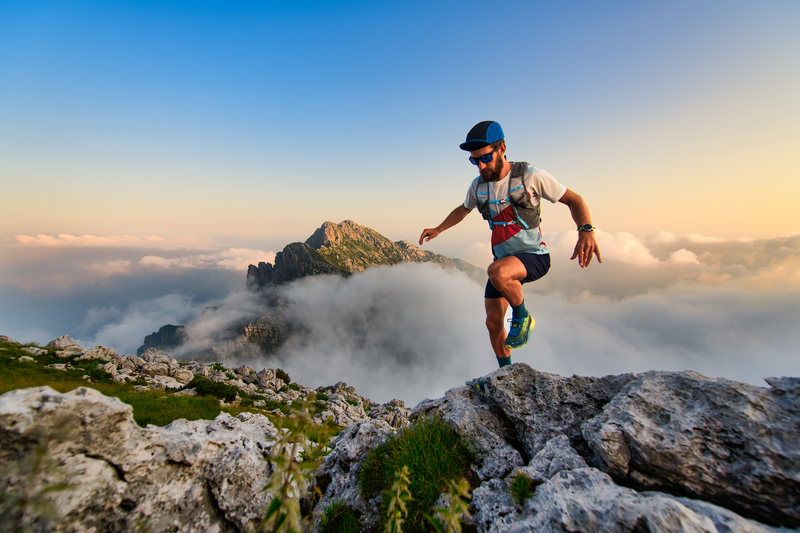
95% of researchers rate our articles as excellent or good
Learn more about the work of our research integrity team to safeguard the quality of each article we publish.
Find out more
CORRECTION article
Front. Endocrinol. , 09 May 2024
Sec. Cancer Endocrinology
Volume 15 - 2024 | https://doi.org/10.3389/fendo.2024.1421538
This article is a correction to:
Machine learning to construct sphingolipid metabolism genes signature to characterize the immune landscape and prognosis of patients with uveal melanoma
A Corrigendum on:
Machine learning to construct sphingolipid metabolism genes signature to characterize the immune landscape and prognosis of patients with uveal melanoma
By Chi H, Peng G, Yang J, Zhang J, Song G, Xie X, Strohmer DF, Lai G, Zhao S, Wang R, Yang F and Tian G (2022). Front. Endocrinol. 13:1056310. doi: 10.3389/fendo.2022.1056310
In the published article, there was an error. There were missing values for the optimal penalty parameter.
A correction has been made to the section Method, subsection Model construction and validation, This sentence previously stated:
“By performing univariate Cox regression analysis, we identified 27 genes associated with survival, followed by Least absolute shrinkage and selection operator (LASSO) regression analysis using ‘glmnet’ in R and using tenfold cross-validation to determine the penalty regularization parameter λ.”
The corrected sentence appears below.
“By performing univariate Cox regression analysis, we identified 27 genes associated with survival, followed by Least absolute shrinkage and selection operator (LASSO) regression analysis using ‘glmnet’ in R, with tenfold cross-validation to determine the optimal penalty parameter lambda.min=9.”
There was another error. The source of the SVM-RFE algorithm was omitted.
A correction has been made to to the section Method, subsection Model construction and validation. This sentence previously stated:
“Using the SVM-RFE algorithm, we obtained 13 valuable variables.”
The corrected sentence appears below.
“We used the SVM-RFE algorithm from the ‘e1071’ R package, with ten-fold cross-validation to obtain 13 valuable variables.”
The authors apologize for these errors and state that this does not change the scientific conclusions of the article in any way. The original article has been updated.
All claims expressed in this article are solely those of the authors and do not necessarily represent those of their affiliated organizations, or those of the publisher, the editors and the reviewers. Any product that may be evaluated in this article, or claim that may be made by its manufacturer, is not guaranteed or endorsed by the publisher.
Keywords: sphingolipid metabolism, UVM, tumor microenvironment, immunotherapy, predictive signature
Citation: Chi H, Peng G, Yang J, Zhang J, Song G, Xie X, Strohmer DF, Lai G, Zhao S, Wang R, Yang F and Tian G (2024) Corrigendum: Machine learning to construct sphingolipid metabolism genes signature to characterize the immune landscape and prognosis of patients with uveal melanoma. Front. Endocrinol. 15:1421538. doi: 10.3389/fendo.2024.1421538
Received: 22 April 2024; Accepted: 30 April 2024;
Published: 09 May 2024.
Edited and Reviewed by:
Antonino Belfiore, University of Catania, ItalyCopyright © 2024 Chi, Peng, Yang, Zhang, Song, Xie, Strohmer, Lai, Zhao, Wang, Yang and Tian. This is an open-access article distributed under the terms of the Creative Commons Attribution License (CC BY). The use, distribution or reproduction in other forums is permitted, provided the original author(s) and the copyright owner(s) are credited and that the original publication in this journal is cited, in accordance with accepted academic practice. No use, distribution or reproduction is permitted which does not comply with these terms.
*Correspondence: Hao Chi, Q2hpaGFvNzUxMUAxNjMuY29t
†These authors have contributed equally to this work
Disclaimer: All claims expressed in this article are solely those of the authors and do not necessarily represent those of their affiliated organizations, or those of the publisher, the editors and the reviewers. Any product that may be evaluated in this article or claim that may be made by its manufacturer is not guaranteed or endorsed by the publisher.
Research integrity at Frontiers
Learn more about the work of our research integrity team to safeguard the quality of each article we publish.