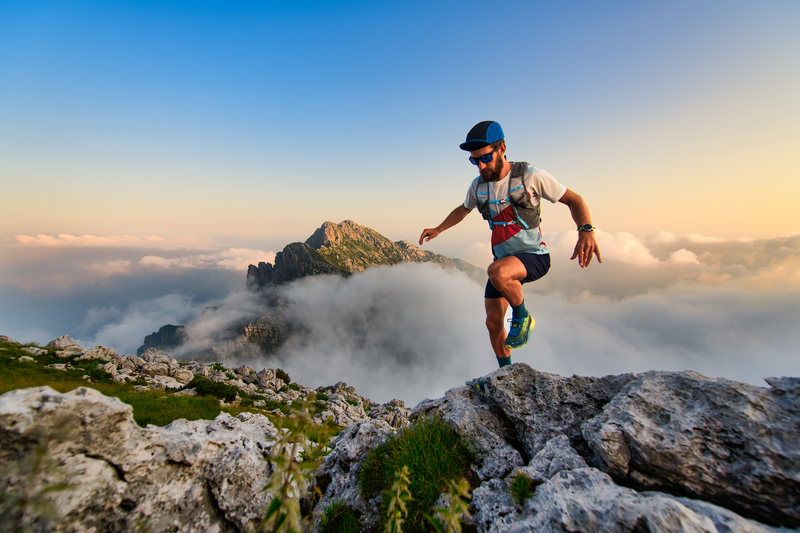
95% of researchers rate our articles as excellent or good
Learn more about the work of our research integrity team to safeguard the quality of each article we publish.
Find out more
ORIGINAL RESEARCH article
Front. Endocrinol. , 11 November 2024
Sec. Clinical Diabetes
Volume 15 - 2024 | https://doi.org/10.3389/fendo.2024.1388751
Objective: Few studies have evaluated the changes in blood glucose status in individuals with prediabetes, and this study aimed to analyze the association between metabolic score for insulin resistance (MetS-IR) and the progression or regression of prediabetes.
Methods: This retrospective cohort study used research data from medical examination institutions under the Rich Healthcare Group in 32 regions across 11 cities in China. Progression of prediabetes to diabetes and regression to normal fasting glucose (NFG) were defined based on glycemic changes during follow-up. The association between MetS-IR and the progression or regression of prediabetes was analyzed using multivariate Cox regression, restricted cubic splines, and piecewise regression models.
Results: Data from 15,421 prediabetic subjects were analyzed. Over an average follow-up of 2.96 years, 6,481 individuals (42.03%) returned to NFG, and 2,424 (15.72%) progressed to diabetes. After controlling for confounding factors, an increase in MetS-IR was observed to increase the risk of diabetes onset in the prediabetic population, whereas a decrease in MetS-IR had a protective effect for returning to NFG. Additionally, a nonlinear relationship between MetS-IR and prediabetes regression was observed, with 37.22 identified as the inflection point; prediabetes regression rates were significantly higher before this point and markedly decreased after it.
Conclusion: For individuals with prediabetes, an increase in MetS-IR may lead to an increased risk of diabetes; conversely, a decrease in MetS-IR enhances the protective effect for returning to NFG and keeping MetS-IR below 37.22 is significant for the regression of prediabetes.
Prediabetes is an intermediate state defined by blood glucose levels that are below the threshold for diabetes but above normal glucose levels (1). Currently, this intermediate state directly affects over 400 million people globally, and it is projected that by 2045, over 600 million people will be in this state (2). Prediabetes, as the term suggests, is a necessary phase for the majority of diabetes patients. However, recent studies have found that prediabetes not only plays a significant role in the onset of diabetes (1–4), but it also substantially increases the risk of vascular-related diseases, neurological disorders, cancer, kidney diseases (5–7), and is closely associated with accelerated brain aging and bone loss (8, 9), even significantly impacting life expectancy (8). These findings emphasize the widespread adverse effects of prediabetes on health; fortunately, the prediabetic state offers considerable potential for reversing blood glucose regulation back to normal and significantly reducing the risk of diabetes and its complications. Randomized controlled trials have shown that approximately 32.2%-52.1% of individuals with prediabetes return to normal blood glucose levels in the short term through intensified lifestyle or pharmacological interventions, and these remissions and benefits continue to increase over time (10–21). Therefore, from a public health perspective, early identification and monitoring of important modifiable factors that can affect the progression and regression of prediabetes are crucial for diabetes prevention and intervention.
Insulin resistance (IR) is one of the most important pathophysiological characteristics of both prediabetes and diabetes (1, 3, 4), and early monitoring and control of IR are helpful for the regression of prediabetes (22–24). The hyperinsulinemic-euglycemic clamp (HEGC), developed in the 1970s, has long been the gold standard for measuring IR (25). However, with the rapid development of epidemiology, large-scale population screening and monitoring have become more common, making HEGC less suitable for epidemiological screening due to its relative complexity and invasiveness. To address this, researchers have developed various simple and convenient surrogate measures of IR to replace HEGC (26). Metabolic score for insulin resistance (MetS-IR), proposed by Professor Bello-Chavolla OY and his team, is a promising new surrogate measure of IR (27). In initial studies, Bello-Chavolla OY et al. compared the diagnostic performance of MetS-IR against the M-value adjusted by fat-free mass obtained by the HEGC and found that MetS-IR had a better correlation with detecting IR compared to HEGC. Subsequent studies further confirmed the significant value of MetS-IR in predicting and assessing the risk of diabetes (28, 29). Recently, we also evaluated the effects of multiple IR surrogates including triglycerides glucose index, triglycerides glucose-body mass index, triglycerides/high-density lipoprotein cholesterol (TG/HDL-C) ratio and MetS-IR on diabetes progression based on two independent cohorts in China and Japan (30); Our preliminary study found that MetS-IR is the best IR surrogate for Chinese and Japanese populations in terms of risk assessment and prediction of diabetes progression. However, the association of MetS-IR with the progression or regression of prediabetes remains unclear. Given the modifiable nature of the prediabetic state, clarifying the relationship between the IR surrogate MetS-IR and the progression or regression of prediabetes early on has significant clinical value for early intervention and reversal of prediabetes. To address this issue, in this study, we aimed to determine the relationship between MetS-IR and the progression or regression of prediabetes.
All data for this study were sourced from a multicenter health examination cohort of the Rich Healthcare Group in China. The multicenter retrospective cohort study, composed of a general examination population, aimed to explore risk factors influencing the onset of diabetes. The available dataset was organized and uploaded to the Dryad database by Professor Chen and his team for public sharing (31). According to the Dryad Data Sharing Terms and the Creative Commons Attribution-NonCommercial-ShareAlike 4.0 International License, researchers are permitted to use the datasets publicly shared in the Dryad database for secondary creation, following the proper citation of the data source (31).
The Rich Healthcare Group multicenter health examination cohort was established by Professor Chen and his team. It includes adults (685,277) who had at least two health examinations at Rich Healthcare Group facilities across 32 areas in 11 Chinese cities from 2010 to 2016. Initially, Chen and colleagues aimed to explore the impact of the obesity measurement parameter, body mass index (BMI), on diabetes onset (32). Based on the research objectives, they excluded subjects with: (i) a clear diabetes diagnosis at baseline or diabetic status could not be determined during subsequent visits (6,630 subjects); (ii) less than a 2-year interval between two visits (324,233 subjects); (iii) a BMI less than 15 kg/m² or higher than 55 kg/m² at baseline (152 subjects); (iv) missing age, gender, and fasting plasma glucose (FPG) data at baseline (135,317 subjects). Ultimately, 211,833 subjects were included in Chen et al.’s study.
The current study, using the shared dataset (31) provided by Chen and colleagues, aimed to further explore the association between the IR surrogate MetS-IR and the progression or regression of prediabetes. Based on the inclusion and exclusion criteria established by Chen et al., we further excluded subjects with: (i) non-prediabetes status at baseline (185,815 subjects); (ii) missing MetS-IR data at baseline (10,594 subjects); (iii) missing FPG measurements during follow-up (3 subjects). Finally, our study included 15,421 subjects for analysis.
As a secondary analysis, the current study’s protocol was submitted to and approved by the Ethics Committee of Jiangxi Provincial People’s Hospital. Considering that this research is a secondary creation based on completed studies and the dataset is anonymized, the Committee waived the requirement for informed consent from each study participant.
During each visit to the examination centers, each participant, assisted by medical staff, recorded their diabetes history, family history of diabetes, personal lifestyle information (drinking/smoking status), demographic information (gender and age), and basic physical measurement parameters (blood pressure, height, and weight). Blood pressure was measured using a sphygmomanometer, recording systolic blood pressure (SBP) and diastolic blood pressure (DBP). Height and weight were measured without shoes and in light clothing, recorded to 0.001m and 0.1kg precision, respectively, and used to calculate each individual’s BMI.
Biochemical blood measurements were conducted on venous blood drawn after at least 10 hours of fasting. In standard laboratories, professional technicians measured total cholesterol (TC), TG, HDL-C, low-density lipoprotein cholesterol (LDL-C), FPG, creatinine (Cr), blood urea nitrogen (BUN), alanine aminotransferase (ALT), and aspartate aminotransferase (AST) using an automatic analyzer (Beckman 5800).
Calculation of MetS-IR: (27).
The study outcomes were primarily categorized into three groups: regression of prediabetes, maintenance of prediabetes status, and progression to diabetes. Based on the American Diabetes Association’s criteria for prediabetes/diabetes using FPG, we defined the following study outcomes (33): (i) regression of prediabetes defined as an FPG measurement less than 5.6 mmol/L during follow-up; (ii) maintenance of the prediabetic state defined as FPG levels between 5.6 and 6.9 mmol/L during follow-up. (iii) progression to diabetes defined as an FPG measurement above 7.0 mmol/L during follow-up or self-reported diagnosis of diabetes by other healthcare professionals.
Participants were categorized according to the study outcomes and their baseline characteristics were compared using univariate analysis of variance/Chi-square test/Kruskal-Wallis H test. Baseline information results were presented as median (interquartile range)/mean (standard deviation)/frequency (%).
For the three-category study outcomes, we considered participants who continued to maintain prediabetes status during follow-up as the non-event group and used a one-to-one method to split the data into binary datasets with prediabetes regression or progression as outcomes (34, 35). The association between MetS-IR and the progression or regression of prediabetes was analyzed using Cox regression models. Prior to model establishment, we assessed the collinearity of MetS-IR with other covariates using prediabetes progression or regression as outcome variables (Supplementary Tables 1, 2), as well as the Schoenfeld residuals plots of MetS-IR over time (Supplementary Figures 1, 2) (36, 37). After confirming that the Cox models met the proportional hazards assumption and identifying weight, BMI, and TC as collinear variables, we constructed four differently adjusted Cox regression models according to the STROBE statement: The unadjusted model was first constructed, analyzing the association between MetS-IR and the progression or regression of prediabetes without adjusting for any variables; then, Model I adjusted for demographic and lifestyle factors (age, gender, height, family history of diabetes, smoking status, and drinking status); Model II further considered blood pressure, blood glucose, and blood lipid-related factors (SBP, DBP, FPG, TG, HDL-C, and LDL-C) based on Model I; Model III, the final model, additionally considered liver and kidney function impacts (ALT, BUN, Cr). On the basis of adjusting covariates in Model III, we also plotted dose-response curves of MetS-IR with prediabetes regression or progression using 4-knot restricted cubic splines (RCS) regression model. When nonlinear associations were detected, potential inflection point values were calculated using recursive algorithms, and segmented Cox regression was used to assess HR values for prediabetes progression or regression before and after the inflection point.
Additionally, several sensitivity analyses were conducted: (i) To further verify the validity of the association between MetS-IR and prediabetes progression or regression, we treated the continuous variable MetS-IR as a categorical variable based on its quintiles and conducted similar analyses. (ii) According to the World Health Organization (WHO) standards for prediabetes/diabetes based on FPG (38), we analyzed the association between MetS-IR and prediabetes progression or regression in a new study population. (iii) We further validated the aforementioned associations by applying models with diabetes progression and return to normal fasting glucose (NFG) as competing risks. (iv) Considering the indirect influence of family history of diabetes (39), we selected a study population without a family history of diabetes and repeated the above analyses. (v) To verify the appropriateness of the dose-response curves between MetS-IR and prediabetes progression or regression, we continued using 3- and 5-knot additional analyses to test the stability of RCS results.
We also conducted stratification to further explore whether the association between MetS-IR and prediabetes progression or regression existed in potential specific populations, based on age (according to WHO age division standards) (40), gender, and BMI (according to the Chinese Obesity Working Group BMI division standards) (41), and used likelihood ratio tests to detect whether this association was significantly different between stratifications.
All analyses were performed using R language version 4.2.1 and Empower(R) version 2.20 statistical software. A two-sided p-value of less than 0.05 was considered statistically significant.
Figure 1 illustrates the selection process of the study population. A total of 15,421 eligible prediabetic subjects were included in this study, with an average age of 50 years and a male-to-female ratio of 1.85:1. During the average follow-up period of 2.96 years, 6,481 individuals (42.03%) returned to NFG, 2,424 (15.72%) progressed to diabetes, and 6,481 (42.03%) continued to maintain their prediabetic status. The cumulative incidence function calculated the cumulative risk of prediabetes progression or regression, showing that the cumulative incidence rates of prediabetes progression or regression at the third, fourth, fifth, and sixth years were 9.86%/30.11%, 17.46%/48.22%, 24.86%/62.51%, and 29.02%/70.72%, respectively. Figure 2 displays the cumulative incidence curves of prediabetes progression or regression, indicating a higher adjustability for regression to NFG compared to progression to diabetes.
Figure 2. Cumulative incidence curve of prediabetes recovering to NFG or progressing to diabetes. NFG: normal fasting glucose.
We reviewed study characteristics of participants at baseline according to study outcomes at follow-up (Table 1). Reports indicated that female participants and those who quit smoking and drinking were more likely to return to NFG in the future. Additionally, subjects who returned to NFG typically had lower age, weight, BMI, SBP, DBP, FPG, TC, TG, LDL-C, ALT, AST, BUN, Cr levels at baseline. In addition, it is worth noting that among the subjects, those who reverted to NFG and those who later progressed to diabetes had the lowest and highest baseline MetS-IR, respectively (Figure 3); This result suggested that high MetS-IR may be a factor promoting the occurrence of diabetes, while low MetS-IR may be an important factor in promoting NFG recovery.
Table 1. Baseline characteristics summarized according to subjects' glycemic status during follow-up.
Figure 3. Violin chart showing baseline valus of MetS-IR according to glucose status during follow-up. Mets-IR: metabolic score for insulin resistance; NFG: normal fasting glucose.
The estimated association between MetS-IR (as a continuous variable) and the progression or regression of prediabetes from Cox regression models was displayed in Table 2. From four models with different adjustment levels, we found a significant negative correlation between continuous MetS-IR and prediabetes regression, and a positive correlation with prediabetes progression. According to the final model (Model III), each unit increase in MetS-IR resulted in a 26% increased risk of diabetes in prediabetic patients [HR: 1.26, 1.19-1.33], while a decrease in MetS-IR was protective for prediabetic patients (increased probability of returning to NFG), quantified as a 10% protective effect for each unit decrease in MetS-IR [HR: 0.90, 1.19-1.33].
Table 2. Multivariate Cox regression analysis of the role of MetS-IR in assessing changes in glycemic status in patients with prediabetes.
Figures 4 and 5 show the dose-response curves of MetS-IR with the progression or regression of prediabetes. After multivariate adjustment based on Model III, we found a nonlinear correlation between MetS-IR and prediabetes regression (P for non-linearity < 0.001), and a linear correlation with prediabetes progression (P for non-linearity = 0.346). It was observed in the dose-response curve (Figure 5) that the recovery rate to NFG was higher when MetS-IR was lower, significantly dropping when MetS-IR reached a certain value (between 35-40) (P for log likelihood ratio test < 0.001). Using recursive algorithms and segmented regression, the inflection point for MetS-IR related to prediabetes regression was determined to be 37.22 (Table 3), with HRs of 0.95 (0.93-0.96) before and 0.98 (0.97-0.99) after the inflection point.
Figure 4. Apply the 4-knots RCS model to fit the dose-response curve of MetS-IR with the progression of prediabetes. Mets-IR, metabolic score for insulin resistance; RCS, restricted cubic splines.
Figure 5. Apply the 4-knots RCS model to fit the dose-response curve of MetS-IR with the regression of prediabetes. Mets-IR, metabolic score for insulin resistance; RCS, restricted cubic splines; NFG, normal fasting glucose.
Table 3. The threshold effect of MetS-IR on the regression of pre-diabetes to NFG was analyzed by two-piecewise cox regression model.
Several sensitivity analyses were conducted, showing consistent results. First, treating MetS-IR as a categorical variable yielded consistent results (Table 2). Further analyses were repeated with changed inclusion criteria (WHO) and excluding participants with a family history of diabetes, showing no significant change in results (Supplementary Table 3). The Fine-Gray method considering competing risks was used, again yielding consistent results (Supplementary Table 3); finally, to verify the appropriateness of the 4-knot RCS in the current study, additional analyses using 3-knot and 5-knot RCS were performed, with no substantial changes in results (Supplementary Figures 3–6).
As shown in Table 4, within subgroups stratified by gender, age, and BMI, we observed a significant effect of the association between MetS-IR and prediabetes progression only in the age subgroup (P interaction < 0.05). In the age subgroup, compared to participants aged ≥45, those aged <45 had a stronger positive correlation between MetS-IR and prediabetes progression (HR: 1.42 vs 1.19, P-interaction < 0.0001).
Table 4. Exploratory subgroup analysis of the role and differences of MetS-IR in assessing changes in glycemic status in prediabetes patients.
In our study of 15,421 prediabetic subjects, we observed that an increase in the surrogate for IR, MetS-IR, was associated with an increased risk of developing diabetes, while a decrease in MetS-IR was protective in returning to NFG. Additionally, we found a nonlinear relationship between MetS-IR and the regression of prediabetes, with 37.22 identified as the inflection point; prediabetes regression rates were significantly higher before this point and markedly decreased thereafter.
Prediabetes is an early stage of diabetes and various chronic complications (1–4), in which IR is the main pathophysiological feature of the disease. To date, numerous studies have been undertaken globally to evaluate the effects of various intervention strategies on prediabetes, with non-pharmacologic (lifestyle adjustment) as well as pharmacologic methods (magnesium supplements, lipase inhibitor, glucagon-like peptide 1 receptor agonists, fenofibrate, alpha-glucosidase inhibitors, insulin sensitizer and traditional Chinese medicine) considered to have good potential for application (11, 17–21, 42). It is important to note that despite the large number of studies showing the effectiveness of pharmacologic approaches to treating prediabetes, no medications have been approved by regulatory agencies for the treatment of prediabetes, and expert statements only recommend lifestyle interventions as a first-line treatment for prediabetes (42, 43). In this context, it is important to monitor IR in the context of enhanced lifestyle interventions in prediabetic patients. Considering that HEGC, the gold standard for measuring IR, has major limitations, monitoring IR alternatives may have better application and promotion potential. As a new simple IR surrogate indicator, MetS-IR covers blood glucose, blood lipids, and obesity factors (27), and this parameter has been confirmed in recent studies to be the best IR surrogate for assessing diabetes risk in Chinese and Japanese populations (30). Therefore, evaluating the role of MetS-IR in glycemic outcomes among individuals with prediabetes in this study may offer valuable insights for future prevention and treatment strategies.
The association between MetS-IR and diabetes has been supported by evidence from various studies across different ethnicities. Overall, a positive correlation exists between MetS-IR and diabetes, with high MetS-IR identified as a significant risk factor for both the prevalence and incidence of diabetes (27–30, 44, 45), including in baseline non-diabetic subjects, non-obese individuals, and hypertensive patients. In our study, we further confirm the role of high MetS-IR as a risk factor for the development of diabetes; notably, unlike previous studies, our research specifically evaluates subjects with prediabetes. Specifically, among prediabetic participants, each unit increase in MetS-IR is associated with a 26% increase in the risk of developing diabetes within three years. Additionally, it is worth mentioning that recent evidence from a rural cohort study in China suggests that there may be no significant association between MetS-IR and diabetes (46). On reviewing this study, we noted that their sample size (n=1,205) and limited number of diabetes outcomes (n=97) might have affected their findings. When categorizing MetS-IR, each group’s sample size reduces further, which can weaken or nullify associations due to insufficient test power (47). Moreover, the inclusion of similar variables like BMI, waist circumference, and conventional lipids, which often have collinearity, might have confounded their results (48). Combining these findings, we lean towards MetS-IR being a significant predictor of diabetes across various ethnicities.
Another key finding was the negative correlation between MetS-IR and prediabetes regression, suggesting that lowering MetS-IR has a protective role in recovering to NFG of prediabetic individuals. This finding was consistent across multiple sensitivity analyses, confirming its stability and significance for prediabetes intervention. We further explored this relationship by plotting dose-response curves, revealing a nonlinear correlation between MetS-IR and prediabetes regression. The probability of returning to NFG was significantly higher when MetS-IR was lower, particularly below the inflection point of 37.22. To the best of our knowledge, these findings have not been previously reported and therefore could not be analyzed in the current study for comparisons between different races, populations. Considering our main results, maintaining MetS-IR below 37.22 is crucial for prediabetics to return to normal glucose regulation.
Previous reports suggested that prediabetes and diabetes predominantly occur in men, older individuals, and those who are overweight/obese (5, 6, 49, 50). Our stratified analysis by gender, age, and BMI showed similar trends, with higher proportions of men, older individuals, and those overweight/obese among those progressing to diabetes, and higher proportions of women, younger individuals, and non-obese individuals among those regressing from prediabetes (Table 4). However, a potentially contradictory finding was that younger individuals (<45 years) showed a stronger positive correlation between MetS-IR and prediabetes progression (HR: 1.42 vs 1.19, P-interaction < 0.0001) compared to those aged ≥ 45. This suggested that MetS-IR may be particularly effective in assessing diabetes risk in younger populations. The reasons for this unique finding are unknown, but some speculations might help explain it: (i) Overweight/obesity surveys in China from 1989-2011 show a rapid increase in obesity rates among young adults (18-39 years) (51). Given that obesity is a major cause of IR (52), this rapid weight gain could be a significant factor in the increased diabetes risk associated with MetS-IR in young people. (ii) The rapid development of China’s economy in recent decades has brought immense mental and psychological stress, particularly to the working population (mainly younger individuals), exacerbating IR and metabolic disorders (53, 54), thus increasing the diabetes risk associated with MetS-IR in this group. Additionally, the national family planning policy has led to a rapid decline in the workforce (55, 56), potentially impacting these trends.
This study is noteworthy due to the following strengths: (i) Based on the multicenter health examination cohort of Rich Healthcare Group, this study benefits from a large sample size, enabling extensive sensitivity and subgroup analyses, enhancing the robustness of the results. (ii) This analysis is the first to report the association between MetS-IR and the regression of prediabetes, offering valuable insights for prediabetes intervention based on MetS-IR thresholds. Moreover, no previous studies have explored the relationship between MetS-IR and diabetes in a prediabetic population.
However, some inherent limitations must be acknowledged: (i) Participants were primarily from the Chinese population, necessitating further research to ascertain the extent to which these findings are generalizable to other ethnic groups. (ii) Due to the lack of oral glucose tolerance test data and HbA1c measurement data in the original dataset, the diagnosis of outcomes in this study relied solely on FPG, which may lead to some bias in the incidence rates of outcomes; considering the diagnostic shortcomings, current research evidence recommends promotion mainly in patients with impaired fasting glucose. It’s important to note that most prediabetes-related studies diagnose based on FPG (2), as large-scale epidemiological surveys typically involve large sample sizes, with only a small proportion undergoing oral glucose tolerance testing. (iii) This study is a secondary creation based on the multicenter health examination cohort of Rich Healthcare Group, and the covariates included in the regression models were based on available variables in the dataset, inevitably leading to residual confounding from variables not included in the dataset (57). (iv) Although the follow-up period of the current study is substantial (2-7 years), the average follow-up duration remains relatively short; longer follow-up studies are needed to further clarify the impact of MetS-IR on long-term blood glucose changes in prediabetic patients. (v) The current study lacks MetS-IR data during follow-up, necessitating future research to assess the impact of increases/decreases in MetS-IR on the progression/regression of prediabetes. (vi) The current study did not identify people who used antidiabetic drugs for indications other than diabetes, which may have partially affected the results.
In conclusion, our study provides new evidence from a general population that an increase in MetS-IR may lead to an increased risk of diabetes in individuals with prediabetes; similarly, a decrease in MetS-IR enhances the protective effect for returning to NFG.
The datasets presented in this study can be found in online repositories. The names of the repository/repositories and accession number(s) can be found in the article/Supplementary Material.
The studies involving humans were approved by the Institutional Ethics Committee of Jiangxi Provincial People’s Hospital. The studies were conducted in accordance with the local legislation and institutional requirements. The ethics committee/institutional review board waived the requirement of written informed consent for participation from the participants or the participants’ legal guardians/next of kin because in accordance with local laws and regulations, the Institutional Ethics Committee of Jiangxi Provincial People’s Hospital, after reviewing the design of the current study and the anonymized research data set, authorized the implementation of the current study and exempted the subjects from signing informed consent forms. The current study was conducted in line with the Declaration of Helsinki and adhered to the STROBE reporting guidelines.
GS: Writing – original draft, Validation, Data curation. MK: Writing – original draft, Validation, Software, Formal analysis, Data curation. RY: Writing – original draft, Software, Formal analysis. YZ: Writing – review & editing, Supervision, Project administration, Methodology, Conceptualization.
The author(s) declare financial support was received for the research, authorship, and/or publication of this article. This work was supported by Natural Science Foundation of Jiangxi Province (No.20232BAB216004 to YZ).
We would like to thank Dr. Chen Ying and his team members for their great efforts in data collection and organization.
The authors declare that the research was conducted in the absence of any commercial or financial relationships that could be construed as a potential conflict of interest.
All claims expressed in this article are solely those of the authors and do not necessarily represent those of their affiliated organizations, or those of the publisher, the editors and the reviewers. Any product that may be evaluated in this article, or claim that may be made by its manufacturer, is not guaranteed or endorsed by the publisher.
The Supplementary Material for this article can be found online at: https://www.frontiersin.org/articles/10.3389/fendo.2024.1388751/full#supplementary-material
1. Echouffo-Tcheugui JB, Perreault L, Ji L, Dagogo-Jack S. Diagnosis and management of prediabetes: A review. JAMA. (2023) 329:1206–16. doi: 10.1001/jama.2023.4063
PubMed Abstract | PubMed Abstract | Crossref Full Text | Google Scholar
2. Rooney MR, Fang M, Ogurtsova K, Ozkan B, Echouffo-Tcheugui JB, Boyko EJ, et al. Global prevalence of prediabetes. Diabetes Care. (2023) 46:1388–94. doi: 10.2337/dc22-2376
PubMed Abstract | PubMed Abstract | Crossref Full Text | Google Scholar
3. Khetan AK, Rajagopalan S. Prediabetes. Can J Cardiol. (2018) 34:615–23. doi: 10.1016/j.cjca.2017.12.030
PubMed Abstract | PubMed Abstract | Crossref Full Text | Google Scholar
4. Ferrannini E, Gastaldelli A, Iozzo P. Pathophysiology of prediabetes. Med Clin North Am. (2011) 95:327–39. doi: 10.1016/j.mcna.2010.11.005
PubMed Abstract | PubMed Abstract | Crossref Full Text | Google Scholar
5. Beulens J, Rutters F, Rydén L, Schnell O, Mellbin L, Hart HE, et al. Risk and management of pre-diabetes. Eur J Prev Cardiol. (2019) 26:47–54. doi: 10.1177/2047487319880041
PubMed Abstract | PubMed Abstract | Crossref Full Text | Google Scholar
6. Tabák AG, Herder C, Rathmann W, Brunner EJ, Kivimäki M. Prediabetes: a high-risk state for diabetes development. Lancet. (2012) 379:2279–90. doi: 10.1016/S0140-6736(12)60283-9
PubMed Abstract | PubMed Abstract | Crossref Full Text | Google Scholar
7. Cai X, Zhang Y, Li M, Wu JH, Mai L, Li J, et al. Association between prediabetes and risk of all cause mortality and cardiovascular disease: updated meta-analysis. BMJ. (2020) 370:m2297. doi: 10.1136/bmj.m2297
PubMed Abstract | PubMed Abstract | Crossref Full Text | Google Scholar
8. Lindsay C, Shieh A. Prediabetes and skeletal health. Curr Opin Endocrinol Diabetes Obes. (2023) 30:200–5. doi: 10.1097/MED.0000000000000812
PubMed Abstract | PubMed Abstract | Crossref Full Text | Google Scholar
9. Roriz-Filho J S, Sá-Roriz TM, Rosset I, Camozzato AL, Santos AC, Chaves ML, et al. (Pre)diabetes, brain aging, and cognition. Biochim Biophys Acta. (2009) 1792:432–43. doi: 10.1016/j.bbadis.2008.12.003
PubMed Abstract | PubMed Abstract | Crossref Full Text | Google Scholar
10. Chen Y, Zhang P, Wang J, Gong Q, An Y, Qian X, et al. Associations of progression to diabetes and regression to normal glucose tolerance with development of cardiovascular and microvascular disease among people with impaired glucose tolerance: a secondary analysis of the 30 year Da Qing Diabetes Prevention Outcome Study. Diabetologia. (2021) 64:1279–87. doi: 10.1007/s00125-021-05401-x
PubMed Abstract | PubMed Abstract | Crossref Full Text | Google Scholar
11. Tuomilehto J, Lindström J, Eriksson JG, Valle TT, Hämäläinen H, Ilanne-Parikka P, et al. Prevention of type 2 diabetes mellitus by changes in lifestyle among subjects with impaired glucose tolerance. N Engl J Med. (2001) 344:1343–50. doi: 10.1056/NEJM200105033441801
PubMed Abstract | PubMed Abstract | Crossref Full Text | Google Scholar
12. Pratte KA, Johnson A, Beals J, Bullock A, Manson SM, Jiang L, et al. Regression to normal glucose regulation in American Indians and Alaska natives of a diabetes prevention program. Diabetes Care. (2019) 42:1209–16. doi: 10.2337/dc18-1964
PubMed Abstract | PubMed Abstract | Crossref Full Text | Google Scholar
13. Vistisen D, Kivimäki M, Perreault L, Hulman A, Witte DR, Brunner EJ, et al. Reversion from prediabetes to normoglycaemia and risk of cardiovascular disease and mortality: the Whitehall II cohort study. Diabetologia. (2019) 62:1385–90. doi: 10.1007/s00125-019-4895-0
PubMed Abstract | PubMed Abstract | Crossref Full Text | Google Scholar
14. Perreault L, Pan Q, Mather KJ, Watson KE, Hamman RF, Kahn SE, et al. Effect of regression from prediabetes to normal glucose regulation on long-term reduction in diabetes risk: results from the Diabetes Prevention Program Outcomes Study. Lancet. (2012) 379:2243–51. doi: 10.1016/S0140-6736(12)60525-X
PubMed Abstract | PubMed Abstract | Crossref Full Text | Google Scholar
15. Perreault L, Pan Q, Schroeder EB, Kalyani RR, Bray GA, Dagogo-Jack S, et al. Regression from prediabetes to normal glucose regulation and prevalence of microvascular disease in the diabetes prevention program outcomes study (DPPOS). Diabetes Care. (2019) 42:1809–15. doi: 10.2337/dc19-0244
PubMed Abstract | PubMed Abstract | Crossref Full Text | Google Scholar
16. Perreault L, Temprosa M, Mather KJ, Horton E, Kitabchi A, Larkin M, et al. Regression from prediabetes to normal glucose regulation is associated with reduction in cardiovascular risk: results from the Diabetes Prevention Program outcomes study. Diabetes Care. (2014) 37:2622–31. doi: 10.2337/dc14-0656
PubMed Abstract | PubMed Abstract | Crossref Full Text | Google Scholar
17. Chiasson JL, Josse RG, Gomis R, Hanefeld M, Karasik A, Laakso M, et al. Acarbose for prevention of type 2 diabetes mellitus: the STOP-NIDDM randomised trial. Lancet. (2002) 359:2072–7. doi: 10.1016/S0140-6736(02)08905-5
PubMed Abstract | PubMed Abstract | Crossref Full Text | Google Scholar
18. Amer OE, Sabico S, Alfawaz HA, Aljohani N, Hussain SD, Alnaami AM, et al. Reversal of prediabetes in Saudi adults: results from an 18 month lifestyle intervention. Nutrients. (2020) 12:804. doi: 10.3390/nu12030804
PubMed Abstract | PubMed Abstract | Crossref Full Text | Google Scholar
19. Knowler WC, Barrett-Connor E, Fowler SE, Hamman RF, Lachin JM, Walker EA, et al. Reduction in the incidence of type 2 diabetes with lifestyle intervention or metformin. N Engl J Med. (2002) 346:393–403. doi: 10.1056/NEJMoa012512
PubMed Abstract | PubMed Abstract | Crossref Full Text | Google Scholar
20. Ramachandran A, Snehalatha C, Mary S, Selvam S, Kumar CK, Seeli AC, et al. Pioglitazone does not enhance the effectiveness of lifestyle modification in preventing conversion of impaired glucose tolerance to diabetes in Asian Indians: results of the Indian Diabetes Prevention Programme-2 (IDPP-2). Diabetologia. (2009) 52:1019–26. doi: 10.1007/s00125-009-1315-x
PubMed Abstract | PubMed Abstract | Crossref Full Text | Google Scholar
21. Eriksson KF, Lindgärde F. Prevention of type 2 (non-insulin-dependent) diabetes mellitus by diet and physical exercise. 6-year Malmö feasibility study. Diabetologia. (1991) 34:891–8. doi: 10.1007/BF00400196
22. Snehalatha C, Mary S, Selvam S, Sathish Kumar CK, Shetty SB, Nanditha A, et al. Changes in insulin secretion and insulin sensitivity in relation to the glycemic outcomes in subjects with impaired glucose tolerance in the Indian Diabetes Prevention Programme-1 (IDPP-1). Diabetes Care. (2009) 32:1796–801. doi: 10.2337/dc09-0676
PubMed Abstract | PubMed Abstract | Crossref Full Text | Google Scholar
23. Magkos F, Lee MH, Lim M, Cook AR, Chhay V, Loh TP, et al. Dynamic assessment of insulin secretion and insulin resistance in Asians with prediabetes. Metabolism. (2022) 128:154957. doi: 10.1016/j.metabol.2021.154957
PubMed Abstract | PubMed Abstract | Crossref Full Text | Google Scholar
24. Bi Y, Zhu D, Jing Y, Hu Y, Feng W, Shen S, et al. Decreased beta cell function and insulin sensitivity contributed to increasing fasting glucose in Chinese. Acta Diabetol. (2012) 49 Suppl 1:S51–8. doi: 10.1007/s00592-010-0194-4
PubMed Abstract | PubMed Abstract | Crossref Full Text | Google Scholar
25. DeFronzo RA, Tobin JD, Andres R. Glucose clamp technique: a method for quantifying insulin secretion and resistance. Am J Physiol. (1979) 237:E214–23. doi: 10.1152/ajpendo.1979.237.3.E214
PubMed Abstract | PubMed Abstract | Crossref Full Text | Google Scholar
26. Muniyappa R, Lee S, Chen H, Quon MJ. Current approaches for assessing insulin sensitivity and resistance in vivo: advantages, limitations, and appropriate usage. Am J Physiol Endocrinol Metab. (2008) 294:E15–26. doi: 10.1152/ajpendo.00645.2007
PubMed Abstract | PubMed Abstract | Crossref Full Text | Google Scholar
27. Bello-Chavolla OY, Almeda-Valdes P, Gomez-Velasco D, Viveros-Ruiz T, Cruz-Bautista I, Romo-Romo A, et al. METS-IR, a novel score to evaluate insulin sensitivity, is predictive of visceral adiposity and incident type 2 diabetes. Eur J Endocrinol. (2018) 178:533–44. doi: 10.1530/EJE-17-0883
PubMed Abstract | PubMed Abstract | Crossref Full Text | Google Scholar
28. Zhang M, Liu D, Qin P, Liu Y, Sun X, Li H, et al. Association of metabolic score for insulin resistance and its 6-year change with incident type 2 diabetes mellitus. J Diabetes. (2021) 13:725–34. doi: 10.1111/1753-0407.13161
PubMed Abstract | PubMed Abstract | Crossref Full Text | Google Scholar
29. Cai XT, Zhu Q, Liu SS, Wang MR, Wu T, Hong J, et al. Associations between the metabolic score for insulin resistance index and the risk of type 2 diabetes mellitus among non-obese adults: insights from a population-based cohort study. Int J Gen Med. (2021) 14:7729–40. doi: 10.2147/IJGM.S336990
PubMed Abstract | PubMed Abstract | Crossref Full Text | Google Scholar
30. Shangguan Q, Liu Q, Yang R, Zhang S, Sheng G, Kuang M, et al. Predictive value of insulin resistance surrogates for the development of diabetes in individuals with baseline normoglycemia: findings from two independent cohort studies in China and Japan. Diabetol Metab Syndr. (2024) 16:68. doi: 10.1186/s13098-024-01307-x
PubMed Abstract | PubMed Abstract | Crossref Full Text | Google Scholar
31. Chen Y, Zhang XP, Yuan J, Cai B, Wang XL, Wu XL, et al. Data from: Association of body mass index and age with incident diabetes in Chinese adults: a population-based cohort study. Dryad Dataset. (2018). doi: 10.5061/dryad.ft8750v
32. Chen Y, Zhang XP, Yuan J, Cai B, Wang XL, Wu XL, et al. Association of body mass index and age with incident diabetes in Chinese adults: a population-based cohort study. BMJ Open. (2018) 8:e021768. doi: 10.1136/bmjopen-2018-021768
PubMed Abstract | PubMed Abstract | Crossref Full Text | Google Scholar
34. Mugler EM, Tate MC, Livescu K, Templer JW, Goldrick MA, Slutzky MW. Differential representation of articulatory gestures and phonemes in precentral and inferior frontal gyri. J Neurosci. (2018) 38:9803–13. doi: 10.1523/JNEUROSCI.1206-18.2018
PubMed Abstract | PubMed Abstract | Crossref Full Text | Google Scholar
35. Gharavi E, Gu A, Zheng G, Smith JP, Cho HJ, Zhang A, et al. Embeddings of genomic region sets capture rich biological associations in lower dimensions. Bioinformatics. (2021) 37:4299–306. doi: 10.1093/bioinformatics/btab439
PubMed Abstract | PubMed Abstract | Crossref Full Text | Google Scholar
36. Schoenfeld D. Partial residuals for the proportional hazards regression-model. Biometrika. (1982) 69:239–41. doi: 10.1093/biomet/69.1.239
37. Wax Y. Collinearity diagnosis for a relative risk regression analysis: an application to assessment of diet-cancer relationship in epidemiological studies. Stat Med. (1992) 11:1273–87. doi: 10.1002/sim.4780111003
PubMed Abstract | PubMed Abstract | Crossref Full Text | Google Scholar
38. Alberti KG, Zimmet PZ. Definition, diagnosis and classification of diabetes mellitus and its complications. Part 1: Diagnosis and classification of diabetes mellitus provisional report of a WHO consultation. Diabetes Med. (1998) 15:539–53. doi: 10.1002/(SICI)1096-9136(199807)15:7<539::AID-DIA668>3.0.CO;2-S
39. Zhao Y, Song C, Ma X, Ma X, Wang Q, Ji H, et al. Synergistic effect of family history of diabetes and dietary habits on the risk of type 2 diabetes in central China. Int J Endocrinol. (2017) 2017:9707284. doi: 10.1155/2017/9707284
PubMed Abstract | PubMed Abstract | Crossref Full Text | Google Scholar
41. Zhou BF, Cooperative Meta-Analysis Group of the Working Group on Obesity in China. Predictive values of body mass index and waist circumference for risk factors of certain related diseases in Chinese adults–study on optimal cut-off points of body mass index and waist circumference in Chinese adults. BioMed Environ Sci. (2002) 15:83–96.
42. Galaviz KI, Weber MB, Suvada K BS, Gujral UP, Wei J, Merchant R, et al. Interventions for reversing prediabetes: A systematic review and meta-analysis. Am J Prev Med. (2022) 62:614–25. doi: 10.1016/j.amepre.2021.10.020
PubMed Abstract | PubMed Abstract | Crossref Full Text | Google Scholar
43. Boltri JM, Tracer H, Strogatz D, Idzik S, Schumacher P, Fukagawa N, et al. The national clinical care commission report to congress: leveraging federal policies and programs to prevent diabetes in people with prediabetes. Diabetes Care. (2023) 46:e39–50. doi: 10.2337/dc22-0620
PubMed Abstract | PubMed Abstract | Crossref Full Text | Google Scholar
44. Wang T, Yu C, Yu GT, Zhou W, Zhu LJ, Huang X, et al. Predictive effects of different insulin resistance indexes on diabetes risk in hypertensive population. Zhongguo Yi Xue Ke Xue Yuan Xue Bao. (2023) 45:206–12. doi: 10.3881/j.issn.1000-503X.15127
PubMed Abstract | PubMed Abstract | Crossref Full Text | Google Scholar
45. Chen Z, Huang C, Zhou Z, Zhang Y, Xu M, Tang Y, et al. A nonlinear associations of metabolic score for insulin resistance index with incident diabetes: A retrospective Chinese cohort study. Front Clin Diabetes Healthc. (2023) 3:1101276. doi: 10.3389/fcdhc.2022.1101276
PubMed Abstract | PubMed Abstract | Crossref Full Text | Google Scholar
46. Li X, Xue Y, Dang Y, Liu W, Wang Q, Zhao Y, et al. Association of non-insulin-based insulin resistance indices with risk of incident prediabetes and diabetes in a Chinese rural population: A 12-year prospective study. Diabetes Metab Syndr Obes. (2022) 15:3809–19. doi: 10.2147/DMSO.S385906
PubMed Abstract | PubMed Abstract | Crossref Full Text | Google Scholar
47. Devane D, Begley CM, Clarke M. How many do I need? Basic principles of sample size estimation. J Adv Nurs. (2004) 47:297–302. doi: 10.1111/j.1365-2648.2004.03093.x
PubMed Abstract | PubMed Abstract | Crossref Full Text | Google Scholar
48. Kim JH. Multicollinearity and misleading statistical results. Korean J Anesthesiol. (2019) 72:558–69. doi: 10.4097/kja.19087
PubMed Abstract | PubMed Abstract | Crossref Full Text | Google Scholar
49. Ciarambino T, Crispino P, Leto G, Mastrolorenzo E, Para O, Giordano M. Influence of gender in diabetes mellitus and its complication. Int J Mol Sci. (2022) 23:8850. doi: 10.3390/ijms23168850
PubMed Abstract | PubMed Abstract | Crossref Full Text | Google Scholar
50. Maggio CA, Pi-Sunyer FX. Obesity and type 2 diabetes. Endocrinol Metab Clin North Am. (2003) 32:805–22. doi: 10.1016/s0889-8529(03)00071-9
PubMed Abstract | PubMed Abstract | Crossref Full Text | Google Scholar
51. Chen Y, Peng Q, Yang Y, Zheng S, Wang Y, Lu W. The prevalence and increasing trends of overweight, general obesity, and abdominal obesity among Chinese adults: a repeated cross-sectional study. BMC Public Health. (2019) 19:1293. doi: 10.1186/s12889-019-7633-0
PubMed Abstract | PubMed Abstract | Crossref Full Text | Google Scholar
52. Tong Y, Xu S, Huang L, Chen C. Obesity and insulin resistance: Pathophysiology and treatment. Drug Discovery Today. (2022) 27:822–30. doi: 10.1016/j.drudis.2021.11.001
PubMed Abstract | PubMed Abstract | Crossref Full Text | Google Scholar
53. Motoyama S, Yamada H, Yamamoto K, Wakana N, Terada K, Kikai M, et al. Social stress increases vulnerability to high-fat diet-induced insulin resistance by enhancing neutrophil elastase activity in adipose tissue. Cells. (2020) 9:996. doi: 10.3390/cells9040996
PubMed Abstract | PubMed Abstract | Crossref Full Text | Google Scholar
54. Sanghez V, Razzoli M, Carobbio S, Campbell M, McCallum J, Cero C, et al. Psychosocial stress induces hyperphagia and exacerbates diet-induced insulin resistance and the manifestations of the Metabolic Syndrome. Psychoneuroendocrinology. (2013) 38:2933–42. doi: 10.1016/j.psyneuen.2013.07.022
PubMed Abstract | PubMed Abstract | Crossref Full Text | Google Scholar
55. Eriksson M, Ng N. Changes in access to structural social capital and its influence on self-rated health over time for middle-aged men and women: a longitudinal study from northern Sweden. Soc Sci Med. (2015) 130:250–8. doi: 10.1016/j.socscimed.2015.02.029
PubMed Abstract | PubMed Abstract | Crossref Full Text | Google Scholar
56. Wang F. Can China afford to continue its one-child policy? Asia Pacific Issues. (2005) 17. http://hdl.handle.net/10125/3796.
57. Black N. Why we need observational studies to evaluate the effectiveness of health care. BMJ. (1996) 312:1215–8. doi: 10.1136/bmj.312.7040.1215
PubMed Abstract | PubMed Abstract | Crossref Full Text | Google Scholar
Keywords: diabetes, metabolic score for insulin resistance, progression of prediabetes, regression of prediabetes, Chinese
Citation: Sheng G, Kuang M, Yang R and Zou Y (2024) Association of metabolic score for insulin resistance with progression or regression of prediabetes: evidence from a multicenter Chinese medical examination cohort study. Front. Endocrinol. 15:1388751. doi: 10.3389/fendo.2024.1388751
Received: 20 February 2024; Accepted: 23 October 2024;
Published: 11 November 2024.
Edited by:
Åke Sjöholm, Gävle Hospital, SwedenReviewed by:
Alina Kurylowicz, Polish Academy of Sciences, PolandCopyright © 2024 Sheng, Kuang, Yang and Zou. This is an open-access article distributed under the terms of the Creative Commons Attribution License (CC BY). The use, distribution or reproduction in other forums is permitted, provided the original author(s) and the copyright owner(s) are credited and that the original publication in this journal is cited, in accordance with accepted academic practice. No use, distribution or reproduction is permitted which does not comply with these terms.
*Correspondence: Yang Zou, anh5eHl6eUAxNjMuY29t
Disclaimer: All claims expressed in this article are solely those of the authors and do not necessarily represent those of their affiliated organizations, or those of the publisher, the editors and the reviewers. Any product that may be evaluated in this article or claim that may be made by its manufacturer is not guaranteed or endorsed by the publisher.
Research integrity at Frontiers
Learn more about the work of our research integrity team to safeguard the quality of each article we publish.