- 1The First Clinical College of Traditional Chinese Medicine, Hunan University of Chinese Medicine, Changsha, China
- 2College of Acupuncture & Moxibustion, Tuina, and Rehabilitation, Hunan University of Chinese Medicine, Changsha, China
- 3Andrology, The First Hospital of Hunan University of Chinese Medicine, Changsha, China
Background: In recent years, the decline in sperm quality in men has become a global trend. There is a close relationship between sperm quality and pregnancy outcome. There is a large body of literature supporting the role of plasma lipidome in male infertility, while the complex mechanisms between them and male infertility are still less clear. Systematic study of the causal relationship between plasma lipidome and MI can help to provide new therapeutic ideas and targets for male infertility.
Methods: In this study, we used a two-sample Mendelian randomization analysis based on Genome-wide association studies pooled data of 179 causal relationships between plasma lipidome and male infertility. We used employed the inverse variance weighted method as the main analysis to assess causality between exposure and outcome, in addition to MR-Egger, Weighted median as complementary methods, and tests for multiplicity and heterogeneity.
Results: We identified 13 plasma lipidome comprising 4 types of plasma lipidome that were associated with male infertility. Among these, 9 plasma lipidome were found to be protective factors, while 4 were risk factors. Notably, the largest proportion of these plasma lipidome were triglyceride types, with Sphingomyelin (d40:1) exhibiting the strongest association with male infertility.
Conclusion: These findings contribute to the current better understanding of male infertility and provide new perspectives on the underlying etiology of male infertility as well as prevention and treatment strategies. In addition, clinical trial validation is needed to assess the potential of these plasma lipidome as biomarkers.
Introduction
Infertility is typically defined as the inability to achieve pregnancy naturally after one year of regular, unprotected sexual intercourse (1).According to the World Health Organization, approximately 50% of infertility cases in couples can be attributed to male factors (2–4). Studies have demonstrated a global decline in male sperm concentration ranging from 1.4% to 1.6% annually, varying by geographical region (5). Exploring the causes of male infertility (MI) and seeking effective treatments remain ongoing scientific pursuits. Single-cell RNA sequencing (sc-RNA-seq) has revealed the molecular complexities of testicular physiology and MI-related diseases. Recent studies have identified key transcriptional profiles of germ cells, linking developmental processes to disease manifestations in the testicular microenvironment (6). These insights provide valuable references for diagnosing and treating unexplained and idiopathic MI. MI has emerged as a significant factor impacting global population dynamics (7), profoundly affecting patients’ psychological well-being, quality of life, family dynamics, and imposing substantial economic and emotional burdens (8). The etiology and pathogenesis of MI remain incompletely understood, with semen abnormalities being a prominent risk factor (1). Lifestyle factors such as smoking (9), alcohol consumption (10), sleep deprivation, obesity, sedentary habits, frequent sexual activity, and adverse psychosocial conditions can all influence semen quality (11, 12). Additionally, obesity (13), sleep deprivation, and lack of physical activity can impact plasma lipidome (PL) levels. Indeed, the lipidome plays a significant role in MI. Previous studies have shown that adipokines, such as adiponectin and chemerin, are closely associated with diseases of the male reproductive system (14). For example, scientific research has demonstrated that adiponectin can mitigate testicular damage in diabetic mice (15). As a result, the lipidome has emerged as a critical area of research. Building on this, we hypothesize whether PL are closely linked to MI as well. In recent years, the study of PL has garnered significant attention. PL serve as crucial energy sources, form the structural and functional basis of biological membranes, and act as essential signaling molecules without which biological processes would not be possible. Modern lipidomics techniques have revolutionized our understanding of the diversity and complexity of circulating lipids. PL encompass a range of lipid classes including cholesteryl ester, ceramide, diacylglycerol, lysophosphatidylcholine, phosphatidylcholine, phosphatidylcholine-ether, phosphatidylethanolamine, phosphatidylethanolamine-ether, sphingomyelin, and triglyceride, among others. The utilization of histological techniques in exploring PL presents a robust platform for comprehensive investigations into the intricate interplay among nutrition, metabolism, and genotypic variability. PL have emerged as significant players strongly linked to human aging (16), cardiometabolic disorders (17), and hematologic disorders (18), offering potential avenues for diagnosis and treatment. However, limited research has delved into their association with MI. Current research has focused on common lipoproteins such as triglycerides (19), high-density lipoprotein, low-density lipoprotein (20), but there is still a lack of comprehensive investigation into the relationship between the complete PL system and MI, warranting further exploration. While the causes of MI have been largely identified by scientists, approximately 40% of patients still have unclear reasons for their condition. Therefore, it is crucial to continue exploring the associated risk factors for its onset (21).
Mendelian randomization (MR) is an emerging epidemiological research approach utilizing genetic variation, particularly single nucleotide polymorphisms (SNPs), as instrumental variables (IVs) for causal inference (22). Randomized controlled trials (RCTs) are the gold standard to establish causal relationships (23). Proper randomization ensures that study groups are comparable in all characteristics, except for the exposure of interest, which often is a therapeutic intervention. However, RCTs cannot always be conducted, because they can be excessively costly, impractical, or even unethical. Mendelian randomization refers to an analytic approach to assess the causality of an observed association between a modifiable exposure or risk factor and a clinically relevant outcome. It presents a valuable tool, especially when randomized controlled trials to examine causality are not feasible and observational studies provide biased associations because of confounding or reverse causality. These issues are addressed by using genetic variants as instrumental variables for the tested exposure: the alleles of this exposure-associated genetic variant are randomly allocated and not subject to reverse causation. This, together with the wide availability of published genetic associations to screen for suitable genetic instrumental variables make Mendelian randomization a time- and cost-efficient approach and contribute to its increasing popularity for assessing and screening for potentially causal associations. An observed association between the genetic instrumental variable and the outcome supports the hypothesis that the exposure in question is causally related to the outcome (24). This method offers advantages in overcoming the limitations of unknown confounders and reverse causality commonly encountered in traditional observational epidemiological studies (25). In our study, we employed a two-sample MR design to investigate the causal relationship between PL and MI. Our aim was to identify potential risk factors and explore possible treatment strategies for MI.
Methods and materials
Study design
In this study, PL was selected as the exposure factor, and SNPs associated with PL were selected as IVs. MR of PL and MI was performed using two-sample MR analysis, and heterogeneity and horizontal polytropy were assessed using Cochran’s Q with MR Egger’s method test, and finally sensitivity analyses were performed to verify the stability of the results. We assessed the causal association between 179 PL and MI based on two-sample MR analysis. MR uses genetic variants to represent risk factors; therefore, IVs in causal inference must satisfy three key assumptions MR analysis needs to satisfy 3 conditional assumptions (26): (1) the association assumption: there is a direct correlation between the genetic variants and the exposure factors; (2) Independence assumption: genetic variation is independent of possible confounders between exposure and outcome; (3) Exclusivity assumption: genetic variation can only have an effect on outcome through the pathway of exposure (Figure 1).
Data sources
SNPs significantly associated with PL were selected as IVs, and Genome-wide association studies (GWAS) summary statistics for PL were publicly available from the GWAS catalog (27), which includes 495 genetic associations (GCST90277238 to GCST90277416). The data for MI were downloaded from the IEU Open GWAS database (https://gwas.mrcieu.ac.uk/) under the number finn-b-N14_MALEINFERT dataset, with 8,305 cases in the MI group and 72,799 cases in the normal control group, including 16,377,329 SNPs, and this study population were all European populations.(Year: 2021; Category: Binary; Population: European; ncase: 680; Build: HG19/GRCh37).
Selection of instrumental variables
We used that exposed SNPs should reach genome-wide significant levels (P < 5 × 10 - 8) as a screening condition (28), and in the absence of significant genome-wide SNPs as IV, SNPs below the genome-wide significance threshold (P < 5 × 10 - 6) were used as a new screening condition (24). to discover more potential causal associations. Meanwhile, to mitigate the bias caused by linkage disequilibrium, r2 = 0.001 and kb = 10000 were set as the thresholds for removing linkage disequilibrium (29); only the SNPs with the strongest impact on the outcome were selected as tools. Statistical differences were considered to exist if P < 0. 05, and the F value was set to >10 (29), which indicated the absence of weak instrumental variable bias, calculated as F = R2(N-2)/(1-R2), where R2 is the percentage of variance explained by SNPs in the exposure database and N is the sample size of the exposed GWAS. MI as an outcome was a dichotomous variable and was expressed as odds ratio (OR) and 95% confidence interval (CI). R4.2.3 and R studio software and the R package “Two Sample MR” were used for the above analyses, with a standardized test of α = 0.05. β is the allele effect value and SE is the standard error (SE); finally, the palindromic SNPs were removed by palindromic sequence detection to prevent alleles from influencing the results. Initial studies were obtained with informed consent from all participants, and these data are publicly available on the website. We then used the PhenoScanner V2 website (http://www.phenoscanner.medschl.cam.ac.uk) to exclude SNPs that are potentially confounding factors and related to the outcome (male fertility description) to eliminate the possibility of genetic pleiotropy. After a series of rigorous screenings, the remaining SNPS were considered eligible for IV.
Mendelian randomization analysis
In this study, several MR methods were used to assess and validate the causal relationship between LP and MI risk, including IVW (30), MR-Egger regression (31), and weighted median (31). IVW is essentially a meta-analysis method that analyzes PL and the effect of MI through weighted linear regression to obtain an overall estimate of the effect of PL and MI. IVW can be used for causal assessment when there is no horizontal pleiotropy between SNPs. If multinomiality exists in the IV, the MR-Egger method shows horizontal multinomiality in the IV by using an intercept term. If the intercept term is equal to 0, the results of MR-Egger regression and IVW are the same. For up to 50% of invalid IVs, the weighted median model yields consistent causal estimation of the relationship.
According to MR, genetic tools can only affect outcomes by exposing people to them, and genetic variants may have pleiotropic effects. In the main analysis, we calculated Wald ratio estimates for each genetic variant and summarized the estimates using an IVW approach. The IVW method with multiplicative random effects provides a parsimonious estimate and takes into account potential heterogeneity among Wald ratio estimates for SNPs. Estimates may be inaccurate if the SNPs used as instruments have horizontal pleiotropic effects that cause results to be influenced by pathways other than exposure. Potential heterogeneity was estimated using the Cochran’s Q test, and fixed-effects IVW models were applied when P > 0.05, when no heterogeneity between SNPs was considered to exist, and random-effects IVW models were applied if P < 0.05, when heterogeneity between SNPs was considered to exist. The sensitivity of the effect estimate after removing SNPs one by one was analyzed by Leave-one-out analysis to assess whether there was an effect of excluding individual SNP observations on the final results. Potential pleiotropy was assessed by intercepts tested by MR-Egger regression, and when P > 0.05, it indicated the absence of pleiotropy.
Results
Result of MR
Utilizing instrumental variable screening principles, a total of 13 PL were identified as causally associated with MI by at least one MR method (p < 0.05): four were identified as risk factors, while nine were deemed protective factors (Figure 2).
IVW analysis showed (Table 1) that Phosphatidylethanolamine (18:0_0:0) levels [OR = 0.697, 95% CI (0.507 - 0.958), p = 0.026], Phosphatidylcholine (18:0_18:3) levels [OR = 0.689, 95% CI (0.478 - 0.992), p = 0.045], Sphingomyelin (d40:1) levels [OR = 0.762, 95% CI (0.625 - 0.930), p = 0.0076], Triacylglycerol (46:1) levels [OR = 0.687, 95% CI (0.496 - 0.951), p = 0.024], Triacylglycerol (51:2) levels [OR = 0.660, 95% CI (0.467 - 0.933), p = 0.019], Triacylglycerol (52:2) levels [OR = 0.756, 95% CI (0.573 - 0.999), p = 0.049], Triacylglycerol (53:3) levels [OR = 0.763, 95% CI (0.592 - 0.984), p = 0.037], Triacylglycerol (56:3) levels [OR = 0.765, 95% CI (0.596 - 0.982), p = 0.035], Triacylglycerol (56:4) levels [OR = 0.647, 95% CI (0.460 - 0.910), p = 0.012] were protective factors for MI. Phosphatidylcholine (16:0_16:0) levels [OR = 1.390, 95% CI (1.068 - 1.809), p = 0.014], Phosphatidylcholine (O-16:0_20:4) levels [OR = 1.245, 95% CI (1.008 - 1.538), p = 0.042], Phosphatidylcholine (O-18:0_20:4) levels [OR = 1.357, 95% CI (1.048 - 1.756), p = 0.021], Phosphatidylethanolamine (O-18:1_20:4) levels [OR = 1.583, 95% CI (1.205 - 2.078), p = 0.001] risk of MI was a risk factor.
For Sphingomyelin (d40:1) levels, the p-value of all three methods (IVW, weighted median, and MR-Egger) was less than 0.05 (Figure 3), and we therefore concluded that Sphingomyelin (d40:1) levels are the PL most closely associated with MI.
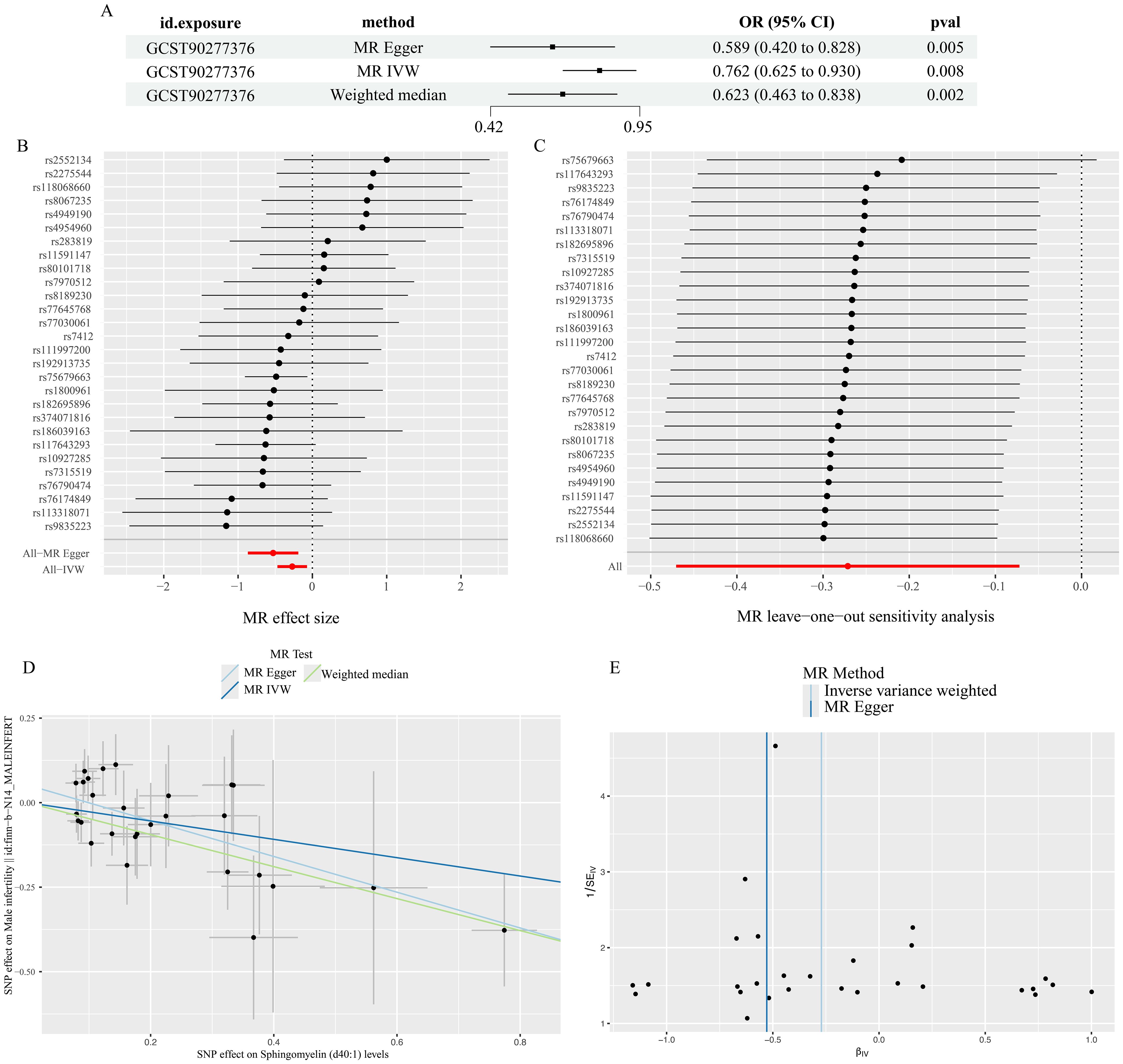
Figure 3. Results of Sphingomyelin (d40:1) levels analysis. (A) results of three methods (B) forest plot (C)leave-one-out plot (D)scatter plot (E)funnel plot.
Heterogeneity test, sensitivity analysis, multiple validity analysis
The results of Cochran’s Q and MR-Egger regression methods showed that there was no significant heterogeneity or pleiotropy in this study. The results of leave-one-out method showed that the results did not change after removing SNPs one by one. These analyses proved to some extent the robustness of the results of this study (Table 2).
Discussion
With the accelerated pace of life and increased work pressure, MI has become a social problem of general concern (32). MI is a global health problem that requires attention from the medical profession. By pooling data from 16 studies (33), researchers found that Central and Eastern Europe had the highest prevalence of MI (8-12%). MI leads to serious adverse health, psychological, social, and economic consequences related to the disease. Results have shown that infertile men have a higher risk of cancer (e.g., testicular cancer, prostate cancer) and other adverse health outcomes (e.g., heart disease, diabetes, and autoimmune disorders) than the normally fertile population (34–36), so it is important to characterize the etiology of MI and treat the symptoms.
MR analysis is a scientific and effective method to identify the causal relationship between risk factors and diseases.MR is an effective scientific method for identifying causal relationships between risk factors and diseases. It can be used to evaluate the causality between modifiable exposures or risk factors and clinically relevant outcomes. MR uses genetic variations as instrumental variables, which are randomly assigned. Compared to observational studies, this method reduces the influence of confounding factors. Moreover, since genetic variations are determined at birth and are not influenced by outcomes, it can avoid the interference of reverse causation. The wide availability of published genetic associations for screening suitable genetic instrumental variables makes Mendelian randomization a time - and cost - efficient approach, contributing to its increasing popularity for assessing and screening potentially causal associations. Scientists have confirmed numerous mechanisms affecting male infertility, yet many unknown areas still warrant exploration by researchers. In the context of declining global sperm quality, it is crucial to employ scientific research and experimental methods to explore factors influencing MI and develop new treatments. MR is a robust method for identifying causal relationships, and many researchers are actively using this approach to investigate various factors affecting male infertility (37, 38). Although MR cannot replace RCTs, it can provide supplementary evidence or offer new design insights for RCTs, thereby enhancing clinical diagnosis and treatment strategies. Thus, utilizing MR to uncover causal relationships related to MI is highly important. In this study, we employed MR to comprehensively assess the impact of 495 PL on MI. Our MR results indicate that PL may significantly influence MI, suggesting that lipoproteins play a crucial role in its pathogenesis.
PL refer to the overall composition and characterization of all lipid molecules in plasma. Lipids are a class of biomolecules that play important roles in maintaining cell membrane structure, energy storage, and signaling. The analysis of PL can help us to understand the lipid metabolism of an individual or a group of individuals in healthy and diseased states, which is important for the study of metabolic diseases, reproductive health and so on. There is a large body of literature supporting that PL play an important role in MI (39), and PL has emerged as a comprehensive approach to identify specific biomarkers associated with reproductive disorders (40, 41).Researchers have noted that low sperm counts in men are associated with abnormal PL levels and have suggested that fertility assessment could be improved by increasing lipid screening (42). And abnormalities in PL may directly affect sperm formation and function (43).
Through our study we found a total of 13 PL associated with MI in the European population, which were Phosphatidylethanolamine(18:0_0:0) levels, Phosphatidylcholine (18:0_18:3) level, Phosphatidylcholine (18:0_18:3) levels, Phosphatidylcholine (O-16:0_20:4) levels, Phosphatidylcholine (O-18:0_20:4) levels, Phosphatidylethanolamine (O-18:1_20:4) levels, Sphingomyelin(d40:1)levels, Triacylglycerol (46:1) levels, Triacylglycerol (51:2) levels, Triacylglycerol (52:2) levels, Triacylglycerol (53:3) levels, Triacylglycerol (56:3) levels, Triacylglycerol (56:4) levels。The most diverse of these were triglycerides, and all six were protective factors. Triglycerides are important determinants of sperm composition. It has been suggested that special attention should be paid to triglycerides in classical lipid screening because it may be a sensitive indicator of male reproductive dysfunction (19), which has a consistent outlook with our findings. Many studies have found that sperm immaturity may be and male reproductive defects with dyslipidemia, and a relevant meta-analysis showed that triglycerides do have a significant correlation with semen parameters (44).Correnti et al. conducted a multilevel analysis of PL in 50 subjects (31 infertile patients and 19 normal fertile men) (45). Triglyceride (14:0_16:0_18:0) showed a positive correlation with MI, while negative correlations were observed for phosphatidylcholine (PC O-16:2_18:1)-CH3, phosphatidylethanolamine (PE O-16:1_20:3), etc., which are consistent with our findings. However, some researchers have also suggested (46) a significant negative correlation between riglycerides and semen parameters as well as serum total testosterone. For instance, Alterman et al. suggested (47) that lipid parameters such as triglycerides are negatively correlated with sperm morphology. Conversely, some scientists argue that lipid levels do not significantly correlate with MI. This discrepancy conflicts with our results, highlighting the dual nature of triglycerides. Lipid testing is commonly accessible in hospitals. Historically, elevated triglycerides have been a primary concern, and we advocate for maintaining triglyceride levels within reasonable limits, neither too high nor too low. Timely intervention is recommended in cases where triglyceride levels are excessively low.
In addition, we also discovered that among the 13 relevant PL, Sphingomyelin (d40:1) levels exhibited the strongest association with MI. Experimental evidence has demonstrated that men with oligospermia exhibit a significant reduction in sphingomyelin levels compared to normal men, indicating its protective role (48), which is consistent with our findings. Sphingomyelin is the most abundant phospholipid in spermatozoa and plays a crucial role in sperm maturation, being an essential component of the epididymis (49). In various types of cells, different stimuli can activate sphingomyelinase to hydrolyze sphingolipids. Sphingomyelin plays a crucial role in the hydrolysis of sperm phospholipids, which is closely linked to sperm capacitation and fertilization processes. Additionally, it can inhibit gonadotropin-induced testosterone synthesis in mesenchymal cells and reduce gonadotropin binding to receptors. This not only decreases androgen synthesis but also leads to excessive apoptosis of spermatogonia and spermatocytes (50). Wittmann et al. (51) conducted an experimental study and found that sphingomyelinase 1 is indispensable for fertility in mice. Similarly, Datar (52) discovered that metabolites of sphingomyelin, triglycerides, etc., are closely associated with testicular growth in mice.
Sphingolipids, a family of lipids with a common sphingomyelin backbone, are integral to various physiological and pathological processes in cells. They act as important regulators of cellular processes such as cell differentiation and apoptosis, and are universally expressed in all mammalian cells. During epididymal transit, there occurs an exchange of sphingolipids between spermatozoa and the surrounding fluid. Sphingolipid metabolites in the reproductive system have garnered significant attention in recent years. Studies have indicated (53) a potential association between sphingolipids and impaired gonadal function as well as infertility. In our study, sphingomyelin emerged as a protective factor for MI. This leads us to consider focusing on the measurement and preservation of sphingomyelin levels in future clinical treatments, particularly for patients with unexplained MI. This approach offers new perspectives for clinical management strategies.
Generally, men with primary infertility tend to have poorer health compared to fertile men, necessitating more reliable diagnostic tools and robust personalized interventions. Through our study, we identified 13 PL as major contributors to MI. We advocate for a more comprehensive assessment of PL in men seeking fertility, incorporating it as a routine part of clinical evaluations for infertility, beyond the standard PL tests. Special attention should be given to the screening of sphingomyelins and triglycerides, thereby enhancing the diagnostic tools for MI. Diet (40) and obesity (17) are recognized as risk factors for MI. Therefore, it is crucial to conduct thorough PL screening, particularly in men with higher BMI or obesity. Even in young patients without overt lipid abnormalities (such as severe overweight, obesity, and metabolic syndrome), PL levels should be meticulously monitored. Early intervention for those with abnormal PL levels can mitigate the adverse effects on fertility. In the realm of pharmacological research for MI, regulating PL levels, especially sphingomyelins and triglycerides, could be a promising approach. Developing new targeted drugs that modulate various PL offers potential new treatment options for MI. Additionally, clinical education should emphasize the impact of diet and obesity on fertility. From what we know, this study stands as the inaugural in-depth investigation into the causal connection between all categories of PL and MI. Our study design was meticulously crafted to minimize confounding factors and potential sources of observational bias. All instrumental variables used were sourced from publicly available GWAS, ensuring a rich dataset and statistical robustness in assessing the plasma lipoproteins relevant to male fertility.
In the future, we deem it necessary to continue conducting RCTs to ascertain the precise role of PL in MI. Our study findings provide a robust theoretical foundation for similar RCTs. Furthermore, our research has identified PL as both protective factors and risk factors for MI, guiding early interventions, treatments, and prognostic assessments. Future large-scale studies and RCTs on PL will further elucidate the causal relationships between these PL and MI. Promoting PL screening globally can provide new strategies and methods to improve male fertility.
Limitation
Our study has several limitations. Firstly, the results of the analysis are specific to European populations and may not accurately reflect populations in other regions. Secondly, there is potential bias in the study due to the relatively small number of MI cases in the GWAS data. Lastly, the GWAS for MI was not categorized, which limits our ability to provide detailed insights into the association of PL with specific types of MI. Future studies and randomized controlled trials are necessary to elucidate the causal relationship between PL and MI more effectively.
Conclusion
MR analyses using large datasets analyzed by GWAS have revealed a causal relationship between PL and MI. However, a larger GWAS database is necessary to further investigate the mechanisms underlying this association. Sphingomyelin and triglycerides are closely linked to the development of MI, and effective control of these lipid levels can potentially reduce the prevalence of MI, providing new avenues for treatment and prevention.
Data availability statement
The original contributions presented in the study are included in the article/Supplementary Material, further inquiries can be directed to the corresponding author.
Author contributions
YY: Writing – review & editing, Writing – original draft, Visualization, Validation, Software, Resources, Methodology, Investigation, Formal Analysis, Data curation, Conceptualization. XX: Writing – original draft, Resources, Methodology, Investigation. JZ: Writing – review & editing, Supervision. ZQ: Writing – original draft, Visualization, Software. BW: Writing – original draft, Visualization. GO: Writing – original draft, Visualization, Validation. QZ: Writing – review & editing, Supervision, Project administration, Funding acquisition.
Funding
The author(s) declare financial support was received for the research, authorship, and/or publication of this article. We would like to acknowledge funding from Hunan Provincial Department of Education Scientific Research (Outstanding Youth) Project (22B0368), “Hunan Provincial Traditional Chinese Medicine Clinical Medicine Research Center for Sexual and Reproductive Health” (Xiangkeji 2020SK4014),Hunan Provincial Key Specialty in Traditional Chinese Medicine (Traditional Chinese Medicine Andrology) (Letter of Hunan Traditional Chinese Medicine [2023] No.4), Hunan University of Chinese Medicine Graduate Innovation Project “Yifang” (2024YF05) and National Key Specialty in Traditional Chinese Medicine (Surgery)(National Administration of Traditional Chinese Medicine Document [2024] No. 90).
Acknowledgments
We are grateful for the suggestions of the editor and reviewers. We also thank for the open data set OpenGWAS.
Conflict of interest
The authors declare that the research was conducted in the absence of any commercial or financial relationships that could be construed as a potential conflict of interest.
Publisher’s note
All claims expressed in this article are solely those of the authors and do not necessarily represent those of their affiliated organizations, or those of the publisher, the editors and the reviewers. Any product that may be evaluated in this article, or claim that may be made by its manufacturer, is not guaranteed or endorsed by the publisher.
Supplementary material
The Supplementary Material for this article can be found online at: https://www.frontiersin.org/articles/10.3389/fendo.2024.1412684/full#supplementary-material
Supplementary Table 1 | Characteristics of SNPs used as instrumental variables for Sphingomyelin(d40:1) levels.
Abbreviations
PL, plasma lipidome; MI, male infertility; MR, Mendelian randomization; GWAS, Genome-wide association studies; RCT, Randomized controlled trial; IVW, Inverse variance weighted; SNP, single nucleotide polymorphism; IV, instrumental variable; OR, odds ratio; CI, confidence interval; SE, standard error.
References
1. World Health Organization (WHO). International classification of diseases, 11th revision (ICD-11). Geneva: WHO (2018).
2. Diaz P, Dullea A, Chu KY, Zizzo J, Loloi J, Reddy R, et al. Future of male infertility evaluation and treatment: brief review of emerging technology. Urology. (2022) 169:9–16. doi: 10.1016/j.urology.2022.06.036
3. Minhas S, Bettocchi C, Boeri L, Capogrosso P, Carvalho J, Cilesiz NC, et al. European association of urology guidelines on male sexual and reproductive health: 2021 update on male infertility. Eur Urol. (2021) 80:603–20. doi: 10.1016/j.eururo.2021.08.014
4. Vander Borght M, Wyns C. Fertility and infertility: Definition and epidemiology. Clin Biochem. (2018) 62:2–10. doi: 10.1016/j.clinbiochem.2018.03.012
5. Skakkebæk NE, Lindahl-Jacobsen R, Levine H, Andersson AM, Jørgensen N, Main KM, et al. Environmental factors in declining human fertility. Nat Rev Endocrinol. (2022) 18:139–57. doi: 10.1038/s41574-021-00598-8
6. Tirumalasetty MB, Bhattacharya I, Mohiuddin MS, Baki VB, Choubey M. Understanding testicular single cell transcriptional atlas: from developmental complications to male infertility. Front Endocrinol. (2024) 15:1394812. doi: 10.3389/fendo.2024.1394812
7. Agarwal A, Mulgund A, Hamada A, Chyatte MR. A unique view on male infertility around the globe. Reprod Biol Endocrinol. (2015) 13:37. doi: 10.1186/s12958-015-0032-1
8. Fernández-Zapata WF, Cardona-Maya W. Male infertility - what about mental health? Infertilidade masculina – E a saúde mental? Rev Bras Ginecol Obstet. (2023) 45:e620–1. doi: 10.1055/s-0043-1772471
9. Kahn BE, Brannigan RE. Obesity and male infertility. Curr Opin Urol. (2017) 27:441–5. doi: 10.1097/MOU.0000000000000417
10. Finelli R, Mottola F, Agarwal A. Impact of alcohol consumption on male fertility potential: A narrative review. Int J Environ Res Public Health. (2021) 19:328. doi: 10.3390/ijerph19010328
11. Agarwal A, Baskaran S, Parekh N, Cho CL, Henkel R, Vij S, et al. Male infertility. Lancet. (2021) 397:319–33. doi: 10.1016/S0140-6736(20)32667-2
12. Okonofua FE, Ntoimo LFC, Omonkhua A, Ayodeji O, Olafusi C, Unuabonah E, et al. Causes and risk factors for male infertility: A scoping review of published studies. Int J Gen Med. (2022) 15:5985–97. doi: 10.2147/IJGM.S363959
13. Duft RG, Bonfante ILP, Palma-Duran SA, Chacon-Mikahil MPT, Griffin JL, Cavaglieri CR. Moderate-intensity combined training induces lipidomic changes in individuals with obesity and type 2 diabetes. J Clin Endocrinol Metab. (2024) 31:dgae328. doi: 10.1210/clinem/dgae177
14. Singh A, Choubey M, Bora P, Krishna A. Adiponectin and chemerin: contrary adipokines in regulating reproduction and metabolic disorders. Reprod Sci. (2018) 25:1462–73. doi: 10.1177/1933719118770547
15. Choubey M, Ranjan A, Bora PS, Krishna A. Protective role of adiponectin against testicular impairment in high-fat diet/streptozotocin-induced type 2 diabetic mice. Biochimie. (2020) 168:41–52. doi: 10.1016/j.biochi.2019.10.014
16. Hornburg D, Wu S, Moqri M, Zhou X, Contrepois K, Bararpour N, et al. Dynamic lipidome alterations associated with human health, disease and ageing. Nat Metab. (2023) 5:1578–94. doi: 10.1038/s42255-023-00880-1
17. Eichelmann F, Sellem L, Wittenbecher C, Jäger S, Kuxhaus O, Prada M, et al. Deep lipidomics in human plasma: cardiometabolic disease risk and effect of dietary fat modulation. Circulation. (2022) 146:21–35. doi: 10.1161/CIRCULATIONAHA.121.056805
18. Ruan J, Yang C, Du Y, Chen M, Han B. Plasma lipidome acts as diagnostic marker and predictor for cyclosporin response in patients with aplastic anemia. Clin Exp Med. (2023) 23:767–76. doi: 10.1007/s10238-022-00826-z
19. Andrade G, Iori I, Hsieh MK, Milani G, Zandoná PCE, Teixeira TA, et al. Serum lipid profile levels and semen quality: new insights and clinical perspectives for male infertility and men’s health. Int Urol Nephrol. (2023) 55:2397–404. doi: 10.1007/s11255-023-03688-w
20. Cardoso AM, Alves MG, Mathur PP, Oliveira PF, Cavaco JE, Rato L. Obesogens and male fertility. Obes Rev. (2017) 18:109–25. doi: 10.1111/obr.12469
21. Krausz C, Riera-Escamilla A. Genetics of male infertility. Nat Rev Urol. (2018) 15:369–84. doi: 10.1038/s41585-018-0003-3
22. Birney E. Mendelian randomization. Cold Spring Harb Perspect Med. (2022) 12:a041302. doi: 10.1101/cshperspect.a041302
23. Evans DM, Davey Smith G. Mendelian randomization: New applications in the coming age of hypothesis-free causality. Annu Rev Genomics Hum Genet. (2015) 16:327–50. doi: 10.1146/annurev-genom-090314-050016
24. Sekula P, Del Greco MF, Pattaro C, Köttgen A. Mendelian randomization as an approach to assess causality using observational data. J Am Soc Nephrol. (2016) 27:3253–65. doi: 10.1681/ASN.2016010098
25. Davies NM, Holmes MV, Davey Smith G. Reading Mendelian randomisation studies: a guide, glossary, and checklist for clinicians. BMJ. (2018) 362:k601. doi: 10.1136/bmj.k601
26. Burgess S, Scott RA, Timpson NJ, Davey Smith G, Thompson SG, EPIC- InterAct Consortium. Using published data in Mendelian randomization: a blueprint for efficient identification of causal risk factors. Eur J Epidemiol. (2015) 30:543–52. doi: 10.1007/s10654-015-0011-z
27. Ottensmann L, Tabassum R, Ruotsalainen SE, Gerl MJ, Klose C, Widén E, et al. Genome-wide association analysis of plasma lipidome identifies 495 genetic associations. Nat Commun. (2023) 14:6934. doi: 10.1038/s41467-023-42532-8
28. Larsson SC, Butterworth AS, Burgess S. Mendelian randomization for cardiovascular diseases: principles and applications. Eur Heart J. (2023) 44:4913–24. doi: 10.1093/eurheartj/ehad736
29. Zhao L, Wu R, Wu Z, Liu X, Li J, Zhang L, et al. Genetically predicted 486 blood metabolites concerning risk of systemic lupus erythematosus: a Mendelian randomization study. Sci Rep. (2023) 13:22543. doi: 10.1038/s41598-023-49233-8
30. Lee CH, Cook S, Lee JS, Han B. Comparison of two meta-analysis methods: inverse-variance-weighted average and weighted sum of Z-scores. Genomics Inform. (2016) 14:173–80. doi: 10.5808/GI.2016.14.4.173
31. Bowden J, Davey Smith G, Burgess S. Mendelian randomization with invalid instruments: effect estimation and bias detection through Egger regression. Int J Epidemiol. (2015) 44:512–25. doi: 10.1093/ije/dyv080
32. World Health Organization. Infertility prevalence estimates, 1990–2021 . Geneva: World Health Organization (2023).
33. Eisenberg ML, Esteves SC, Lamb DJ, Hotaling JM, Giwercman A, Hwang K, et al. Male infertility. Nat Rev Dis Primers. (2023) 9:49. doi: 10.1038/s41572-023-00459-w
34. Chen T, Belladelli F, Del Giudice F, Eisenberg ML. Male fertility as a marker for health. Reprod BioMed Online. (2022) 44:131–44. doi: 10.1016/j.rbmo.2021.09.023
35. Faja F, Esteves S, Pallotti F, Cicolani G, Di Chiano S, Delli Paoli E, et al. Environmental disruptors and testicular cancer. Endocrine. (2022) 78:429–35. doi: 10.1007/s12020-022-03171-z
36. Del Giudice F, Kasman AM, Chen T, De Berardinis E, Busetto GM, Sciarra A, et al. The association between mortality and male infertility: systematic review and meta-analysis. Urology. (2021) 154:148–57. doi: 10.1016/j.urology.2021.02.041
37. Fu ZD, Wang Y, Yan HL. Male infertility risk and gut microbiota: a Mendelian randomization study. Front Microbiol. (2023) 14:1228693. doi: 10.3389/fmicb.2023.1228693
38. Zhu XB, Niu ZH, Fan WM, Sheng CS, Chen Q. Type 2 diabetes mellitus and the risk of male infertility: a Mendelian randomization study. Front Endocrinol (Lausanne). (2023) 14:1279058. doi: 10.3389/fendo.2023.1279058
39. Cardozo E, Pavone ME, Hirshfeld-Cytron JE. Metabolic syndrome and oocyte quality. Trends Endocrinol Metab. (2011) 22:103–9. doi: 10.1016/j.tem.2010.12.002
40. Panner Selvam MK, Finelli R, Agarwal A, Henkel R. Proteomics and metabolomics - Current and future perspectives in clinical andrology. Andrologia. (2021) 53:e13711. doi: 10.1111/and.13711
41. Aderemi AV, Ayeleso AO, Oyedapo OO, Mukwevho E. Metabolomics: A scoping review of its role as a tool for disease biomarker discovery in selected non-communicable diseases. Metabolites. (2021) 11:418. doi: 10.3390/metabo11070418
42. Núñez Calonge R, Guijarro JA, Andrés C, Cortés S, Saladino M, Caballero P, et al. Relationships between lipids levels in blood plasma, follicular fluid and seminal plasma with ovarian response and sperm concentration regardless of age and body mass index. Rev Int Androl. (2022) 20:178–88. doi: 10.1016/j.androl.2021.02.004
43. Ferramosca A, Zara V. Diet and male fertility: the impact of nutrients and antioxidants on sperm energetic metabolism. Int J Mol Sci. (2022) 23:2542. doi: 10.3390/ijms23052542
44. Pakpahan C, Rezano A, Margiana R, Amanda B, Agustinus A, Darmadi D. The association between lipid serum and semen parameters: a systematic review. Reprod Sci. (2023) 30:761–71. doi: 10.1007/s43032-022-01040-8
45. Correnti S, Preianò M, Fregola A, Gamboni F, Stephenson D, Savino R, et al. Seminal plasma untargeted metabolomic and lipidomic profiling for the identification of a novel panel of biomarkers and therapeutic targets related to male infertility. Front Pharmacol. (2023) 14:1275832. doi: 10.3389/fphar.2023.1275832
46. Turan E, Öztekin Ü. Relationship between visceral adiposity index and male infertility. Andrologia. (2020) 52:e13548. doi: 10.1111/and.13548
47. Schisterman EF, Mumford SL, Chen Z, Browne RW, Boyd Barr D, Kim S, et al. Lipid concentrations and semen quality: the LIFE study. Andrology. (2014) 2:408–15. doi: 10.1111/j.2047-2927.2014.00198.x
48. Boguenet M, Bocca C, Bouet PE, Serri O, Chupin S, Tessier L, et al. Metabolomic signature of the seminal plasma in men with severe oligoasthenospermia. Andrology. (2020) 8:1859–66. doi: 10.1111/andr.12882
49. Sandhoff R. Very long chain sphingolipids: tissue expression, function and synthesis. FEBS Lett. (2010) 584:1907–13. doi: 10.1016/j.febslet.2009.12.032
50. Wang D, Tang Y, Wang Z. Role of sphingolipid metabolites in the homeostasis of steroid hormones and the maintenance of testicular functions. Front Endocrinol (Lausanne). (2023) 14:1170023. doi: 10.3389/fendo.2023.1170023
51. Wittmann A, Grimm MO, Scherthan H, Horsch M, Beckers J, Fuchs H, et al. Sphingomyelin synthase 1 is essential for male fertility in mice. PloS One. (2016) 11:e0164298. doi: 10.1371/journal.pone.0164298
52. Datar J, Regassa A, Kim WK, Taylor CG, Zahradka P, Suh M. Lipid metabolism is closely associated with normal testicular growth based on global transcriptome profiles in normal and underdeveloped testis of obese zucker (fa/fa) rats. Lipids. (2017) 52:951–60. doi: 10.1007/s11745-017-4298-2
Keywords: male infertility, Mendelian randomization, plasma lipidome, GWAS, sperm quality
Citation: Yang Y, Xue X, Zhou J, Qiu Z, Wang B, Ou G and Zhou Q (2024) Male infertility risk and plasma lipidome: a Mendelian randomization study. Front. Endocrinol. 15:1412684. doi: 10.3389/fendo.2024.1412684
Received: 05 April 2024; Accepted: 25 July 2024;
Published: 14 August 2024.
Edited by:
Mayank Choubey, NYU Grossman Long Island School of Medicine, United StatesReviewed by:
Sara Darbandi, Cleveland Clinic, United StatesGennaro Lettieri, University of Naples Federico II, Italy
Copyright © 2024 Yang, Xue, Zhou, Qiu, Wang, Ou and Zhou. This is an open-access article distributed under the terms of the Creative Commons Attribution License (CC BY). The use, distribution or reproduction in other forums is permitted, provided the original author(s) and the copyright owner(s) are credited and that the original publication in this journal is cited, in accordance with accepted academic practice. No use, distribution or reproduction is permitted which does not comply with these terms.
*Correspondence: Qing Zhou, c3VwZXJnb29uQDE2My5jb20=
†ORCID: Qing Zhou, orcid.org/0000-0002-9633-7542