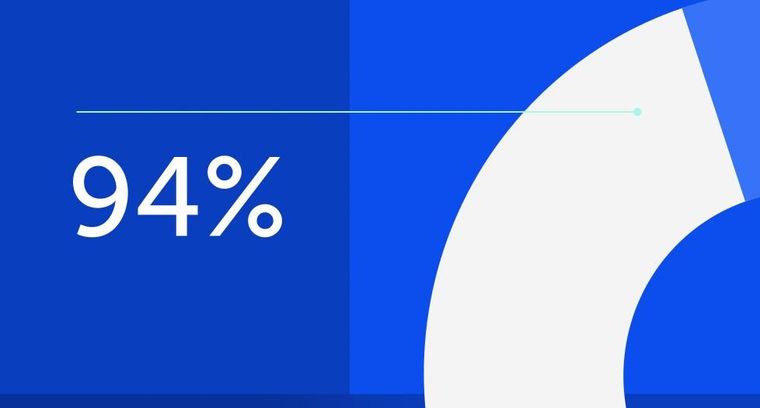
94% of researchers rate our articles as excellent or good
Learn more about the work of our research integrity team to safeguard the quality of each article we publish.
Find out more
ORIGINAL RESEARCH article
Front. Endocrinol., 04 September 2024
Sec. Reproduction
Volume 15 - 2024 | https://doi.org/10.3389/fendo.2024.1424033
Background: Infertility is a fertility disorder caused by various factors, with lipid metabolism playing a crucial role in its development. The cardiometabolic index (CMI), which combines blood lipids (TG/HDL-C) and obesity-related parameters (WHtR), is a new quantitative indicator. This study used NHANES data to investigate the relationship between CMI and the incidence of infertility.
Methods: We utilized data from women who took part in the National Health and Nutrition Examination Survey (NHANES) from 2015 to March 2020 to calculate the CMI index. Subsequently, we used multivariate logistic regression, smooth curve fitting, and subgroup analysis to investigate the relationship between the CMI index and infertility.
Results: The logistic regression model revealed a significant positive correlation between the CMI index and infertility, even after adjusting for all confounding variables (OR=3.23, 95%CI: 1.55-6.73, p=0.0017). This association remained consistent across all subgroups (p>0.05 for all interactions). Smooth curve fitting demonstrated a positive nonlinear relationship between CMI and infertility. However, the CMI index had limited diagnostic power for infertility (AUC=0.60, 95%CI: 0.56-0.65). However, the CMI-BMI index combined with BMI had good predictive performance (AUC=0.722, 95%CI: 0.676-0.767).
Conclusion: The CMI index shows a positive correlation with infertility, but its diagnostic value is restricted. The combination with BMI has good diagnostic value. Further investigation is required to determine the effectiveness of the CMI index as an early indicator of infertility.
Infertility is defined as the inability to become pregnant after engaging in regular, unprotected sexual intercourse for over a year without using any form of contraception (1, 2). Around 7% to 15.5% of women in the United States who are of childbearing age experience infertility (3). Infertility is considered a significant public health issue by the World Health Organization (WHO), impacting approximately 186 million individuals globally. The condition affects up to 15% of women in their childbearing years (4–6). It is estimated that over half of women who are unable to conceive suffer from severe mental health conditions like depression, anxiety, and social dysregulation.
Various epidemiological studies have revealed that infertility is a fertility disorder with multiple causes. Previous studies have shown that factors such as obesity, alcohol consumption, smoking, education level, and past medical history (1, 7–9) are associated with female infertility. Another retrospective study highlighted that smoking was associated with increased PSA levels in men undergoing radical prostatectomy (10). Therefore, the role of lifestyle interventions may not be limited to the management of metabolic markers, but may be critical in the prevention and management of chronic diseases more broadly. Recent research has shown that female infertility caused by polycystic ovary syndrome, obesity, thyroid dysfunction, and endometriosis is linked to a higher risk of cardiovascular disease (CVD) (11). Women with infertility have a higher prevalence of cardiovascular risk factors even before pregnancy (12), suggesting that common risk factors such as diabetes, chronic hypertension (13) and obesity (14) may underlie infertility and cardiovascular disease. Cardiovascular disease (CVD) has become the leading cause of death worldwide, and although it can be effectively treated, there are still serious complications that seriously affect the quality of life (15). Research has indicated a strong correlation between female infertility and metabolic syndrome, as well as its individual components like dyslipidemia and hypertension, in the later stages of life (16). Considering the correlation between Mets, CVD, and infertility, we hypothesize that there could be a connection between CMI and infertility.
The cardiometabolic index is a novel measure that combines triglycerides, high-density lipoprotein cholesterol, and waist-to-height ratio. It serves as an indicator of visceral adipose tissue distribution and dysfunction, providing insight into an individual’s blood lipid levels and degree of obesity (17–19). The TG/HDL-C ratio has been proven to be a reliable risk factor for cardiovascular disease for a long time (20). In addition, studies have reported that TG/HDL-C ratios are strongly associated with insulin resistance (21) and Mets (22). It is a commonly known fact that waist circumference (WC) is a simple indicator of abdominal obesity, while waist-to-height ratio (WHtR) is considered to be more accurate than waist circumference (23). WHtR has been proven to be a more effective indicator of cardiovascular disease risk factors and infertility when compared to body mass index (BMI) and waist circumference (WC) (24, 25). At present, the link between lipid levels and infertility remains uncertain. Therefore, we analyzed data from the National Health and Nutrition Survey (NHANES) in order to investigate the association between CMI and the prevalence of infertility.
The data for this study were obtained from the National Health and Nutrition Survey (NHANES), a program conducted by the National Center for Health Statistics (NCHS) to evaluate nutrition and health in the United States. The survey utilized a complex multi-stage probabilistic design to ensure a nationally representative sample of non-institutionalized Americans. Participants underwent a family interview to provide information on their health, socioeconomic status, and other relevant factors. Physical and laboratory examinations were then conducted in a mobile examination facility.
Approval for all NHANES study methods was granted by the NCHS Research Ethics Review Committee, with written informed consent obtained from all survey respondents. The CDC website (www.cdc.gov/nchs/nhanes/) provides access to detailed NHANES study design and data for the public. This cross-sectional study followed the criteria for enhanced epidemiological observational reporting (24).
Health problems related to infertility were only included in the NHANES cycle from 2015 to 2020; Therefore, we use these periods as our data. In our analysis, we included participants with comprehensive information on infertility and CMI. Initially, a total of 25,531 participants participated. After excluding male participants (n=12,613), participants lacking CMI data (n=9,334), fertility information (n=1,580), and women older than 45 years and younger than 18 years (n=724), our final analysis included 1,280 eligible participants (Figure 1).
CMI is calculated using anthropometric indicators obtained from blood samples. Rigorous testing and measurement protocols were followed to ensure the accuracy and consistency of the data. Blood samples were collected either on a research vehicle or at a specific location, and then analyzed in a laboratory. Height and waist circumference of participants were measured by trained health professionals at a mobile screening unit. Using these indicators, WHtR and CMI were calculated individually.
The reproductive health questionnaire for each woman was self-reported as a dependent variable for infertility (name of variable in questionnaire: RHQ 074). The researchers surveyed the subjects, asking questions such as “Trying to conceive for a year?” (26). If the answer is “yes”, it means “sterile”; If the answer is not “yes”, it means “fertile”.
Covariates such as sex, age, ethnicity, education level, income-to-poverty ratio (PIR), body mass index (BMI), alcohol use, smoking status, hypertension status, diabetes status, age at menarche, previous treatment for pelvic infection/pelvic inflammatory disease, regular menstruation within 12 months, HDL-C, TC, TG, and LDL-C were all included in the analysis.
The statistical analyses for this study were carried out in accordance with Centers for Disease Control and Prevention (CDC) guidelines. When we divided the 2-year weights for each cycle by 2, we obtained the new sample weights for the combined survey periods.
In this study, we compared two groups based on infertility status using weighted Student t-tests for continuous variables and weighted chi-square tests for categorical data. Categorical data were presented as proportions, while continuous variables were described with mean and standard deviation. We used weighted multiple linear and logistic regression to analyze the linear relationship between CMI and infertility. By categorizing CMI into thirds, we conducted a trend test to assess the linear association with infertility. Subgroup analysis was performed to explore how factors such as age, BMI, hypertension, diabetes, menstrual regularity, and menarche age influenced the relationship between CMI and infertility, with interaction tests to assess consistency across subgroups. We also examined the nonlinear relationship between CMI and infertility using smoothing curve fitting. The diagnostic accuracy of the CMI index for infertility was assessed using the ROC curve and calculating the AUC. Statistical analysis was carried out using R Studio (version 4.3.2), with significance set at p<0.05. A weighting strategy was implemented to minimize dataset fluctuations.
A total of 1,280 participants between the ages of 18 and 45 were included in the study, with 132 of them being infertility patients. The characteristics of the study participants are detailed in Table 1 based on their infertility status. Older women with a higher BMI, alcohol intake, high blood pressure, and diabetes were found to have a higher prevalence of self-reported infertility. Additionally, women with a higher CMI, averaging 0.54 ± 0.41, also reported higher rates of infertility. At the same time, women with higher triglycerides (TG) also have a higher prevalence of infertility.
Table 2 displays the association between CMI and infertility. The findings revealed that as CMI levels increased, the risk of infertility also increased (OR=3.23, 95%CI:1.55, 6.7, p=0.0017) after adjusting for all variables. Even after categorizing CMI into tritiles, the correlation remained statistically significant. Individuals in the highest CMI quartile had a higher risk of infertility compared to those in the lowest CMI tritile (OR=3.68, 95%CI:1.66-8.17). Furthermore, the results from the smooth-fitting curve supported a linear positive relationship between CMI and infertility (see Figure 2).
We conducted subgroup analyses to determine if the association between CMI and infertility remained consistent across different factors. Our results indicate that there is no link between CMI and infertility. As demonstrated in Table 3, variables such as age, BMI, hypertension, diabetes, age at menarche, and menstrual regularity over the past year did not have a significant impact on the positive relationship between CMI and infertility (all p>0.05).
The diagnostic validity of the CMI index was assessed through the receiver operating characteristic (ROC) curve (Figure 3), revealing a cut-off value of 0.345 for diagnosing infertility. The AUC was 0.60 (95%CI: 0.56-0.65) with a sensitivity of 65.2% and specificity of 53.8%. However, we found that CMI in combination with BMI can improve its diagnostic utility. The cut-off value of CMI-BMI index was 12.652, the AUC was 0.722 (95%CI: 0.676-0.767), the sensitivity was 68.4%, and the specificity was 67.7%.
The study examined the connection between CMI levels and infertility using data from the NHANES database. It found that CMI levels were higher in the infertility group compared to the non-infertility group, and that infertility risk increased with higher CMI levels. Smooth curve fitting analysis revealed a positive linear relationship between CMI and infertility. Even after adjusting for various factors, there was still a significant correlation between CMI and infertility, suggesting that CMI could be a useful indicator for assessing infertility in the future. However, the diagnostic accuracy of the CMI index for infertility is limited, and more research is needed to fully explore its potential as an early predictor of infertility risk. However, the combination of CMI and BMI is more useful in diagnosing infertility, probably because CMI-BMI contains not only blood lipids and cardiometabolic abnormalities compared to the CMI index, but also the BMI, one of the obesity indexes, to improve its diagnostic ability.
This study is believed to be the first to examine the connection between CMI and infertility. Wakabayashi et al. initially introduced CMI, which combines indicators of blood lipids and obesity (TG/HDL-C, WHtR) in a novel way, demonstrating that CMI is a valuable new tool for detecting diabetes mellitus (18). Yin et al. discovered that abdominal obesity, which is defined by the presence of visceral fat, is closely linked to metabolic disorders. Their analysis of 1509 female adults in the United States revealed a strong correlation between waist circumference and/or abdominal obesity and a heightened risk of infertility (27). Both infertility and obesity are often associated with high serum lipid concentrations and increased cortisol levels. It is worth noting that most obese individuals tend to have consistently elevated blood lipid levels, which can create a harmful cycle (28). In this study, it was found that having a BMI of 30kg/m2 or higher can increase the risk of infertility. This may be due to the fact that BMI is closely associated with obesity-related metabolic disorders, leading to the accumulation of visceral fat and an increased risk of cardiovascular disease. A randomized trial involving women aged 18 to 40 years also showed that elevated levels of triglycerides, total cholesterol, and LDL cholesterol, as well as decreased levels of HDL cholesterol, were linked to a higher risk of female infertility (29). Cai et al. carried out a randomized controlled trial involving 1000 patients diagnosed with PCOS. They examined the impact of preconception LDLC, HDL-C, and TG levels on pregnancy outcomes and discovered a negative correlation between serum lipid concentration and reproductive results. Additionally, they determined that high LDL-C levels were linked to ovulation, clinical pregnancy, and a decreased likelihood of live birth (30). Our study revealed that elevated levels of triglycerides (TG) were linked to female infertility, while the impact of low-density lipoprotein cholesterol (LDL-C) on female infertility was not significant. Furthermore, infertility was strongly correlated with individuals who had hypertension or diabetes. These findings suggest that infertility may be influenced by lipid metabolism disorders, particularly in individuals with hypertension and diabetes. Additionally, infertility could potentially disrupt lipid metabolism and increase the risk of cardiovascular diseases in affected individuals. In addition to the above systemic metabolic factors, local reproductive pathology also plays a key role in infertility. One study highlighted the prevalence of varicocele in men with primary infertility by up to 35% (19), suggesting that we can propose a multifaceted approach to infertility treatment and research by exploring the local link between these systemic metabolic disorders and varicocele.
CMI is a new method for assessing visceral fat distribution and dysfunction that can better predict the presence of diabetes, hypertension, and related cardiometabolism, as well as other more important fundamental aspects (31–33). The CMI is calculated by multiplying WHtR with TG/HDL-L. WHtR indicates the presence of fat in the body, and it has been found that abdominal obesity is strongly linked to infertility (34). TG/HDL-C is a strong predictor of metabolic syndrome and insulin resistance, playing a crucial role in the onset of infertility (35). The development of infertility and metabolic disorders is intricate and varied, with lipid metabolism disorders potentially playing a crucial role in follicular development, egg maturation, and hormone secretion (36). Women who follow a long-term high-fat diet may experience an accumulation of lipids in their eggs, leading to toxicity and hindering the maturation process (37). A large number of animal experiments have confirmed that dyslipidemia can lead to decreased female reproductive ability (38), and low-density lipoprotein receptor (LDLR) is a key factor in regulating lipid metabolism (39). Deletion of LDLR leads to dyslipidemia, which markedly decreases estrogen levels and fertility in female mice (40). Studies have shown that lipid metabolism disorders are associated with the reproductive performance of assisted reproductive technology, and hyperlipidemia is inversely correlated with pregnancy outcomes in patients with freeze-thaw embryo transfer (41). Other studies have shown that women with abnormal lipid metabolism have a higher risk of infertility (42). Hence, CMI has the ability to indicate the abnormal metabolic state within the body, allowing for the prediction of infertility risk and serving as a foundation for health promotion.
Our study benefits from a complex multi-stage probabilistic sampling design, which enhances the reliability and representativeness of our research. However, there are limitations to our study. Firstly, the cross-sectional analysis design prevents us from establishing a causal relationship between CMI and infertility. Additionally, due to database constraints, we were unable to include data on all covariates that may impact infertility and lipids in order to maintain a large enough sample size. Lastly, while we accounted for some confounders, we cannot completely eliminate the potential influence of other confounding factors. For example, polycystic ovary syndrome is a very common and important cause of infertility, but our study did not include people with PCOS, which may bias our findings. In addition, when performing sample exclusion, we only selected samples of women of childbearing age based on previous studies, which may have led to biased research results.
The prevalence of infertility in women in the United States is linked to CMI levels, with a higher CMI level corresponding to a higher prevalence of infertility. CMI has limited power in diagnosing infertility, and interestingly, the CMI-BMI index has good diagnostic power and can be used for early diagnosis of infertility. However, further prospective studies with large sample sizes are needed to confirm this relationship.
The datasets presented in this study can be found in online repositories. The names of the repository/repositories and accession number(s) can be found in the article/supplementary material.
HC: Writing – original draft, Formal Analysis, Data curation, Conceptualization. XJ: Writing – review & editing, Visualization, Validation, Supervision, Funding acquisition. XH: Writing – original draft, Methodology, Formal Analysis, Data curation.
The author(s) declare that no financial support was received for the research, authorship, and/or publication of this article.
The authors express their gratitude towards the participants and staff of the NHANES database for their noteworthy contributions.
The authors declare that the research was conducted in the absence of any commercial or financial relationships that could be construed as a potential conflict of interest.
All claims expressed in this article are solely those of the authors and do not necessarily represent those of their affiliated organizations, or those of the publisher, the editors and the reviewers. Any product that may be evaluated in this article, or claim that may be made by its manufacturer, is not guaranteed or endorsed by the publisher.
1. Broughton DE, Moley KH. Obesity and female infertility: potential mediators of obesity’s impact. Fertility Sterility. (2017) 107:840–7. doi: 10.1016/j.fertnstert.2017.01.017
2. Caballero B. Humans against obesity: who will win? Adv Nutr. (2019) 10:S4–9. doi: 10.1093/advances/nmy055
3. Ding C, Shi Y, Li J, Li M, Hu L, Rao J, et al. Association of weight-adjusted-waist index with all-cause and cardiovascular mortality in China: A prospective cohort study. Nutrition Metab Cardiovasc Diseases. (2022) 32:1210–7. doi: 10.1016/j.numecd.2022.01.033
4. Buzadzic B, Vucetic M, Jankovic A, Stancic A, Korac A, Korac B, et al. New insights into male (in)fertility: the importance of NO. Br J Pharmacol. (2014) 172:1455–67. doi: 10.1111/bph.12675
5. Palermo GD, O’Neill CL, Chow S, Cheung S, Parrella A, Pereira N, et al. Intracytoplasmic sperm injection: state of the art in humans. Reproduction. (2017) 154:F93–F110. doi: 10.1530/REP-17-0374
6. Yokota R, Okuhara T, Ueno H, Okada H, Furukawa E, Kiuchi T. Online Japanese-language information on lifestyle factors associated with reduced fertility: content analysis. J Med Internet Res. (2020) 22(8):e19777. doi: 10.2196/19777
7. Zhou Z, Zheng D, Wu H, Li R, Xu S, Kang Y, et al. Epidemiology of infertility in China: a population-based study. BJOG. (2018) 125:432–41. doi: 10.1111/1471-0528.14966
8. Finelli R, Mottola F, Agarwal A. Impact of alcohol consumption on male fertility potential: A narrative review. Int J Environ Res Public Health. (2021) 19(1):328. doi: 10.3390/ijerph19010328
9. Wesselink AK, Hatch EE, Rothman KJ, Mikkelsen EM, Aschengrau A, Wise LA. Prospective study of cigarette smoking and fecundability. Hum Reprod. (2019) 34:558–67. doi: 10.1093/humrep/dey372
10. Tarantino G, Crocetto F, Vito CD, Martino R, Pandolfo SD, Creta M, et al. Clinical factors affecting prostate-specific antigen levels in prostate cancer patients undergoing radical prostatectomy: a retrospective study. Future Sci OA. (2021) 7:FSO643. doi: 10.2144/fsoa-2020-0154
11. Verit FF, Yildiz Zeyrek F, Zebitay AG, Akyol H. Cardiovascular risk may be increased in women with unexplained infertility. Clin Exp Reprod Med. (2017) 44(1):28–32. doi: 10.5653/cerm.2017.44.1.28
12. Udell JA, Lu H, Redelmeier DA. Long-term cardiovascular risk in women prescribed fertility therapy. J Am Coll Cardiol. (2013) 62:1704–12. doi: 10.1016/j.jacc.2013.05.085
13. Luke B, Gopal D, Cabral H, Stern JE, Diop H. Adverse pregnancy, birth, and infant outcomes in twins: effects of maternal fertility status and infant gender combinations; the Massachusetts Outcomes Study of Assisted Reproductive Technology. Am J Obstetrics Gynecology. (2017) 217:330.e1–.e15. doi: 10.1016/j.ajog.2017.04.025
14. DeAngelis AM, Roy-O’Reilly M, Rodriguez A. Genetic alterations affecting cholesterol metabolism and human fertility1. Biol Reprod. (2014) 91(5):117. doi: 10.1095/biolreprod.114.119883
15. Zhao D, Liu J, Wang M, Zhang X, Zhou M. Epidemiology of cardiovascular disease in China: current features and implications. Nat Rev Cardiol. (2018) 16:203–12. doi: 10.1038/s41569-018-0119-4
16. O’Kelly AC, Michos ED, Shufelt CL, Vermunt JV, Minissian MB, Quesada O, et al. Pregnancy and reproductive risk factors for cardiovascular disease in women. Circ Res. (2022) 130:652–72. doi: 10.1161/CIRCRESAHA.121.319895
17. Acosta García EJ, Paez MC. [amp]]Iacute;ndice cardiometabólico como predictor de factores de riesgo cardiovascular en adolescentes. Rev Salud Pública. (2018) 20:340–5. doi: 10.15446/rsap.V20n3.61259
18. Wakabayashi I, Daimon T. The “cardiometabolic index” as a new marker determined by adiposity and blood lipids for discrimination of diabetes mellitus. Clinica Chimica Acta. (2015) 438:274–8. doi: 10.1016/j.cca.2014.08.042
19. Napolitano L, Pandolfo SD, Aveta A, Cirigliano L, Martino R, Mattiello G, et al. The management of clinical varicocele: robotic surgery approach. Front Reprod Health. (2022) 4:791330. doi: 10.3389/frph.2022.791330
20. Yu H, Tao L, Li Y-G, Yang L, Liu D, Wang Y, et al. Association between triglyceride-glucose index trajectories and carotid atherosclerosis progression. Cardiovasc Diabetol. (2023) 22(1):130. doi: 10.1186/s12933-023-01847-y
21. McLaughlin T, Reaven G, Abbasi F, Lamendola C, Saad M, Waters D, et al. Is there a simple way to identify insulin-resistant individuals at increased risk of cardiovascular disease? Am J Cardiol. (2005) 96:399–404. doi: 10.1016/j.amjcard.2005.03.085
22. Cordero A, Laclaustra M, León M, Casasnovas JA, Grima A, Luengo E, et al. Comparison of serum lipid values in subjects with and without the metabolic syndrome. Am J Cardiol. (2008) 102:424–8. doi: 10.1016/j.amjcard.2008.03.079
23. Hsieh SD, Muto T. Metabolic syndrome in Japanese men and women with special reference to the anthropometric criteria for the assessment of obesity: Proposal to use the waist-to-height ratio. Prev Med. (2006) 42:135–9. doi: 10.1016/j.ypmed.2005.08.007
24. Lee CMY, Huxley RR, Wildman RP, Woodward M. Indices of abdominal obesity are better discriminators of cardiovascular risk factors than BMI: a meta-analysis. J Clin Epidemiol. (2008) 61:646–53. doi: 10.1016/j.jclinepi.2007.08.012
25. Hewage N, Wijesekara U, Perera R. Determining the best method for evaluating obesity and the risk for non-communicable diseases in women of childbearing age by measuring the body mass index, waist circumference, waist-to-hip ratio, waist-to-height ratio, A Body Shape Index, and hip index. Nutrition. (2023) 114:112135. doi: 10.1016/j.nut.2023.112135
26. McGuire S, Frayar DC, Ervin RB. Caloric intake from fast food among adults: United States, 2007–2010. NCHS data brief, no. 114, february 2013. Hyattsville, MD: national center for health statistics, 2013. Adv Nutr. (2013) 4(5):578. doi: 10.3945/an.113.003889
27. Yin Y-H, Zhou S-Y, Lu D-F, Chen X-P, Liu B, Lu S, et al. Higher waist circumference is associated with increased likelihood of female infertility: NHANES 2017-2020 results. Front Endocrinol. (2023) 14. doi: 10.3389/fendo.2023.1216413
28. Zhu X, Hong X, Wu J, Zhao F, Wang W, Huang L, et al. The association between circulating lipids and female infertility risk: A univariable and multivariable mendelian randomization analysis. Nutrients. (2023) 15(14):3130. doi: 10.3390/nu15143130
29. Jansen H, Lieb W, Schunkert H. Mendelian randomization for the identification of causal pathways in atherosclerotic vascular disease. Cardiovasc Drugs Ther. (2016) 30:41–9. doi: 10.1007/s10557-016-6640-y
30. Cai WY, Luo X, Ma HL, Shao XG, Wu XK. Association between preconception serum lipid concentrations and treatment outcomes in women with PCOS who underwent ovulation induction. Reprod BioMed Online. (2022) 45:805–14. doi: 10.1016/j.rbmo.2022.04.013
31. Shi WR, Wang HY, Chen S, Guo XF, Li Z, Sun YX. Estimate of prevalent diabetes from cardiometabolic index in general Chinese population: a community-based study. Lipids Health Dis. (2018) 17:236. doi: 10.1186/s12944-018-0886-2
32. Wang H, Chen Y, Sun G, Jia P, Qian H, Sun Y. Validity of cardiometabolic index, lipid accumulation product, and body adiposity index in predicting the risk of hypertension in Chinese population. Postgrad Med. (2018) 130:325–33. doi: 10.1080/00325481.2018.1444901
33. Abolnezhadian F, Hosseini SA, Alipour M, Zakerkish M, Cheraghian B, Ghandil P, et al. Association metabolic obesity phenotypes with cardiometabolic index, atherogenic index of plasma and novel anthropometric indices: A link of FTO-rs9939609 polymorphism. Vasc Health Risk Manage. (2020) 16:249–56. doi: 10.2147/VHRM.S251927
34. Owiredu W, Ofori PN, Turpin CA, Obirikorang C, Acheampong E, Anto EO, et al. Weight management merits attention in women with infertility: a cross-sectional study on the association of anthropometric indices with hormonal imbalance in a Ghanaian population. BMC Res Notes. (2019) 12:545. doi: 10.1186/s13104-019-4593-5
35. van der Ham K, Koster MPH, Velthuis BK, Budde RPJ, Fauser B, Laven JSE, et al. Change in androgenic status and cardiometabolic profile of middle-aged women with polycystic ovary syndrome. J Clin Med. (2023) 12(16):5226. doi: 10.3390/jcm12165226
36. Jacewicz-Swiecka M, Kowalska I. Polycystic ovary syndrome and the risk of cardiometabolic complications in longitudinal studies. Diabetes Metab Res Rev. (2018) 34:e3054. doi: 10.1002/dmrr.3054
37. Oliver-Williams C, Vassard D, Pinborg A, Schmidt L. Polycystic ovary syndrome as a novel risk factor for atrial fibrillation: results from a national Danish registry cohort study. Eur J Prev Cardiol. (2021) 28:e20–e2. doi: 10.1177/2047487320922927
38. Lindley KJ, Bairey Merz CN, Davis MB, Madden T, Park K, Bello NA, et al. Contraception and reproductive planning for women with cardiovascular disease: JACC focus seminar 5/5. J Am Coll Cardiol. (2021) 77:1823–34. doi: 10.1016/j.jacc.2021.02.025
39. de Melo AS, Dos Reis RM, Ferriani RA, Vieira CS. Hormonal contraception in women with polycystic ovary syndrome: choices, challenges, and noncontraceptive benefits. Open Access J Contracept. (2017) 8:13–23. doi: 10.2147/OAJC
40. Lidegaard O, Nielsen LH, Skovlund CW, Skjeldestad FE, Lokkegaard E. Risk of venous thromboembolism from use of oral contraceptives containing different progestogens and oestrogen doses: Danish cohort study, 2001-9. BMJ. (2011) 343:d6423. doi: 10.1136/bmj.d6423
41. Yang F, Mao W, Ge YM, Zhao X, Song J, Lu JC, et al. Hyperlipidemia is negatively associated with pregnancy outcomes in patients following frozen-thawed embryo transfer: A retrospective study. Health Sci Rep. (2024) 7:e1947. doi: 10.1002/hsr2.1947
Keywords: cross-sectional study, NHANES, infertility, cardiometabolic index, IR
Citation: Cheng H, He X and Jin X (2024) The relationship between cardiometabolic index and infertility in American adults: a population-based study. Front. Endocrinol. 15:1424033. doi: 10.3389/fendo.2024.1424033
Received: 27 April 2024; Accepted: 21 August 2024;
Published: 04 September 2024.
Edited by:
Achille Aveta, University of Naples Federico II, ItalyReviewed by:
Savio Domenico Pandolfo, Federico II University Hospital, ItalyCopyright © 2024 Cheng, He and Jin. This is an open-access article distributed under the terms of the Creative Commons Attribution License (CC BY). The use, distribution or reproduction in other forums is permitted, provided the original author(s) and the copyright owner(s) are credited and that the original publication in this journal is cited, in accordance with accepted academic practice. No use, distribution or reproduction is permitted which does not comply with these terms.
*Correspondence: Xiaoke Jin, NjE1NTEwNzUzQHFxLmNvbQ==
Disclaimer: All claims expressed in this article are solely those of the authors and do not necessarily represent those of their affiliated organizations, or those of the publisher, the editors and the reviewers. Any product that may be evaluated in this article or claim that may be made by its manufacturer is not guaranteed or endorsed by the publisher.
Research integrity at Frontiers
Learn more about the work of our research integrity team to safeguard the quality of each article we publish.