduced glutathione in the treatment of diabetic chronic kidney disease. J Cell Biochem. (2019) 120:8483–91. doi: 10.1002/jcb.28135
29. Grant EJ, Nüssing S, Sant S, Clemens EB, Kedzierska K. The role of CD27 in anti-viral T-cell immunity. Curr Opin Virol. (2017) 22:77–88. doi: 10.1016/j.coviro.2016.12.001
30. Pichler R, Afkarian M, Dieter BP, Tuttle KR. Immunity and inflammation in diabetic kidney disease: translating mechanisms to biomarkers and treatment targets. Am J Physiol Renal Physiol. (2017) 312:F716–31. doi: 10.1152/ajprenal.00314.2016
31. Ji Z, Zhang C, Yuan J, He Q, Zhang X, Yang D, et al. Is there a causal association between gestational diabetes mellitus and immune mediators? A bidirectional Mendelian randomization analysis. Front Endocrinol (Lausanne). (2024) 15:1358144. doi: 10.3389/fendo.2024.1358144
32. Saner B, Bonney E, Barbosa J. HLA-DR antigens and diabetic nephropathy. Diabetologia. (1983) 25:452. doi: 10.1007/BF00282529
33. Dyck R, Bohm C, Klomp H. Increased frequency of HLA A2/DR4 and A2/DR8 haplotypes in young saskatchewan aboriginal people with diabetic end-stage renal disease. Am J Nephrol. (2003) 23:178–85. doi: 10.1159/000070747
Keywords: mendelian randomization study, diabetic nephropathy, causal inference, immunization, sensitivity analysis
Citation: Zhou Y, Zhang H, Yan H, Huang C and Liu Y (2024) Mendelian randomization based on immune cells in diabetic nephropathy. Front. Endocrinol. 15:1460652. doi: 10.3389/fendo.2024.1460652
Received: 06 July 2024; Accepted: 22 July 2024;
Published: 06 August 2024.
Edited by:
Yun Shen, Pennington Biomedical Research Center, United StatesReviewed by:
Yi Mu, ClinChoice Inc, United StatesCopyright © 2024 Zhou, Zhang, Yan, Huang and Liu. This is an open-access article distributed under the terms of the Creative Commons Attribution License (CC BY). The use, distribution or reproduction in other forums is permitted, provided the original author(s) and the copyright owner(s) are credited and that the original publication in this journal is cited, in accordance with accepted academic practice. No use, distribution or reproduction is permitted which does not comply with these terms.
*Correspondence: Yangwen Liu, MTcxNjI2NTkzOUBxcS5jb20=
†These authors have contributed equally to this work and share first authorship
Disclaimer: All claims expressed in this article are solely those of the authors and do not necessarily represent those of their affiliated organizations, or those of the publisher, the editors and the reviewers. Any product that may be evaluated in this article or claim that may be made by its manufacturer is not guaranteed or endorsed by the publisher.
Research integrity at Frontiers
Learn more about the work of our research integrity team to safeguard the quality of each article we publish.