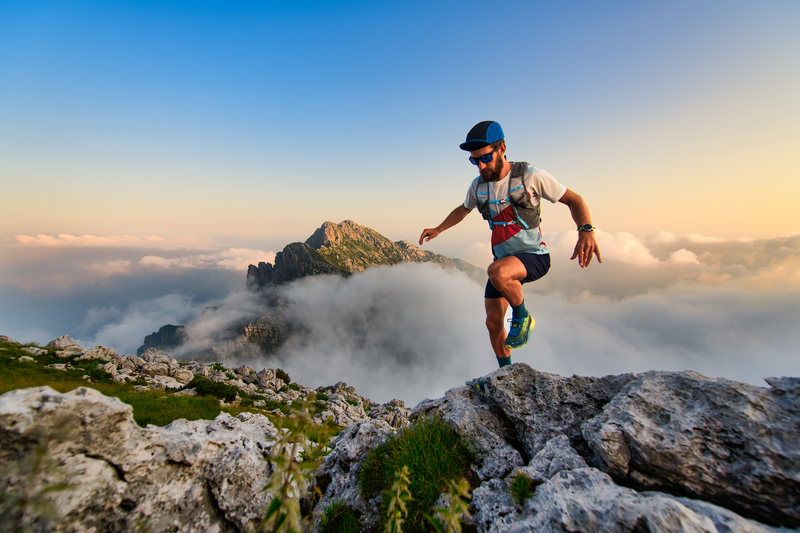
95% of researchers rate our articles as excellent or good
Learn more about the work of our research integrity team to safeguard the quality of each article we publish.
Find out more
ORIGINAL RESEARCH article
Front. Endocrinol. , 29 April 2024
Sec. Clinical Diabetes
Volume 15 - 2024 | https://doi.org/10.3389/fendo.2024.1386639
This article is part of the Research Topic Novel Insights into the Pathophysiology of Diabetes-related Complications: Implications for Improved Therapeutic Strategies, Volume II View all 37 articles
Background: Increasing evidence emphasizes the potential relationship between diabetes and OAB (overactive bladder). However, large population epidemiology is still lacking.
Methods: This cross-sectional study included six cycle NHANES surveys, with a total of 23863 participants. Logistic regression models were constructed to analyze the association between diabetes mellitus, diabetes-related markers, and inflammatory biomarkers with OAB. Restricted cubic splines were used to analyze the non-linear associations. Mediating analysis was performed to test the effect of inflammatory biomarkers on the relationship between diabetes-related markers and OAB. Finally, machine learning models were applied to predict the relative importance and construct the best-fit model.
Results: Diabetes mellitus participants’ OAB prevalence increased by 77% compared with non-diabetes. As the quartiles of diabetes-related markers increased, the odds of OAB monotonically increased in three models (all p for trend < 0.001). Glycohemoglobin exhibited a linear association with OAB (p for nonlinearity > 0.05). White blood cells significantly mediated the associations between diabetes-related markers (glycohemoglobin, fasting glucose, and insulin) with OAB, and the proportions were 7.23%, 8.08%, and 17.74%, respectively (all p < 0.0001). Neutrophils partly mediated the correlation between (glycohemoglobin, fasting glucose, and insulin) and OAB at 6.58%, 9.64%, and 17.93%, respectively (all p < 0.0001). Machine learning of the XGBoost model constructs the best fit model, and XGBoost predicts glycohemoglobin is the most important indicator on OAB.
Conclusion: Our research revealed diabetes mellitus and diabetes-related markers were remarkably associated with OAB, and systemic inflammation was an important mediator of this association.
Overactive bladder (OAB) is a syndrome characterized by urinary urgency, often accompanied by frequency and nocturia, and may or may not be accompanied by urgent urinary incontinence, which negatively affects the quality of life of patients and their interactions with society (1, 2). There are several theories regarding the pathophysiology of OAB, including the myogenic hypothesis, the urotheliogenic hypothesis, the supraspinal hypothesis, metabolic syndrome, and urinary microbiota involvement, but a full explanation is still missing (3).
As one of the main components of the metabolic syndrome, diabetes mellitus (DM) has profound effects on multiple organ systems, including the kidney and bladder (4, 5). Diabetic cystopathy, also known as diabetic bladder dysfunction (DBD), is a common urological complication whose modern definition encompasses overactive bladder, voiding dysfunction, and urinary retention (6). Some studies estimated that the prevalence rate of diabetic cystopathy was highly varied, ranging from 25% to 90% (7, 8). Several epidemiological studies have shown that OAB is more common in patients with type 2 DM than in the general population, including women with DM treated with insulin and diabetic children aged 11–17 (9–11). Chiu A.F. et al. reported that higher glycosylated hemoglobin levels were independent predictors of OAB symptoms in DM patients, and a study of 36,560 OAB patients in the US found that patients with DM are more persistent and adherent to OAB medications (12, 13). Although it is known that overactive bladder is more common in patients with diabetes, the mechanism by which type 2 diabetes may lead to its development is still unclear (14).
Recent studies have shown that the vicious cycle of chronic inflammation and related stresses is associated with several diabetic complications, including atherosclerosis, nephropathy, and cystopathy, which have been demonstrated in both human and animal experiments (15–17). The pathophysiology of OAB is not well understood; however, chronic systemic inflammation and bladder urothelial inflammation, including some inflammatory proteins and cytokines, may contribute to the onset of OAB (15, 18). An increasing number of studies suggest that DBD and OAB are slow-onset and slow-progressing complications, and among them, inflammatory factors such as IL-1β and NLRP3 play an important role (19, 20). Collectively, based on the importance of systemic inflammation for diabetes mellitus and OAB, respectively, we hypothesized that diabetes mellitus may increase OAB risk by promoting systemic inflammation.
Hence, we conducted a cross-sectional study to investigate the associations of diabetes mellitus with OAB risk based on the National Health and Nutrition Examination Survey (NHANES) 2007–2018. Further, we measured systemic inflammation status from multiple perspectives and explored the mediated effects of systemic inflammation.
In order to evaluate the health and nutritional status of adults and children in the United States, the National Center for Health Statistics (NCHS) and the Centers for Disease Control and Prevention (CDC) conduct the NHANES research program. Data from the NHANES survey was accessible to the general public via the official website (https://www.cdc.gov/nchs/nhanes/about_nhanes.htm). The NHANES methods were approved by the Centers for Disease Control and Prevention’s National Center for Health Statistics, and informed permission was given by each participant. Cross-sectional data from six consecutive NHANES iterations, conducted between 2007 and 2018, were included into our study. Five iterations in a row with 59842 participants. Initially, 29814 people were removed from the sample as a whole because their OAB assessments were lacking. Next, we removed the individuals (n = 241) who were pregnant from the NHANES questionnaire data. Finally, we eliminated the individuals who lacked information on their poverty income ratio (n = 2252), sedentary time (n = 31), and education level (n = 3651). A total of 23863 individuals were kept for further analysis after these patients were eliminated (Figure 1).
(1) Fasting plasma glucose levels between 6.1 and 6.9 mmol/L (2); glycohemoglobin (HbA1c) levels between 6.0% and 6.4%; and (3) self-reported physician diagnosis of prediabetes are the diagnostic criteria for borderline diabetes (prediabetes). Diabetes mellitus is defined as follows (1): plasma glucose level of 7.0 mmol/L while fasting (2); blood glucose level of 11.11 mmol/L after a 2-hour oral glucose tolerance test (3); glycohemoglobin (HbA1c) of 6.5%; and (4) self-reported medical diagnosis of diabetes (21). Serum insulin, fasting glucose, and glycohemoglobin were among the diabetes-related indicators that were derived from NHANES laboratory data.
We assessed overactive bladder symptoms in the NHANES population using the NHANES Kidney Conditions-Urology questionnaire, which covered urge urine incontinence and nocturia, based on the Overactive Bladder Symptom Score [OABSS], which was presented in Supplementary Table S2 (22). The person who had a total score of ≥3 was determined to have overactive bladder disorder.
Covariates were gathered via questionnaire, laboratory, and demographic data. Using a standardized questionnaire, we have incorporated variables such as age, sex, educational attainment, race, poverty income ratio (PIR), alcohol intake, smoking status, sedentary time, hypertension, congestive heart failure, coronary heart disease, angina, heart attack, and stroke history. The NHANES website provides descriptions of each of these variables. Three categories were used to categorize educational levels: below high school, high school, and above high school. A person was considered a smoker if they had smoked 100 cigarettes or more in their lifetime. Anyone who drank alcohol in excess of 12 times in a single year was considered an alcohol user. Weight in kilograms (kg) divided by height in meters squared (m2) yielded the BMI. Through participant interviews, the amount of time spent in sedentary activity on a normal day was determined. Through laboratory examination, the number of white blood cells, neutrophils, lymphocytes, and platelets in blood samples were determined. We also identified the diabetes controlling status from “DIQ280 - What was your last A1C level and DIQ290 What does Dr say A1C should be”. If DIQ280 higher than DIQ290, we identified diabetes controlling status was not good.
The complex sampling methodology of the NHANES meant that sample weights, clustering, and stratification were all taken into consideration in the data analysis. Using multivariable logistic regression models, we assessed the associations between diabetes, diabetes-related indicators, inflammatory markers, and overactive bladder. Model 1 was not adjusted. Model 2 was adjusted for age, sex, race, education level, poverty income ratio. Model 3 was adjusted for age, sex, race, educational level, poverty income ratio, BMI, smoking, alcohol use, sedentary time, hypertension, congestive heart failure, coronary heart failure, angina, heart attack, stroke. Restricted cubic splines were used to analyze the non-linear associations. Linear regression models were performed to analyze the relationship of diabetes related markers and inflammatory biomarkers. The association between inflammatory biomarkers and diabetes-related indicators was examined using linear regression models. For causal mediation studies, we estimated the direct effect (DE), indirect effect (IE), and total effect (TE) using the R package called “mediation” (23). There should be a connection between the mediator and the result and the exposure (24). The OAB prediction model was constructed using Python software (version 3.7). The xgboost package, adaboost package, lightgbm package, decision tree package, and MLP package execute different machine learning algorithms while modeling different machine learning methods. Subgroup analysis was conducted to analyze the prevalence differ socioeconomics including age, gender, races, education and poverty income ratio (PIR). Last, we conducted the sensitivity analysis using multiple interpolation to supplement the socioeconomic status. We have multiple interpolated the socioeconomic status including poverty income ratio (n = 2252), sedentary time (n = 31), and education level (n = 3651) and to validate the research results. All statistical analyses were performed using R software (version 4.0.1). Significance was set at p < 0.05 (two-sided).
Table 1 summarizes the detailed baseline characteristics of participants by OAB, and Supplementary Table S1 illustrates the participants with survey-weighted descriptive statistics. A total of 23,863 participants were included in our research, with a median age of 49.7 ± 17.6 years. Among them, 4894 participants were OAB participants, and 18969 were non-OAB participants. We found inflammatory markers, including white blood cells, neutrophil count, and platelet count, had different distributions between OAB participants and non-OAB participants. OAB participants had a higher white blood cell count and neutrophil count. Moreover, OAB participants had higher levels of diabetes-related markers, including glycohemoglobin, fasting glucose, and insulin.
Figure 2 reveals the association between diabetes mellitus and OAB. We identified diabetes mellitus as no-diabetes, borderline, and diabetes. Compared with non-diabetes participants, borderline diabetes participants’ OAB prevalence increased by 26% after adjusting for all covariates (Model 3: OR = 1.26; 95% CI: 1.03, 1.53). Diabetes mellitus participants’ OAB prevalence increased 77% compared with non-diabetes participants in the full adjusted model (Model 3: OR = 1.77; 95% CI: 1.62, 1.94).
Figure 2 Forest plots illustrating the correlation between diabetes mellitus and overactive bladder. Model 1 was not adjusted. Model 2 was adjusted for age, sex, race, education level, poverty income ratio. Model 3 was adjusted for age, sex, race, educational level, poverty income ratio, BMI, smoking, alcohol use, sedentary time, hypertension, congestive heart failure, coronary heart failure, angina, heart attack, stroke.
Table 2 shows the associations of diabetes-related markers with OAB in adults using multiple logistic regression analysis. We found the log10-transformed exposures, including glycohemoglobin, fasting glucose, and insulin, had a significant positive association with OAB. As the quartiles of diabetes-related markers increased, the odds of OAB monotonically increased in three models (all p for trend <0.01). In addition, the cubic spline curves revealed non-linear associations between diabetes-related markers and overactive bladder (Figure 3). Glycohemoglobin exhibited a linear association with OAB (p for nonlinearity >0.05), while associations between fasting glucose, insulin, and OAB were nonlinearity curves (p for nonlinearity < 0.05).
Table 2 Multiple logistic regression associations of diabetes related markers with overactive bladder in adults.
Figure 3 The non-linear associations between diabetes related markers and overactive bladder by restricted cubic splines. (A) The association between glycohemoglobin and overactive bladder; (B) The association fasting glucose and overactive bladder; (C) The association insulin and overactive bladder; Model was adjusted for age, sex, race, educational level, poverty income ratio, BMI, smoking, alcohol use, sedentary time, hypertension, congestive heart failure, coronary heart failure, angina, heart attack, stroke.
As shown in Table 3, we found significant correlations between inflammatory markers (white blood cell count, neutrophil count, lymphocyte count, and platelet count) and OAB. In the multiple linear regression analysis, we found log10-transformed white blood cell count and neutrophil count were significantly associated with OAB (all p < 0.001). As the quartiles of white blood cell count and neutrophil count increased, the odds of OAB monotonically increased in three models (p for trend <0.001). Restricted cubic splines reveal the non-linear associations between inflammatory markers and OAB. Four inflammatory markers exhibited a non-linear association with OAB with a statistical difference (Supplementary Figure S1).
Table 3 Multiple linear regression associations of inflammatory markers with overactive bladder in adults.
From the multiple linear regression analysis, we found a significant correlation between the diabetes-related markers (glycohemoglobin, fasting glucose, and insulin) and inflammatory markers (white blood cells, neutrophils, lymphocytes, and platelets), which is shown in Table 4. Diabetes-related markers all showed a positive association with inflammatory markers, with statistical differences. Among them, we found glycohemoglobin to be the most significantly correlated with inflammatory markers. Glycohemoglobin was linked to higher WBC (β = 4.67; 95% CI: 3.76, 5.58), neutrophil (β = 2.56; 95% CI: 2.11, 3.01), lymphocyte (β = 1.80; 95% CI: 1.11, 2.49), and platelet counts (β = 105.68; 95% CI: 89.89, 121.47).
Table 4 Multiple linear regression associations of inflammatory markers with diabetes related markers in adults.
Mediation analysis was used to evaluate the mediation effect between the diabetes-related markers and OAB by systemic inflammation (Supplementary Table S3). We found the white blood cells significantly mediated the associations between diabetes-related markers (glycohemoglobin, fasting glucose, and insulin) and OAB, and the proportions were 7.23%, 8.08%, and 17.74%, respectively. (all p < 0.0001). Similarly, neutrophils partly mediated the correlation between glycohemoglobin, fasting glucose, and insulin with OAB at 6.58%, 9.64%, and 17.93%, respectively (all p < 0.0001) (Figure 4). There were no significant mediation effects observed in the associations of diabetes-related markers with overactive bladder by lymphocyte or platelet (all p > 0.05).
Figure 4 Estimated proportion of the association between diabetes related markers and overactive bladder mediated by inflammatory markers. (A) Glycohemoglobin mediated by White blood cell; (B) Glycohemoglobin mediated by Neutrophils; (C) Fasting glucose mediated by White blood cell; (D) Fasting glucose mediated by Neutrophils; (E) Insulin mediated by White blood cell; (F) Insulin mediated by Neutrophils. Models were adjusted for age, sex, race, educational level, poverty income ratio, BMI, smoking, alcohol use, sedentary time, hypertension, congestive heart failure, coronary heart failure, angina, heart attack, stroke. IE, indirect effect; DE, direct effect; Proportion of mediation = IE / (DE + IE); ***P < 0.001.
As shown in Figure 5, we used machine learning in the XGBoost model to determine the relative significance of the diabetes-related markers and inflammatory markers in OAB. We observed that glycohemoglobin was the most important indicator in determining OAB. The forest chart below shows the ROC results of OAB prediction by each machine learning model, including XGBboost, LightGBM, Decision Tree, MLP, and AdaBoost. The error lines in the chart are the ROC mean value and SD. Among all the current models, XGBboost is the best-performing training set; meanwhile, the best validation set is XGBboost as well (sorted by AUC).
Figure 5 (A) Using XGBoost model predict the relative importance; (B) Forest map shows the ROC results of OAB prediction by each model; (C) Predicted ROC curve of adverse events in the training sets of the five models; (D) Predicted ROC curve of adverse events in the validation sets of the five models.
As shown in Supplementary Table S7, we conducted subgroup analysis by socioeconomics including age, gender, race, education, ratio of family income to assessed the associations between diabetes and OAB. In the age <60 group, compared with non-diabetes participants, borderline diabetes participants’ OAB prevalence increased by 146%% after adjusting for all covariates and diabetes mellitus participants’ OAB prevalence increased 67%. However, this association was not statistically different in the older population. In addition, it is likely that male, education level more than high school, non-Hispanic Black and middle income (PIR 1.0-3.0) participants had significant significance of diabetes and OAB association. We have multiple interpolated the socioeconomic status including poverty income ratio (n = 2252), sedentary time (n = 31), and education level (n = 3651) and to validate the research results. We found that multiple interpolation results had almost correlated result with delete missing values results. (Supplementary Tables S4–S6). In the sensitivity analysis of model 3, we further adjusted the covariates of diabetes controlling status and they had almost same result with previous result.
The presence of OAB exerts a substantial impact on the overall well-being and health status of patients, significantly burdening their physical and mental health as well as their overall quality of life. However, the pathophysiology of OAB is complicated, and the underlying processes remain unknown. This research examines the link between OAB, diabetes, and systemic inflammation, as well as the associated variables that influence bladder overactivity.
First, we investigated the association between diabetes and OAB. The prevalence of OAB in the diabetes group increased by 77% compared to those without diabetes, and multiple logistic regression analysis showed positive correlations between diabetes-related markers and overactive bladder in adults. We also identified inflammatory markers that were significantly correlated with OAB and diabetes-related markers by multiple linear regression. Mediation analysis showed that white blood cells strongly influenced the correlations between diabetes-related indicators (glycohemoglobin, fasting glucose, and insulin) and OAB. Through the application of machine learning, we have finally identified glycoproteins as the most significant markers for bladder overactivity.
Previously, several studies have found that diabetes is an independent risk factor for OAB (12, 25). A number of clinical trials have also shown the connection between OAB and diabetes (26–28), which is consistent with the research results in this paper. On the one hand, urotheliogenic has been identified as the main cause of OAB (29, 30), and the changes in the urothelium may lead to increased spontaneous activity of the detrusor muscle, causing symptoms of OAB to worsen (31). On the other hand, Wang et al. reported that diabetic OAB patients noted lower expression of urothelial adhesion and higher urothelial inflammation (17). Moreover, diabetes is associated with increased levels of systemic inflammatory markers, which have major effects (32, 33). However, the above research is mostly single-center and with a small sample. The evidence of large-population epidemiology is still lacking. Through our research, we found white blood cells and neutrophils mediated the correlation between glucose, fasting glucose, and insulin and an overactive bladder. Therefore, our hypothesis based on the analysis results of the association between OAB, diabetes, and systemic inflammation is that diabetes mellitus may increase OAB risk by promoting systemic inflammation.
The primary advantages of this research are as follows: Firstly, we conducted a cross-sectional study based on the National Health and Nutrition Examination Survey (NHANES) 2007–2018, which includes an unprecedented amount of data. Second, we suggest for the first time that inflammation mediates the relationship between diabetes and OAB. Finally, glycohemoglobin has been regarded as the most significant marker of diabetes-related indicators and inflammatory markers for bladder overactivity, which contains some clinical value. However, our study still has some limitations. First, the data in this paper is cross-sectional; therefore, we are unable to speculate on cause-and-effect relationships. Second, to a certain extent, they had some overlapping diagnosis between OAB and diabetic cystopathy. And, we could not identify the diabetic cystopathy with OAB. According to AUA guide (34), OAB is not a disease, but a complex syndrome. Our research was mainly focused on the syndrome of OAB diagnosis which based on clinical signs and symptoms. Last, NHANES was a descriptive study and could not prove related risk between diabetes and urinary infections or overactive bladder. We could only provide the association cross-sectional relevance between the OAB and diabetes. Our research illustrated that diabetes associated with OAB was partly attributed by systemic inflammation in terms of public epidemiology. Systemic inflammation is different from urinary system inflammation or urinary system infection. It is true that infection or neurogenic lesions may partly lead to OAB as well which need further study.
Our research is the first large-population epidemiology that revealed that diabetes mellitus and diabetes-related markers were remarkably associated with OAB in the American general adult population. We also observed systemic inflammation as an important mediator between diabetes-related markers and OAB, which will guide the direction for further study on OAB function and mechanism.
The original contributions presented in the study are included in the article/Supplementary Material. Further inquiries can be directed to the corresponding authors.
The studies involving humans were approved by NAHNES Institutional Review Board (IRB)/NCHS Research Ethics Review Board (ERB). The studies were conducted in accordance with the local legislation and institutional requirements. The participants provided their written informed consent to participate in this study.
QH: Writing – review & editing, Writing – original draft, Visualization, Software, Methodology, Formal analysis, Data curation, Conceptualization. LW: Writing – review & editing, Supervision, Methodology, Funding acquisition, Conceptualization. CD: Writing – review & editing, Methodology, Conceptualization. JH: Writing – review & editing, Methodology, Conceptualization. JW: Writing – review & editing, Validation. CW: Writing – review & editing, Writing – original draft, Visualization, Software, Methodology, Formal analysis, Data curation, Conceptualization. ZY: Writing – review & editing, Supervision, Methodology, Funding acquisition, Conceptualization.
The author(s) declare financial support was received for the research, authorship, and/or publication of this article. This research was funded by Startup Fund for scientific research, Fujian Medical University (Grant No.2020QH1125).
CW much thanks to Dr. Qi Xiong for her support and help.
The authors declare that the research was conducted in the absence of any commercial or financial relationships that could be construed as a potential conflict of interest.
All claims expressed in this article are solely those of the authors and do not necessarily represent those of their affiliated organizations, or those of the publisher, the editors and the reviewers. Any product that may be evaluated in this article, or claim that may be made by its manufacturer, is not guaranteed or endorsed by the publisher.
The Supplementary Material for this article can be found online at: https://www.frontiersin.org/articles/10.3389/fendo.2024.1386639/full#supplementary-material
1. Raju R, Linder BJ. Evaluation and treatment of overactive bladder in women. Mayo Clin Proc. (2020) 95:370–7. doi: 10.1016/j.mayocp.2019.11.024
2. El-Zawahry A. Combination pharmacotherapy for treatment of overactive bladder (Oab). Curr Urol Rep. (2019) 20:33. doi: 10.1007/s11934-019-0893-5
3. Peyronnet B, Mironska E, Chapple C, Cardozo L, Oelke M, Dmochowski R, et al. A comprehensive review of overactive bladder pathophysiology: on the way to tailored treatment. Eur Urol. (2019) 75: 995. doi: 10.1016/j.eururo.2019.02.038
4. Grundy SM, Cleeman JI, Daniels SR, Donato KA, Eckel RH, Franklin BA, et al. Diagnosis and management of the metabolic syndrome: an american heart association/national heart, lung, and blood institute scientific statement. Circulation. (2005) 112:2735–52. doi: 10.1161/CIRCULATIONAHA.105.169404
5. Akhtar M, Taha NM, Nauman A, Mujeeb IB, Al-Nabet ADMH. Diabetic kidney disease: past and present. Adv Anat Pathol. (2020) 27:87–97. doi: 10.1097/PAP.0000000000000257
6. Yuan Z, Tang Z, He C, Tang W. Diabetic cystopathy: A review. J Diabetes. (2015) 7:442–7. doi: 10.1111/1753-0407.12272
7. Frimodt-Møller C. Diabetic cystopathy: epidemiology and related disorders. Ann Intern Med. (1980) 92:318–21. doi: 10.7326/0003-4819-92-2-318
8. Liu R-T, Chung M-S, Lee W-C, Chang S-W, Huang S-T, Yang KD, et al. Prevalence of overactive bladder and associated risk factors in 1359 patients with type 2 diabetes. Urology. (2011) 78:1040–5. doi: 10.1016/j.urology.2011.05.017
9. Jackson RA, Vittinghoff E, Kanaya AM, Miles TP, Resnick HE, Kritchevsky SB, et al. Urinary incontinence in elderly women: findings from the health, aging, and body composition study. Obstet Gynecol. (2004) 104:301–7. doi: 10.1097/01.AOG.0000133482.20685.d1
10. Kelly MS, Routh JC, Davis LG, Purves JT, Wiener JS, Maciejewski ML. Lower urinary tract symptoms in older children with and without diabetes mellitus. Clin Pediatr (Phila). (2018) 57:1576–81. doi: 10.1177/0009922818796605
11. Bolgeo T, Maconi A, Bertolotti M, Roveta A, Betti M, Gatti D, et al. Physiopathology of the diabetic bladder. Arch Ital Urol Androl. (2020) 92:314. doi: 10.4081/aiua.2020.4.314
12. Chiu A-F, Huang M-H, Wang C-C, Kuo H-C. Higher glycosylated hemoglobin levels increase the risk of overactive bladder syndrome in patients with type 2 diabetes mellitus. Int J Urol. (2012) 19:999. doi: 10.1111/j.1442-2042.2012.03095.x
13. Johnston S, Janning SW, Haas GP, Wilson KL, Smith DM, Reckard G, et al. Comparative persistence and adherence to overactive bladder medications in patients with and without diabetes. Int J Clin Pract. (2012) 66:1042–51. doi: 10.1111/ijcp.2012.66.issue-11
14. Brown JS. Diabetic cystopathy–what does it mean? J Urol. (2009) 181:13–4. doi: 10.1016/j.juro.2008.10.078
15. Wang C-C, Jiang Y-H, Kuo H-C. The pharmacological mechanism of diabetes mellitus-associated overactive bladder and its treatment with botulinum toxin A. Toxins (Basel). (2020) 12:6. doi: 10.3390/toxins12030186
16. Inouye BM, Hughes FM, Jin H, Lütolf R, Potnis KC, Routh JC, et al. Diabetic bladder dysfunction is associated with bladder inflammation triggered through hyperglycemia, not polyuria. Res Rep Urol. (2018) 10:219–25. doi: 10.2147/RRU.S177633
17. Wang C-C, Kuo H-C. Urothelial dysfunction and chronic inflammation in diabetic patients with overactive bladder. Low Urin Tract Symptoms. (2017) 9:151–6. doi: 10.1111/luts.12126
18. Futyma K, Nowakowski Ł, Ziętek-Strobl A, Kamińska A, Taoussi N, Rechberger T. Urine proteomic study in oab patients-preliminary report. J Clin Med. (2020) 9:5. doi: 10.3390/jcm9051389
19. Verma V, Gupta S, Kumar P, Yadav S, Dhanda RS, Gaind R, et al. Involvement of nlrp3 and nlrc4 inflammasome in uropathogenic E. Coli Mediated Urinary Tract Infections. Front Microbiol. (2019) 10:2020. doi: 10.3389/fmicb.2019.02020
20. Hughes FM, Odom MR, Cervantes A, Purves JT. Inflammation triggered by the nlrp3 inflammasome is a critical driver of diabetic bladder dysfunction. Front Physiol. (2022) 13:920487. doi: 10.3389/fphys.2022.920487
21. Qiu Z, Chen X, Geng T, Wan Z, Lu Q, Li L, et al. Associations of serum carotenoids with risk of cardiovascular mortality among individuals with type 2 diabetes: results from nhanes. Diabetes Care. (2022) 45:1453–61. doi: 10.2337/dc21-2371
22. Blaivas JG, Panagopoulos G, Weiss JP, Somaroo C. Validation of the overactive bladder symptom score. J Urol. (2007) 178:543–7. doi: 10.1016/j.juro.2007.03.133
23. VanderWeele TJ. Explanation in causal inference: developments in mediation and interaction. Int J Epidemiol. (2016) 45:1904–8. doi: 10.1093/ije/dyw277
24. Valeri L, Vanderweele TJ. Mediation analysis allowing for exposure-mediator interactions and causal interpretation: theoretical assumptions and implementation with sas and spss macros. psychol Methods. (2013) 18:137–50. doi: 10.1037/a0031034
25. Palleschi G, Pastore AL, Maggioni C, Fuschi A, Pacini L, Petrozza V, et al. Overactive bladder in diabetes mellitus patients: A questionnaire-based observational investigation. World J Urol. (2014) 32:1021–5. doi: 10.1007/s00345-013-1175-3
26. Martin SA, Haren MT, Marshall VR, Lange K, Wittert GA. Prevalence and factors associated with uncomplicated storage and voiding lower urinary tract symptoms in community-dwelling Australian men. World J Urol. (2011) 29:179–84. doi: 10.1007/s00345-010-0605-8
27. Golabek T, Kiely E, O'Reilly B. Detrusor overactivity in diabetic and non-diabetic patients: is there a difference? Int Braz J urol Off J Braz Soc Urol. (2012) 38:652–9. doi: 10.1590/s1677-55382012000500010
28. Bunn F, Kirby M, Pinkney E, Cardozo L, Chapple C, Chester K, et al. Is there a link between overactive bladder and the metabolic syndrome in women? A systematic review of observational studies. Int J Clin Pract. (2015) 69:199–217. doi: 10.1111/ijcp.12518
29. Maggi CA, Patacchini R, Santicioli P, Geppetti P, Cecconi R, Giuliani S, et al. Multiple mechanisms in the motor responses of the Guinea-pig isolated urinary bladder to bradykinin. Br J Pharmacol. (1989) 98:619–29. doi: 10.1111/j.1476-5381.1989.tb12636.x
30. Birder LA, de Groat WC. Mechanisms of disease: involvement of the urothelium in bladder dysfunction. Nat Clin Pract Urol. (2007) 4:46–54. doi: 10.1038/ncpuro0672
31. Meng E, Young JS, Brading AF. Spontaneous activity of mouse detrusor smooth muscle and the effects of the urothelium. Neurourology urodynamics. (2008) 27:79–87. doi: 10.1002/nau.20456
32. Borst SE. The role of tnf-alpha in insulin resistance. Endocrine. (2004) 23:177–82. doi: 10.1385/endo:23:2-3:177
33. Nesto RW, Rutter MK. Impact of the atherosclerotic process in patients with diabetes. Acta diabetologica. (2002) 39 Suppl 2:S22–8. doi: 10.1007/s005920200022
Keywords: diabetes, inflammation, overactive bladder, NHANES, epidemiology
Citation: He Q, Wu L, Deng C, He J, Wen J, Wei C and You Z (2024) Diabetes mellitus, systemic inflammation and overactive bladder. Front. Endocrinol. 15:1386639. doi: 10.3389/fendo.2024.1386639
Received: 15 February 2024; Accepted: 10 April 2024;
Published: 29 April 2024.
Edited by:
Jian Ma, Harbin Medical University, ChinaReviewed by:
Jad Ahmad Degheili, Children’s Hospital of Eastern Ontario (CHEO), CanadaCopyright © 2024 He, Wu, Deng, He, Wen, Wei and You. This is an open-access article distributed under the terms of the Creative Commons Attribution License (CC BY). The use, distribution or reproduction in other forums is permitted, provided the original author(s) and the copyright owner(s) are credited and that the original publication in this journal is cited, in accordance with accepted academic practice. No use, distribution or reproduction is permitted which does not comply with these terms.
*Correspondence: Zhijiao You, emhhb2h1aWNoZW5AaHVzdC5lZHUuY24=; Chengcheng Wei, Y2hlbmdjaGVuZ3dlaUBodXN0LmVkdS5jbg==; Jun Wen, d2VuY2VqQHN0dS5jcW11LmVkdS5jbg==
†These authors have contributed equally to this work
Disclaimer: All claims expressed in this article are solely those of the authors and do not necessarily represent those of their affiliated organizations, or those of the publisher, the editors and the reviewers. Any product that may be evaluated in this article or claim that may be made by its manufacturer is not guaranteed or endorsed by the publisher.
Research integrity at Frontiers
Learn more about the work of our research integrity team to safeguard the quality of each article we publish.