- 1Department of Thyroid Surgery, China-Japan Union Hospital of Jilin University, Changchun, Jilin, China
- 2Department of Otolaryngology Surgery, China-Japan Union Hospital of Jilin University, Changchun, Jilin, China
Objective: The unique metastatic pattern of skip lateral lymph node metastasis (SLLNM) in PTC patients may lead to missed diagnosis of lateral cervical metastatic lymph nodes. Therefore, many different SLLNM prediction models were constructed. In this study, partially eligible models (Hu 2020, Wang 2020, and Zhao 2023 nomograms) were selected for external validation, and then new variables were incorporated for model reconstruction to extend clinical applicability.
Methods: 576 PTC patients from our center were selected to evaluate the performance of the three nomograms using the receiver operating characteristic curve (ROC), calibration curves, and decision curve analyses (DCA). Three new variables were added to calibrate the model, including assessment of LN status on ultrasound (US-SLLNM), the distance from the tumor to the capsule (Capsular distance), and the number of central lymph node dissections (CLND number). Univariate and multivariate logistic regression analyses were used to screen independent predictors to reconstruct the model, and 1000 Bootstrap internal validations were performed.
Results: SLLNM were present in 69/576 patients (12.0%). In external validation, the area under the ROC curves (AUCs) for Hu 2020, Wang 2020, and Zhao 2023 nomograms were 0.695 (95% CI:0.633-0.766), 0.792 (95% CI=0.73-0.845), and 0.769 (95% CI:0.713-0.824), respectively. The calibration curves for the three models were overall poorly fitted; DCA showed some net clinical benefit. Model differentiation and net clinical benefit improved by adding three new variables. Based on multivariate analysis, female, age, and maximum tumor diameter ≤ 10 mm, located at the upper pole, Capsular distance < 0mm, US-SLLNM, CLND number ≤ 5 were identified as independent predictors of SLLNM and were used to construct the new model. After 1000 Bootstrap internal validations, the mean AUC of the model was 0.870 (95% CI:0.839-0.901), the calibration curve was close to the ideal curve, and the net clinical benefit was significant.
Conclusion: Overall, these nomograms were well differentiated and provided some net clinical benefit, but with varying degrees of underestimation or overestimation of the actual risk and high false-negative rates. New dynamic nomogram was constructed based on the addition of new variables and larger samples, showing better performance.
1 Introduction
Papillary thyroid carcinoma (PTC) is the most common thyroid malignancy, accounting for approximately 90% of all thyroid cancers (1). Generally, LNM of PTC occurs stepwise, first involving the central regional lymph nodes, then reaching the ipsilateral lateral cervical lymph nodes, and finally the contralateral lateral cervical and mediastinal compartments. However, some patients do not follow this pattern and develop lateral neck lymph node metastasis (LLNM) in the absence of central lymph node metastasis (CLNM), which is known as “skipping lateral lymph node metastasis (SLLNM)” (2, 3). This is not uncommon in clinical practice, and the frequency of skip metastases has been reported in the literature to range from 3.4% to 22.5% (2–17). Studies have shown that cervical lymph node metastasis increases the risk of local recurrence of PTC and decreases survival, especially in the lateral neck region (18–20). The prognosis of PTC patients with concomitant SLLNM was found to have a 10-year recurrence rate of 13% (20/151), which seriously affects patients’ quality of life (21).
However, prophylactic cervical lymph node dissection has been controversial. According to the 2015 version of the ATA guidelines and other studies, prophylactic central lymph node dissection is not recommended for patients with stage T1, T2, and noninvasive cN0 PTC; and prophylactic lateral lymph node dissection is not recommended if there is no preoperative evidence of lateral cervical lymph node metastasis as well as negative intraoperative central lymph nodes (22, 23). Ultrasound is one of the best methods for assessing the status of lymph nodes, but it is affected by complex anatomical structures, gas interference, and the subjective judgment of physicians, resulting in low sensitivity and high false-negative rates (24). Therefore, in-depth study of the pattern of SLLNM in patients with PTC and construction of relevant models are of great significance in guiding the treatment of patients, and it has been demonstrated that SLLNM in patients with PTC can be predicted (25).
At present, there are many constructed nomograms for predicting SLLNM. After rigorous screening (Table 1), three models of Hu 2020 (6), Wang 2020 (7) and Zhao 2023 (10) were selected. The original models showed good discrimination or consistency. Hu 2020, Wang 2020 were based on clinicopathological features, and Zhao 2023 was based on preoperative ultrasound features to construct prediction models, but the performance of the models was not externally verified. In this paper, a larger sample size will be used for external verification, and the evaluation of lymph node status under preoperative ultrasound (US-SLLNM) and the number of central lymph nodes dissected during operation (CLND number) will be used as one of the factors to explore. In addition, capsular invasion and ETE cannot be accurately judged under ultrasound. Therefore, the distance between the tumor and the capsule on ultrasound (Capsular distance) is used as another variable to explore new independent predictors, improve the predictive performance of the models, and provide help for the precise treatment of PTC patients.
2 Materials and methods
2.1 Research subjects
This study used a single-center retrospective analysis. Approval was obtained from the Research Ethics Committee (2023072602), and the requirement for informed consent was waived.
A total of 576 patients from the China-Japan Union Hospital of Jilin University from January 2020 to December 2022 were retrieved from the electronic medical record system. Inclusion criteria: (1) postoperative pathology confirmed as PTC (including papillary carcinoma, papillary adenocarcinoma, papillary carcinoma follicular subtype, micropapillary carcinoma); (2) First thyroid surgery with total thyroidectomy, central lymph node dissection and lateral cervical lymph node dissection; (3) Postoperative pathology confirmed the presence of LLNM; (4) No history of head and neck radiation exposure.
Exclusion criteria: (1) other types of thyroid cancer (including follicular thyroid cancer, medullary thyroid cancer, undifferentiated thyroid cancer, and high-risk subtypes of PTC such as diffuse sclerosis); (2) previous history of thyroid surgery; (3) PTC patients with tumors located in the isthmus or occupying most or even all of the glands (to better determine the effect of tumor location on SLLNM); (4) Postoperative pathology confirmed that there was no LLNM; (5) There were other malignant tumors; (6) History of head and neck radiotherapy.
2.2 Surgery treatment
In this study, all patients underwent total thyroidectomy, central lymph node dissection, and lateral cervical lymph node dissection. Central lymph node dissection ranged from superiorly by the hyoid bone, inferiorly to the sternal notch, and lateral to the carotid sheath, posteriorly to prevertebral fascia. Lateral lymph node dissection covers zones II, III, IV, and V. Zone I or VII dissection was performed only if evidence of metastasis was found on imaging, cytopathology, or intraoperatively. In our study, no patient underwent zone I and VII clearance. All enrolled patients had preoperative findings of suspected LLNM or were at high risk of LLNM based on clinician experience.
2.3 Data collection
Three nomograms used to predict SLLNM were considered in our study. For all included patients, parameters related to Hu 2020 nomogram were collected, such as age (> 55, ≤ 55), bilaterality under pathology, and location (upper pole, non-upper pole); Wang 2020 nomogram parameters such as age, pathological tumor size (≤ 10mm, > 10mm), location (upper, middle and lower poles); Zhao 2023 nomogram parameters such as age (> 40, ≤ 40), tumor size under ultrasound (< 9.1 mm, ≥ 9.1 mm), location (upper pole, non-upper pole), margin and extrathyroidal extension (ETE), as shown in Table 1. In addition to this, patient’s gender, BMI; ultrasound characteristics such as bilaterality, multifocality, calcification status, aspect ratio, and nodule blood supply; and pathologic characteristics such as bilaterality, multifocality, capsular invasion, ETE, Hashimoto’s thyroiditis (HT), nodular goiter (NG). In the above three models, the grouping criteria for some variables were inconsistent; for example, for multifocal, Hu 2020 specified ≥2 lesions in a unilateral lobe, and Wang 2020 and Zhao 2023 specified ≥2 PTC lesions in bilateral lobes; the grouping criteria for age, location, and size were also inconsistent. Here, capsular invasion refers to the tumor invading the thyroid capsule but not penetrating it. ETE is the tumor penetrating the capsule or invading the surrounding soft tissues and structures (26).
In addition, three variables, Capsular distance (Figure 1), US-SLNM, and intraoperative CLND number, were collected into the model to see whether there was a gain. US-SLLNM, referring to the 2013 European Thyroid Association guidelines (27), which divided lymph nodes into normal, uncertain, and suspected malignant; uncertain and suspicious malignancy on ultrasound was defined as suspicious lymph nodes; no abnormality was found in the central lymph nodes under ultrasound, and the suspicious cases of lateral cervical lymph nodes were positive.
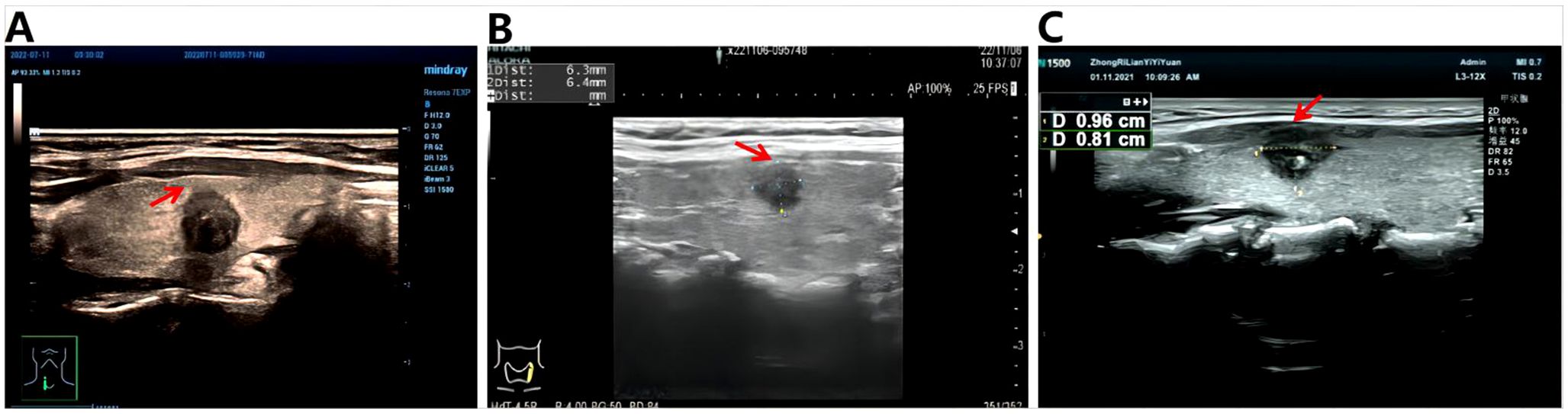
Figure 1. Schematic diagram of capsule distance. (A) >0mm, indicating that the tumor is completely within the gland; (B) =0mm, indicating that the tumor is close to the capsule; (C) <0mm, which means the tumor breaks through the capsule.
Hu 2020, Wang 2020 and Zhao 2023 nomograms are shown in the Supplementary Figures.
2.4 statistical analysis
Statistical analysis was performed using R software 4.3.0 and SPSS 26.0 software. Categorical variables were described as frequencies and percentages; continuous variables were expressed as means and standard deviations. The predictive performance of original nomograms was assessed by area under the ROC curve (AUC), calibration curves, and decision curve analysis (DCA). AUC values quantified the differentiation of the external validation cohort across the three nomograms; calibration curves were used to assess the agreement between predicted and actual SLLNM risk; and DCA was used to visualize the net benefit of the three nomograms. Taking SLLNM or not as the dependent variable, the predicted probability of the original nomogram and Capsular distance, US-SLLNM, and CLND number as the independent variables, logistic regression was used to incorporate each of the three new variables into the original nomograms and to plot the ROC curves and the DCA in order to compare the predictive performance of the original model to the model with the addition of the new variables. Next, univariate and multivariate logistic regression analyses were performed based on larger sample sizes to screen for stable independent predictors and construct a dynamic nomogram, with values of P<0.05 considered statistically significantly different. Internal validation was performed using 1000 Bootstrap, ROC curves, and calibration curves were used to evaluate the predictive accuracy and consistency of the model, and DCA reflected the net benefit of the model to patients. The original models were all static nomograms, and the regression parameters of the models were not provided, so we used an approximation, which is also the only approach used clinically. We placed the information of each patient into three original models to obtain the corresponding total score and predicted probability. Flow chart of the entire experiment is shown in Figure 2.
3 Results
3.1 Baseline characteristics
In the external cohort, the incidence of SLLNM was 12.0%, while the incidence of SLLNM was 9.7%, 11.6%, and 15.4% in Hu 2020 (Model 1), Wang 2020 (Model 2), and Zhao 2023 (Model 3), respectively. The baseline characteristics of the external validation cohort and the original cohort of the three models are shown in Table 2.
3.2 Risk assessment of the original nomograms
None of the original models provided the optimal cutoff value; therefore, in the external validation queue, the cutoff value when maximizing the Yoden index was selected based on the ROC curves of each model (Figure 3). From the ROC curves of the three models, it is easy to see that, although they are all reduced to a certain extent compared with the original models, they also all show a better degree of differentiation, see Tables 3, 4.
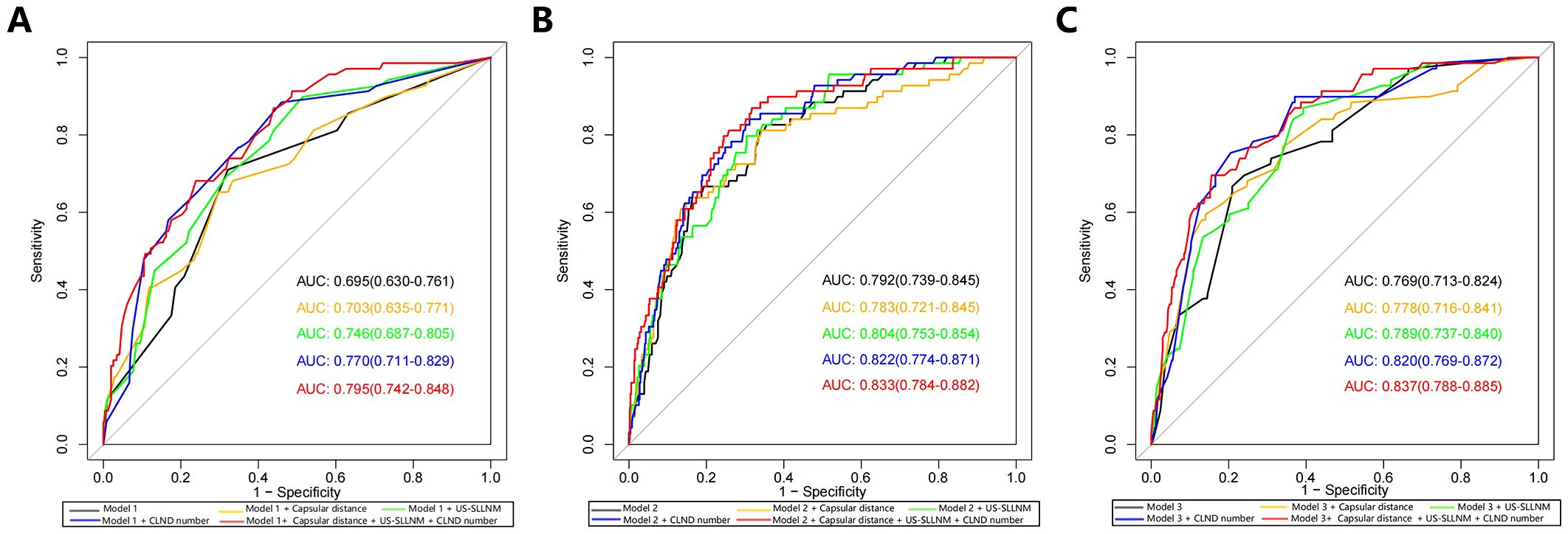
Figure 3. ROC curves (A) Model 1; (B) Model 2; (C) Model 3. AUCs from top to bottom are original model, model with added Capsular distance, model with added US-SLLNM, model with added CLND number, model with added three factors. ROC, receiver operating characteristic curve; AUC, Area under the curve; CLND, Central lymph node dissection.
Model 1: The optimal cutoff value for this nomogram was 8.2% (AUC = 0.695, 95CI%: 0.630-0.761), and sensitivity and specificity associated with the optimal threshold were 71.0% and 67.9%, respectively. Applying this cutoff value, 29.0% (20/69) of SLLNM-positive patients would be below the threshold, would be incorrectly judged to be at low risk of developing SLLNM, and would be advised against the need for lateral cervical lymph node dissection.
Model 2: The optimal cutoff value of this nomogram was 26.4% (AUC = 0.792, 95CI%: 0.739-0.845), and sensitivity and specificity associated with the optimal threshold were 63.8% and 83.6%, respectively. Applying this cutoff value, 36.2% (25/69) of patients would have escaped positive screening.
Model 3: The optimal cutoff value for this nomogram was 31.7% (AUC = 0.769, 95CI%: 0.713-0.824), and sensitivity and specificity associated with the optimal threshold were 66.7%, respectively, 79.1%. Applying this cutoff value, 33.3% (23/69) of patients would miss positive screening. We believe that this non-negligible percentage of patients is too high and that this finding represents a major limitation of the original nomograms.
3.3 Performance of the original nomograms
The calibration curves for Model 1, Model 2, and Model 3 are shown in Figure 4. Overall, the three original models passed the Hosmer-Lemeshow goodness-of-fit test, which showed that the models all fit poorly (P < 0.05.) Model 1 was more consistent at predicted probabilities of 15.5% to 24.5% and underestimated the risk of SLLNM in the current cohort at predicted probabilities of 6.5% to 15.5% and >24.5% (Figure 4A). With Model 2, there is more agreement with actual risk at predicted probabilities <35%; however, when larger predicted probabilities (>35%) occur, there tends to be an overestimation of observed risk (Figure 4B). Model 3, on the other hand, has a degree of overestimation over the entire range of predicted probabilities (Figure 4C). The current incidence of SLLNM ranges from 3.4% to 22.5%, as described in the relevant literature (2–17). Thus, in the lower-risk patient cohort, Model 1 underestimates the actual risk to some extent, Model 2 is more consistent with the actual risk, and Model 3 overestimates the actual risk.
The DCA of Model 1 showed that the threshold probability was between 6.5% -20% and 22% -56%, with a certain clinical net benefit. The optimal Cutoff value of this nomogram is 8.2%, and the use of which will bring a net clinical benefit of 5.5% (Figure 5A). The DCA of Model 2 and Mode 3 showed a net clinical benefit when the threshold probabilities were 17.9% -36.3% and 4.1% -37%, respectively (Figures 5B, C). When the respective optimal Cutoff values are taken, there is also a certain clinical net benefit, indicating that the use of this nomogram has clinical significance.
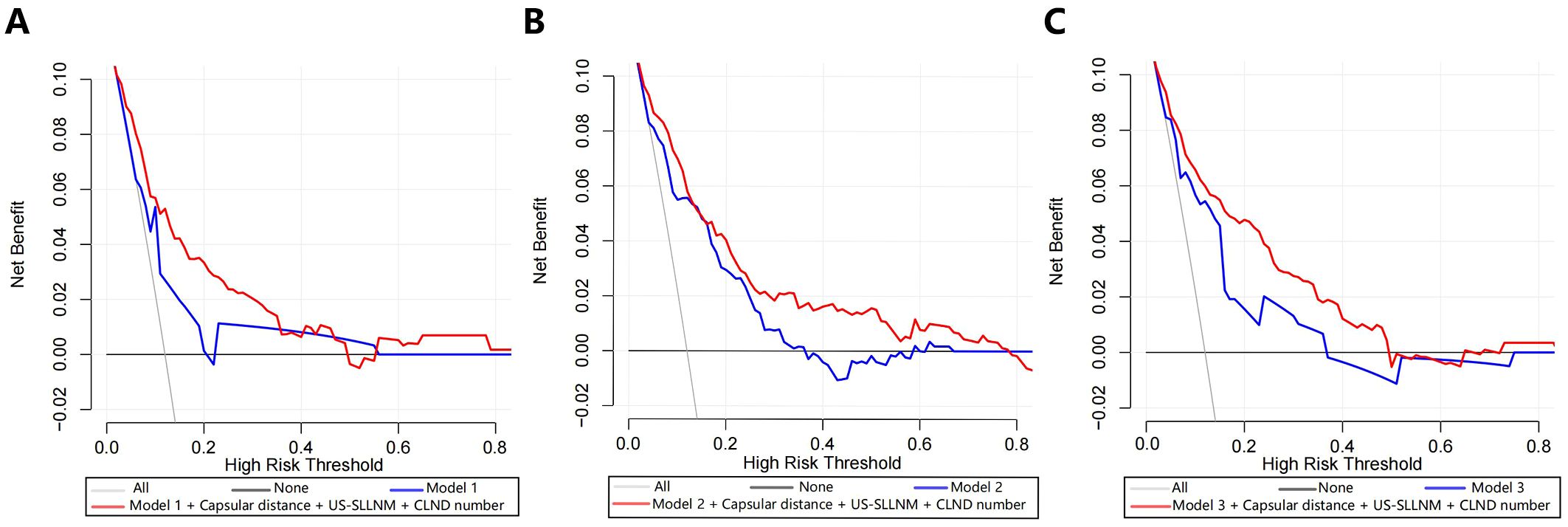
Figure 5. Decision curve analyses (including original model, model with added three factors). (A) Model 1; (B) Model 2; (C) Model 3.
3.4 Performance of nomograms after adding new variables
Three new variables, Capsular distance, US-SLLNM, and CLND number, were included in this study. The ROC curve analysis of the CLND number showed that the AUC was 0.713 (0.643-0.783) (Figure 6A). In order to divide its optimal cut-point, this study selected the cutoff when the Yoden index is at its maximum, which is 5 (Figure 6B). As can be seen from Figure 3, except for the Capsular distance in Model 2, which did not increase the AUC (Figure 3B), the remaining two variables increased the AUC to varying degrees in all three original models. Comparison of AUCs for the original cohort, the external cohort, and the cohort calibrated with the added variables is shown in Table 4. The DCA in Figure 5 shows that the model with the addition of the three variables resulted in a significant increase in both the risk threshold range and the net benefit compared to the original model.
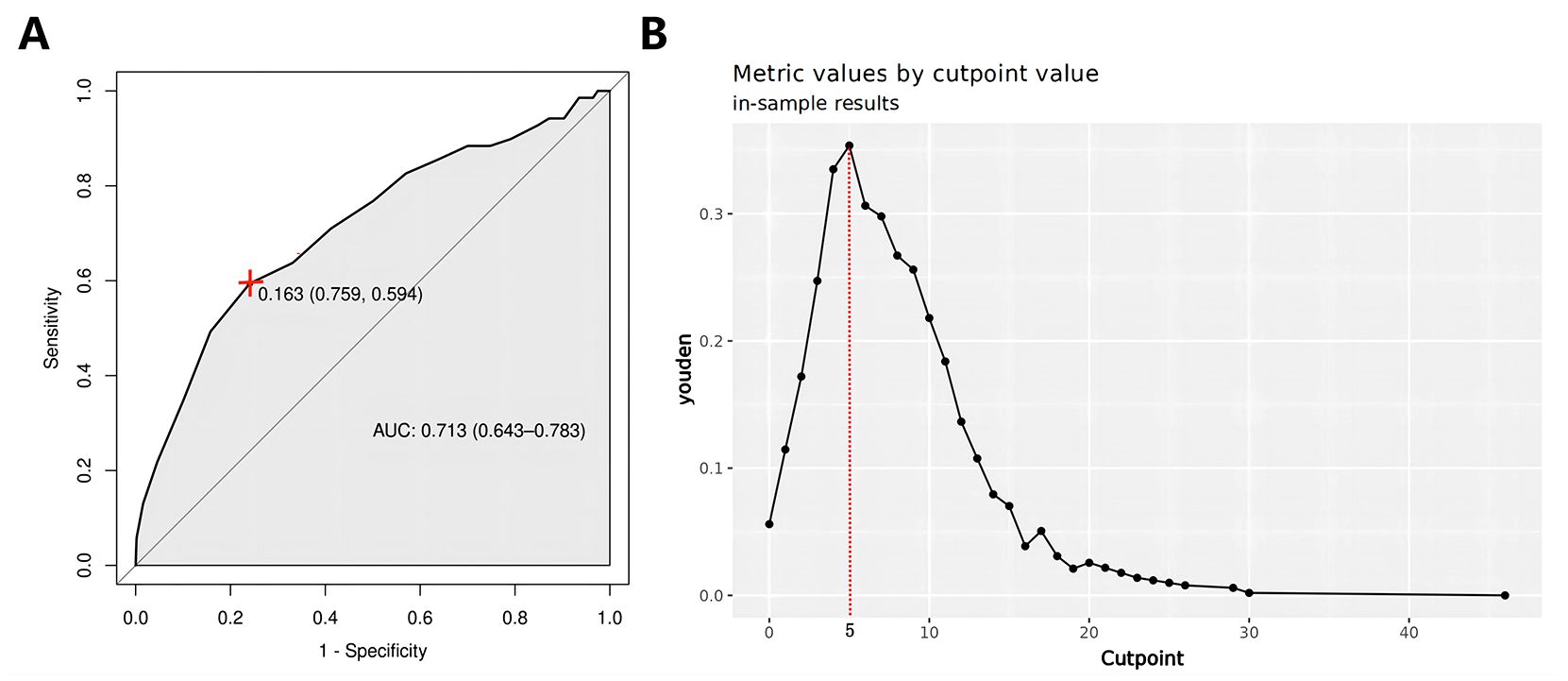
Figure 6. (A) CLND number ROC curve; (B) CLND number Optimal cut-point plot. ROC, receiver operating characteristic curve; CLND, central lymph node dissections.
4 Model construction
4.1 Risk factors for cervical SLLNM in PTC patients
Based on the gain brought by the new variables to the model, a larger sample size (576 cases) will be used this time to explore the influences of previous studies again, in order to construct a model with higher performance. The sample sizes for the three nomograms constructed above were 521, 378, and 267, respectively. In the univariate logistic regression analysis, it was found that female (P=0.014); age (P<0.001); BMI ≥25Kg/m2 (P=0.030); upper pole (P<0.001); maximal diameter of the tumor ≤10mm (P=0.009); Capsular distance < 0mm (P=0.006); US-SLLNM (P<0.001); and CLND number ≤ 5 (P<0.001) were significantly associated with SLLNM in PTC patients (P<0.05). On the contrary, the differences in margins, aspect ratio, nodule blood supply, calcification, bilaterality, multifocality, capsular invasion, ETE, HT, and NG were not statistically significant between the SLLNM (+) group and the SLLNM (-) group (all P>0.05). The results of univariate logistic regression analysis are shown in Table 5.
4.2 Independent risk factors for cervical SLLNM in PTC patients
The eight variables that were statistically different from those mentioned above were included in the multivariate logistic regression, and the results showed that female (OR 2.740, 95%CI 1.279-5.870, P=0.009), age (OR 1.061, 95%CI 1.031-1.091, P<0.001), tumor located in the upper pole (OR 3.427, 95%CI 1.749- 6.715, P < 0.001), maximum tumor diameter ≤ 10 mm (OR 2.544, 95%CI 1.360-4.758, P = 0.003), Capsular distance < 0 mm (OR 3.287, 95%CI 1.217-8.875, P = 0.019), US-SLLNM (OR 3.009, 95%CI 1.635-5.536, P<0.001), and CLND number ≤5 (OR 3.244, 95%CI 1.766-5.959, P<0.001) were independent risk factors for cervical SLLNM in PTC patients. The results of the multivariate logistic regression analysis are shown in Table 6.
4.3 Construction and validation of the nomogram
Based on the seven independent risk factors described above, the logistic regression equation of the resulting model is as follows: Y= -7.893 + 1.119(χ1)+ 0.060(χ2) - 0.298(χ4-2) + 1.239(χ4-3) + 0.978(χ5) + 0.613(χ6-2) + 1.203(χ6-3) + 1.053(χ7) + 1.173(χ8) (χ1:Female; χ2:Age value; χ4-2: Lower; χ4-3:Upper; χ5:Maximum diameter ≤ 10mm; χ6-2:Capsular distance=0mm; χ6-3:Capsular distance<0mm; χ7:US-SLLNM(Yes); χ8:CLND number ≤ 5).
Apart from this, static (Figure 7) and dynamic nomograms were constructed respectively: https://thyroidnomo.shinyapps.io/dynnomapp.
The new model had an AUC value of 0.869 (95% CI:0.833-0.906), with a sensitivity of 89.9% and a specificity of 69.4%. In order to evaluate the ability of our nomogram to predict SLLNM in PTC patients, we used 1,000 bootstrap resamplings for internal validation and obtained a mean AUC value of 0.870 (95% CI:0.839-0.901), showing good discrimination (Figure 8A); the calibration curve was also close to the ideal curve, with good consistency (Figure 8B). On the DCA curve, the net clinical benefit was seen from the 0 - 62% prediction probability interval (Figure 8C). According to the ROC curve, 7.9% is the optimal critical value of the model. When the prediction probability is 7.9%, a clinically significant net benefit can be obtained. Moreover, 89.9% (62/69) of PTC patients were correctly screened for SLLNM, which is of great significance in guiding the treatment of patients.
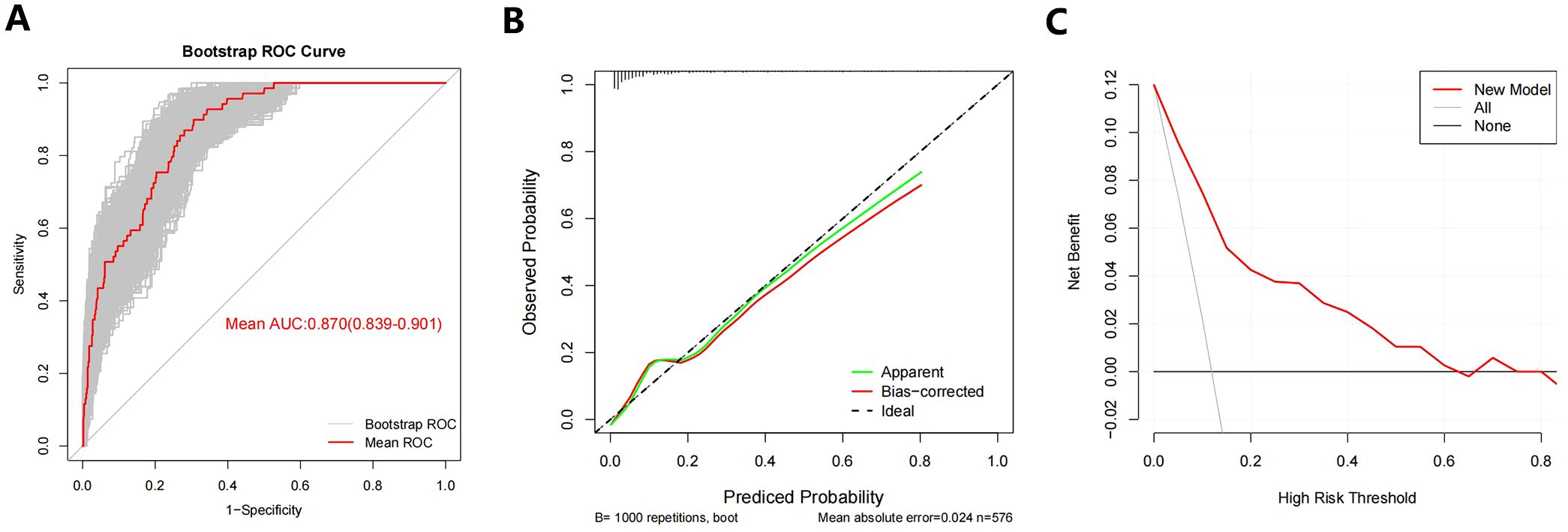
Figure 8. (A) Bootstrap ROC Curve; (B) Bootstrap Calibration Curve; (C) Bootstrap Decision curve analyses. ROC= receiver operating characteristic curve.
5 Discussion
In our external cohort, we evaluated the performance of three models for predicting SLLNM in PTC patients (Hu 2020 (6), Wang 2020 (7), and Zhao 2023 (10) nomograms). In the ROC curve analysis, the AUCs were all lower than the original models but also demonstrated good discrimination. However, when the Cutoff value at maximization of the Yoden index was applied to each of the three models, 29.0%, 36.2%, and 33.3% of SLLNM-positive patients, respectively, would be below the threshold, considered to be at low risk of SLLNM, and would not be considered for lymph node dissection of the lateral neck, which demonstrated a major limitation of the original models. As shown by the calibration curves (Figure 4), the original models fitted poorly overall in our external validation cohort. In the lower-risk patient cohort, the Hu 2020 nomogram (6) underestimated the actual risk to some extent, making it likely that SLLNM was present in patients expected to be at low risk. The Wang 2020 nomogram (7) was more consistent with the actual risk in the patient cohort with lower expected risk. In contrast, the Zhao 2023 nomogram (10) overestimated the actual risk, allowing more SLLNM-negative patients to receive intervention. The DCA curves for all three original models also show some net clinical benefit at the lower threshold probability intervals (Figure 5). These nomograms did not perform as well as the original cohort in our external cohort, probably because the baseline characteristics of the original cohort differed somewhat from our baseline characteristics. The difference in baseline characteristics may come from some subjective factors of tumor ultrasound characteristics and pathological characteristics, such as the judgment of tumor edge, ETE, etc. Furthermore, with advances in technology, fine-needle aspiration (FNA) of the lateral cervical lymph nodes is performed preoperatively to assess lymph node status. More precise clearance of metastatic lateral cervical lymph nodes has resulted in a higher incidence of LLNM in the study population, and there may be variations among centers in the timing and extent of FNA application, which may lead to bias in the selection of the populations in each cohort. In addition, surgeons’ subjective judgments of high risk for LLNM may be inconsistent across centers, which will also contribute to selection bias in the populations in each cohort.
To further refine the models, we took the approach of adding relevant risk factors by incorporating the results of Capsular distance, US-SLLNM, and CLND number into the original models, respectively (Figure 3). It can be seen that the performance of the models has been improved except for the Capsular distance which has no gain on the basis of Wang 2020.When all three risk factors were included in the three original models, the AUC increased significantly and exceeded that of the original cohort. As can be seen from the DCA curves in Figure 5, where the addition of the three factors significantly increased the range of threshold probabilities under the net benefit, as well as the percentage of net benefit under the same threshold probability. This means that these three factors can be included in the model to predict the outcome of SLLNM more accurately. Based on the results obtained above, we would like to explore further the relevant influencing factors involved in the above study by using a larger sample size to seek more stable independent predictors and construct a new model to identify PTC patients with SLLNM more accurately.
Expectedly, in our multivariate analysis, the three newly added variables, Capsular distance <0 mm, US-SLLNM, and CLND number ≤5 were all independent risk factors for SLLNM. The risk of SLLNM in patients with Capsular distance < 0mm was about 3.287 times higher than that in patients with Capsular distance > 0mm (OR 3.287, P = 0.019). In fact, this proves the study of Zhao 2023 that ETE under ultrasound is an independent risk factor for SLLNM (10). Furthermore, we could not precisely determine ETE under ultrasound and only assess it by envelope discontinuity. Therefore, we used a new variable, Capsular distance, to explore the effect on SLLNM. US-SLLNM incorporates the judgment of ultrasound on lymph nodes into the model. Even though ultrasound has low diagnostic efficacy for cervical lymph nodes, especially for the screening of central lymph nodes, ultrasound is routinely used as a preoperative examination in PTC patients, and it is still of certain value for the diagnosis of lymph nodes (28). The present ultrasound diagnosis of the SLLNM had a sensitivity of 59.4%, a specificity of 68.2%, and an accuracy of 67.2%. In this study, the CLND number was divided into cutoff values, and it was found that the risk of SLLNM in CLND ≤ 5 was approximately 2.244 times higher compared to CLND > 5 (OR 3.244, P < 0.001). This is consistent with the study of Zhu et al. (8), which also found that the reduction of CLND number was an independent risk factor for SLLNM, and the incidence of SLLNM was negatively correlated with CLND number (P < 0.05) (2, 10).
In addition, age, tumor location, and size were significantly associated with the risk of SLLNM, consistent with previous studies. The older the age, the higher the risk of SLLNM (6, 7, 14), but there is no consistency regarding the cutoff for age. Some studies have suggested 40 years of age (10, 25), while others have suggested 45 years of age as the cutoff for SLLNM (2, 29); Hu et al. and Dou et al. found that age >55 years was an independent risk factor for SLLNM by multivariate analysis (6, 15). Tumor size is generally defined as a cutoff point of 10 mm, and microcarcinomas ≤10 mm are considered more likely to develop SLLNM (2–5, 7, 8, 11, 13, 29). One study even suggested that SLLNM was more common when the primary tumor size ≤0.5 cm (OR = 12.9, P = 0.001) (12). This suggests that skip metastases may be in the early stages of cancer (25). However, Yang et al. (9) concluded that ≥10 mm was an independent risk factor for SLLNM, which may be because a larger proportion of SLLNM patients with larger tumors were located in the upper pole of the thyroid gland in that study, and the effects of tumor location and size were not distinguished, and the incidence of skip metastasis was only 3.4% in that study, which may have been a bias during the statistical analysis. Active surveillance has now become an alternative strategic choice for patients with micro PTC, requiring clinicians to carefully assess lateral cervical lymph node status. Numerous studies have shown a strong correlation with the development of SLLNM when the tumor is located in the upper pole (3–6, 8, 9, 14, 15, 17, 29–31). The same explanation is given: the upper pole of the thyroid lobe has a different lymphatic drainage system than the rest. Lymphatic drainage through the superior thyroid artery is transferred to the lateral lymph nodes (5, 7, 10, 32), and it is suggested that the lateral lymph nodes may be the first lymphatic drainage stations for tumors of the upper pole (15).
In this study, females became an independent risk factor for SLLNM (OR 2.740, 95% CI 1.279-5.870, P=0.009), which is in line with the studies of Zhao et al. and Jiwang et al. (2, 17), which also suggested that the female gender (OR 2.29, 95%CI 1.02-5.16) may contribute to an increased risk of SLLNM, again based on a larger sample. However, some studies concluded that gender was not significantly associated with SLLNM (3, 6–10), which may be due to inconsistencies in the inclusion criteria as well as grouping criteria for patients. In addition, the ratio of female to male patients in skip metastasis may also contribute to the heterogeneity between studies (3).
In summary, the model was also established to corroborate the findings of numerous studies and furthermore to summarize the reasons for the occurrence of SLLNM. It is more accepted that skip metastasis seems to develop by bypassing the normal lymphatic system of the central lymph nodes (5, 11, 16, 17) and is more commonly found in the lateral cervical zone III and zone II (4, 5, 11, 30). Using this model, it is possible to identify patients with PTC who are at high risk for SLLNM, for which we should carefully evaluate the lateral cervical lymph nodes and appropriately perform FNA of the lateral cervical lymph nodes or prophylactic lateral cervical lymph node dissection.
Our study is not without limitations. First, this study is a retrospective, single-center study, which may lead to selection and information bias. Second, in our external validation, we used approximate predictive probabilities because we did not obtain the regression parameters of the original models, and the validation results may have certain errors, but this is also a way to be close to the clinic. Further, the assessment of ultrasound characteristics involves some subjectivity, which means that observational differences between sonographers may be a factor affecting the results of external validation. In addition, the new variables we included and the reconstructed model need to be further validated in multi-center studies with larger samples.
6 Conclusion
We validated the predictive models of Hu 2020, Wang 2020, and Zhao 2023, and these nomograms showed good discrimination and some clinical benefit, but with varying degrees of underestimation or overestimation of the actual risk, and high false-negative rates. Besides, the added new variables all showed good gains in the original models, and the new dynamic nomogram was further constructed based on large samples, showing better performance.
Data availability statement
The original contributions presented in the study are included in the article/Supplementary Material. Further inquiries can be directed to the corresponding author.
Ethics statement
The studies involving humans were approved by The Research Ethics Committee of China-Japan Union Hospital of Jilin University. The studies were conducted in accordance with the local legislation and institutional requirements. The ethics committee/institutional review board waived the requirement of written informed consent for participation from the participants or the participants’ legal guardians/next of kin because This study does not involve interventions for patients, only relevant data was collected.
Author contributions
QL: Writing – review & editing, Writing – original draft, Validation, Software, Methodology, Investigation, Data curation. CH: Writing – review & editing, Validation, Investigation. HZ: Writing – review & editing, Validation, Investigation. JZ: Writing – review & editing, Validation. JX: Writing – review & editing, Supervision, Funding acquisition, Conceptualization.
Funding
The author(s) declare financial support was received for the research, authorship, and/or publication of this article. This study was funded by the Jilin Province Direct Health Special Project, Grant No. Sczsy201605.
Acknowledgments
The authors would like to thank Director Sun Hui for her support of this study and all the patients who participated in this study.
Conflict of interest
The authors declare that the research was conducted in the absence of any commercial or financial relationships that could be construed as a potential conflict of interest.
Publisher’s note
All claims expressed in this article are solely those of the authors and do not necessarily represent those of their affiliated organizations, or those of the publisher, the editors and the reviewers. Any product that may be evaluated in this article, or claim that may be made by its manufacturer, is not guaranteed or endorsed by the publisher.
Supplementary material
The Supplementary Material for this article can be found online at: https://www.frontiersin.org/articles/10.3389/fendo.2024.1366679/full#supplementary-material
References
1. Health Commission Of The People’s Republic Of China N. National guidelines for diagnosis and treatment of thyroid cancer 2022 in China (English version). Chin J Cancer Res. (2022) 34:131–50. doi: 10.21147/j.issn.1000-9604.2022.03.01
2. Zhao H, Huang T, Li H. Risk factors for skip metastasis and lateral lymph node metastasis of papillary thyroid cancer. Surgery. (2019) 166:55–60. doi: 10.1016/j.surg.2019.01.025
3. Lei J, Zhong J, Jiang K, Li Z, Gong R, Zhu J. Skip lateral lymph node metastasis leaping over the central neck compartment in papillary thyroid carcinoma. Oncotarget. (2017) 8:27022–33. doi: 10.18632/oncotarget.15388
4. Park JH, Lee YS, Kim BW, Chang HS, Park CS. Skip lateral neck node metastases in papillary thyroid carcinoma. World J Surg. (2012) 36:743–7. doi: 10.1007/s00268-012-1476-5
5. Qiu Y, Fei Y, Liu J, Liu C, He X, Zhu N, et al. Prevalence, risk factors and location of skip metastasis in papillary thyroid carcinoma: A systematic review and meta-analysis. Cancer Manag Res. (2019) 11:8721–30. doi: 10.2147/cmar.S200628
6. Hu D, Lin H, Zeng X, Wang T, Deng J, Su X. Risk factors for and prediction model of skip metastasis to lateral lymph nodes in papillary thyroid carcinoma. World J Surg. (2020) 44:1498–505. doi: 10.1007/s00268-019-05332-0
7. Wang W, Yang Z, Ouyang Q. A nomogram to predict skip metastasis in papillary thyroid cancer. World J Surg Oncol. (2020) 18:167. doi: 10.1186/s12957-020-01948-y
8. Zhu S, Wang Q, Zheng D, Zhu L, Zhou Z, Xu S, et al. A novel and effective model to predict skip metastasis in papillary thyroid carcinoma based on a support vector machine. Front Endocrinol (Lausanne). (2022) 13:916121. doi: 10.3389/fendo.2022.916121
9. Yang Z, Heng Y, Zhao Q, Cao Z, Tao L, Qiu W, et al. A specific predicting model for screening skip metastasis from patients with negative central lymph nodes metastasis in papillary thyroid cancer. Front Endocrinol (Lausanne). (2021) 12:743900. doi: 10.3389/fendo.2021.743900
10. Zhao M, Shi X, Zou Z, Wen R, Lu Y, Li J, et al. Predicting skip metastasis in lateral lymph nodes of papillary thyroid carcinoma based on clinical and ultrasound features. Front Endocrinol (Lausanne). (2023) 14:1151505. doi: 10.3389/fendo.2023.1151505
11. Feng JW, Qin AC, Ye J, Pan H, Jiang Y, Qu Z. Predictive factors for lateral lymph node metastasis and skip metastasis in papillary thyroid carcinoma. Endocr Pathol. (2020) 31:67–76. doi: 10.1007/s12022-019-09599-w
12. Nie X, Tan Z, Ge M. Skip metastasis in papillary thyroid carcinoma is difficult to predict in clinical practice. BMC Cancer. (2017) 17:702. doi: 10.1186/s12885-017-3698-2
13. Ryu YJ, Kwon SY, Lim SY, Na YM, Park MH. Predictive factors for skip lymph node metastasis and their implication on recurrence in papillary thyroid carcinoma. Biomedicines. (2022) 10:179. doi: 10.3390/biomedicines10010179
14. Hou J, Zhang Y, Fan Y, Wu B. Risk factors of skip lateral lymph node metastasis in papillary thyroid carcinoma. Eur Arch Otorhinolaryngol. (2021) 278:493–8. doi: 10.1007/s00405-020-06176-4
15. Dou Y, Hu D, Chen Y, Xiong W, Xiao Q, Su X. PTC located in the upper pole is more prone to lateral lymph node metastasis and skip metastasis. World J Surg Oncol. (2020) 18:188. doi: 10.1186/s12957-020-01965-x
16. Lim YC, Koo BS. Predictive factors of skip metastases to lateral neck compartment leaping central neck compartment in papillary thyroid carcinoma. Oral Oncol. (2012) 48:262–5. doi: 10.1016/j.oraloncology.2011.10.006
17. Jiwang L, Jinghui B, Fengqin F, Tao Y, Yuejiao Z. Comprehensive analysis of clinicopathologic and sonographic features in thyroid cancer with skip lymph node metastasis: establish and assessment of a prediction nomogram. Braz J Otorhinolaryngol. (2023) 89:101301. doi: 10.1016/j.bjorl.2023.101301
18. Sapuppo G, Palermo F, Russo M, Tavarelli M, Masucci R, Squatrito S, et al. Latero-cervical lymph node metastases (N1b) represent an additional risk factor for papillary thyroid cancer outcome. J Endocrinol Invest. (2017) 40:1355–63. doi: 10.1007/s40618-017-0714-y
19. Zhang J, Cheng X, Shen L, Wang X, Wang L, Sun X, et al. The association between lymph node stage and clinical prognosis in thyroid cancer. Front Endocrinol (Lausanne). (2020) 11:90. doi: 10.3389/fendo.2020.00090
20. Amit M, Tam S, Boonsripitayanon M, Cabanillas ME, Busaidy NL, Grubbs EG, et al. Association of lymph node density with survival of patients with papillary thyroid cancer. JAMA Otolaryngol Head Neck Surg. (2018) 144:108–14. doi: 10.1001/jamaoto.2017.2416
21. Bertin JB, Buffet C, Leenhardt L, Menegaux F, Chereau N. Effect of skip metastasis to lateral neck lymph nodes on outcome of patients with papillary thyroid carcinoma. Langenbecks Arch Surg. (2022) 407:3025–30. doi: 10.1007/s00423-022-02604-7
22. Haugen BR, Alexander EK, Bible KC, Doherty GM, Mandel SJ, Nikiforov YE, et al. 2015 American thyroid association management guidelines for adult patients with thyroid nodules and differentiated thyroid cancer: the American thyroid association guidelines task force on thyroid nodules and differentiated thyroid cancer. Thyroid. (2016) 26:1–133. doi: 10.1089/thy.2015.0020
23. Lo CY. Lymph node dissection for papillary thyroid carcinoma. Methods Mol Biol. (2022) 2534:57–78. doi: 10.1007/978-1-0716-2505-7_5
24. Zhou L, Yao J, Ou D, Li M, Lei Z, Wang L, et al. A multi-institutional study of association of sonographic characteristics with cervical lymph node metastasis in unifocal papillary thyroid carcinoma. Front Endocrinol (Lausanne). (2022) 13:965241. doi: 10.3389/fendo.2022.965241
25. Shadi A, Mohamed A. ‘Skip metastases of papillary thyroid cancer’: Is it predictable? Eur J Surg Oncol. (2023) 49:e144–e. doi: 10.1016/J.EJSO.2022.11.405
26. Tuttle RM, Haugen B, Perrier ND. Updated American joint committee on cancer/tumor-node-metastasis staging system for differentiated and anaplastic thyroid cancer (Eighth edition): what changed and why? Thyroid. (2017) 27:751–6. doi: 10.1089/thy.2017.0102
27. Leenhardt L, Erdogan MF, Hegedus L, Mandel SJ, Paschke R, Rago T, et al. 2013 European thyroid association guidelines for cervical ultrasound scan and ultrasound-guided techniques in the postoperative management of patients with thyroid cancer. Eur Thyroid J. (2013) 2:147–59. doi: 10.1159/000354537
28. Yao F, Yang Z, Li Y, Chen W, Wu T, Peng J, et al. Real-world evidence on the sensitivity of preoperative ultrasound in evaluating central lymph node metastasis of papillary thyroid carcinoma. Front Endocrinol (Lausanne). (2022) 13:865911. doi: 10.3389/fendo.2022.865911
29. Zhan X, Xue S, Yin Y, Chen G. [Related factors on skip metastasis of neck lymph node in papillary thyroid carcinoma]. Zhonghua Wai Ke Za Zhi. (2017) 55:599–602. doi: 10.3760/cma.j.issn.0529-5815.2017.08.009
30. Lee YS, Shin SC, Lim YS, Lee JC, Wang SG, Son SM, et al. Tumor location-dependent skip lateral cervical lymph node metastasis in papillary thyroid cancer. Head Neck. (2014) 36:887–91. doi: 10.1002/hed.23391
31. Graceffa G, Orlando G, Cocorullo G, Mazzola S, Vitale I, Proclamà MP, et al. Predictors of central compartment involvement in patients with positive lateral cervical lymph nodes according to clinical and/or ultrasound evaluation. J Clin Med. (2021) 10:3407. doi: 10.3390/jcm10153407
Keywords: papillary thyroid carcinoma, skip lateral lymph node metastasis, external validation, nomogram, internal validation
Citation: Li Q, Huang C, Zou H, Zhang J and Xin J (2024) Single-center external validation and reconstruction of multiple predictive models for skip lateral lymph node metastasis in papillary thyroid carcinoma. Front. Endocrinol. 15:1366679. doi: 10.3389/fendo.2024.1366679
Received: 07 January 2024; Accepted: 05 August 2024;
Published: 23 August 2024.
Edited by:
David Viola, University of Pisa, ItalyReviewed by:
Pia Pace-Asciak, University of Toronto, CanadaRui Liu, Hunan Provincial People’s Hospital, China
Ludovico Docimo, University of Campania Luigi Vanvitelli, Italy
Copyright © 2024 Li, Huang, Zou, Zhang and Xin. This is an open-access article distributed under the terms of the Creative Commons Attribution License (CC BY). The use, distribution or reproduction in other forums is permitted, provided the original author(s) and the copyright owner(s) are credited and that the original publication in this journal is cited, in accordance with accepted academic practice. No use, distribution or reproduction is permitted which does not comply with these terms.
*Correspondence: Jingwei Xin, eGluandAamx1LmVkdS5jbg==