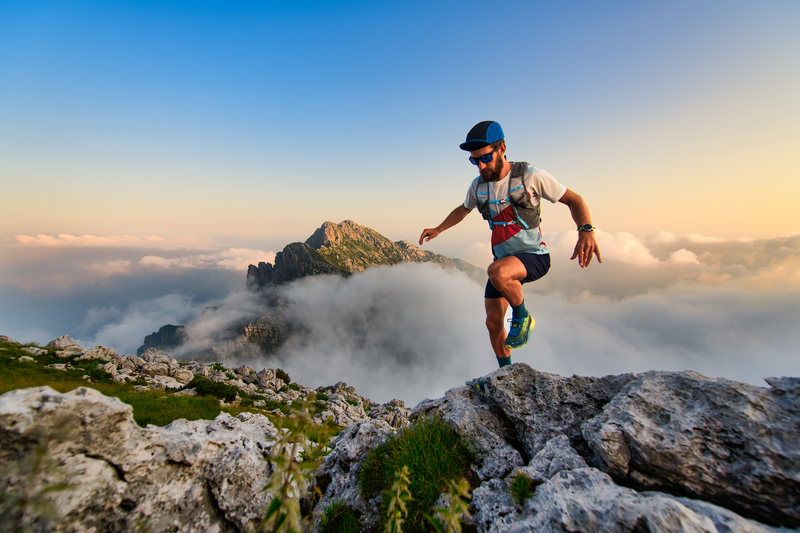
94% of researchers rate our articles as excellent or good
Learn more about the work of our research integrity team to safeguard the quality of each article we publish.
Find out more
ORIGINAL RESEARCH article
Front. Endocrinol. , 08 July 2024
Sec. Diabetes: Molecular Mechanisms
Volume 15 - 2024 | https://doi.org/10.3389/fendo.2024.1363757
This article is part of the Research Topic Novel Insights into the Pathophysiology of Diabetes-related Complications: Implications for Improved Therapeutic Strategies, Volume II View all 38 articles
Background: Diabetes mellitus (DM) is a global public health problem characterized by an elevated blood glucose level. Monitoring blood sugar levels is vital for effective diabetes management and preventing complications. However, the association between longitudinal biomarkers and the incidence of diabetic complications is often overlooked. Therefore, this study aimed to assess the incidence of diabetic retinopathy, predictors, and association with longitudinal fasting blood sugar level changes among diabetes mellitus patients in Ethiopia.
Methods: A multicenter retrospective follow-up study was carried out in referral hospitals in Amhara region, Ethiopia. A random sample of 462 newly diagnosed DM patients was selected. The proportional hazard assumption was checked for the survival sub-model, and for the longitudinal sub-model, the normality assumption was checked. Then the joint modeling with time-dependent lagged parameterizations was fitted. Model assumptions and comparisons were checked. Finally, the hazard ratio with a 95% confidence interval (CI) with a corresponding P-value<0.05 was used to identify predictors.
Results: In this study, Overall, 54 patients developed DR, and the incidence rate was 2.33 per 1000 person-months over the follow-up period, with a 95% CI of [1.78, 3.05]. Rural residence (AHR = 2.21, 95% CI: [1.21, 4.05]), hypertension co-morbidity (AHR = 3.01, 95% CI: [1.85, 6.53]), and longer duration of DM (>5 years) (AHR = 2.28, 95% CI: [1.91, 5.15]) were important predictors for the incidence of DR. In addition, the incidence of DR was substantially correlated with the time-dependent lagged value of FBS change (AHR = 4.20, 95% CI [1.62, 10.85]).
Conclusions: In this study, the incidence of diabetic retinopathy was somewhat high when compared to prior similar studies in Ethiopia. A joint model of longitudinal fasting blood sugar level changes was significantly associated with an increased risk of DR. Besides, being rural residence, hypertension co-morbidity, and a longer duration of DM were significant predictors for the incidence of DR. Therefore, public awareness, an integrated care approach, and prioritizing glycemic control are highly recommended.
Diabetes mellitus is a prevalent global public health issue, affecting millions of people worldwide (1). In 2021, approximately 537 million individuals had diabetes worldwide (2). Diabetes disproportionately affects the global south country (3). The African region is particularly affected, with an estimated 23.6 million adults living with diabetes. However, the prevalence of diabetes varies between countries in the region (4). Ethiopia, a global south country, ranks among the leading five African nations with a substantial number of diabetic patients, with around 1.92 million in 2021 (5).
Uncontrolled diabetes mellitus, characterized by elevated blood glucose levels, can severely affect overall health (6). If left unmanaged, it can lead to macro- or microvascular complications affecting multiple systems in the body, such as diabetic retinopathy and others (2, 7). Diabetic retinopathy, which is characterized by damage to the blood vessels in the retina (8), is one of the most prevalent micro-vascular complications and the leading cause of vision loss among diabetes patients (9, 10). Additionally, uncontrolled diabetes can have detrimental effects on other organs and systems, increasing the risk of heart disease, stroke, kidney failure, neuropathy, and lower limb amputations, thereby impacting individuals’ quality of life and resulting in increased healthcare costs and socioeconomic burdens (7, 11).
Approximately one-third of all individuals with diabetes worldwide have some degree of diabetic retinopathy (12). In Africa, the prevalence of diabetic retinopathy ranges from 7.0 to 62.4% (13). In Ethiopia, the prevalence of DR is increasing, with reports indicating that 34.1% of diabetes patients in the country have some form of DR (8).
The development of diabetic retinopathy among diabetes patients is closely associated with uncontrolled blood glucose levels (14). Over time, persistently elevated blood sugar levels can damage the small blood vessels in the retina, leading to the onset and progression of diabetic retinopathy (15). Proper management of blood glucose levels is crucial to reducing the risk of developing diabetic retinopathy (16, 17). Numerous factors, including duration of diabetes, type of diabetes, body mass index, and comorbidity, impact the incidence of diabetic retinopathy (18–21).
Fasting blood sugar tests are essential predictors for diabetes diagnosis and management, as they can help prevent complications (22). However, in resource-limited settings like Ethiopia, the longitudinal association between clinical biomarkers and the incidence of diabetic retinopathy among diabetes patients is rarely considered.
Therefore, this study aims to assess the incidence and predictors of diabetic retinopathy and their association with longitudinal changes in FBS levels among diabetic mellitus patients. Estimating the incidence of diabetic retinopathy provides insights for implementing effective strategies to reduce diabetic-related complications. Joint modeling analysis enables a comprehensive understanding of the relationship between blood glucose levels and the incidence of diabetic retinopathy, helping identify high-risk individuals for targeted prevention and improved management of diabetes mellitus.
A multi-centered and institutionally based retrospective follow-up study was carried out between January 1, 2011, and December 30, 2021. The University of Gondar, Felege Hiwot, and Debre Tabor Compressive Referral Hospitals in the Amhara region of Ethiopia were the three referral hospitals where the study was carried out.
The study included newly diagnosed diabetes patients aged 15 or older who received follow-up care at selected referral hospitals between January 1, 2011, and December 30, 2021. A total of 462 study subjects were estimated. For the survival parts, the sample size was determined using factors significantly associated with the incidence of DR from previous studies, and the sample size was calculated using the Schoenfeld formula (23).
Where E= number of required events, n= sample size, HR is the hazard ratio of selected covariates, p1 proportion of subjects under the exposure group, p2 = 1 – p1, and P(E) is the probability of an event.
Therefore, based on the results we found from previous retrospective follow-up studies done in Ethiopia, being male associated with diabetes retinopathy (AHR =1.94) (20), and assuming the following assumptions: power = 80%, ᾳ = 0.05, ß = 0.2, the sample size becomes 280 under this approach.
For the longitudinal part, the sample size was determined using the Diggle formula taken for repeated measurements for longitudinal parts (24).
N: is the total sample size, d is the effect size, m is the number of time points repeated measurement, ρ is a correlation between repeated measurements and σ2 is the variance of outcome variables. Assuming a significance level of 0.05, power of 0.8, ρ = 0.5, and effect size of 0.8, m = 8 times points based on a previous study, From a study conducted in Jimma on longitudinal FBS change we have, σ^2 (random intercept model) (25). Using zα/2 = 1.96, Zβ = 0.842, and inserting all quantities in the formula.
Therefore, the final sample size for this study was 280, then after adding 10% incompleteness and considering design effect 1.5. The final sample size was 462 DM patients.
A computer-generated simple random sampling technique was employed to select records from the newly diagnosed diabetes patients. DM Patients with uncertain dates of enrollment, as well as those who had diabetic retinopathy at the time the research started, were excluded.
Based on previously published research and the medical records of patients with diabetes mellitus (DM), a structured data collection checklist has been developed for this study. The checklist consisted of four parts, each covering different aspects of the patient’s characteristics.
The first part of the checklist focused on socio-demographic characteristics, including variables such as the age of study participants, sex, and residence. This information provided insights into the demographic profile of the study participants. The second part of the checklist captured clinical characteristics, encompassing factors such as body mass index (BMI), type of treatment, family history of DM, duration of DM, type of DM, presence of diabetic nephropathy, hypertension, diabetic neuropathy, peripheral arterial disease, and history of stroke. The third part of the checklist encompassed physiological characteristics, including measurements of high-density lipoprotein (HDL), low-density lipoprotein (LDL), creatinine levels, triglyceride levels, cholesterol levels, protein urea, systolic blood pressure (mmHg), and diastolic blood pressure (mmHg). Lastly, the fourth part of the checklist focused on capturing fasting blood sugar levels (FBS), which were measured repeatedly throughout the study. FBS levels were an important indicator for assessing the patient’s glycemic control.
In this study, the dependent variable was diabetic retinopathy, defined as the presence of at least one micro-aneurysm in any field, the presence of hemorrhages, or the occurrence of maculopathy in individuals with diabetes mellitus (26). The determination of whether a patient had diabetic retinopathy or not was made by reviewing their medical documents.
For this study, version 4.4 of EpiData was used to enter the data, while R version 4.3 was used for analysis. To prepare the data for analysis, the data was combined and coded as required. Descriptive statistics were computed for both categorical and continuous variables to summarize the data. Individual and average profile plots were created for the longitudinal analysis to visualize the trends over time. Model comparison was conducted using AIC (Akaike Information Criterion) values to select the best-fitting longitudinal sub-model.
Additionally, the normality assumption of the linear mixed effect model was assessed for fasting blood sugar (FBS) values. In the survival analysis, the incidence of diabetic retinopathy was estimated using Kaplan-Meier (KM) curves, and the log-rank test was employed to compare survival times between different variables. Before fitting the survival sub-models, proportional hazard assumptions (PHA) were investigated.
The relationship between changes in longitudinal FBS and diabetic retinopathy was evaluated using the alpha value obtained from the employed joint model. A variable was taken to be significant in the multivariable joint model if its p-value was less than 0.05 and it was within the 95% confidence interval.
This study received ethical approval from the University of Gondar, College of Medicine and Health Science, Institute of Public Health, Institutional Review Board (IRB) with reference number Ref No/IPH/1397/2020. Official permission was also obtained from the clinical directors of the selected referral hospitals. The study adhered to relevant guidelines and regulations. Informed consent was waived by the Institutional Review Board due to the retrospective nature of the study, and the data were anonymized and kept confidential.
A total of 462 patients with newly diagnosed DM were included in this study. The study participants had a mean age of 45.78 (SD ±15.92) years. Most of the study participants, 257 (55.6%) and 238 (51.5%), were male and urban residents. Almost two-thirds of the study’s participants, 290 (62.7%), had type two diabetes mellitus (T2DM), and one-third of them, 134 (29.0%), had hypertension. Most of the 220 (47.6%) patients enrolled in this study were on oral hypoglycemic agent (OHA) treatment (Table 1).
Table 1 Sociodemographic and clinical characteristics of DM patients on treatment in the Amhara region of Ethiopia.
Among the patients, 10.9% had elevated triglyceride levels, while 13.7% had borderline total cholesterol levels. A majority of the patients (58.5%) had high-density lipoprotein cholesterol (HDL-C) levels above 40mg/dl, and 6.9% DM patients had diabetic nephropathy, as shown in (Table 2).
Table 2 Physiologic characteristics, and diabetic-related complications among diabetic patients at treatment in Amhara region, Ethiopia.
The study followed a cohort of diabetes patients ranging from 6 to 120 months, with a median follow-up time of 46 months (interquartile range: 29 to 71 months). Among the 462 study participants, a total of 54 individuals (11.69%, 95% CI [8.74, 14.62]) developed diabetic retinopathy throughout 23,111.0 person-months (PM) of observation. The calculated incidence density was 2.33 per 1000 PM (95% CI: [1.78, 3.05]), or 2.97 per 100 person-years (95% CI: [2.26, 3.91]), as depicted in (Figure 1).
During the study period, diabetes patients on treatment underwent a maximum of twenty-eight and a minimum of three fasting blood sugar (FBS) measurements. The individual profile plots provided a comprehensive visualization of the FBS trends for each patient, revealing significant variability both within and between patients (Figure 2). Furthermore, the analysis of this study revealed that the subject variability among diabetes mellitus (DM) patients with events was higher compared to the censored parts. This observation provides evidence for the suitability of applying joint modeling techniques to this dataset (Figure 3).
Figure 2 Individual profile plots Fasting Blood Sugar (FBS) over time for DM patients on treatment in Ethiopia.
Figure 3 Individual profile plots by status of diabetic retinopathy for DM patients on treatment in Ethiopia.
The mean of FBS among DM patients shows that individuals who developed diabetic retinopathy had higher FBS levels compared to those who did not develop diabetic retinopathy (Figure 4).
Figure 4 Mean profile of Fasting Blood Sugar (FBS) by status of diabetic retinopathy for DM patients on treatment in Ethiopia.
Joint modeling indicates the associated factors for both changes in FBS and the incidence of diabetic retinopathy. The results indicated a significant association between the 3-month lagged value of FBS and the risk of DR. The results from (Table 3), shows that there is a significant association (α = 1.43, p-value 0.003) between the longitudinal change in fasting blood sugar levels (log of FBS) and the risk of DR. This finding suggests that a unit increase in the past 3-month value of the log of FBS corresponds to a 4.2-fold increase in the current risk of developing DR. In addition, being a rural resident, having hypertension (comorbidity), and having a duration of diabetes greater than five years were significant predictors for the incidence of diabetic retinopathy.
Table 3 Joint modeling of the survival and longitudinal sub-models for diabetic mellitus patients on treatment in Amhara region referral hospitals, Ethiopia.
After controlling for other variables in the model, rural patients had a hazard of developing diabetic retinopathy (DR) that was 2.21 times higher compared to urban patients (adjusted hazard ratio [AHR] = 2.21, 95% CI: [1.21, 4.05]). DM patients with hypertension (co-morbidity) had a hazard of developing DR that was 3.01 times higher than those without hypertension (co-morbidity) when accounting for other variables (AHR = 3.01, 95% CI: [1.85, 6.53]). Furthermore, after controlling other factors in the model, DM patients with a diabetes duration greater than five years had a hazard of experiencing DR that was 2.28 times higher compared to those with a duration of less than five years (AHR = 2.28, 95% CI: [1.91, 5.15]). These findings are presented in (Table 3).
Diabetic retinopathy (DR) is a significant global public health concern and a leading cause of blindness. Understanding the incidence of DR and identifying its predictors are vital for effective prevention and management strategies. Furthermore, investigating the impact of fasting blood sugar (FBS) changes on the risk of DR can provide valuable insights into the disease progression. This study aimed to determine the incidence of DR, identify its predictors, and examine the association between longitudinal FBS trajectory and the incidence of DR among newly diagnosed diabetes patients on treatment in referral hospitals in the Amhara region, Ethiopia. By utilizing a joint modeling approach, the researchers could simultaneously analyze the longitudinal FBS data and the occurrence of DR to assess their association.
In this study, the incidence of diabetic retinopathy was observed in 11.69% of the participants, with an incidence density of 2.9 per 100 person-years (PY) of observation. This finding aligns with a previous study in central Ethiopia (20). However, it is noteworthy that the incidence reported in this study is higher compared to studies conducted in northwest Ethiopia (26), China (27), and Bangladesh (28), where the incidence of diabetic retinopathy was 2.0, 1.81 and 1.75 per 100 PY of observation, respectively. This discrepancy in incidence rates could be attributed to several factors. Firstly, variations in the study population, such as differences in lifestyle factors and comorbidities, may contribute to differences in diabetic retinopathy incidence. Additionally, variations in healthcare facilities and the quality of care provided in different settings could impact the detection and management of diabetic retinopathy, thereby influencing the reported incidence rates.
According to this finding, the risk of developing diabetic retinopathy (DR) was higher for rural patients than for urban patients. This is consistent with previous research conducted in Bangladesh (29), which demonstrated that semi-urban subjects faced a higher risk of diabetic retinopathy when compared to their urban counterparts. Additionally, a study in Beijing, China, revealed a significant association between diabetic retinopathy and patients residing in rural regions (30). This could be because rural diabetes mellitus (DM) patients may have poorer self-care practices and limited access to healthcare services, leading to suboptimal management of their diabetes and higher susceptibility to DR (31). Poor health care-seeking behaviors among rural populations, coupled with limited resources and infrastructure, can contribute to delays in diagnosis and treatment of DM-related complications, including DR. Furthermore, individuals residing in rural areas may face additional challenges, such as limited availability of specialized eye care services and reduced awareness about the importance of regular eye examinations for DM patients.
Our study found that DM patients who also have hypertension co-morbidity had an increased risk of developing Diabetic retinopathy compared with their counterparts without hypertension. This finding is supported by studies conducted in Debre Markos (32), which showed that hypertensive patients are three times more likely to develop Diabetic retinopathy compared to non-hypertensive patients. This correlation is consistent with similar studies conducted in Arbaminch General Hospital in Ethiopia (33) and Khartoum in Sudan (34). Furthermore, existing research indicates that hypertension serves as a risk factor for both the onset and progression of retinopathy (35). The heightened blood flow associated with hypertension can potentially damage the retinal capillary endothelial cells in individuals with diabetes, leading to the manifestation of retinopathy.
Diabetes patients with a duration exceeding five years were at a 2.2 times higher risk of developing Diabetic retinopathy (DR) compared to patients with a duration of DM lower than five years. This finding is in line with similar studies conducted in Debre Markos (32), Beijing (30), and Denmark (36), which all reported a significant association between longer durations of DM and the development of DR. However, a study conducted at the University of Gondar Comprehensive Specialized Hospital in Ethiopia (26) showed conflicting results, indicating a negative association between the duration of diabetes and the likelihood of developing DR. This discrepancy may be due to the reason that the study conducted in University of Gondar specialized hospital exclusively focused on type-2 diabetes patients, who may have better metabolic control and lower levels of fasting blood sugar. This disparity in findings suggests that the improved metabolic control and lower FBS levels observed in type-2 DM patients could explain the contradictory results.
In this research, we employed a time-dependent lagged value parameterization of joint modeling to investigate the association between fasting blood sugar levels and the risk of diabetic retinopathy (DR). Our finding showed a significant correlation between the fasting blood sugar levels from the past three months and the current risk of DR. Specifically, an increase of one unit in the log-transformed fasting blood sugar level over the past three months corresponded to a 4.2-fold increase in the risk of developing DR. These results are consistent with previous studies done at the Arbaminch General Hospital and in France, which demonstrated a strong relationship between elevated levels of hemoglobin A1c and fasting plasma glucose for the presence of diabetic retinopathy (33, 37). These findings suggest that higher fasting blood glucose levels, indicative of inadequate blood glucose control, may contribute to the development of diabetic retinopathy.
The findings of this study have significant clinical and public health implications. It is crucial to increase awareness and education about the risk factors associated with diabetic retinopathy (DR). Health education programs should emphasize regular screening, glycemic control, and blood pressure management to reduce the risk of DR. Implementing regular screening programs for early detection and intervention is essential. Prioritizing glycemic control through medication adherence, lifestyle changes, and regular monitoring of blood sugar levels is crucial in reducing the risk of DR.
Effective management of hypertension is also important since it is a significant predictor of DR incidence. Controlling blood pressure levels can decrease the risk of developing DR. Further research is needed to explore additional risk factors and preventive strategies for DR, including lifestyle factors. By implementing these recommendations, healthcare providers, policymakers, and patients can collaboratively reduce the burden of diabetic retinopathy and improve outcomes for individuals with diabetes.
This study has its strengths and limitations; the study may overcome previously unexplored associations between longitudinal clinical biomarkers of FBS and the incidence of DR. It has significant clinical and public health importance by providing stronger evidence for established risk factors and helps to guide clinical practice. However, the data were collected retrospectively using secondary sources, thereby being incomplete.
In this study, the incidence of diabetic retinopathy was somewhat high when compared to prior similar studies in Ethiopia. A joint model of longitudinal fasting blood sugar level changes was significantly associated with an increased risk of DR. Besides, being rural residence, hypertension co-morbidity, and a longer duration of DM were significant predictors for the incidence of DR. Therefore, public awareness, an integrated care approach, and prioritizing glycemic control are highly recommended.
Based on the findings of this study, the following recommendations are made for the concerned bodies: Health professionals should give greater attention to DM patients with the identified risk factors for DR. Patients with diabetes mellitus who also have hypertension and a duration of DM greater than five years should closely monitor and control their blood glucose levels. Further studies on this topic, including behavioral factors and prospective studies, are recommended.
The original contributions presented in the study are included in the article/supplementary material. Further inquiries can be directed to the corresponding author.
Ethical approval to conduct the study was approved by ethical review board of the University of Gondar, College of Medicine and Health Science, Institute of Public Health, Institutional review board (IRB) with reference number Ref No/IPH/1397/2020. The studies were conducted in accordance with the local legislation and institutional requirements. Due to the retrospective nature of this study, based on secondary data from the patient’s medical record, the IRB waived informed consent.
HA: Conceptualization, Data curation, Formal analysis, Funding acquisition, Investigation, Methodology, Project administration, Resources, Software, Supervision, Validation, Visualization, Writing – original draft, Writing – review & editing. MY: Conceptualization, Methodology, Supervision, Validation, Writing – original draft, Writing – review & editing. HW: Conceptualization, Methodology, Software, Supervision, Validation, Writing – original draft, Writing – review & editing. BM: Conceptualization, Data curation, Validation, Writing – review & editing. ML: Conceptualization, Data curation, Validation, Writing – review & editing. AL: Conceptualization, Formal analysis, Methodology, Software, Supervision, Validation, Writing – original draft, Writing – review & editing.
The author(s) declare that no financial support was received for the research, authorship, and/or publication of this article.
We would like to express our sincere gratitude to the University of Gondar College of Medicine and Health Sciences and the Institute of Public Health. We also want to thank selected referral hospital clinical directors and chart room workers for their contributions to this study.
The authors declare that the research was conducted in the absence of any commercial or financial relationships that could be construed as a potential conflict of interest.
All claims expressed in this article are solely those of the authors and do not necessarily represent those of their affiliated organizations, or those of the publisher, the editors and the reviewers. Any product that may be evaluated in this article, or claim that may be made by its manufacturer, is not guaranteed or endorsed by the publisher.
AOR, Adjusted Odds Ratio; BP, Blood Pressure; CI, Confidence Interval; DBP, Diastolic Blood Pressure; DR, Diabetic retinopathy; DM, Diabetes Mellitus; DTCRH, Debre Tabor Compressive Referral Hospital; FBS, Fasting Blood Sugar; FHCSH, Felege Hiwot Compressive Specialized Hospital; HDL, High-Density Lipoprotein; HR, Hazard Ratio; IRB, Ethical Review Board; KM, Kaplan Meier; LDL, Low-Density Lipoprotein; OHA, Oral Hypoglycemic Agents; PHA, Proportional Hazard Assumption; PY, Person Years; SBP, Systolic Blood Pressure; UoG, University of Gondar; UoGCSH, University of Gondar Compressive Specialized Hospital; WHO, World Health Organization.
1. Al-Lawati JA. Diabetes mellitus: a local and global public health emergency! Oman Med J. (2017) 32:177. doi: 10.5001/omj.2017.34
2. Matoori S. Diabetes and its complications. ACS Publications. (2022) pp:513–5. doi: 10.1021/acsptsci.2c00122
3. Ali MK, Galaviz KI, Weber MB, Narayan KV. The global burden of diabetes. In: Textbook of diabetes (2017). p. 65–83. doi: 10.1002/9781118924853.ch5
4. International Diabetes Federation. IDF Diabetes Atlas te (2021). Available online at: https://diabetesatlas.org/atlas/tenth-edition/.
5. region tpoditA. (2019). Available online at: https://www.openaccessgovernment.org/diabetes-african-region/73153.
6. Organization WH. Use of glycated haemoglobin (HbA1c) in diagnosis of diabetes mellitus: abbreviated report of a WHO consultation. World Health Organization (2011). Available at: https://iris.who.int/bitstream/handle/10665/70523/WHO_NMH_CHP_CPM_11.1_eng.pdf.
7. Balaji R, Duraisamy R, Kumar M. Complications of diabetes mellitus: A review. Drug Invention Today. (2019) 12:98–103.
8. Alemu Mersha G, Alimaw YA, Woredekal AT. Prevalence of diabetic retinopathy among diabetic patients in Northwest Ethiopia—A cross sectional hospital based study. PLoS One. (2022) 17:e0262664. doi: 10.1371/journal.pone.0262664
9. Yamagishi S-I, Imaizumi T. Diabetic vascular complications: pathophysiology, biochemical basis and potential therapeutic strategy. Curr Pharm design. (2005) 11:2279–99. doi: 10.2174/1381612054367300
10. Chawla A, Chawla R, Jaggi S. Microvasular and macrovascular complications in diabetes mellitus: distinct or continuum? Indian J Endocrinol Metab. (2016) 20:546. doi: 10.4103/2230-8210.183480
11. Stitt AW, Curtis TM, Chen M, Medina RJ, McKay GJ, Jenkins A, et al. The progress in understanding and treatment of diabetic retinopathy. Prog retinal eye Res. (2016) 51:156–86. doi: 10.1016/j.preteyeres.2015.08.001
12. Takele MB, Boneya DJ, Alemu HA, Tsegaye TB, Birhanu MY, Alemu S, et al. Retinopathy among adult diabetics and its predictors in Northwest Ethiopia. J Diabetes Res. (2022) 2022. doi: 10.1155/2022/1362144
13. Burgess P, MacCormick I, Harding S, Bastawrous A, Beare N, Garner P. Epidemiology of diabetic retinopathy and maculopathy in Africa: a systematic review. Diabetic Med. (2013) 30:399–412. doi: 10.1111/j.1464-5491.2012.03756.x
14. Huri HZ, Huey CC, Mustafa N, Mohamad NF, Kamalden TA. Association of glycemic control with progression of diabetic retinopathy in type 2 diabetes mellitus patients in Malaysia. Braz J Pharm Sci. (2018) 54. doi: 10.1590/s2175-97902018000217484
15. Kusuhara S, Fukushima Y, Ogura S, Inoue N, Uemura A. Pathophysiology of diabetic retinopathy: the old and the new. Diabetes Metab J. (2018) 42:364–76. doi: 10.4093/dmj.2018.0182
16. Simó-Servat O, Hernández C, Simó R. Diabetic retinopathy in the context of patients with diabetes. Ophthalmic Res. (2019) 62:211–7. doi: 10.1159/000499541
17. Burgess PI, Msukwa G, Beare NA. Diabetic retinopathy in sub-Saharan Africa: meeting the challenges of an emerging epidemic. BMC Med. (2013) 11:1–7. doi: 10.1186/1741-7015-11-157
18. Sahiledengle B, Assefa T, Negash W, Tahir A, Regasa T, Tekalegn Y, et al. Prevalence and factors associated with diabetic retinopathy among adult diabetes patients in Southeast Ethiopia: A hospital-based cross-sectional study. Clin Ophthalmol. (2022) 16:3527–45. doi: 10.2147/OPTH.S385806
19. Shani M, Eviatar T, Komaneshter D, Vinker S. Diabetic retinopathy–incidence and risk factors in a community setting-a longitudinal study. Scandinavian J primary Health Care. (2018) 36:237–41. doi: 10.1080/02813432.2018.1487524
20. Azeze TK, Sisay MM, Zeleke EG. Incidence of diabetes retinopathy and determinants of time to diabetes retinopathy among diabetes patients at Tikur Anbessa Hospital, Ethiopia: a retrospective follow up study. BMC Res Notes. (2018) 11:1–6. doi: 10.1186/s13104-018-3660-7
21. Moges WK, Muralidharan A, Tadesse HZ. Gaussian longitudinal analysis of progression of diabetes mellitus patients using fasting blood sugar level: A case of debre berhan referral hospital, Ethiopia. Am J Theor Appl Stat. (2018) 7:21. doi: 10.11648/j.ajtas.20180701.13
22. Center of Disease Control (CDC) CfDCaP. Monitoring Your Blood Sugar March 20 (2019). Available online at: https://www.cdc.gov/diabetes/managing/managing-blood-sugar/bloodglucosemonitoring.html.
23. Schoenfeld DA. Sample-size formula for the proportional-hazards regression model. Biometrics. (1983) 39:499–503. doi: 10.2307/2531021
24. Diggle PJ, Heagerty P, Liang K-y, Zeger SL. Analysis of Longitudinal Data. Oxford University Press (2002). doi: 10.1093/oso/9780198524847.001.0001
25. Debele GR, Hajure M, Wolde HF, Yenit MK. Incidence and predictors of chronic kidney disease among diabetes mellitus patients: a retrospective follow-up study at a tertiary health-care setting of Ethiopi. Diabetes Metab Syndr Obes. (2021) 14:4381–90. doi: 10.2147/DMSO.S335572
26. Kebede SA, Tessema ZT, Balcha SA, Ayele TA. Joint modeling of time to diabetic retinopathy and change in fasting blood sugar among type 2 diabetic patients, Northwest Ethiopia. Sci Rep. (2022) 12:1–9. doi: 10.1038/s41598-022-06240-5
27. Liu L, Wu J, Yue S, Geng J, Lian J, Teng W, et al. Incidence density and risk factors of diabetic retinopathy within type 2 diabetes: a five-year cohort study in China (Report 1). Int J Environ Res Public Health. (2015) 12:7899–909. doi: 10.3390/ijerph120707899
28. Ahmed KR. Incidence of diabetic retinopathy: A 15 year follow up in a hospital population (Bangladesh). (2009). doi: 10.1111/j.1753-0407.2012.00208.x
29. Huq MS, Islam K, Sumsujjaman M, Shoma RA, Saifullah AFA, Hussain F. Diabetic retinopathy and associated risk factors among diabetic patients attending eye camps in Northern Bangladesh. J Prev Soc Med. (2021) 40:16–25. doi: 10.3329/jopsom.v40i2.61792
30. Xie XW, Xu L, Wang YX, Jonas JB. Prevalence and associated factors of diabetic retinopathy. The Beijing Eye Study 2006. Graefe's Arch Clin Exp Ophthalmol. (2008) 246:1519–26. doi: 10.1007/s00417-008-0884-6
31. Ayele BH, Mengesha MM, Tesfa T. Predictors of self-care activities of outpatient diabetic residents in Harar and Dire Dawa: A hospital-based cross-sectional study. SAGE Open Med. (2019) 7:2050312119865646. doi: 10.1177/2050312119865646
32. Tilahun M, Gobena T, Dereje D, Welde M, Yideg G. Prevalence of diabetic retinopathy and its associated factors among diabetic patients at Debre Markos referral hospital, Northwest Ethiopia, 2019: Hospital-Based Cross-Sectional Study. Diabetes Metab Syndrome Obesity: Targets Ther. (2020) 13:2179. doi: 10.2147/DMSO.S260694
33. Chisha Y, Terefe W, Assefa H. Incidence and factors associated with diabetic retinopathy among diabetic patients at arbaminch general hospital, Gamo gofa Zone (longitudinal follow up data analysis). J Diabetol. (2017) 8:1–6. doi: 10.4103/jod.jod_6_17
34. Elwali ES, Almobarak AO, Hassan MA, Mahmooud AA, Awadalla H, Ahmed MH. Frequency of diabetic retinopathy and associated risk factors in Khartoum, Sudan: population based study. Int J Ophthalmol. (2017) 10:948. doi: 10.18240/ijo.2017.06.18
35. Gillow J, Gibson J, Dodson P. Hypertension and diabetic retinopathy—what’s the story? Br J Ophthalmol. (1999) 83:1083–7. doi: 10.1136/bjo.83.9.1083
36. Dinesen S, Stokholm L, Subhi Y, Peto T, Savarimuthu TR, Dine S, et al. Five-year incidence of proliferative diabetic retinopathy and associated risk factors in a nationwide cohort of 201 945 danish patients with diabetes. Ophthalmol Sci. (2023) 3:100291. doi: 10.1016/j.xops.2023.100291
Keywords: incidence, diabetic retinopathy, fasting blood sugar, diabetic mellitus, joint modeling, Ethiopia
Citation: Abuhay HW, Lakew AM, Wolde HF, Mengistu B, Legesse MT and Yenit MK (2024) Diabetic retinopathy incidence, predictors and its association with longitudinal fasting blood sugar level changes among diabetes mellitus patients in Ethiopia: joint model. Front. Endocrinol. 15:1363757. doi: 10.3389/fendo.2024.1363757
Received: 31 December 2023; Accepted: 25 June 2024;
Published: 08 July 2024.
Edited by:
Chunjie Jiang, University of Texas MD Anderson Cancer Center, United StatesReviewed by:
Sudhanshu Kumar Bharti, Patna University, IndiaCopyright © 2024 Abuhay, Lakew, Wolde, Mengistu, Legesse and Yenit. This is an open-access article distributed under the terms of the Creative Commons Attribution License (CC BY). The use, distribution or reproduction in other forums is permitted, provided the original author(s) and the copyright owner(s) are credited and that the original publication in this journal is cited, in accordance with accepted academic practice. No use, distribution or reproduction is permitted which does not comply with these terms.
*Correspondence: Habtamu Wagnew Abuhay, aGFiN3dhZ25ld0BnbWFpbC5jb20=; SGFidGFtdS5XYWduZXdAdW9nLmVkdS5ldA==
Disclaimer: All claims expressed in this article are solely those of the authors and do not necessarily represent those of their affiliated organizations, or those of the publisher, the editors and the reviewers. Any product that may be evaluated in this article or claim that may be made by its manufacturer is not guaranteed or endorsed by the publisher.
Research integrity at Frontiers
Learn more about the work of our research integrity team to safeguard the quality of each article we publish.