- 1Department of Emergency, Henan Provincial People’s Hospital, People’s Hospital of Zhengzhou University, People’s Hospital of Henan University, Zhengzhou, China
- 2Department of Pediatric Rehabilitation, Henan Children’s Hospital Zhengzhou Children’s Hospital, Children’s Hospital Affiliated to Zhengzhou University, Zhengzhou, China
- 3Department of Convalescent Four Areas Nine Departments, Navy Qingdao Special Service Recuperation Center, Qingdao, China
- 4Department of General Surgery, Affiliated Xiaoshan Hospital, Hangzhou Normal University, Hangzhou, China
Background: The global increase in the aging population presents considerable challenges, particularly regarding cognitive impairment, a major concern for public health. This study investigates the association between the triglyceride-glucose (TyG) index, a measure of insulin resistance, and the risk of cognitive impairment in the elderly.
Methods: This prospective cohort study enrolled 2,959 participants aged 65 and above from the 2015 and 2020 waves of the China Health and Retirement Longitudinal Study (CHARLS). The analysis employed a logistic regression model to assess the correlation between the TyG index and cognitive impairment.
Results: The study included 2,959 participants, with a mean age of 71.2 ± 5.4 years, 49.8% of whom were female. The follow-up in 2020 showed a decrease in average cognitive function scores from 8.63 ± 4.61 in 2015 to 6.86 ± 5.45. After adjusting for confounding factors, a significant association was observed between TyG index quartiles and cognitive impairment. Participants in the highest quartile (Q4) of baseline TyG had a higher risk of cognitive impairment compared to those in the lowest quartile (Q1) (odds ratio [OR]: 1.97, 95% confidence intervals [CI]: 1.28–2.62, P<0.001).
Conclusion: The study highlights a significant connection between elevated TyG index levels and cognitive impairment among older adults in China. These findings suggest that targeted interventions to reduce the TyG index could mitigate cognitive impairment and potentially lower the incidence of dementia.
1 Introduction
The global aging phenomenon poses unprecedented challenges, notably in aggravating concerns associated with cognitive impairment (1–5). Cognitive impairment encompasses the reception and processing of information, characterized by memory loss, diminished understanding, impaired focus, and difficulties in calculation (6, 7). These cognitive alterations are regarded as the preclinical phase of dementia, possibly affected by factors like fasting glucose levels, physical performance, and other variables, highlighting its significance as a major public health concern (3, 8–10). Therefore, identifying risk factors to prevent cognitive impairment at early stages is essential (3, 6, 11–13).
There is growing evidence supporting the association between insulin resistance (IR) and the risk of cognitive decline (14–17). However, previous studies have primarily relied on the gold standard methods of insulin clamp and intravenous glucose tolerance test for diagnosing IR, which are not commonly performed in clinical settings (18–20).
The triglyceride-glucose (TyG) index, a readily available and cost-effective metric derived from triglyceride (TG) and fasting blood glucose (FBG) levels, has been recognized as a promising surrogate marker for IR (14, 18, 19, 21). Extensive epidemiological research has indicated significant links between the TyG index and various diseases, including cardiovascular diseases, cancers, and diabetes (22, 23). Nonetheless, the validity of the TyG index as an alternative indicator of IR in assessing its relationship with cognitive impairment is still in question. Prior investigations have largely concentrated on the TyG and cognitive function relationship within specific demographics, such as non-diabetic, gender-specific cohorts, or individuals living in rural areas, often focusing on specific cognitive domains (24–27).
This study, grounded in population-based research, seeks to explore the relationship between the TyG index and the occurrence of cognitive impairment in a wider elderly population. Utilizing the most recent cognitive function follow-up data from the 2020 China Health and Retirement Longitudinal Study (CHARLS) database, our work aims to offer an exhaustive and prolonged examination of this association across the entire elderly cohort. The study hypothesis is that elevated TyG index may be associated with cognitive impairment among older adults in China.
2 Materials and methods
2.1 Study population
The CHARLS is a comprehensive national study comprising five waves aimed at collecting health and social data from Chinese citizens aged 45 years and older (28). The CHARLS project aims to analyze the issue of population aging in China and promote interdisciplinary research on aging. CHARLS is a recurring survey conducted every 2 to 3 years. CHARLS employs a multi-stage probability proportional to size sampling approach, encompassing a sample frame of 450 villages, 150 counties, and 28 provinces. The study involves participation from over 20,000 individuals residing in approximately 10,000 households. Participants undergo face-to-face interviews at home using computer-assisted personal interviewing technology. Survey topics include basic demographic information of respondents and their families, intergenerational transfers within households, health status, medical insurance coverage, employment, income, expenditures, and assets. Additionally, CHARLS includes physical measurements and blood sample collection. Detailed information about CHARLS has been published in previous literature, and the CHARLS dataset is available for download on the CHARLS homepage at http://charls.pku.edu.cn/en. For our study, we used data from the 2015 and 2020 CHARLS surveys, with the former serving as the baseline. Initially, 5,511 participants aged 65 and older were selected from the database (25). To ensure the integrity of the data, we applied specific exclusion criteria: (1) participants with missing data on TG and FBG in 2015 (n = 1,844); (2) participants with missing data on cognitive function in 2015 and 2020 (n = 99); (3) participants with cognitive impairment in 2015 (n = 400); (4) ever having memory disorders or mental health conditions (n = 209). As a result, 2,959 participants satisfied all inclusion criteria and were incorporated into the study (Figure 1).
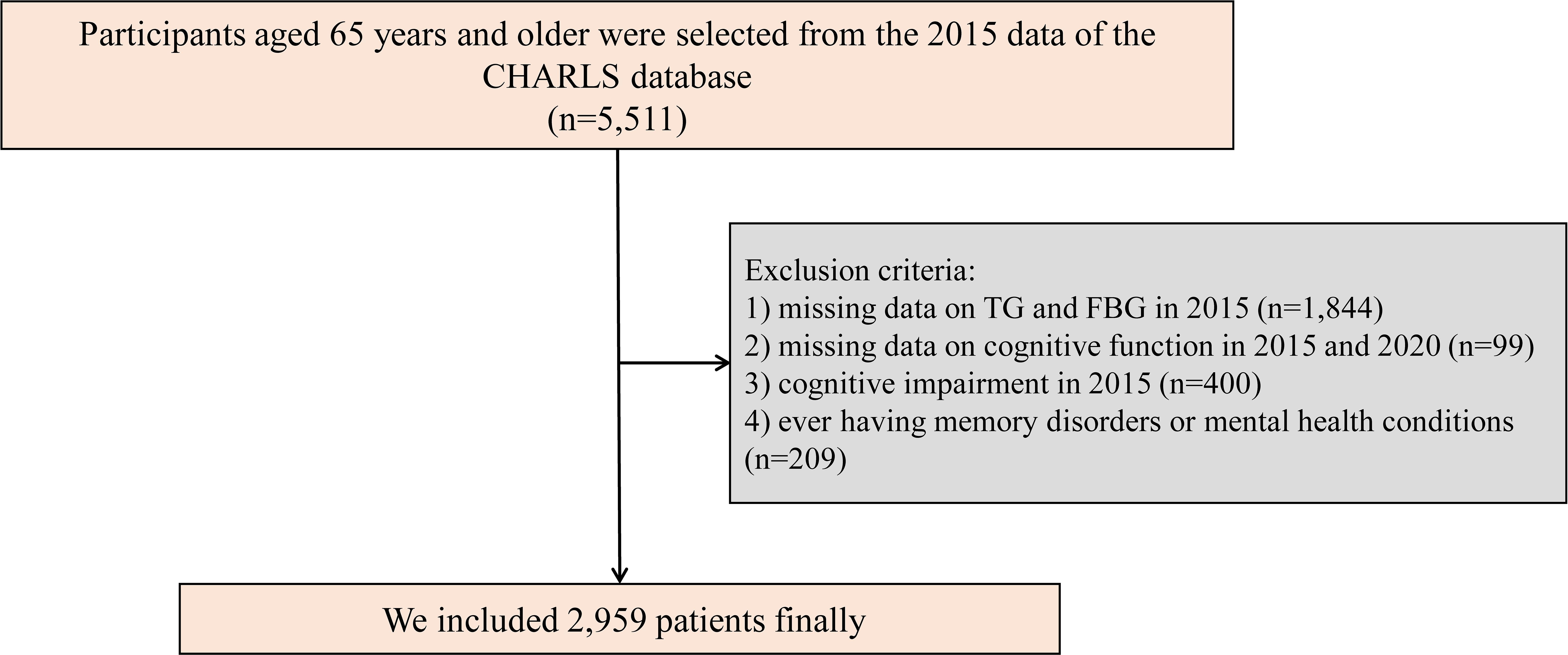
Figure 1 Study flowchart. CHARLS, China Health and Retirement Longitudinal Study; TG, triglyceride; FBG, fasting blood glucose.
CHARLS received ethical approval from the Ethical Review Committee of Peking University (IRB00001052-11015). All participants provided informed consent by signing consent forms before taking part in the study.
2.2 Measurement of cognitive function and TyG index
Assessments of cognitive function were conducted during the follow-up surveys in 2015 and 2020, incorporating tests for episodic memory and mental acuity using a method akin to that employed in the American Health and Retirement Study (28). Participants were first asked to remember a list of ten words immediately after an interviewer read them aloud. Approximately 4 minutes later, they were asked to recall these words once more (delayed recall). The evaluation of episodic memory was based on the average scores from both the immediate and delayed recall tasks, with possible scores ranging from 0 to 10. For assessing mental acuity, participants completed a series of tasks including drawing a specific figure accurately, answering questions regarding the current date, season, and day of the week, and performing a serial subtraction task (subtracting 7 from 100 in five consecutive attempts). Each correct answer earned one point, culminating in a maximum possible score of 11 points (29, 30). The aggregate of these scores represented the participant’s overall cognitive status, with total scores varying between 0 and 21, where higher scores signified superior cognitive function. According to previous research, cognitive impairment was determined as a score 1.0 standard deviation or more below the mean value of cognitive function (31–33).
TG and FBG levels were measured using a standard enzymatic colorimetric method. The TyG index was computed as ln (fasting TG [mg/dL] × FBG [mg/dL]/2) (14, 34). Participants were categorized into four groups (Q1, Q2, Q3, and Q4) based on quartiles of their TyG index.
2.3 Covariates
Covariates were selected based on previous research, baseline differences, and clinical significance (26, 33, 35). The potential confounders considered included age, gender, body mass index (BMI), educational level (illiterate, elementary school, middle school, and higher), place of residence (urban and rural), alcohol consumption (more than once a month, less than once a month, never), smoking status (current, former, never), hypertension (yes/no), diabetes (yes/no), overall health status (poor, fair, good, very good, and higher), and cognitive function in 2015.
2.4 Statistical analysis
Descriptive statistics were employed to summarize the data, presented as the mean (standard deviation [SD]), median (interquartile range [IQR]), or count and percentage, as appropriate. The chi-square test was used to examine differences among categorical variables. For continuous variables adhering to a normal distribution, one-way analysis of variance (ANOVA) was utilized. When continuous variables did not follow a normal distribution, the Kruskal–Wallis test was applied. These statistical methods aimed to evaluate the disparities and relationships between variables in the study.
Participants were categorized into four groups based on quartiles of the TyG index: Q1 (<8.21), Q2 (≥8.21–8.55), Q3 (≥8.55–8.97), and Q4 (≥8.97). A logistic regression model was used to investigate the independent associations between the TyG index and cognitive impairment, with results presented as adjusted odds ratios (OR) with 95% confidence intervals (CI). Covariates were selected based on previous literature and clinical insight (26, 33, 35). Model 1 was unadjusted; Model 2 adjusted for age and gender; and Model 3 further adjusted for age, gender, BMI, educational level, residence, drinking and smoking status, hypertension, diabetes, overall health, and cognitive function in 2015. We have also utilized Cox regression as a sensitivity analysis to further bolster our research findings. A subgroup analysis also explored the relationship between the TyG index and cognitive impairment across specific subgroups, including gender (male and female), age (65–75 years and ≥75 years), BMI (<24 kg/m2 and ≥24 kg/m2) (25), presence of chronic diseases (0 and ≥1), and diabetes (yes and no).
The statistical significance was determined using two-tailed tests, with a significance threshold of < 0.05 for the p values. All statistical analyses were performed using R software, version 4.2.2, developed by the R Foundation for Statistical Computing, based in Vienna, Austria.
3 Results
3.1 Baseline characteristics
The current study comprised a cohort of 2,959 participants. The participants’ mean age was 71.2 ± 5.4 years, with 49.8% being female (n = 1,475). They were categorized into four groups according to the quartiles of the TyG index: Q1 (739), Q2 (740), Q3 (740), and Q4 (740). A majority of the participants lived in rural areas (59.5%) and had attained an elementary school level of education (47.8%). The average BMI was recorded at 23.85 ± 7.68 kg/m2, and the mean score for depressive symptoms was 8.05 ± 6.50. Furthermore, 32.8% of the participants reported a history of chronic diseases, whereas 56.1% identified as current smokers. Participants in the highest TyG index group displayed a higher BMI, a greater proportion of females, and a more prevalent history of ≥2 chronic diseases, notably diabetes and hypertension. Table 1 details the baseline characteristics more comprehensively.
3.2 Association between TyG index and cognition function
In the cognitive function assessments conducted in 2015, the average score was 8.63 ± 4.61. A follow-up visit in 2020 indicated a progressive decline in cognitive function scores to 6.86 ± 5.45 (Table 1). After adjusting for confounding variables, a significant relationship emerged between the TyG index quartiles and cognitive impairment. Participants in Q4 were found to have a higher risk of cognitive impairment compared to those in Q1 (OR: 1.97, 95% CI: 1.28–2.62, P < 0.001). Nevertheless, the associations between the TyG index in Q2 and Q3 quartiles and cognitive impairment did not reach statistical significance in the 2020 follow-up (P > 0.05) (Table 2).
3.3 Subgroup and sensitivity analyses
The subgroup analysis demonstrated consistent outcomes across various stratified subgroups, such as gender, age, BMI, chronic diseases, and diabetes, with no significant interaction effects observed (P-interaction > 0.05). Participants in the highest quartile of the TyG index (Q4) exhibited an increased risk of cognitive impairment, with the exception of individuals characterized by a lower BMI, absence of chronic diseases, and male gender (Table 3). The sensitivity analysis yielded results consistent with the main findings, indicating a significant correlation between TyG index and cognitive impairment (Additional Table 1).
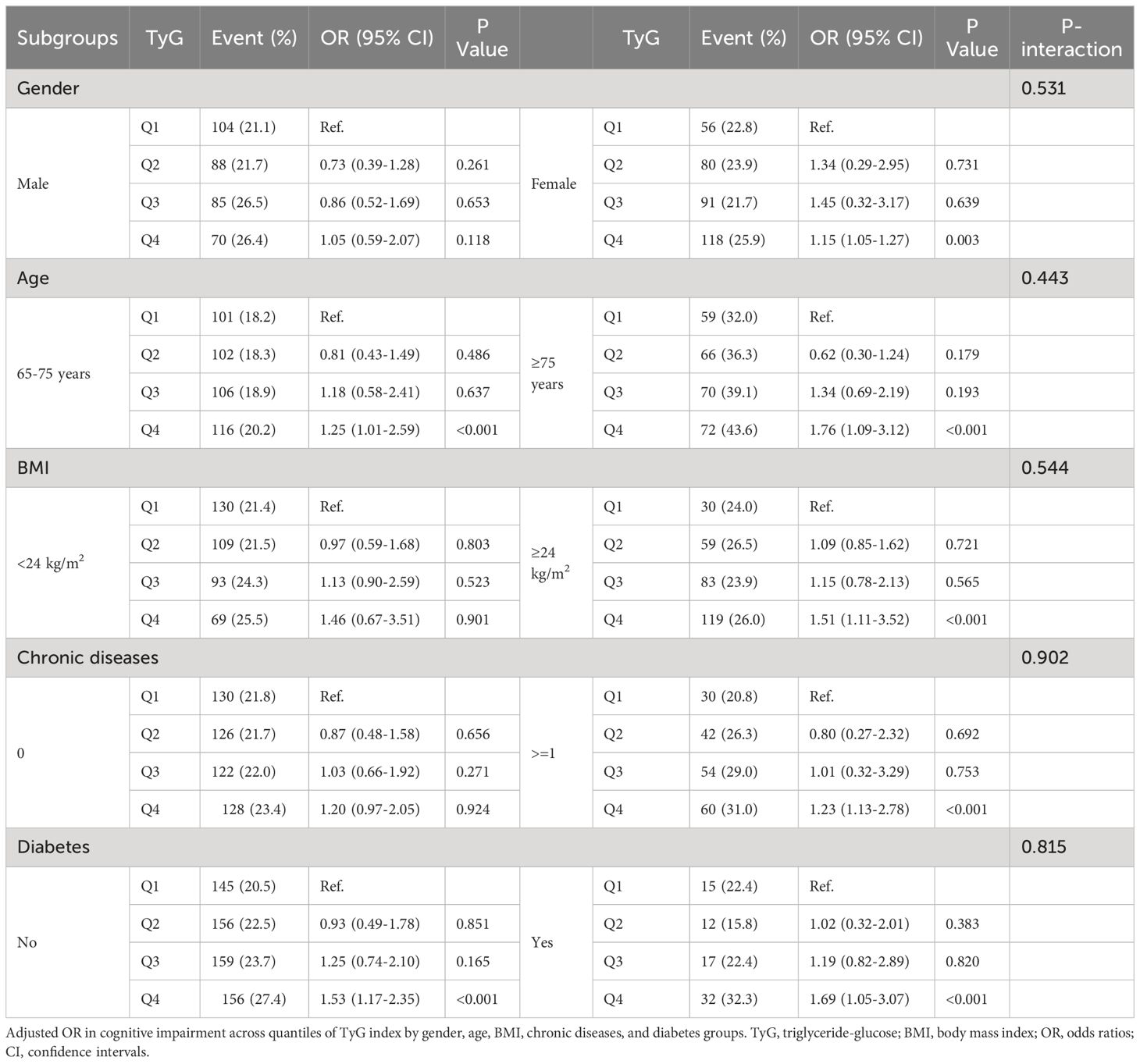
Table 3 Subgroup and interaction analysis between the TyG index and cognitive impairment in 2020 across various subgroups.
4 Discussion
In this study, we observed a significant association between a higher TyG index and cognitive impairment in older adults (aged 65 years and above). A follow-up visit in 2020 revealed a gradual decline in cognitive function scores compared to the baseline in 2015. Stratified analysis by age, gender, BMI, chronic diseases, and diabetes showed that individuals with a higher TyG index faced a greater risk of cognitive impairment, with the exception of those with lower BMI, those without chronic diseases, and males. These findings suggest that a higher TyG index could be a potential risk factor for cognitive impairment in older adults, particularly in females and in those with higher BMI and chronic diseases.
The aging global population presents an unprecedented challenge, raising significant concerns (1–3). The rapid pace of aging intensifies the challenges associated with cognitive impairment (4, 5). The age-related decline in cognitive function has become a significant public health issue, leading to adverse health outcomes (11, 13). Cognitive impairment often manifests years before the onset of dementia, underscoring the need to explore its mechanisms and to implement preventive measures aimed at risk factors. Previous research has identified several key factors contributing to cognitive decline, including genetic predisposition, cardiovascular disease, and exposure to air pollution (36–40).
IR is characterized by reduced sensitivity and responsiveness to the effects of insulin, acting as a central factor in the emergence of various health issues, such as diabetes, cardiovascular diseases, and cognitive decline (3, 14). Moreover, some evidence suggests that IR is linked to an increased risk of cognitive decline (14–16). The hyperinsulinemic euglycemic clamp, despite its exceptional sensitivity in assessing the body’s response to insulin, is costly and complex, limiting its use in clinical environments. The homeostasis model assessment of insulin resistance (HOMA-IR), based on FPG and insulin measurements, is considered the gold standard for evaluating insulin sensitivity. However, the measurement of insulin levels is not commonly included in routine clinical practice, thereby restricting the use of HOMA-IR in such contexts. The TyG index, a practical measure of IR derived from TG and FBG levels, offers a cost-effective and accessible alternative for IR assessment, gaining widespread adoption in research (14, 18, 19). Epidemiological studies have linked the TyG index to various conditions, including cardiovascular diseases, cancers, and diabetes. Yet, the effectiveness of the TyG index as an indirect marker for IR in exploring its association with cognitive impairment remains to be fully ascertained.
Previous research on the TyG index and cognitive function has predominantly focused on specific demographic groups, such as non-diabetic individuals, gender-specific populations, and rural dwellers, often categorizing cognitive function into distinct domains (24–27). Utilizing data from the National Health and Nutrition Examination Survey (NHANES), Wei et al. found a notable correlation between a high TyG index and reduced cognitive function, as determined by the CERAD test, in non-diabetic elderly individuals in the United States (24). Similarly, a study among elderly residents in rural China linked elevated TyG index values with diminished cognitive performance and brain atrophy (25).
Our population-based study aimed to explore the relationship between the TyG index and cognitive impairment among the elderly population in China. Utilizing data from a continuous cohort, we collected information from consecutive cognitive assessments conducted in 2020. We found a significant association between a higher TyG index and cognitive impairment in older adults during the follow-up visits in 2020, especially pronounced in females, individuals with a higher BMI, and those suffering from chronic conditions. Additionally, our findings showed that cognitive function scores were lower in females than in males. These results align with previous research. Earlier investigations have consistently indicated that females are at a greater risk of cognitive impairments compared to males (3, 41, 42). A study examining a 5-year change in the TyG index and its impact on cognitive function found that females in the second quartile of longitudinal TyG index change exhibited a significant association with reduced cognitive performance as measured by the CERAD test (26). Moreover, it was noted that females have a higher vulnerability to cognitive impairment compared to males (3).
Several factors might elucidate the observed results, necessitating consideration of the underlying mechanisms. Insulin possesses the ability to traverse the blood-brain barrier through specific receptors, affecting both behavioral and metabolic functions (17, 43). IR represents a unique metabolic disorder often characterized by increased insulin levels, which can lead to neurodegeneration and persistent memory impairments due to prolonged exposure of brain neurons to elevated insulin levels (44). Furthermore, IR could diminish cerebral glucose metabolism in particular brain regions, possibly adversely affecting memory function in individuals (45). Some clinical studies have indicated that TG can cross the blood-brain barrier, potentially impairing cognitive function by inducing insulin receptor resistance (46). In our study, most female participants were postmenopausal, a phase associated with reduced estrogen levels. Previous research has identified estrogen as vital for learning and memory, providing neuroprotection and potentially enhancing cognitive function through estrogen therapy (47, 48). This could explain the more pronounced cognitive decline observed in females compared to males in our study. In the BMI-stratified analysis, the link between an elevated TyG index and cognitive impairment was observed solely in overweight and obese individuals, highlighting IR’s traditional association with obesity, which is closely related to brain atrophy (25). Additionally, a study examining the relationship between the TyG index and lower brain volume found this association exclusively in individuals with a BMI ≥24 kg/m2 (25).
The issue of cognitive impairment is becoming increasingly evident as the population ages. Serving as an early indicator of dementia, cognitive impairment is associated with a notably adverse prognosis, affecting individual quality of life and placing burdens on families and society. The early prevention of cognitive impairment is thus critical and urgent. Our research suggests that the TyG index could serve as an alternative marker of IR for predicting cognitive impairment in individuals over 65 years old in China, with a significant relationship observed between high TyG index and cognitive impairment. Targeted interventions could help in mitigating cognitive impairment, potentially decreasing the incidence of dementia. Additionally, further exploration into identifying more predictive risk factors for cognitive impairment is necessary to achieve the objective of early prevention.
This study has several limitations. First, it is important to recognize that the study was observational. Despite efforts to adjust for known confounders, the potential impact of unmeasured confounders on the outcomes cannot be disregarded. Therefore, the applicability of our findings may be somewhat restricted. Moreover, we evaluate cognitive function using episodic memory and mental acuity rather than clinical diagnosis. Comprehensive assessment across multiple dimensions is crucial for understanding overall cognitive function, highlighting limitations when evaluating solely based on episodic memory and mental acuity. However, these neuropsychological tests are widely regarded as reliable screening tools for measuring cognitive function and are extensively used in clinical practice (49). Finally, due to data availability limitations, our analysis was confined to a 5-year follow-up period from 2015 to 2020. Future studies should consider employing repeated-measures designs over longer durations and examining more potential pathways. Subsequent research is warranted to refine these findings further.
5 Conclusion
The results of this study reveal a notable association between elevated TyG index levels and the occurrence of cognitive impairment among the elderly Chinese demographic. The initiation of targeted intervention strategies may effectively mitigate cognitive impairment, potentially decreasing the prevalence of dementia.
Data availability statement
The datasets presented in this study can be found in online repositories. The names of the repository/repositories and accession number(s) can be found below: http://charls.pku.edu.cn/pages/data/111/zhcn.html.
Ethics statement
The studies involving humans were approved by the Ethical Review Committee of Peking University (IRB00001052-11015). The studies were conducted in accordance with the local legislation and institutional requirements. Written informed consent for participation was not required from the participants or the participants’ legal guardians/next of kin in accordance with the national legislation and institutional requirements.
Author contributions
WMB: Data curation, Formal analysis, Investigation, Methodology, Validation, Writing – original draft. SA: Data curation, Investigation, Project administration, Validation, Writing – original draft. HJ: Data curation, Formal analysis, Investigation, Project administration, Software, Writing – original draft. JX: Conceptualization, Formal analysis, Methodology, Resources, Supervision, Writing – review & editing. LJQ: Data curation, Formal analysis, Investigation, Methodology, Resources, Supervision, Writing – review & editing.
Funding
The author(s) declare that no financial support was received for the research, authorship, and/or publication of this article.
Acknowledgments
We extend our sincere gratitude to Peking University for granting access to the CHARLS database, and we appreciate the participation of all individuals included in the database.
Conflict of interest
The authors declare that the research was conducted in the absence of any commercial or financial relationships that could be construed as a potential conflict of interest.
Publisher’s note
All claims expressed in this article are solely those of the authors and do not necessarily represent those of their affiliated organizations, or those of the publisher, the editors and the reviewers. Any product that may be evaluated in this article, or claim that may be made by its manufacturer, is not guaranteed or endorsed by the publisher.
Supplementary material
The Supplementary Material for this article can be found online at: https://www.frontiersin.org/articles/10.3389/fendo.2024.1398235/full#supplementary-material
References
1. Beard JR, Officer A, de Carvalho IA, Sadana R, Pot AM, Michel JP, et al. The World report on ageing and health: a policy framework for healthy ageing. Lancet. (2016) 387:2145–54. doi: 10.1016/S0140-6736(15)00516-4
2. Gerland P, Raftery AE, Sevčíková H, Li N, Gu D, Spoorenberg T, et al. World population stabilization unlikely this century. Science. (2014) 346:234–7. doi: 10.1126/science.1257469
3. Jia L, Du Y, Chu L, Zhang Z, Li F, Lyu D, et al. Prevalence, risk factors, and management of dementia and mild cognitive impairment in adults aged 60 years or older in China: a cross-sectional study. Lancet Public Health. (2020) 5:e661–71. doi: 10.1016/S2468-2667(20)30185-7
4. Hebert LE, Weuve J, Scherr PA, Evans DA. Alzheimer disease in the United States (2010-2050) estimated using the 2010 census. Neurology. (2013) 80:1778–83. doi: 10.1212/WNL.0b013e31828726f5
5. Weuve J, Hebert LE, Scherr PA, Evans DA. Deaths in the United States among persons with Alzheimer's disease (2010-2050). Alzheimer's Dementia J Alzheimer's Assoc. (2014) 10:e40–46. doi: 10.1016/j.jalz.2014.01.004
6. Global, regional, and national burden of Alzheimer's disease and other dementias, 1990-2016: a systematic analysis for the Global Burden of Disease Study 2016. Lancet Neurol. (2019) 18:88–106. doi: 10.1016/S1474-4422(18)30403-4
7. McArdle JJ, Ferrer-Caja E, Hamagami F, Woodcock RW. Comparative longitudinal structural analyses of the growth and decline of multiple intellectual abilities over the life span. Dev Psychol. (2002) 38:115–42. doi: 10.1037/0012-1649.38.1.115
8. Aarsland D, Creese B, Politis M, Chaudhuri KR, Ffytche DH, Weintraub D, et al. Cognitive decline in Parkinson disease. Nat Rev Neurol. (2017) 13:217–31. doi: 10.1038/nrneurol.2017.27
9. Bianco A, Pomara F, Thomas E, Paoli A, Battaglia G, Petrucci M, et al. Type 2 diabetes family histories, body composition and fasting glucose levels: a cross-section analysis in healthy sedentary male and female. Iranian J Public Health. (2013) 42:681–90.
10. Patti A, Bianco A, Karsten B, Montalto MA, Battaglia G, Bellafiore M, et al. The effects of physical training without equipment on pain perception and balance in the elderly: A randomized controlled trial. Work. (2017) 57:23–30. doi: 10.3233/WOR-172539
11. Yuan L, Zhang X, Guo N, Li Z, Lv D, Wang H, et al. Prevalence of cognitive impairment in Chinese older inpatients and its relationship with 1-year adverse health outcomes: a multi-center cohort study. BMC Geriatrics. (2021) 21:595. doi: 10.1186/s12877-021-02556-5
12. Seshadri S, Wolf PA. Lifetime risk of stroke and dementia: current concepts, and estimates from the Framingham Study. Lancet Neurol. (2007) 6:1106–14. doi: 10.1016/S1474-4422(07)70291-0
13. 2021 Alzheimer's disease facts and figures. Alzheimer's Dementia J Alzheimer's Assoc. (2021) 17:327–406. doi: 10.1002/alz.12328
14. Hong S, Han K, Park CY. The insulin resistance by triglyceride glucose index and risk for dementia: population-based study. Alzheimer's Res Ther. (2021) 13:9. doi: 10.1186/s13195-020-00758-4
15. Hooshmand B, Rusanen M, Ngandu T, Leiviskä J, Sindi S, von Arnim CAF, et al. Serum insulin and cognitive performance in older adults: A longitudinal study. Am J Med. (2019) 132:367–73. doi: 10.1016/j.amjmed.2018.11.013
16. Neergaard JS, Dragsbæk K, Christiansen C, Nielsen HB, Brix S, Karsdal MA, et al. Metabolic syndrome, insulin resistance, and cognitive dysfunction: does your metabolic profile affect your brain? Diabetes. (2017) 66:1957–63. doi: 10.2337/db16-1444
17. Kullmann S, Heni M, Hallschmid M, Fritsche A, Preissl H, Häring HU. Brain insulin resistance at the crossroads of metabolic and cognitive disorders in humans. Physiol Rev. (2016) 96:1169–209. doi: 10.1152/physrev.00032.2015
18. Brito ADM, Hermsdorff HHM, Filgueiras MS, Suhett LG, Vieira-Ribeiro SA, Franceschini S, et al. Predictive capacity of triglyceride-glucose (TyG) index for insulin resistance and cardiometabolic risk in children and adolescents: a systematic review. Crit Rev Food Sci Nutr. (2021) 61:2783–92. doi: 10.1080/10408398.2020.1788501
19. Minh HV, Tien HA, Sinh CT, Thang DC, Chen CH, Tay JC, et al. Assessment of preferred methods to measure insulin resistance in Asian patients with hypertension. J Clin Hypertension (Greenwich Conn). (2021) 23:529–37. doi: 10.1111/jch.14155
20. Wallace TM, Levy JC, Matthews DR. Use and abuse of HOMA modeling. Diabetes Care. (2004) 27:1487–95. doi: 10.2337/diacare.27.6.1487
21. García AG, Urbina Treviño MV, Villalpando Sánchez DC, Aguilar CA. Diagnostic accuracy of triglyceride/glucose and triglyceride/HDL index as predictors for insulin resistance in children with and without obesity. Diabetes Metab Syndrome. (2019) 13:2329–34. doi: 10.1016/j.dsx.2019.05.020
22. Won KB, Lee BK, Park HB, Heo R, Lee SE, Rizvi A, et al. Quantitative assessment of coronary plaque volume change related to triglyceride glucose index: The Progression of AtheRosclerotic PlAque DetermIned by Computed TomoGraphic Angiography IMaging (PARADIGM) registry. Cardiovasc Diabetol. (2020) 19:113. doi: 10.1186/s12933-020-01081-w
23. Fritz J, Bjørge T, Nagel G, Manjer J, Engeland A, Häggström C, et al. The triglyceride-glucose index as a measure of insulin resistance and risk of obesity-related cancers. Int J Epidemiol. (2020) 49:193–204. doi: 10.1093/ije/dyz053
24. Wei B, Dong Q, Ma J, Zhang A. The association between triglyceride-glucose index and cognitive function in nondiabetic elderly: NHANES 2011-2014. Lipids Health Dis. (2023) 22:188. doi: 10.1186/s12944-023-01959-0
25. Tian N, Song L, Hou T, Fa W, Dong Y, Liu R, et al. Association of triglyceride-glucose index with cognitive function and brain atrophy: A population-based study. Am J Geriatric Psychiatry Off J Am Assoc Geriatric Psychiatry. (2024) 32:151–62. doi: 10.1016/j.jagp.2023.09.007
26. Wang K, Xu L, Liu L, Zhan S, Wang S, Song Y. Sex differences in the association between the change in triglyceride−glucose index and cognitive decline: A population-based cohort study. J Affect Disord. (2022) 316:42–9. doi: 10.1016/j.jad.2022.08.014
27. Li S, Deng X, Zhang Y. The triglyceride-glucose index is associated with longitudinal cognitive decline in a middle-aged to elderly population: A cohort study. J Clin Med. (2022) 11(23):7153. doi: 10.3390/jcm11237153
28. Zhao Y, Hu Y, Smith JP, Strauss J, Yang G. Cohort profile: the China health and retirement longitudinal study (CHARLS). Int J Epidemiol. (2014) 43:61–8. doi: 10.1093/ije/dys203
29. Ding R, He P. Associations between childhood adversities and late-life cognitive function: Potential mechanisms. Soc Sci Med. (2021) 291:114478. doi: 10.1016/j.socscimed.2021.114478
30. Lu N, Wu B, Pei Y. Exploring the reciprocal relationship between cognitive function and edentulism among middle-aged and older adults in China. Age Ageing. (2021) 50:809–14. doi: 10.1093/ageing/afaa173
31. Bai A, Shi H, Huang X, Xu W, Deng Y. Association of C-reactive protein and motoric cognitive risk syndrome in community-dwelling older adults: the China health and retirement longitudinal study. J Nutrition Health Aging. (2021) 25:1090–5. doi: 10.1007/s12603-021-1678-3
32. Jak AJ, Bondi MW, Delano-Wood L, Wierenga C, Corey-Bloom J, Salmon DP, et al. Quantification of five neuropsychological approaches to defining mild cognitive impairment. Am J Geriatric Psychiatry Off J Am Assoc Geriatric Psychiatry. (2009) 17:368–75. doi: 10.1097/JGP.0b013e31819431d5
33. Chai S, Zhao D, Gao T, Wang X, Wang X, Luo J, et al. The relationship between handgrip strength and cognitive function among older adults in China: Functional limitation plays a mediating role. J Affect Disord. (2024) 347:144–9. doi: 10.1016/j.jad.2023.11.056
34. Wang A, Tian X, Zuo Y, Chen S, Meng X, Wu S, et al. Change in triglyceride-glucose index predicts the risk of cardiovascular disease in the general population: a prospective cohort study. Cardiovasc Diabetol. (2021) 20:113. doi: 10.1186/s12933-021-01305-7
35. Pan X, Luo Y, Zhao D, Zhang L. Associations among drinking water quality, dyslipidemia, and cognitive function for older adults in China: evidence from CHARLS. BMC Geriatrics. (2022) 22:683. doi: 10.1186/s12877-022-03375-y
36. Schram MT, Euser SM, de Craen AJ, Witteman JC, Frölich M, Hofman A, et al. Systemic markers of inflammation and cognitive decline in old age. J Am Geriatrics Society. (2007) 55:708–16. doi: 10.1111/j.1532-5415.2007.01159.x
37. Baumgart M, Snyder HM, Carrillo MC, Fazio S, Kim H, Johns H. Summary of the evidence on modifiable risk factors for cognitive decline and dementia: A population-based perspective. Alzheimer's Dementia J Alzheimer's Assoc. (2015) 11:718–26. doi: 10.1016/j.jalz.2015.05.016
38. Griffiths CJ, Mudway IS. Air pollution and cognition. BMJ. (2018) 363:k4904. doi: 10.1136/bmj.k4904
39. Paul KC, Haan M, Mayeda ER, Ritz BR. Ambient air pollution, noise, and late-life cognitive decline and dementia risk. Annu Rev Public Health. (2019) 40:203–20. doi: 10.1146/annurev-publhealth-040218-044058
40. Schikowski T, Altuğ H. The role of air pollution in cognitive impairment and decline. Neurochem Int. (2020) 136:104708. doi: 10.1016/j.neuint.2020.104708
41. Cnop M, Havel PJ, Utzschneider KM, Carr DB, Sinha MK, Boyko EJ, et al. Relationship of adiponectin to body fat distribution, insulin sensitivity and plasma lipoproteins: evidence for independent roles of age and sex. Diabetologia. (2003) 46:459–69. doi: 10.1007/s00125-003-1074-z
42. Meyer MR, Clegg DJ, Prossnitz ER, Barton M. Obesity, insulin resistance and diabetes: sex differences and role of oestrogen receptors. Acta Physiol. (2011) 203:259–69. doi: 10.1111/apha.2011.203.issue-1
43. Soto M, Cai W, Konishi M, Kahn CR. Insulin signaling in the hippocampus and amygdala regulates metabolism and neurobehavior. Proc Natl Acad Sci United States America. (2019) 116:6379–84. doi: 10.1073/pnas.1817391116
44. Blázquez E, Velázquez E, Hurtado-Carneiro V, Ruiz-Albusac JM. Insulin in the brain: its pathophysiological implications for States related with central insulin resistance, type 2 diabetes and Alzheimer's disease. Front Endocrinol. (2014) 5:161. doi: 10.3389/fendo.2014.00161
45. Willette AA, Bendlin BB, Starks EJ, Birdsill AC, Johnson SC, Christian BT, et al. Association of insulin resistance with cerebral glucose uptake in late middle-aged adults at risk for alzheimer disease. JAMA Neurol. (2015) 72:1013–20. doi: 10.1001/jamaneurol.2015.0613
46. Banks WA, Farr SA, Salameh TS, Niehoff ML, Rhea EM, Morley JE, et al. Triglycerides cross the blood-brain barrier and induce central leptin and insulin receptor resistance. Int J Obes. (2018) 42:391–7. doi: 10.1038/ijo.2017.231
47. Engler-Chiurazzi EB, Singh M, Simpkins JW. From the 90's to now: A brief historical perspective on more than two decades of estrogen neuroprotection. Brain Res. (2016) 1633:96–100. doi: 10.1016/j.brainres.2015.12.044
48. Hara Y, Waters EM, McEwen BS, Morrison JH. Estrogen effects on cognitive and synaptic health over the lifecourse. Physiol Rev. (2015) 95:785–807. doi: 10.1152/physrev.00036.2014
Keywords: triglyceride glucose, cognitive impairment, elderly, insulin resistance, CHARLS
Citation: Bai W, An S, Jia H, Xu J and Qin L (2024) Relationship between triglyceride-glucose index and cognitive function among community-dwelling older adults: a population-based cohort study. Front. Endocrinol. 15:1398235. doi: 10.3389/fendo.2024.1398235
Received: 09 March 2024; Accepted: 08 July 2024;
Published: 22 July 2024.
Edited by:
Kenju Shimomura, Fukushima Medical University, JapanReviewed by:
Jinhui Zhou, Chinese Center for Disease Control and Prevention, ChinaGuoxin Ni, First Affiliated Hospital of Xiamen University, China
Giuseppe Battaglia, University of Palermo, Italy
Copyright © 2024 Bai, An, Jia, Xu and Qin. This is an open-access article distributed under the terms of the Creative Commons Attribution License (CC BY). The use, distribution or reproduction in other forums is permitted, provided the original author(s) and the copyright owner(s) are credited and that the original publication in this journal is cited, in accordance with accepted academic practice. No use, distribution or reproduction is permitted which does not comply with these terms.
*Correspondence: Lijie Qin, qinlijie1819@163.com; Juan Xu, sophia2932@163.com
†These authors have contributed equally to this work and share first authorship