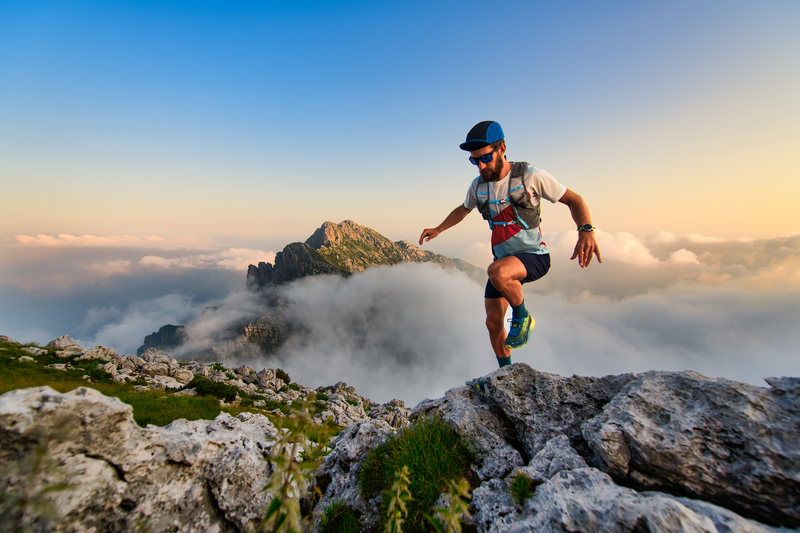
94% of researchers rate our articles as excellent or good
Learn more about the work of our research integrity team to safeguard the quality of each article we publish.
Find out more
ORIGINAL RESEARCH article
Front. Endocrinol. , 13 January 2025
Sec. Cardiovascular Endocrinology
Volume 15 - 2024 | https://doi.org/10.3389/fendo.2024.1438254
This article is part of the Research Topic The Role of Metabolic Syndrome and Disorders in Cardiovascular Disease - Volume II View all 19 articles
Background: The rising global prevalence of metabolic syndrome (MetS), characterized by a constellation of cardiovascular risk factors, underscores the urgent need to identify reliable predictive biomarkers. We hypothesize that an elevated atherogenic index of plasma (AIP) predicts MetS risk through lipid imbalance, but population-specific variations in its predictive strength remain unexplored. Our study aimed to assess AIP), a ratio of triglycerides to high-density lipoprotein cholesterol, as a predictor of MetS.
Method: Between 2014 and 2018, our cross-sectional study collected and analyzed health examination data from 9,202 Northern Taiwan Medical Center employees without cardiovascular diseases, diabetes, and end-stage renal disease (ESRD). Our study classified AIP levels equally into three tertiles and evaluated their impact on MetS through a logistic regression model.
Results: After adjusting for age, gender, BMI, SBP, FPG, and LDL in our models, the ORs for MetS in the second and third tertiles of the AIP were 3.81 (95% CI: 2.33 to 6.21; OR: 37.14, 95%: 23.22 to 59.39). In addition, women have a higher MetS risk associated with elevated AIP than men across all models.
Conclusion: Our research identified the AIP as a significant predictive marker for the prevalence of MetS, suggesting its potential utility in clinical risk assessment and indicating the need for further research to explore its application in preventive strategies and therapeutic interventions.
Metabolic syndrome (MetS) represents a clustering of cardiovascular risk factors that significantly elevate the risk of developing heart disease, stroke, and diabetes. These factors include increased blood pressure, high blood sugar, excess body fat around the waist, and abnormal cholesterol or triglyceride levels. The prevalence of MetS has been rising globally, concomitant with the obesity epidemic, making it a critical focus for public health initiatives (1–3). Therefore, identifying biomarkers that can predict the development of MetS is paramount for early intervention strategies.
The atherogenic index of plasma (AIP), a logarithmic calculation based on the ratio of triglycerides (TG) to high-density lipoprotein cholesterol (HDL-C), has emerged as a potent predictor of atherosclerosis and cardiovascular disease (CVD) (4–6). Recent studies suggest that the AIP could also be intricately linked to MetS, providing a simple yet effective tool for gauging metabolic health and the risk of cardiovascular complications (7–9). The relevance of the AIP as a predictive marker for MetS underscores the need for comprehensive research to further elucidate this relationship, which could lead to better predictive models for cardiovascular risk.
A 9-year longitudinal study in Taiwan highlighted AIP’s strong predictive value for MetS, hypertension, and T2DM, particularly among middle-aged individuals, while a 15-year study confirmed its role as an independent predictor of MetS in men, showing a significant linear trend with increasing tertiles. In India, AIP demonstrated the highest diagnostic accuracy (AUC 0.954) for MetS, and studies in chronic kidney disease and schizophrenia populations further emphasized its robust association with MetS risk factors. A Moroccan study linked elevated AIP, TG levels, and reduced HDL-C to increased cardiovascular risk, surpassing lipid measures alone (7–9). In a cross-sectional analysis, the Atherogenic Index of Plasma (AIP) was highlighted as a predictive marker for Metabolic Syndrome (MetS). A study of chronic kidney disease patients on hemodialysis found a strong correlation between elevated AIP and MetS prevalence, emphasizing its potential in cardiovascular risk management. In schizophrenia patients, AIP showed high diagnostic accuracy for MetS, with an AUC of 0.845 and a cutoff of 0.4. Similarly, research among Moroccan women demonstrated stronger associations of lipid ratios and AIP with cardiovascular risks than individual lipids, suggesting AIP’s vital role in identifying metabolic health risks across diverse populations (10–12). These findings underscore AIP’s critical value in early detection, risk stratification, and intervention strategies across various clinical and demographic settings.
The relationship between AIP and MetS is essential to address the rising prevalence of MetS, a key contributor to cardiovascular diseases and diabetes. AIP, a biomarker derived from TG and HDL-C, shows promise in predicting MetS risk across populations. However, the predictive strength of AIP varies, and population-specific insights are limited. Investigating AIP’s role in MetS can enhance early detection, risk stratification, and intervention strategies, bridging gaps in understanding its utility and offering a simple yet effective tool for managing cardiometabolic health across diverse clinical and demographic settings. This study aimed to explore the association between the AIP and MetS by leveraging a robust analytical approach to understand the extent of their correlation. Through a detailed analysis of the association between AIP and MetS, our research seeks to add a significant piece to the puzzle of metabolic health, with implications for clinical practices and public health policies.
In this longitudinal study, spanning from 2014 to 2018, we meticulously collected data from annual health examinations of 11,507 employees, encompassing both medical staff and general personnel, at a major medical center hospital located in Northern Taiwan. Following stringent exclusion criteria that removed participants with cardiovascular diseases, diabetes, and incomplete entries (n=2305) while ensuring privacy through encoding, a total of 9,202 employees aged between 20 and 80 years were included in the analysis. Detailed inclusion and exclusion criteria are shown in Figure 1.
Vital sign assessments were systematically conducted by trained nursing staff. Waist circumference was measured at the umbilical level in a standing posture to the nearest centimeter using tape with constant tension. Body mass index (BMI) was calculated by dividing the weight of the subjects in kilograms by their height in meters squared (kg/m2) (13). Blood pressure readings, including both systolic blood pressure (SBP) and diastolic blood pressure (DBP), were taken with the participants in a seated position using standard mercury sphygmomanometers following a rest period of five minutes. To ensure accuracy, blood pressure was measured twice per session with a 30- to 60-second interval between measurements, and the average of these readings was recorded (14).
Fasting blood samples were collected after a 12-hour fast in EDTA-containing tubes through venipuncture in a controlled setting. These samples were analyzed to determine the serum levels of total cholesterol, TG, high-density lipoprotein cholesterol (HDL-C), low-density lipoprotein cholesterol (LDL-C), alanine aminotransferase (ALT), and fasting plasma glucose (FBG). TG and TC levels were analyzed using enzymatic methods with a Fuji Dri-Chem analyzer, while HDL-C and LDL-C concentrations were determined using cholesterol assays following dextran sulfate precipitation. FBG was measured using the glucose oxidase method, and ALT levels were assessed through the International Federation of Clinical Chemistry method. The AIP was subsequently calculated using the formula AIP = log10(TG/HDL-C), which is a critical measure for assessing cardiovascular risk by evaluating the balance between triglycerides and HDL cholesterol. Metabolic syndrome is diagnosed based on the International Diabetes Federation Global Consensus Definition, which requires central obesity as a mandatory criterion, defined by WC with ethnicity-specific values, accompanied by any two of the following four factors: elevated TG (≥150 mg/dL or specific treatment for this lipid ab-normality), reduced HDL-C (<40 mg/dL in males and <50 mg/dL in females or specific treatment for this lipid abnormality), elevated blood pressure (SBP ≥130 or DBP ≥85 mm Hg or treatment of previously diagnosed hypertension), and elevated FBG (≥100 mg/dL or previously diagnosed type 2 diabetes) (15, 16).
The basic characteristics of categorical variables were expressed as counts and percentages, while those of continuous variables were described using means and standard deviations. AIP levels were categorized into three groups according to tertiles: T1 (≤ 33.3rd percentile), T2 (33.4th to 66.6th percentile), and T3 (> 66.6th percentile), with tertile comparisons conducted using ANOVA for continuous variables and the chi-square test for categorical variables. Pearson’s correlation analysis was utilized to explore the relationship between AIP levels and metabolic syndrome risk factors. Adhering to the STROBE statement, our analysis implemented three models: a univariate logistic regression model (model 1), a model adjusted for age and gender (model 2), and a fully adjusted model incorporating additional adjustments for BMI, SBP, FPG, and LDL-C (model 3). Statistical significance was indicated by two-tailed p-values less than 0.05. All analyses were conducted using PASW SPSS Statistics for Windows, version 26.0 (SPSS Inc., Chicago, IL, USA).
To further justify the statistical methods employed, this study utilized a comprehensive approach to ensure robust and reliable analysis of the relationship between AIP and MetS. The use of ANOVA for continuous variables allowed for detecting significant differences across tertile groups of AIP, while the chi-square test effectively identified associations in categorical data. Pearson’s correlation analysis was chosen to evaluate linear relationships between AIP and individual risk factors for MetS, including BMI, WC, TG, HDL-C, and fasting glucose. Logistic regression models were specifically selected to estimate odds ratios (ORs) for MetS across AIP tertiles, providing a clear understanding of risk magnitudes while adjusting for potential confounders in a stepwise manner. The univariate model (Model 1) identified baseline associations without adjustment, whereas Model 2 accounted for age and gender, addressing demographic variations. Model 3 further incorporated BMI, SBP, FPG, and LDL-C to control for metabolic and cardiovascular confounders, ensuring the robustness of the findings. Gender-specific subgroup analyses were performed to explore potential differences in AIP-MetS associations between men and women, providing critical insights into sex-based variations. The statistical software PASW SPSS Statistics version 26.0 was chosen for its reliability and advanced analytical capabilities, ensuring precise data handling, computation, and result presentation. Its comprehensive suite of statistical tools supported multivariate modeling, subgroup analysis, and hypothesis testing, aligning with the study’s objectives. The use of two-tailed p-values less than 0.05 as the threshold for statistical significance ensured rigorous and conservative interpretations, minimizing the likelihood of Type I errors.
Table 1 reveals the basic characteristics of the employees, equally stratified by AIP levels into three tertiles: T1 (<-0.3557), T2 (-0.3557 to -0.0858), and T3 (>-0.0858). The prevalence of MetS and the percentage of men significantly increased with higher AIP levels, with MetS affecting 39.88% of the population in T3 and only 0.81% in T1, and the proportion of men increased to 49.69% in T3 from 9.04% in T1. Continuous variables such as SBP, DBP, BMI, WC, and FPG also demonstrated significant differences across tertiles. Particularly notable were the changes in HDL-C levels, which significantly decreased from 69.88 mg/dL in T1 to 47.02 mg/dL in T3, underscoring the atherogenic risk associated with higher AIP levels.
Table 2 elucidates the correlation between the AIP and MetS risk factors, utilizing Pearson’s coefficient for analysis. Notably, BMI and WC demonstrated strong positive correlations with AIP, with coefficients of 0.48 and 0.55, respectively. Conversely, HDL-C exhibited a strong negative correlation with a coefficient of -0.73, indicating an inverse relationship with the AIP. TG had the highest positive correlation coefficient of 0.77, suggesting a significant link with AIP. These findings highlight the potential of the AIP as a significant marker for assessing metabolic syndrome risk, underscored by its strong associations with key risk factors such as BMI, WC, HDL-C, and TG.
Table 2. Correlation between the atherogenic index of plasma and associated risk factors for metabolic syndrome.
Table 3 shows the associations between the AIP and MetS across the three models. According to the unadjusted Model 1, individuals in the second tertile (T2) of the AIP had an odds ratio (OR) of 6.78 with a 95% confidence interval (CI) of 4.43 to 10.36, and those in the third tertile (T3) had an OR of 81.18 with a 95% CI of 54.51 to 121.13, both of which were significant, with p values less than 0.001. When adjusted for age and gender in Model 2, the ORs slightly decreased to 6.28 for T2 and 76.91 for T3, with 95% CIs of 4.10 to 9.62 and 51.31 to 115.28, respectively, maintaining significance at p values less than 0.001. Further adjustments in Model 3 for BMI, SBP, FPG, and LDL resulted in reduced ORs to 3.81 for T2 and 37.14 for T3, with 95% CIs of 2.33 to 6.21 and 23.22 to 59.39, respectively; however, these values were still significant, with p-values less than 0.001. These findings underscore the strong association between higher AIP levels and increased odds of metabolic syndrome, even after adjusting for key demographic and clinical variables.
Table 4 reveals the gender-specific analysis of the association between the atherogenic index of plasma (AIP) and metabolic syndrome (MetS), showing distinct variations between men and women. For men in the highest AIP tertile (T3), the odds ratio (OR) of developing MetS is significant across all models, decreasing from 42.24 in the unadjusted model to 22.58 in the fully adjusted model. Women, however, exhibit a stronger association, with an OR starting at 94.75 in the unadjusted model and decreasing to 37.18 in the fully adjusted model. The ORs for the second tertile (T2) also show significant differences, where men have lower ORs ranging from 2.53 in the unadjusted model to 1.50 in the fully adjusted model, whereas women’s ORs range from 8.22 to 4.44, indicating a consistently higher risk among women compared to men across all adjustments.
Table 4. Gender subgroup analysis of the association between atherogenic index of plasma and metabolic syndrome.
Figure 2 illustrates the prevalence of high levels (T3) of the atherogenic index of plasma (AIP) among individuals with and without metabolic syndrome (MetS). The data reveals a significant difference between the two groups. Among the 1,408 subjects diagnosed with metabolic syndrome, 86% exhibited a high AIP level, indicating a strong association between MetS and elevated AIP. In contrast, among the 7,794 sub-jects without metabolic syndrome, only 23% showed high AIP levels, suggesting a lower risk of atherogenic conditions in the absence of MetS. This disparity underscores the link between metabolic syndrome and cardiovascular risk factors.
Figure 2. The prevalence of high level (T3) of atherogenic index of plasma between metabolic syn-drome patients and non- metabolic syndrome patients. MetS, metabolic syndrome; AIP, atherogenic index of plasma; T3, Tertile 3.
Our study elucidates the robust association between elevated levels of the AIP and the increased prevalence and risk factors for MetS, demonstrating a graded relationship where higher AIP tertiles significantly correlate with a higher prevalence of MetS and its components, thus reaffirming the potential utility of the AIP as a predictive marker for MetS in a clinical setting. Besides, women show a stronger association between high AIP and MetS risk compared to men, with notably higher odds ratios across all models.
The possible biological mechanisms linking higher AIP to increased MetS risk may involve dyslipidemia, with dyslipidemia’s role in CVD highlighted by the critical diagnostic criteria of triglycerides and HDL-C in MetS and the demonstrated contribution of triglyceride-rich particles to the development and progression of atheromatous plaques (17–19). Triglyceride-rich lipoproteins, such as chylomicrons and very low-density lipoproteins (VLDL), play essential roles in lipid metabolism and energy homeostasis. These lipoproteins transport TG from the intestine and liver to peripheral tissues. The metabolism of these lipoproteins is significantly influenced by enzymes such as lipoprotein lipase, hepatic triglyceride lipase, and endothelial lipase (EL). LPL, anchored to the endothelial surface of capillaries, primarily in adipose tissue and muscle, hydrolyzes the triglycerides in chylomicrons and VLDL into free fatty acids and glycerol, which are then taken up by cells for energy production or storage. This hydrolysis process transforms chylomicrons into chylomicron remnants and VLDL into intermediate-density lipoproteins (IDL) and subsequently into LDL-C. HTGL, mainly found in the liver, further hydrolyzes triglycerides in IDL and chylomicron remnants, facilitating their conversion to LDL-C and their uptake by hepatic receptors, respectively. EL, while primarily hydrolyzing phospholipids, also influences the metabolism of TG-rich lipoproteins by modulating HDL metabolism and indirectly affecting plasma TG levels. Dysfunction in any of these enzymes can lead to elevated plasma triglyceride levels and contribute to metabolic disorders such as hypertriglyceridemia and CVD (20–26).
The observed gender-specific differences in the association between high AIP and MetS risk, as highlighted by notably higher odds ratios in women across all models, merit careful consideration. This variation may stem from inherent biological differences between men and women in lipid metabolism and cardiovascular risk profiles. Typically, women are known to have higher baseline levels of HDL-C, which could modulate the impact of AIP differently compared to men (27–30). Furthermore, hormonal differences, particularly the protective effects of estrogen, may influence lipid and glucose metabolism, altering the risk profile for MetS in premenopausal women (31, 32). However, this protective effect diminishes with age, aligning with an increased MetS risk as seen in the postmenopausal phase (33, 34). Additionally, genetic factors and lifestyle choices, which often vary between genders, might contribute to the observed disparities (35–37).
The strength of our study is its comprehensive approach and nuanced insights into the relationship between AIP and MetS. First, our robust data collection and longitudinal analysis span a significant period, from 2014 to 2018, capturing a large cohort of 9,202 employees with diverse backgrounds, ensuring a broad representation of the population. This extensive dataset allows for a detailed examination of trends and associations over time, enhancing the reliability of our findings. Second, the methodological rigor of categorizing AIP levels into tertiles and conducting a stratified analysis underscores the graded relationship between AIP and MetS, providing a nuanced understanding that higher AIP tertiles significantly correlate with an increased prevalence of MetS and its components. This stratification methodologically enriches the predictive utility of the AIP in a clinical setting. Third, our study benefits from a multivariable adjustment strategy that accounts for various confounding factors, including age, gender, BMI, blood pressure, and FBG levels. This approach ensures that the observed associations between AIP levels and MetS prevalence are not merely artifacts of these confounders but reflect a genuine underlying relationship. Last, the statistical analysis, grounded in Pearson’s correlation and logistic regression models, offers a robust framework for evaluating the strength and significance of the association between AIP and MetS, providing compelling evidence of AIP’s potential as a predictive marker for MetS in diverse clinical settings.
Our study has several inherent limitations. First, relying on a single medical center’s employee cohort might not fully represent the broader population. To mitigate this, we carefully selected a diverse sample of employees, encompassing both medical staff and general personnel, to enhance the generalizability of our findings. Moreover, we applied stringent exclusion criteria to ensure the data’s integrity and reliability, focusing on a well-defined and sizable cohort for analysis. Second, one of the primary constraints is the study’s observational nature, which, despite the robust longitudinal design, may not fully account for all potential confounding variables. To address this issue, we performed a comprehensive statistical analysis, adjusting for a wide range of demographic and clinical factors, such as age, gender, BMI, blood pressure, and FBG levels, to ensure that the observed associations were as accurate as possible. Third, calculating the AIP itself, a recognized marker for cardiovascular risk, depends heavily on accurate triglyceride and HDL cholesterol measurements. Therefore, we ensured that all blood samples were collected and analyzed following stringent, standardized protocols to minimize variability and enhance the reliability of the AIP calculations. Fourth, while our study provides significant insights into the predictive utility of the AIP for MetS, the evolving nature of MetS definitions and criteria poses challenges for longitudinal research. We navigated this by adhering to the most current and widely accepted diagnostic guidelines, allowing for a consistent and relevant assessment of MetS across the study period. Fifth, our study lacks data on dyslipidemia therapies, particularly statins, which could impact the model’s accuracy. Statin use may influence AIP levels and bias the results. According to a study using the Taiwan National Health Insurance Research Database (NHIRD), the use of statins has grown substantially over a decade. In 2011, approximately 6.3% of adults were identified as statin users (38). Sixth, our study highlights AIP’s association with moderate and high-risk MetS cases; future research should include longitudinal designs to evaluate cardiovascular outcomes like myocardial infarction. Additionally, since our study population consists primarily of working adults aged 20 to 65, the lower expected rate of statin use in this group reduces the potential bias on the model’s accuracy (39). Future research should further explore the mechanistic pathways linking AIP and MetS, with a focus on longitudinal and interventional studies to validate the predictive utility of AIP and explore potential therapeutic targets.
In conclusion, our research provides substantial evidence for the significant association between AIP and MetS, emphasizing its predictive value for MetS and related risk factors. The findings reinforce AIP’s utility as a clinically accessible biomarker, facilitating early diagnosis and personalized risk stratification across diverse populations. This study underscores the critical need for longitudinal research to elucidate the mechanisms underlying AIP’s role in metabolic pathways and its temporal relationship with cardiovascular outcomes, such as myocardial infarction. Future studies should incorporate larger, heterogeneous cohorts and advanced imaging techniques to explore AIP’s impact on subclinical atherosclerosis and microvascular complications. Additionally, integrating AIP assessments with genetic, proteomic, and metabolomic profiles could further refine its application in precision medicine. Clinical implications of this work suggest incorporating AIP into routine metabolic health evaluations to enhance early intervention strategies, ultimately improving outcomes in patients at high risk for MetS and cardiovascular diseases. This research marks a pivotal step toward bridging gaps in the literature and advancing metabolic health management frameworks.
The original contributions presented in the study are included in the article/supplementary material. Further inquiries can be directed to the corresponding author.
The studies involving humans were approved by Institute Review Board of the Chang-Gung Memorial Hospital (IRB No: 201901599B0). The studies were conducted in accordance with the local legislation and institutional requirements. The human samples used in this study were acquired from De-identified employee health examination database. Written informed consent for participation was not required from the participants or the participants' legal guardians/next of kin in accordance with the national legislation and institutional requirements.
L-SC: Conceptualization, Data curation, Methodology, Project administration, Writing – original draft, Writing – review & editing. Y-RC: Data curation, Investigation, Writing – original draft. Y-HL: Formal analysis, Investigation, Methodology, Writing – original draft. H-KW: Investigation, Methodology, Writing – original draft. YL: Formal analysis, Investigation, Methodology, Writing – original draft. J-YC: Data curation, Formal analysis, Methodology, Project administration, Writing – original draft, Writing – review & editing, Supervision.
The author(s) declare financial support was received for the research, authorship, and/or publication of this article.
The authors declare that the research was conducted in the absence of any commercial or financial relationships that could be construed as a potential conflict of interest.
All claims expressed in this article are solely those of the authors and do not necessarily represent those of their affiliated organizations, or those of the publisher, the editors and the reviewers. Any product that may be evaluated in this article, or claim that may be made by its manufacturer, is not guaranteed or endorsed by the publisher.
1. Lemieux I, Despres JP. Metabolic syndrome: past, present and future. Nutrients. (2020) 12:3501–8. doi: 10.3390/nu12113501
2. Ansari-Moghaddam A, Adineh HA, Zareban I, Kalan Farmanfarma KH. Prevalence of metabolic syndrome and population attributable risk for cardiovascular, stroke, and coronary heart diseases as well as myocardial infarction and all-cause mortality in middle-east: systematic review & Meta-analysis. Obes Med. (2019) 14:100086. doi: 10.1016/j.obmed.2019.100086
3. Sedaghat Z, Khodakarim S, Nejadghaderi SA, Sabour S. Association between metabolic syndrome and myocardial infarction among patients with excess body weight: A systematic review and meta-analysis. BMC Public Health. (2024) 24:444. doi: 10.1186/s12889-024-17707-7
4. Li Y, Feng Y, Li S, Ma Y, Lin J, Wan J, et al. The atherogenic index of plasma (Aip) is a predictor for the severity of coronary artery disease. Front Cardiovasc Med. (2023) 10:1140215. doi: 10.3389/fcvm.2023.1140215
5. Lioy B, Webb RJ, Amirabdollahian F. The association between the atherogenic index of plasma and cardiometabolic risk factors: A review. Healthcare. (2023) 11:966. doi: 10.3390/healthcare11070966
6. Ulloque-Badaracco JR, Hernandez-Bustamante EA, Alarcon-Braga EA, Mosquera-Rojas MD, Campos-Aspajo A, Salazar-Valdivia FE, et al. Atherogenic index of plasma and coronary artery disease: A systematic review. Open Med (Wars). (2022) 17:1915–26. doi: 10.1515/med-2022-0590
7. Li YW, Kao TW, Chang PK, Chen WL, Wu LW. Atherogenic index of plasma as predictors for metabolic syndrome, hypertension and diabetes mellitus in Taiwan citizens: A 9-year longitudinal study. Sci Rep. (2021) 11:9900. doi: 10.1038/s41598-021-89307-z
8. Sabarinathan M, Deepak Rajan DS, Ananthi N, Krishnan M. Atherogenic index of plasma, lipid accumulation and visceral adiposity in metabolic syndrome patients. Bioinformation. (2022) 18:1109–13. doi: 10.6026/973206300181109
9. Zhang X, Zhang X, Li X, Feng J, Chen X. Association of metabolic syndrome with atherogenic index of plasma in an urban chinese population: A 15-year prospective study. Nutr Metab Cardiovasc Dis. (2019) 29:1214–9. doi: 10.1016/j.numecd.2019.07.006
10. Solis ALG, Cardoza RH, Banik SD, González RMM. Atherogenic index of plasma is the best predictor of metabolic syndrome among mexican adult patients with chronic kidney disease on hemodialysis. Ciencia y Humanismo en la Salud. (2021) 8:38–44. doi: 10.2147/DMSO.S281894.eCollection2021
11. Tien YT, Wang LJ, Lee Y, Lin PY, Hung CF, Chong MY, et al. Comparative predictive efficacy of atherogenic indices on metabolic syndrome in patients with schizophrenia. Schizophr Res. (2023) 262:95–101. doi: 10.1016/j.schres.2023.10.023
12. Essiarab F, Taki H, Lebrazi H, Sabri M, Saïle R. Usefulness of lipid ratios and atherogenic index of plasma in obese Moroccan women with or without metabolic syndrome. Ethnicity Dis. (2014) 24:207–12.
13. Khanna D, Peltzer C, Kahar P, Parmar MS. Body mass index (Bmi): A screening tool analysis. Cureus. (2022) 14:e22119. doi: 10.7759/cureus.22119
14. Stergiou GS, Palatini P, Parati G, O’Brien E, Januszewicz A, Lurbe E, et al. 2021 European society of hypertension practice guidelines for office and out-of-Office blood pressure measurement. J Hypertens. (2021) 39:1293–302. doi: 10.1097/HJH.0000000000002843
15. Nilsson PM, Tuomilehto J, Rydén L. The metabolic syndrome - what is it and how should it be managed? Eur J Prev Cardiol. (2019) 26:33–46. doi: 10.1177/2047487319886404
16. Ambroselli D, Masciulli F, Romano E, Catanzaro GA-O, Besharat ZA-O, Massari MC, et al. New advances in metabolic syndrome, from prevention to treatment: the role of diet and food. Nutrients. (2023) 15:640. doi: 10.3390/nu15030640
17. Su X, Peng D. New insight into sortilin in controlling lipid metabolism and the risk of atherogenesis. Biol Rev Camb Philos Soc. (2019) 95:232–43. doi: 10.1111/brv.12561
18. Summerhill VI, Grechko AV, Yet SF, SoBenin IA, Orekhov AN. The atherogenic role of circulating modified lipids in atherosclerosis. Int J Mol Sci. (2019) 20:3561. doi: 10.3390/ijms20143561
19. Kotlyarov S. Diversity of lipid function in atherogenesis: A focus on endothelial mechanobiology. Int J Mol Sci. (2021) 22:11545. doi: 10.3390/ijms222111545
20. Sascău R, Clement A, Radu R, Prisacariu C, Stătescu C. Triglyceride-rich lipoproteins and their remnants as silent promoters of atherosclerotic cardiovascular disease and other metabolic disorders: A review. Nutrients. (2021) 13: 699-729. doi: 10.3390/nu13061774
21. Kersten S. Physiological regulation of lipoprotein lipase. Biochim Biophys Acta. (2014) 1841:919–33. doi: 10.1016/j.bbalip.2014.03.013
22. Dallinga-Thie GM, Franssen R, Mooij HL, Visser ME, Hassing HC, Peelman F, et al. The metabolism of triglyceride-rich lipoproteins revisited: new players, new insight. Atherosclerosis. (2010) 211:1–8. doi: 10.1016/j.atherosclerosis.2009.12.027
23. Wang H, Eckel RH. Lipoprotein lipase: from gene to obesity. Am J Physiol Endocrinol Metab. (2009) 297:E271–88. doi: 10.1152/ajpendo.90920.2008
24. Kobayashi J, Miyashita K, Nakajima K, Mabuchi H. Hepatic lipase: A comprehensive view of its role on plasma lipid and lipoprotein metabolism. J Atheroscler Thromb. (2015) 22:1001–11. doi: 10.5551/jat.31617
25. Khetarpal SA, Vitali C, Levin MG, Klarin D, Park J, Pampana A, et al. Endothelial lipase mediates efficient lipolysis of triglyceride-rich lipoproteins. PloS Genet. (2021) 17:e1009802. doi: 10.1371/journal.pgen.1009802
26. Packard CJ, Boren J, Taskinen MR. Causes and consequences of hypertriglyceridemia. Front Endocrinol (Lausanne). (2020) 11:252. doi: 10.3389/fendo.2020.00252
27. Vynckier P, De Sutter J, De Pauw M, Vandekerckhove H, De Backer G, Vervaet P, et al. Gender differences in risk factor management and pharmacological treatment among chd patients: Belgian results of the Euroaspire IV and Euroaspire V surveys. Acta Cardiol. (2023) 78:607–13. doi: 10.1080/00015385.2023.2169439
28. Vynckier P, Ferrannini G, Ryden L, Jankowski P, De Backer T, Gevaert S, et al. Gender gap in risk factor control of coronary patients far from closing: results from the European society of cardiology Euroaspire V registry. Eur J Prev Cardiol. (2022) 29:344–51. doi: 10.1093/eurjpc/zwaa144
29. Khoja A, Andraweera PH, Lassi ZS, Ali A, Zheng M, Pathirana MM, et al. Risk factors for premature coronary heart disease in women compared to men: systematic review and meta-analysis. J Womens Health (Larchmt). (2023) 32:908–20. doi: 10.1089/jwh.2022.0517
30. Robinson GA, Pineda-Torra I, Ciurtin C, Jury EC. Sex differences in lipid metabolism: implications for systemic lupus erythematosus and cardiovascular disease risk. Front Med (Lausanne). (2022) 9:914016. doi: 10.3389/fmed.2022.914016
31. Palmisano BT, Zhu L, Eckel RH, Stafford JM. Sex differences in lipid and lipoprotein metabolism. Mol Metab. (2018) 15:45–55. doi: 10.1016/j.molmet.2018.05.008
32. Ndzie Noah ML, Adzika GK, Mprah R, Adekunle AO, Adu-Amankwaah J, Sun H. Sex-gender disparities in cardiovascular diseases: the effects of estrogen on enos, lipid profile, and nfats during catecholamine stress. Front Cardiovasc Med. (2021) 8:639946. doi: 10.3389/fcvm.2021.639946
33. Ko S-H, Kim H-S. Menopause-associated lipid metabolic disorders and foods beneficial for postmenopausal women. Nutrients. (2020) 12: 1-25. doi: 10.3390/nu12010202
34. Lee SW, Hwang IS, Jung G, Kang HJ, Chung YH. Relationship between metabolic syndrome and follicle-stimulating hormone in postmenopausal women. Medicine. (2022) 101:e29027. doi: 10.1097/MD.0000000000029216
35. Hattori T, Konno S, Munakata M. Gender differences in lifestyle factors associated with metabolic syndrome and preliminary metabolic syndrome in the general population: the watari study. Intern Med. (2017) 56:2253–9. doi: 10.2169/internalmedicine.8578-16
36. Santilli F, D’Ardes D, Guagnano MT, Davi G. Metabolic syndrome: sex-related cardiovascular risk and therapeutic approach. Curr Med Chem. (2017) 24:2602–27. doi: 10.2174/0929867324666170710121145
37. Pucci G, Alcidi R, Tap L, Battista F, Mattace-Raso F, Schillaci G. Sex- and gender-related prevalence, cardiovascular risk and therapeutic approach in metabolic syndrome: A review of the literature. Pharmacol Res. (2017) 120:34–42. doi: 10.1016/j.phrs.2017.03.008
38. Hsieh HC, Hsu JC, Lu CY. 10-year trends in statin utilization in Taiwan: A retrospective study using Taiwan’s national health insurance research database. BMJ Open. (2017) 7:e014150. doi: 10.1136/bmjopen-2016-014150
Keywords: atherogenic index of plasma, triglycerides, high-density lipoprotein cholesterol, metabolic syndrome, women
Citation: Chen L-S, Chen Y-R, Lin Y-H, Wu H-K, Lee YW and Chen J-Y (2025) Evaluating atherogenic index of plasma as a predictor for metabolic syndrome: a cross-sectional analysis from Northern Taiwan. Front. Endocrinol. 15:1438254. doi: 10.3389/fendo.2024.1438254
Received: 25 May 2024; Accepted: 16 December 2024;
Published: 13 January 2025.
Edited by:
Carmine Izzo, University of Salerno, ItalyReviewed by:
Nana Liu, Cleveland Clinic, United StatesCopyright © 2025 Chen, Chen, Lin, Wu, Lee and Chen. This is an open-access article distributed under the terms of the Creative Commons Attribution License (CC BY). The use, distribution or reproduction in other forums is permitted, provided the original author(s) and the copyright owner(s) are credited and that the original publication in this journal is cited, in accordance with accepted academic practice. No use, distribution or reproduction is permitted which does not comply with these terms.
*Correspondence: Jau-Yuan Chen, d2VsaW5zQGNnbWgub3JnLnR3
Disclaimer: All claims expressed in this article are solely those of the authors and do not necessarily represent those of their affiliated organizations, or those of the publisher, the editors and the reviewers. Any product that may be evaluated in this article or claim that may be made by its manufacturer is not guaranteed or endorsed by the publisher.
Research integrity at Frontiers
Learn more about the work of our research integrity team to safeguard the quality of each article we publish.