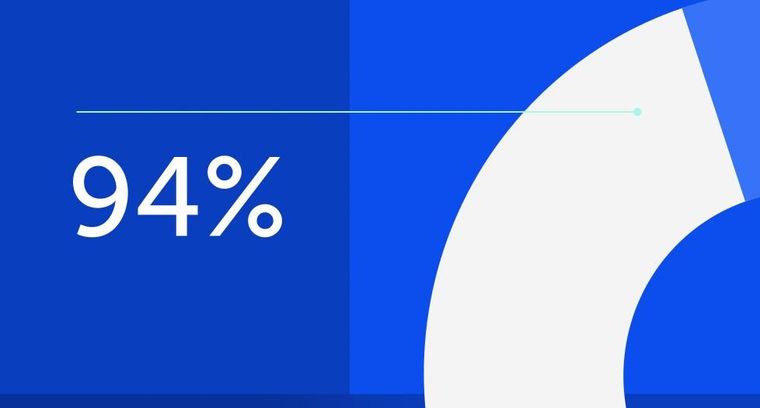
94% of researchers rate our articles as excellent or good
Learn more about the work of our research integrity team to safeguard the quality of each article we publish.
Find out more
ORIGINAL RESEARCH article
Front. Endocrinol., 21 October 2024
Sec. Thyroid Endocrinology
Volume 15 - 2024 | https://doi.org/10.3389/fendo.2024.1374254
This article is part of the Research TopicAdvances in the Research of Diabetic Retinopathy, Volume IIIView all 15 articles
Background: Thyroid dysfunction exhibits a heightened prevalence among people with diabetes compared to those without diabetes. Furthermore, TD emerges as a notable correlated risk factor for the onset of diabetic retinopathy.
Methods: Using data from the FinnGen database (R9), we investigated the causal relationship between thyroid dysfunction (TD) and four stages of diabetic retinopathy (DR). A two-sample univariable Mendelian randomization (UVMR) approach was employed to estimate the total causal effect of TD on four stages of DR, while multivariable Mendelian randomization (MVMR) was used to assess the direct causal effect. The meta-analysis was conducted to summarize the collective effect of TD on four stages of DR. The inverse variance weighted (IVW) method was the primary approach for Mendelian randomization analysis, with heterogeneity, horizontal pleiotropy, and leave-one-out sensitivity analyses performed to validate the robustness of the findings.
Results: In UVMR analysis, thyrotoxicosis (TOS) was significantly associated with an increased risk of diabetic retinopathy across four stages (OR, 1.10–1.19; P<0.025). However, MVMR analysis, after adjusting for Graves’ disease (GD) and/or rheumatoid arthritis (RA), revealed no significant association between TOS and the four stages of diabetic retinopathy. The Meta-analysis demonstrated the collective effect of TOS on diabetic retinopathy across all stages [OR=1.11; 95% CI (1.08–1.15); P<0.01]. In UVMR analysis, the estimates for hypothyroidism (HPT) and GD were similar to those for TOS. In the MVMR analysis, after adjusting for RA, the significant effect of HPT on DR and non-proliferative diabetic retinopathy (NPDR) remained. Additionally, MVMR analysis suggested that the estimates for GD on DR were not affected by TOS, except for GD-proliferative diabetic retinopathy (PDR). However, no significant correlation persisted after adjusting for RA, including for GD-PDR.
Conclusion: Our study demonstrated a significant association between thyroid dysfunction TD and DR, with the relationship being particularly pronounced in HPT-DR.
Diabetic retinopathy(DR), a prominent microvascular complication of diabetes, stands as a leading cause of adult blindness (1). Recent investigations highlight a global prevalence of 34.6% for DR (2). The pathophysiological spectrum of DR encompasses two primary categories: proliferative DR(PDR) and nonproliferative DR(NPDR). In cases where PDR or macular edema with central involvement manifests, intravitreal anti-vascular endothelial growth factor(VEGF) therapy proves efficacious. However, the challenge of rapid recurrence upon drug discontinuation remains a noteworthy concern (3). Nevertheless, due to cost-effectiveness, it remains unrecognized for the treatment of NPDR. DR has garnered growing public concern owing to its widespread prevalence, expensive treatment modalities, and adverse impact on health. Consequently, it is a rising imperative to investigate etiological factors to avert the development of DR. Recent studies have reported that thyroid dysfunction(TD) may elevate the prevalence of DR (4).
TD, encompassing thyrotoxicosis(TOS), hypothyroidism(HPT), and autoimmune thyroid diseases. Diabetes mellitus(DM) and TD are prevalent endocrine system maladies in clinical practice, exhibiting an inherent connection (5). Blood glucose plays a pivotal role in regulating the hypothalamic-pituitary-thyroid axis and the release of thyroid-stimulating hormone(TSH) from the pituitary. Simultaneously, it influences the conversion of thyroxine to triiodothyronine in peripheral tissues (5). Concurrently, numerous observational studies highlight a higher prevalence of TD in diabetic populations compared to non-diabetic counterparts, particularly in type 1 diabetes mellitus, suggesting a robust shared genetic susceptibility. Serum levels of TSH represent an independent risk factor for DR (6). Despite this, the role of TD remains insufficiently emphasized in the context of DR prevention and treatment. Consequently, the paper aims to investigate the potential relationship between TD and diabetic retinopathy.
Nevertheless, existing evidence regarding the link between TD and diabetic retinopathy primarily stems from observational studies, introducing challenges such as confounding bias and reverse causality. To more accurately assess the potential causal relationships between TD, including TOS, HPT and Graves’ disease (GD), and various stages of DR, genetic approaches have surfaced as a reliable alternative for assessing causality. The approach helps circumvent interference from confounding or reverse causality (7). Mendelian randomization(MR) stands out as a robust analytical method for accomplishing this objective. Grounded in Mendel’s laws, MR is less susceptible to confounding factors. Its emphasis lies in investigating the causal relationship between the exposures and the outcomes through the utilization of genetic variants that meet three fundamental assumptions as instrumental variables(IVs) (8).
As illustrated in Figure 1, the study design was based on the latest genome-wide association studies from the FinnGen database (R9) focusing on TD, rheumatoid arthritis (RA), and four stages of DR: background diabetic retinopathy (DBR), DR, NPDR, and PDR. Pooled data were analyzed using two-sample univariable Mendelian randomization (UVMR) to estimate the total causal effect of each exposure and multivariable Mendelian randomization (MVMR) to assess the direct causal effects of multiple exposures simultaneously. Meta-analysis was conducted for the pooled analysis. Additionally, a series of sensitivity analyses were performed to evaluate potential biases, including heterogeneity and horizontal pleiotropy, within the MR framework. The implementation of MR analysis relies on three critical assumptions, constituting an indispensable prerequisite for conducting analyses (9, 10): (1) Correlation: A robust correlation must exist between genetic variants and exposures; (2) Independence: Genetic variation should demonstrate independence from confounding factors; (3) Exclusion restriction: Genetic variation should exclusively influence the outcome through the targeted exposure factors. The present reporting and analysis procedures adhered to the STROBE-MR guidelines (11). Table 1 provides a concise overview of the characteristics of the data sources utilized for the MR analysis, all of which are publicly accessible.
Figure 1. A flow diagram of the process in the MR analysis. (A) The SNPs data in the MR; (B) the selection of the IVs in the MVMR; (C) the selection of the IVs in the UVMR; (D) the analysis methods of MR.
In the study, we queried the FinnGen(R9) database to identify key single-nucleotide polymorphisms(SNPs) serving as IVs, guided by three fundamental hypotheses (Figure 1). Firstly, we evaluated the correlation of IVs with exposures using genome-wide significance levels (P < 5e-08) and an F statistic (>10) (12). The F statistic was computed using the following formula:
R2 is defined as the ability of the genetic variance to explain the exposure; k is the number of IVs used in the model; and n is the sample size.
Secondly, linkage disequilibrium (LD) was employed to guarantee the independence of IVs from other genes (kb = 5000, r2 = 0.01) (13, 14). Proxy SNPs were not used in cases where there were no SNPs associated with exposures in the outcomes. Additionally, we harmonized genetic variation by merging exposures and outcomes, concurrently eliminating palindromic SNPs. Lastly, SNPs significantly linked to outcomes (P < 5e-08) were also excluded.
In the UVMR analysis, several MR methods were employed to substantiate total causal effect between TD and four stages of DR (Figure 1). These methods encompassed inverse variance weighting(IVW), MR-Egger, weighted median(WM), Simple mode, and Weighted mode (15). Among these, IVW, grounded on the equilibrium assumption of horizontal pleiotropy of IVs, stands out for its ability to disregard IVs’ heterogeneity, rendering it the most frequent approach in MR analysis (16). Other MR methods were employed to offer supplementary evaluations. The MR-Egger method, akin to IVW, differs primarily in considering the presence of intercept term in the regression. Furthermore, WM estimates furnish a reliable evaluation of causality even when only 50% of the IVs are valid (17). In MVMR analyses, the estimation of the direct causal effect of TD on four stages of DR predominantly relies on IVW and MR-Egger regression (Figure 1).
Subsequent to detecting causal effects through the aforementioned methods, we conducted sensitivity analyses to scrutinize the robustness of the MR findings, encompassing tests for heterogeneity and horizontal pleiotropy (Figure 1). In UVMR analyses, the identification of outliers is significantly reliant on the MR-PRESSO test and the RadialMR (9). Cochrane’s Q statistic (P > 0.05) and MR-Egger Intercept (P > 0.05) were separately employed in the study to assess the heterogeneity and horizontal pleiotropy (18). Furthermore, we evaluated the impact of individual SNP on the pooled causal estimates using the leave-one-out method, aiming to discern the presence of potentially pleiotropic SNPs that might influence the causal estimates (19). Statistical power for the UVMR study was calculated using the mRnd online tool (https://shiny.cnsgenomics.com/mRnd/). We employed the MR Steiger directionality test to evaluate the directionality of UVMR causal estimates. The TwoSampleMR and MendelianRandomization packages were utilized to identify potential confounders. In MVMR analyses, Cochrane’s Q statistic was primarily used to assess study heterogeneity, and MR-Egger intercept was employed to evaluate horizontal pleiotropy.
The TwoSampleMR package and the RadialMR package were employed for UVMR analyses within the R (version 4.2.3) (20, 21). For MVMR analyses, we utilized the MendelianRandomization package. Meta-analyses were conducted using the Meta package. A significance threshold of P < 0.05 was established for statistical significance. Bonferroni correction was applied to redefine the threshold of statistical significance (P < 0.05/n) to account for multiple testing, where n denotes the number of MR tests (14). The adjusted p-value for the TD-diabetic retinopathy (DBR/DR/NPDR/PDR) analysis was set at 0.025. Similarly, the MVMR analyses also employed an adjusted p-value (0.025). The OR, beta values and their respective 95% confidence intervals were utilized to furnish estimates of relative risk.
The UVMR study examined the total causal effect between TD and DR using three different exposures and four stages of outcomes. All IVs were significantly associated with the exposures (P<5e-08) and had F-statistics greater than 10 (Supplementary Tables 1–12), indicating a strong association with the exposure. Additionally, outliers were eliminated by MR-PRESSO (Supplementary Table: MR results sum1-12) and the RadialMR (Supplementary Figures 1–3), and each SNP was not associated with the outcome (P>5e-08) (Supplementary Tables 1–12). Given GD is a known etiology of TOS, the pleiotropic effects of TOS and GD may violate the exclusion restriction hypothesis. Our analysis using the Phenoscanner package identified RA as a potentially influential confounder or mediator (Supplementary Table: potential confounders), with SNPs associated with RA detailed in Supplementary Table 13. Furthermore, prior reports have hinted at ambiguous associations of RA with both diabetic retinopathy and TD (22–24). Consequently, we employed MVMR to adjust for TOS, GD, and RA.
The results of the UVMR analyses passed Cochrane’s Q test (Tables 2, 3). With the exception of the GD-NPDR analysis, all UVMR analyses passed the MR-Egger intercept test (Tables 2, 3). Leave-one-out analyses confirmed that the causality observed in the UVMR analyses was not driven by any single SNP (Supplementary Figures 4–6). Additionally, the MR Steiger directionality test results supported the accuracy of our causal direction estimates (Supplementary Tables 1–12), further validating the robustness of the UVMR findings. The statistical power of the UVMR studies, as calculated using the web tool, was all greater than 0.9. Although the heterogeneity test (P<0.05) indicated some heterogeneity in the MVMR analyses, no horizontal pleiotropy was detected (P>0.05). The meta-analysis showed heterogeneity in both the overall and partial subgroup analyses, therefore, the random-effects model was used to combine the effect sizes.
In populations of European ancestry (EA), IVW analyses indicated a potential causal relationship between genetically predicted TOS and DBR/DR/NPDR/PDR (Table 2). However, WM and MR-Egger did not provide similar evidence in the TOS-DBR/DR/NPDR/PDR analyses (P>0.025). Notably, the OR values from both WM and MR-Egger were all greater than 1 (Table 2), and the scatter plot suggested a positive correlation between TOS and DBR/DR/NPDR/PDR (Supplementary Figure 7), indicating consistency in the directionality of the results. Sensitivity analyses showed no evidence of bias, supporting the validity of the findings. Similarly, in the HPT-DR analyses, IVW indicated that genetically predicted HPT was potentially causally related to DBR/DR/NPDR/PDR (Table 3). In the MR results for HPT-DR/PDR, MR-Egger did not provide supporting evidence (P>0.025). However, WM provided consistent estimates, and the ORs from MR-Egger and WM were all greater than 1 (Table 3). The scatter plot further demonstrated a potential positive correlation between HPT and DR/PDR (Supplementary Figures 8B, D), which aligns with our overall interpretation of the MR results.
In contrast to the previous findings, IVW, WM, and MR-Egger all indicated a potential causal relationship between genetically predicted GD and DR/DBR/NPDR/PDR (Table 4).
When accounting for GD and/or RA, both IVW and MR-Egger analyses suggested that genetically predicted TOS did not significantly increase the risk of DBR/DR/NPDR/PDR, though the results were not statistically significant (Tables 5, 6).
When RA was considered, both IVW and MR-Egger analyses suggested that genetically predicted HPT could still increase the risk of developing DR (DR/NPDR) (Tables 5, 6). However, for DBR, genetically predicted HPT was found to be non-significant after adjusting for RA in both IVW and MR-Egger analyses (Tables 5, 6). While IVW analysis indicated that genetically predicted HPT was not significantly associated with PDR after adjusting for RA (Table 5), MR-Egger analysis suggested that genetically predicted HPT could still increase the risk of PDR (Table 6), with both MR-Egger and IVW analyses showing a positive association. Therefore, the study concludes that HPT may still increase the risk of PDR after adjusting for RA.
When considering TOS, both IVW and MR-Egger analyses indicated that genetically predicted GD could still increase the risk of developing DBR/DR/NPDR (Tables 5, 6). However, when both TOS and RA were considered, genetically predicted GD was no longer associated with DBR/DR/NPDR/PDR (Tables 5, 6).
In UVMR analysis, this study assessed the effect of TD on diabetic retinopathy across its four stages. A meta-analysis, conducted using R software, summarized the overall impact of TD on DBR/DR/NPDR/PDR based on UVMR results. As shown in Figure 2, the effect of GD and HPT on DBR/DR/NPDR/PDR was greater than that of TOS, with the difference being statistically significant (P<0.01). Although the meta-analysis showed significance in both subgroup and overall analyses (P<0.01), substantial heterogeneity was noted in the HPT/GD subgroup as well as in the overall estimates.
The two-sample UVMR study demonstrated that genetically predicted TD, particularly HPT, can increase the risk of diabetic retinopathy in patients with DM, with the robustness of the analytical procedure confirmed. In MVMR analysis, no significant association was found between TOS and diabetic retinopathy after adjusting for GD. However, GD remained significantly associated with diabetic retinopathy, except for GD-PDR, after adjusting for TOS. This observed difference is thought-provoking. The study by Lin D et al. (6) provides a plausible explanation for the discrepancy. They found that bovine TSH (b-TSH) promotes glucose-induced mitochondrial apoptosis in human peripapillary retinal cells, whereas blocking TSHR significantly inhibits mitochondrial apoptosis in a high-glucose environment (6). In thyrotoxicosis, elevated levels of thyroxine and triiodothyronine inhibit TSH production via negative feedback on the hypothalamic-pituitary-thyroid axis. In contrast, in GD, thyroid-stimulating antibodies (TSAb) mimic the effects of TSH by binding to TSHR, which may explain the observed difference. In contrast, hypothyroidism involves positive feedback activation of the hypothalamic-pituitary-thyroid axis. Both univariate and multivariate MR analyses indicated a significant association between HPT and diabetic retinopathy, aligning with a prior meta-analysis of observational studies that reported a significant correlation between hypothyroidism and diabetic retinopathy (OR=2.13, 95% CI=1.41–3.23, P<0.001) (25). A subsequent meta-analysis reached a similar conclusion (26). Additionally, a case-control study demonstrated a significant association between hypothyroidism and an increased prevalence of diabetic retinopathy in DM patients, even after adjusting for age, gender, diabetes duration, glycosylated hemoglobin, BMI, hypertension, and LDL cholesterol (27). Furthermore, higher thyrotropic hormone levels have been linked to narrower retinal arterioles and lower arteriovenous indices in patients with hypothyroidism compared to those with normal thyroid function (28). In the pooled analysis, we observed that the overall effect of HPT on diabetic retinopathy was greater than that of TOS or GD. While our findings, along with previous studies, seem to implicate TSH as the key factor driving the increased risk of diabetic retinopathy associated with TD. But is this really the case? A retrospective study in a Caucasian population, for instance, found no significant association between TSH levels or hypothyroidism and diabetic retinopathy (29). Moreover, UVMR analysis suggested the presence of horizontal pleiotropy in GD-NPDR, but after adjusting for TOS, the pleiotropy disappeared, and both MR analyses indicated that GD could increase the risk of developing NPDR. This result seems to imply that thyrotoxicosis alone plays a role in the GD-NPDR axis. However, an MR study on thyroid hormones and microvascular complications in diabetes suggested that elevated thyroid hormone levels do not increase the risk of developing DR (30). This may be due to the fact that the study, when selecting IVs, focused solely on hormone levels and overlooked the disease itself. Additionally, variations in the sources of exposure-related IVs may have contributed to the differences in the analytical results.
Diabetic retinopathy constitutes a significant microvascular complication of diabetes (1), wherein microvascular injury plays a pivotal role in its pathogenesis. Various factors contribute to microvascular injury, including hypoxia, endothelial damage, oxidative stress, inflammatory response, and fibrovascular proliferation. In individuals with TD, both TOS and HPT are associated with elevated serum C-reactive protein levels, indicating a heightened systemic inflammatory response (5). Furthermore, conditions such as GD and Hashimoto thyroiditis can all result in increased serum VEGF levels (31). Individuals with HPT exhibit increased activity of plasma malondialdehyde, a specific indicator of oxidative stress levels (5). Moreover, thyroid hormones play a significant role in the normal development of retinal cellular structures, as evidenced by researchers discovering low levels of sirtuin2 in the retinal ganglion cell layer of hypothyroid mice (32). Therefore, the impact of TD on diabetic retinopathy may not solely be attributed to the level of TSH and thyroid hormone but rather the combined influence of numerous factors.
Compared to observational studies, the MR study can significantly mitigate confounding effects. However, the current MR design possesses both its own strengths and inherent limitations, primarily stemming from three essential assumptions that must be satisfied. Firstly, the correlation assumption was supported by the genome-wide significance level (P<5e-08) in the GWAS. Additionally, the UVMR studies are less susceptible to weak instrument bias, as we exclusively incorporated SNPs with substantial instrumental strength (F>10) while excluding those in linkage disequilibrium. Nevertheless, MVMR analyses exclusively considered SNPs with genome-wide significance levels (P<5e-08) and eliminated those in linkage disequilibrium. Secondly, the MR-Egger Intercept evaluated the horizontal pleiotropy in the UVMR analysis, indicating an absence of horizontal pleiotropy. However, during confounder screening, we identified RA as potentially influencing the causal chain of TD on diabetic retinopathy. Consequently, confounding was addressed through the MVMR analysis. Nevertheless, violations may persist, and alternative pleiotropic pathways from IVs to diabetic retinopathy remain unexplored in this study, necessitating investigation in future research. Thirdly, the genetic variant data predominantly relied on GWAS from European ancestry. The approach has the drawback of lacking the ability to fully represent the entire population. However, it offers the advantage of minimizing the risk of population-based confounding. Additionally, heterogeneity was observed in the MVMR analysis, but we employed the IVW with a random-effects model to evaluate the MVMR results. Lastly, given the presence of heterogeneity, the meta-analysis utilized a random-effects model to amalgamate effect sizes.
In conclusion, our study demonstrated a significant correlation between TD and diabetic retinopathy, with a particularly strong association for HPT. In the HPT-DBR/DR/NPDR/PDR MVMR analysis, HPT remained significantly associated with DBR/DR/NPDR/PDR even after adjusting for RA, suggesting that the impact of HPT on diabetic retinopathy is independent of RA.
The original contributions presented in the study are publicly available. This data can be found here: https://github.com/ling-98/TD-DR-MR-analyses.git.
JO: Writing – review & editing, Writing – original draft, Software, Methodology, Investigation, Formal analysis, Data curation, Conceptualization. LZ: Writing – review & editing, Writing – original draft, Software, Methodology, Investigation, Formal analysis, Data curation, Conceptualization. QW: Writing – review & editing, Writing – original draft. WY: Writing – review & editing, Writing – original draft.
The author(s) declare that no financial support was received for the research, authorship, and/or publication of this article.
We sincerely appreciate for all the GWAS data provided by the FinnGen.
The authors declare that the research was conducted in the absence of any commercial or financial relationships that could be construed as a potential conflict of interest.
All claims expressed in this article are solely those of the authors and do not necessarily represent those of their affiliated organizations, or those of the publisher, the editors and the reviewers. Any product that may be evaluated in this article, or claim that may be made by its manufacturer, is not guaranteed or endorsed by the publisher.
The Supplementary Material for this article can be found online at: https://www.frontiersin.org/articles/10.3389/fendo.2024.1374254/full#supplementary-material
1. Cheung N, Mitchell P, Wong TY. Diabetic retinopathy. Lancet. (2010) 376:124–36. doi: 10.1016/S0140-6736(09)62124-3
2. Yau JW, Rogers SL, Kawasaki R, Lamoureux EL, Kowalski JW, Bek T, et al. Global prevalence and major risk factors of diabetic retinopathy. Diabetes Care. (2012) 35:556–64. doi: 10.2337/dc11-1909
3. Tan TE, Wong TY. Diabetic retinopathy: Looking forward to 2030. Front Endocrinol (Lausanne). (2023) 13:1077669. doi: 10.3389/fendo.2022.1077669
4. Prinz N, Tittel SR, Bachran R, Birnbacher R, Brückel J, Dunstheimer D, et al. Characteristics of patients with type 1 diabetes and additional autoimmune disease in the DPV registry. J Clin Endocrinol Metab. (2021) 106:e3381–9. doi: 10.1210/clinem/dgab376
5. Stefanowicz-Rutkowska MM, Baranowska-Jurkun A, Matuszewski W, Bandurska-Stankiewicz EM. Thyroid dysfunction in patients with diabetic retinopathy. Endokrynol Pol. (2020) 71:176–83. doi: 10.5603/EP.a2020.0013
6. Lin D, Qin R, Guo L. Thyroid stimulating hormone aggravates diabetic retinopathy through the mitochondrial apoptotic pathway. J Cell Physiol. (2022) 237:868–80. doi: 10.1002/jcp.30563
7. Burgess S, Timpson NJ, Ebrahim S, Davey Smith G. Mendelian randomization: where are we now and where are we going? Int J Epidemiol. (2015) 44:379–88. doi: 10.1093/ije/dyv108
8. Davies NM, Holmes MV, Davey Smith G. Reading Mendelian randomisation studies: a guide, glossary, and checklist for clinicians. BMJ. (2018) 362:k601. doi: 10.1136/bmj.k601
9. Xian W, Wu D, Liu B, Hong S, Huo Z, Xiao H, et al. Graves disease and inflammatory bowel disease: A bidirectional Mendelian randomization. J Clin Endocrinol Metab. (2023) 108:1075–83. doi: 10.1210/clinem/dgac683
10. Ishigaki K, Akiyama M, Kanai M, Takahashi A, Kawakami E, Sugishita H, et al. Large-scale genome-wide association study in a Japanese population identifies novel susceptibility loci across different diseases. Nat Genet. (2020) 52:669–79. doi: 10.1038/s41588-020-0640-3
11. Skrivankova VW, Richmond RC, Woolf BAR, Davies NM, Swanson SA, VanderWeele TJ, et al. Strengthening the reporting of observational studies in epidemiology using mendelian randomisation (STROBE-MR): explanation and elaboration. BMJ. (2021) 375:n2233. doi: 10.1136/bmj.n2233
12. Burgess S, Thompson SG, CRP CHD Genetics Collaboration. Avoiding bias from weak instruments in Mendelian randomization studies. Int J Epidemiol. (2011) 40:755–64. doi: 10.1093/ije/dyr036
13. Magnus MC, Miliku K, Bauer A, Engel SM, Felix JF, Jaddoe VWV, et al. Vitamin D and risk of pregnancy related hypertensive disorders: mendelian randomisation study. BMJ. (2018) 361:k2167. doi: 10.1136/bmj.k2167
14. Zhou Z, Zhang H, Chen K, Liu C. Iron status and obesity-related traits: A two-sample bidirectional Mendelian randomization study. Front Endocrinol (Lausanne). (2023) 14:985338. doi: 10.3389/fendo.2023.985338
15. Liang Z, Zhao L, Lou Y, Liu S. Causal effects of circulating lipids and lipid-lowering drugs on the risk of epilepsy: a two-sample Mendelian randomization study. QJM. (2023) 116:421–8. doi: 10.1093/qjmed/hcad048
16. Bowden J, Del Greco MF, Minelli C, Davey Smith G, Sheehan N, Thompson J. A framework for the investigation of pleiotropy in two-sample summary data Mendelian randomization. Stat Med. (2017) 36:1783–802. doi: 10.1002/sim.7221
17. Bowden J, Davey Smith G, Haycock PC, Burgess S. Consistent estimation in Mendelian randomization with some invalid instruments using a weighted median estimator. Genet Epidemiol. (2016) 40:304–14. doi: 10.1002/gepi.21965
18. Burgess S, Thompson SG. Interpreting findings from Mendelian randomization using the MR-Egger method. Eur J Epidemiol. (2017) 32:377–89. doi: 10.1007/s10654-017-0255-x
19. Zheng C, Wei X, Cao X. The causal effect of obesity on diabetic retinopathy: A two-sample Mendelian randomization study. Front Endocrinol (Lausanne). (2023) 14:1108731. doi: 10.3389/fendo.2023.1108731
20. Hemani G, Zheng J, Elsworth B, Wade KH, Haberland V, Baird D, et al. The MR-Base platform supports systematic causal inference across the human phenome. Elife. (2018) 7:e34408. doi: 10.7554/eLife.34408
21. Bowden J, Spiller W, Del Greco MF, Sheehan N, Thompson J, Minelli C, et al. Improving the visualization, interpretation and analysis of two-sample summary data Mendelian randomization via the Radial plot and Radial regression. Int J Epidemiol. (2018) 47:1264–78. doi: 10.1093/ije/dyy101
22. Bartels CM, Wong JC, Johnson SL, Thorpe CT, Barney NP, Sheibani N, et al. Rheumatoid arthritis and the prevalence of diabetic retinopathy. Rheumatol (Oxford). (2015) 54:1415–9. doi: 10.1093/rheumatology/kev012
23. Powell ED, Field RA. Diabetic retinopathy and rheumatoid arthritis. Lancet. (1964) 2:17–8. doi: 10.1016/s0140-6736(64)90008-x
24. Conigliaro P, D'Antonio A, Pinto S, Chimenti MS, Triggianese P, Rotondi M, et al. Autoimmune thyroid disorders and rheumatoid arthritis: A bidirectional interplay. Autoimmun Rev. (2020) 19:102529. doi: 10.1016/j.autrev.2020.102529
25. Wu J, Yue S, Geng J, Liu L, Teng W, Liu L, et al. Relationship between diabetic retinopathy and subclinical hypothyroidism: a meta-analysis. Sci Rep. (2015) 5:12212. doi: 10.1038/srep12212
26. Han C, He X, Xia X, Li Y, Shi X, Shan Z, et al. Subclinical hypothyroidism and type 2 diabetes: A systematic review and meta-analysis. PloS One. (2015) 10:e0135233. doi: 10.1371/journal.pone.0135233
27. Yang JK, Liu W, Shi J, Li YB. An association between subclinical hypothyroidism and sight-threatening diabetic retinopathy in type 2 diabetic patients. Diabetes Care. (2010) 33:1018–20. doi: 10.2337/dc09-1784
28. Ittermann T, Dörr M, Völzke H, Tost F, Lehmphul I, Köhrle J, et al. High serum thyrotropin levels are associated with retinal arteriolar narrowing in the general population. Thyroid. (2014) 24:1473–8. doi: 10.1089/thy.2014.0190
29. Ramis JN, Artigas CF, Santiago MA, Mañes FJ, Canonge RS, Comas LM. Is there a relationship between TSH levels and diabetic retinopathy in the Caucasian population? Diabetes Res Clin Pract. (2012) 97:e45–7. doi: 10.1016/j.diabres.2012.05.015
30. Li H, Li M, Dong S, Zhang S, Dong A, Zhang M. Assessment of the association between genetic factors regulating thyroid function and microvascular complications in diabetes: A two-sample Mendelian randomization study in the European population. Front Endocrinol (Lausanne). (2023) 14:1126339. doi: 10.3389/fendo.2023.1126339
31. Ahmed A, Waris A, Naheed A, Anjum A. Diabetic retinopathy and its correlation with thyroid profile and anti thyroid antibodies. IOSR-JDMS. (2017) 16:96–8. doi: 10.9790/0853-1601079698
Keywords: thyroid dysfunction, diabetic retinopathy, Mendelian randomization, meta-analysis, gene
Citation: Ouyang J, Zhou L, Wang Q and Yan W (2024) Genetically mimicked effects of thyroid dysfunction on diabetic retinopathy risk: a 2-sample univariable and multivariable Mendelian randomization study. Front. Endocrinol. 15:1374254. doi: 10.3389/fendo.2024.1374254
Received: 21 January 2024; Accepted: 25 September 2024;
Published: 21 October 2024.
Edited by:
Mohd Imtiaz Nawaz, King Saud University, Saudi ArabiaReviewed by:
Viktor Kravchenko, National Academy of Sciences of Ukraine, UkraineCopyright © 2024 Ouyang, Zhou, Wang and Yan. This is an open-access article distributed under the terms of the Creative Commons Attribution License (CC BY). The use, distribution or reproduction in other forums is permitted, provided the original author(s) and the copyright owner(s) are credited and that the original publication in this journal is cited, in accordance with accepted academic practice. No use, distribution or reproduction is permitted which does not comply with these terms.
*Correspondence: Wei Yan, eWFud2VpX2psdUAxNjMuY29t
Disclaimer: All claims expressed in this article are solely those of the authors and do not necessarily represent those of their affiliated organizations, or those of the publisher, the editors and the reviewers. Any product that may be evaluated in this article or claim that may be made by its manufacturer is not guaranteed or endorsed by the publisher.
Research integrity at Frontiers
Learn more about the work of our research integrity team to safeguard the quality of each article we publish.