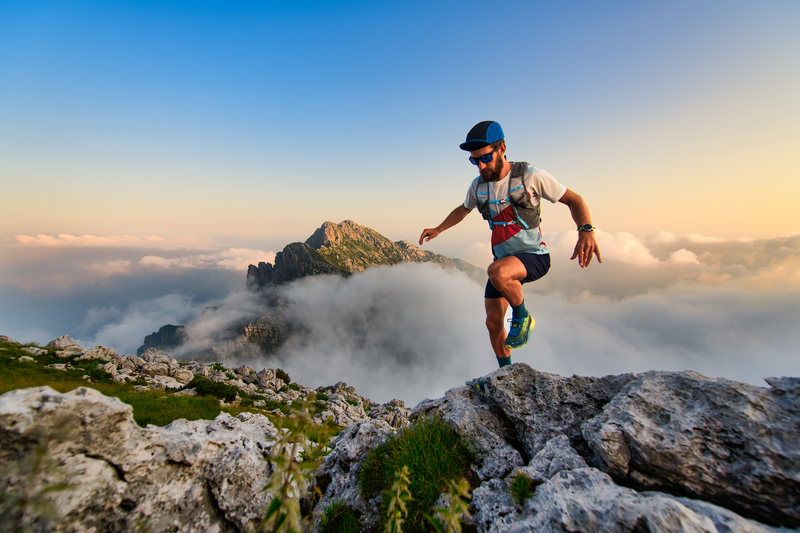
94% of researchers rate our articles as excellent or good
Learn more about the work of our research integrity team to safeguard the quality of each article we publish.
Find out more
ORIGINAL RESEARCH article
Front. Endocrinol. , 05 March 2024
Sec. Clinical Diabetes
Volume 15 - 2024 | https://doi.org/10.3389/fendo.2024.1361906
This article is part of the Research Topic Effects of Midday Naps on Glycemic Control of Diabetic Patients View all 5 articles
Aim: To examine the association between napping characteristics and glycemic control in people with type 2 diabetes.
Design: This study used a cross-sectional design.
Methods: A convenience sample of people with type 2 diabetes (N=226) were included. Glycemic control was indicated by HbA1c which was measured by A1C Now®+. Napping characteristics including napping frequency, duration, timing, and type were measured by validated questionnaires. Other variables, such as insomnia, cognitive impairment, and depression were measured by the Insomnia Severity Index, Montreal Cognitive Assessment, and Patient Health Questionnaire-9, respectively. Multivariate linear regression analyses were performed.
Results: The sample consisted of 122 women (54.0%), with a median age of 67 years. Their median HbA1c was 6.8%. No significant relationship was found between napping frequency and HbA1c. Among nappers, after controlling for covariates, long napping duration (≥60 min) and morning napping were both associated with poorer glycemic control. Compared with appetitive napping, restorative napping was associated with better glycemic control.
Conclusion: Daytime napping (e.g., duration and type) is an important modifiable factor for glycemic control in people with type 2 diabetes. This study provides new insights into the relationship between napping and glucose management among people with diabetes.
In 2021, one in ten adults was living with diabetes, and around 90%-95% were diagnosed with type 2 diabetes (T2D) (1). Diabetes was among the top 10 causes of death (2), causing significant burdens to the individuals and society. Adequate glycemic control is the key to preventing or delaying the development of diabetic complications (3). Hemoglobin A1c (HbA1c), which reflects the overall glycemic control over the past two to three months, has been used as the primary indicator for diabetes management (4). Traditional factors, including demographics (e.g., age and sex) (5), clinical characteristics (e.g., BMI, diabetes duration, and treatment regimen) (5–7), lifestyle factors (e.g., smoking and drinking) (8), and psychosocial factors (e.g., depression and cognitive function) (9, 10) have been associated with HbA1c. Emerging evidence indicates that sleep may also play a role in the development and management of diabetes (11, 12).Sleep is a complex and dynamic process during which there is a reduced responsiveness to external stimulation. In most cases (except for shift workers), it includes nighttime sleep and daytime napping. In recent years, researchers started to examine the impact of napping on human health. A nap is defined as any sleep period with a duration of less than 50% of the average major sleep period. In addition to the traditionally assessed dimensions (e.g., frequency, duration), type and timing of napping are also important dimensions. The type of napping can be categorized as replacement (i.e., a nap taken in response to sleep loss), prophylactic napping (i.e., a nap taken in anticipation of sleep loss), and appetitive napping (i.e., nap for enjoyment or habitual napping such as siesta) (13). The category of “replacement” and “prophylactic” napping may be confusing for participants frequently experiencing sleep deprivation. Thus, they have been labeled as “restorative” (14, 15). Among people with T2D, disturbed nighttime sleep (e.g., short sleep duration, poor sleep quality, and insomnia symptoms) have been associated with poorer glycemic control (12, 16). Napping, which is very common in many cultures (e.g., China), may also play an important role in cardiometabolic health (17).
Several studies have examined the relationship between napping and glycemic control in people with T2D (18–21), with inconsistent results. Specifically, Bawadi, et al. found that those who “sometimes, frequently, or always” napped had an increased risk of poor glycemic control than those who “never or rarely” napped (20). This finding is similar to the one found in another study, showing an approximately threefold risk of having poor glycemic control in nappers compared with non-nappers (21). In contrast, Gozashti, et al. reported that those who napped had better glycemic control than those who did not nap (18). In another study, taking midday naps in short sleepers (sleep duration<5h) was associated with a reduced risk for poor glycemic control (19). Although previous studies conducted in people with T2D provided evidence about the association between napping and glycemic control, they have been mostly focused on one single dimension of napping (i.e., frequency), neglecting the complexity of napping. Studies conducted in other populations found that longer napping duration was associated with hyperglycemia in pregnant women (22) and increased risk for impaired fasting glucose and diabetes in retired workers (23). Based on the above evidence, there is a need to provide a complete picture of the association between napping and glycemic control among people with T2D. This study aims to: 1) describe the napping characteristics of people with T2D and 2) examine the relationship between napping with glycemic control. Findings from this study may provide more evidence for diabetes management and add to current knowledge about the relationship between sleep and diabetes.
A cross-sectional design was used. The study was approved by the Institutional Review Board of Shanghai Jiao Tong University School of Medicine (#SJUPN-201811). All participants signed a paper informed consent form before completing the questionnaires.
Sample size calculation was performed by G*Power 3.1 (Franz Faul, Germany). α (two-tail) and 1-β was set at 0.05 and 0.8, respectively. A total of 15 predictors were considered when calculating a priori sample size, including four napping variables, two night sleep variables (i.e., insomnia and sleep duration) (12, 16), and nine traditional factors as indicated in the earlier section. Based on a study conducted in people with type 1 diabetes examining the association between napping frequency and HbA1c level, the effect size was 0.74 (95%CI=0.09-1.40) (24). In this study, the lower limit of 0.09 was used to ensure adequate power. We assumed that each napping variable would be associated with HbA1c, with a small effect size. F test was used for the calculation, which resulted in a minimum sample size of 222. A total of 226 participants were included in the final analysis.
Participants were recruited using a convenience sampling method. The inclusion criteria were: (a) 18 years of age or older; (b) being diagnosed as T2D according to the WHO diagnosis criteria: random plasma glucose concentration ≥11.1mmol/L, and/or fasting plasma glucose concentration ≥7.0mmol/L, and/or 2-hour postprandial plasma glucose concentration ≥11.1mmol/L, accompanied by diabetic-related symptoms (25), or self-reported T2D (as determined by current use of antidiabetic drugs or insulin), or confirmed by a physician; (c) did not change the therapeutic regimen during the past three months.
The exclusion criteria were self-reported: (a) having serious chronic diseases (e.g., severe cardio-cerebrovascular disease and chronic kidney disease); (b) having severe diabetic complications (e.g., severe retinopathy and nephropathy), (c) pregnant women; (d) on night shift; (e) travelled crossed the time zone in the past week; (f) having mental illness (e.g., schizophrenia) or severe cognitive impairment, causing difficulties in obtaining informed consent.
Participants were recruited from two community healthcare centers in Shanghai and one in Henan, China from May 2023 to July 2023. The process of recruitment is shown in Figure 1. Briefly, 665 participants were contacted, with 273 agreed to participate. Among them, 240 completed the screening, and 236 were enrolled. Ten were excluded due to significant missing data on key questionnaires or invalid responses (response with a clear pattern).
Data were collected using paper-and-pencil questionnaires. The participants were instructed to fill out the questionnaires in a quiet room. Two graduate students were present to answer questions the participants had. The following questionnaires were used to measure variables of interest, including demographic, clinical, psychosocial, and lifestyle characteristics, sleep (e.g., overall sleep health and insomnia) and napping behaviors (e.g., napping frequency, duration, timing and type).
Glycemic control was assessed by HbA1c and poor glycemic control was defined as HbA1c≥7%, consistent with previous evidence (20). HbA1c was measured by A1C Now®+ (PTS Diagnostics) using a fingertip blood sample (26).
Items from the Napping Behavior Questionnaire were used to assess napping (27) frequency and duration. Napping frequency was measured by “On average how many days do you take daytime nap during a typical week”? The response included (a) <1, (b) 1-2, (c) 3-4, (d) 5-6, and (e) >6. It was categorized into (a) <1, (b) 1-2, (c) 3-4, (d) 5-7 during data analysis based on previous evidence (28). Napping duration was measured by “How long is your typical nap”? The response included (a) <15min, (b) 15-30min, (c) 30-45min, (d) 45-60min, and (e) >60min. It was dichotomized as long napping duration (a) yes (≥60 min) and (b) no (<60 min) based on previous evidence (29).
The timing of napping was measured by “What time did your nap usually occur”? The response included (a) in the morning (before 12 pm), (b) in the afternoon (12 pm to 4:30 pm), (c) in the evening (4:30 pm to 9 pm) (30). The type of napping was measured by “Why do you usually nap”? The response included (a) “purely due to habit/enjoyment/convenience, and not related to lack of sleep at night” (appetitive), (b) “didn’t get enough sleep or didn’t sleep well the night before” (replacement), and (c) “expecting lacking sleep at night” (prophylactic) (31). Consistent with previous studies, (b) and (c) were combined and labeled as “restorative” napping (14, 15).
Insomnia was measured by the Insomnia Severity Index (ISI). The ISI contains 7 items assessing the severity and consequences of insomnia in the past two weeks. Each item is scored on a 5-point Likert scale (0 to 4). Adding all items results in the total score, ranging from 0 to 28. Higher total scores indicate severer insomnia symptoms (32). The ISI has good internal consistency (Cronbach’s α=0.90) and a score of 10 or over has been used as the cut-off for clinically significant insomnia (33). The Chinese version of ISI showed good internal consistency (Cronbach’s α=0.84) (34). In this sample, the internal consistency of ISI was 0.92.
Sleep duration was assessed by asking the participants “In the past week, how many hours of sleep did you usually get on weekdays and weekends”? Sleep duration was calculated as (5*sleep duration on weekday+2*sleep duration on weekend)/7 (h). Sleep duration < 6h was considered short sleep (35).
Cognitive function was assessed by the Montreal Cognitive Assessment Beijing Version (MoCA). It measures eight domains of cognitive function. Adding scores for each domain results in a total score of 0-30. One point was added to the total score if the participants had an education years ≤ 12. A total score ≤ 25 indicates mild cognitive impairment (36). The MoCA Beijing version showed good sensitivity and validity in screening cognitive impairment in elderly adults (37). In this sample, the internal consistency 0.65.
The Patient Health Questionnaire-9 (PHQ-9) was used to assess depressive symptoms during the past two weeks. The PHQ-9 consists of nine items, with each scored on a 4-point Likert scale ranging from 0 (not at all) to 3 (nearly every day) (38). Adding all items results in a total score of 0 to 27. Higher scores indicate severer depressive symptoms. A score of 5 or over has been used as the cut-off for depression. The Chinese version of PHQ-9 has been demonstrated good internal consistency (Cronbach’s α=0.89). In this sample, the internal consistency 0.78.
A questionnaire was developed by the research team to measure demographic and clinical characteristics of the participants, such as age, sex, education level, marital status, employment status, smoking, and drinking. We also measured height and weight using objective scales. Clinical characteristics included self-report diagnosis of hypertension, family history of diabetes, diabetes duration, and treatment regimen for diabetes.
The SPSS 24.0 (IBM, Armonk, NY, USA) and OriginPro 9.9 (OriginLab Corp., Northampton, MA, USA) were used for data analysis and visualization. Missing data, normal distribution, and outliers were checked prior to data analysis. Mean substitution was used when missing data was less than 5%. Continuous variables were presented as mean (SD) if normally distributed and as median and interquartile range (IQR) otherwise. Categorical variables were presented as frequency (%). Independent sample t-test or Mann-Whitney U test, analysis of variance (ANOVA) or Kruskal-Wallis H test, and Chi-square test were used for group comparison. Spearman correlation analysis was performed to examine the association between HbA1c and other continuous variables. Multivariate linear regression analysis was performed to examine the association between napping and HbA1c while controlling for a priori covariates including age, sex, BMI, treatment regimen, diabetes duration (5, 39), cognitive impairment, depression (9, 10), and night sleep (e.g., sleep duration and insomnia symptoms) (12, 16). Variables associated with HbA1c at p<0.2 in the bivariate analyses (i.e., hypertension) were also controlled. Statistical significance was set at p<0.05 (two-tailed).
The median age of the participants was 67 years, and 122 (54.0%) were females. The majority of the participants were retired (88.1%). The mean BMI was 24.7 (SD 3.5) kg/m2. About a half of the participants had a family history of diabetes (46.5%), and oral medication was the most commonly used treatment regimen (68.1%). Participants had a median diabetes duration and HbA1c of 10 years and 6.8%, respectively. Detailed characteristics of the participants are shown in Table 1.
In this sample (N=226), 20.4% (n=46) were non-nappers. Among nappers (n=180), 33.9% had long napping duration. A majority had afternoon napping (90.0%). The common type of napping was appetitive napping (72.8%). In addition, the median sleep duration was 6.3 hours, with 32.3% having short sleep duration (<6h). The median score of ISI was 3.0 (IQR 1.0-8.0). Detailed characteristics are shown in Table 2.
Bivariate analyses showed no significant difference in HbA1c between different sex, education, marital status, work status, smoking, drinking, hypertension, family history of diabetes, and insomnia (Table 3). In comparison, significant differences in HbA1c were detected between people using different therapeutic regimen (p=0.022) and those with or without mild cognitive impairment (p=0.021) (Table 3).
Table 3 Bivariate analysis examining relationship between categorical variables (not including napping characteristics) and HbA1c level (N=226).
HbA1c level in participants with different napping frequency was not significantly different (p>0.05) (Figure 2). However, participants with long napping duration (≥60min/day) had a higher level of HbA1c (p=0.027). Compared with morning napping, afternoon napping was related to lower HbA1c (p=0.031). Those with restorative napping had significantly lower HbA1c level than those with appetitive napping (p=0.013) (Figure 2). Detailed data are shown in Supplementary Table 1.
Spearman correlation analyses showed that diabetes duration and MoCA total scores were significantly corelated to HbA1c level (p<0.001 and p=0.002) (Table 4).
Table 4 Correlation between participant characteristics (continuous variables) with HbA1c level (N=226).
Table 5 shows the multivariate linear regression results of predictors of HbA1c. Collectively, the independent variables accounted for 22.4% of the variance in HbA1c level (R2 = 22.4%, p=0.001). Controlling for covariates, long napping duration (≥60min/day) was positively associated with HbA1c level (B=0.640, 95%CI=0.129, 1.151, p=0.014). Morning napping (vs. afternoon napping) was positively associated with HbA1c level (B=0.992, 95%CI=0.112, 1.872, p=0.027). While restorative napping (vs. appetitive) was associated with lower HbA1c level (B=-0.670, 95%CI=-1.269, -0.072, p=0.028).
Table 5 Multivariate linear regression model of predictors of HbA1c level among nappers a.
In this study, we first described the napping characteristics of people with T2D and then examined the relationship between napping with glycemic control. We found that long napping duration (≥60 min) and morning napping were both associated with poorer glycemic control as indicated by higher HbA1c. In addition, restorative napping, compared with appetitive napping, was associated with better glycemic control. Findings from this study may provide more evidence for diabetes management and add to current knowledge about the relationship between sleep and diabetes.
In this sample, 20.4% were non-nappers, comparable to T2D patients in Qatar (19.4%, aged 52 years, 60% females) (20) but significantly lower than that in a Japanese T2D sample (54.5%, aged around 66 years) and Ethiopian T2D sample (68.8%, over 60% aged 45 years and older, 46% females) (19, 40). Among nappers, half of them (48.2%) had a high napping frequency of 5-7 days/week. Similarly, in the Japanese T2D sample, 15.4% napped 4-6 days/week, and 67.4% napped daily (19). In comparison, in the Ethiopian sample, around 39% reported frequently/always napped (40). The inconsistency may be due to different categorization of napping frequency, cultural differences in napping, and other sociodemographic factors. For example, napping was considered a behavior good for health in the elderly in China (41). In addition, around 90% of our participants were retired/unemployed as compared to 11% in the Ethiopian sample. People who were retired or unemployed may have more opportunities to take naps. Around 34% of our sample had long napping duration (≥60 min), in line with a previous study conducted in a Chinese T2D sample (31.1%) (42). The majority of our sample napped in the afternoon (90.0%) and were appetitive nappers (72.8%). Few studies have investigated the timing and type of napping in people with T2D. Among middle-aged adults in Switzerland (aged 61 years, 58% females), 42.5% took naps in the afternoon (12 pm-4:30 pm), 23.3% in the morning, and 31.3% in the evening (30). Climate difference between the two countries might explain the inconsistent finding as sleep propensity and pattern were related to body temperature (43) which could be affected by climates (44). A study in the U.S. showed that college students tended to be restorative nappers rather than appetitive nappers (78% vs. 22%) (45), in contrast to our finding. Participants in this study were middle-aged or older adults and were mainly retired (88.1%). They were less likely to have nighttime sleep loss and thus had lower need for restorative napping. In comparison, they might have more free time to nap for enjoyment and easier to form a habit (46).
In this sample, we did not find a significant association between napping frequency with HbA1c. Association between napping frequency and glycemic metabolism has been examined in a variety of populations, demonstrating conflicting results. One study conducted in pregnant women (aged 23 years) found that napping frequency did not have a significant association with 1-h OGTT values or hyperglycemia (N=63) (47). High napping frequency was found associated with an increased risk of developing T2D (N=435,342) (aged 56 years, 55% females) (48). Compared with non-nappers, people with regular napping were 1.3 times more likely to have poor glycemic control (N=12,997) (aged 59 years, 38% females) (49). Based on a recent meta-analysis, compared with non-nappers, nappers had a 20% increased risks of developing T2D (50). How napping frequency affect glucose metabolism has not been clear. Alterations of melatonin may be a potential mechanism. Melatonin level was found to be elevated temporarily after a 2-hour nap during 12-hour simulated night work (51). As melatonin could improve insulin resistance, napping may thus be beneficial for glycemic control (52). Adiposity may play another role as it attenuated the association between napping and diabetes risk (53). In this study, we controlled for BMI and did not find a significant relationship. This null finding may be explained by the relatively small sample. Future studies with a larger sample are needed.
In this study, we found that long napping duration was associated with higher HbA1c. Long napping duration may be a reflection of impaired nighttime sleep. We thus controlled for the potential confounding effect of night sleep (e.g., sleep duration and insomnia symptoms). The association remained significant. This finding is consistent with the one from a study (aged 64 years, 53% females) indicating that those having napping duration of over 1 hour had a higher risk of developing diabetes and having impaired fasting glucose (23). Several reasons may explain this finding. Based on a meta-analysis, napping duration of over 1 hour was associated with an increased occurrence of obesity, possibly caused by decreased energy expenditure (54), and obesity was a predictor of poor glycemic control (55). In addition, long daytime napping may influence nighttime sleep by disturbing circadian rhythmicity (56). For example, long napping duration was found related to later bedtimes (57), increased nighttime awakenings, shorter nighttime sleep, lower sleep efficiency (58), and poorer sleep quality (59). Impaired night sleep may affect glucose metabolism through increased nocturnal cortisol concentration and sympathetic nervous system activity (60).
Compared with afternoon napping, morning napping was associated with a higher level of HbA1c. Current evidence on the timing of napping in people with T2D has been limited. Typically, napping is most likely to occur in the afternoon accompanied with the highest level of daytime sleepiness or sleep propensity (61). Afternoon napping thus may be of better quality and have bigger restorative effect that meets people’s needs of relieving sleepiness. In comparison, morning napping may be indicative of disturbed night sleep. Overall, the detrimental effect of impaired nocturnal sleep may outweigh the restorative effect of daytime napping. However, our analysis controlled for potential confounding effect of nocturnal sleep, suggesting that the effect of napping could be independent of night sleep. How the timing of napping may affect cardiometabolic health remains unclear, warranting further investigation.
To the best of our knowledge, this study was among the first that examined the relationship between type of napping and glucose metabolism. Compared with appetitive napping, restorative napping was related to better glycemic control. Restorative naps are naps taken in compensation for previous sleep loss, while appetitive naps indicate naps taken purely for psychological satisfaction or enjoyment other than sleep needs. Based on a previous study, appetitive nappers had more stage 1 sleep than restorative nappers (62), suggesting worse sleep quality. In addition, restorative nappers tend to take naps on those days when for the preceding one or more days they reported lacking sleep (45). Sleep deprivation may induce inflammation and cytokine secretion (e.g., IL-1β and TNF-α) in diabetic islets, impairing β cell function and insulin secretion (63, 64). As daytime napping is associated with immune recovery by preserving energy (65), taking restorative naps in response to “sleep debt” may return immune cytokines to baseline levels accompanied with subsequent nighttime sleep (62). Interestingly, based on previous evidence, appetitive nappers had higher sleep needs, slept longer than restorative nappers at night. They nap frequently without shortened nighttime sleep, but can also fall asleep easily (62). Indeed, the nighttime sleep duration of appetitive nappers in this study was longer than that in restorative nappers (6.6 h vs. 5.8 h), and appetitive nappers had a higher napping frequency than that in restorative nappers (69.5% vs. 36.7% napped 5-7 day/weeks). It is likely that in appetitive nappers, their longer sleep time throughout the day could decrease energy expenditure (54) and disturb circadian rhythmicity (56) and thus contribute to poorer glycemic control.
A major strength of this study was a thorough investigation of napping, especially the timing and type of napping, among patients with T2D. We also controlled for potential confounders, including night sleep, depression, and cognitive impairment. However, there are several limitations to this study. Participants were recruited from a community health care center. Their average age was 67 years, and most of them were retired, limiting the representativeness of the sample. Study findings cannot be generalized to the younger population. Different associations have been reported between napping and cardiometabolic health in young and middle-aged adults vs. older adults (66). In addition, exclusion criteria and napping were captured by self-report, which could introduce recall bias. We used HbA1c as a measure of overall glycemic control during the past three months, which only provides a snapshot, precluding us from having a closer examination of how daytime napping may influence glucose metabolism. Lastly, causality cannot be determined due to the cross-sectional design.
Despite the above limitations, this study has implications for future research and clinical practice. In the future, studies conducted in young and middle-aged adults are needed to confirm findings from this study as they may have different napping behaviors. Future research may consider using ecological momentary assessment for napping and glucose to provide a more complete picture (e.g., sleep dairy, actigraphy, and continuous glucose monitor). Longitudinal and interventional studies targeting napping are needed to shed lights on the causal relationship between napping and glycemic control as well as the underlying mechanism (e.g., inflammation). Meanwhile, in clinical practice, healthcare professionals may offer tips about napping, e.g., taking a nap less than an hour, taking a nap in the afternoon instead of in the morning, avoiding appetitive napping.
In summary, this study suggests that daytime napping, when arranged inappropriately, might have detrimental effect on glycemic control among patients with T2D. Specifically, those with a long napping duration, morning napping timing, and appetitive napping may have a higher level of HbA1c. The present study provides new insights into glucose management and sleep-related intervention among people with diabetes. Meanwhile, further research is needed to establish the causal link between napping characteristics and glycemic control and investigate the potential underlying mechanisms.
The raw data supporting the conclusions of this article will be made available by the authors, without undue reservation.
The studies involving humans were approved by the Institutional Review Board of Shanghai Jiao Tong University School of Medicine (#SJUPN-201811). The studies were conducted in accordance with the local legislation and institutional requirements. The participants provided their written informed consent to participate in this study.
JY: Data curation, Formal analysis, Investigation, Methodology, Resources, Software, Writing – original draft, Writing – review & editing. JW: Data curation, Formal analysis, Investigation, Methodology, Resources, Software, Writing – original draft, Writing – review & editing. YC: Investigation, Resources, Writing – original draft, Writing – review & editing. MZ: Investigation, Resources, Writing – original draft, Writing – review & editing. AZ: Investigation, Writing – original draft, Writing – review & editing. JD: Methodology, Writing – original draft, Writing – review & editing. JZ: Writing – original draft, Writing – review & editing. FL: Writing – original draft, Writing – review & editing. YW: Writing – original draft, Writing – review & editing. PC: Writing – original draft, Writing – review & editing. BZ: Conceptualization, Data curation, Formal analysis, Funding acquisition, Investigation, Methodology, Project administration, Resources, Software, Supervision, Validation, Visualization, Writing – original draft, Writing – review & editing.
The author(s) declare financial support was received for the research, authorship, and/or publication of this article. This work was supported by the National Natural Science Foundation of China [71904119]. Bingqian Zhu was supported by Shanghai Municipal Education Commission “Young Eastern Scholar” and “Shanghai Jiao Tong University School of Medicine-Nursing Development Program”. The funding sources have no roles in study design; in the collection, analysis and interpretation of data; in the writing of the report; and in the decision to submit the article for publication.
We would like to thank Dr. Sirimon Reutrakul from the University of Illinois Chicago College of Medicine for the constructive feedback on this manuscript.
The authors declare that the research was conducted in the absence of any commercial or financial relationships that could be construed as a potential conflict of interest.
All claims expressed in this article are solely those of the authors and do not necessarily represent those of their affiliated organizations, or those of the publisher, the editors and the reviewers. Any product that may be evaluated in this article, or claim that may be made by its manufacturer, is not guaranteed or endorsed by the publisher.
The Supplementary Material for this article can be found online at: https://www.frontiersin.org/articles/10.3389/fendo.2024.1361906/full#supplementary-material
1. International Diabetes Federation. Diabetes Atlas . (2021). Available online at: https://diabetesatlas.org/regional-factsheets/ (Accessed August 7 2023).
2. World Health Organization. The top 10 causes of death (2020). Available online at: https://www.who.int/news-room/fact-sheets/detail/the-top-10-causes-of-death (Accessed July 28 2023).
3. Kim YA, Lee Y, Seo JH. Renal complication and glycemic control in korean veterans with type 2 diabetes: A 10-year retrospective cohort study. J Diabetes Res. (2020) 2020:9806790. doi: 10.1155/2020/9806790
4. Laiteerapong N, Ham SA, Gao Y, Moffet HH, Liu JY, Huang ES, et al. The legacy effect in type 2 diabetes: impact of early glycemic control on future complications (The diabetes & Aging study). Diabetes Care. (2019) 42:416–26. doi: 10.2337/dc17-1144
5. Hovestadt I, Kiess W, Lewien C, Willenberg A, Poulain T, Meigen C, et al. HbA1c percentiles and the association between BMI, age, gender, puberty, and HbA1c levels in healthy German children and adolescents. Pediatr Diabetes. (2022) 23:194–202. doi: 10.1111/pedi.13297
6. Verma M, Paneri S, Badi P, Raman PG. Effect of increasing duration of diabetes mellitus type 2 on glycated hemoglobin and insulin sensitivity. Indian J Clin Biochem. (2006) 21:142–6. doi: 10.1007/bf02913083
7. Shita NG, Iyasu AS. Glycemic control and its associated factors in type 2 diabetes patients at Felege Hiwot and Debre Markos Referral Hospitals. Sci Rep. (2022) 12:9459. doi: 10.1038/s41598-022-13673-5
8. Geng T, Zhu K, Lu Q, Wan Z, Chen X, Liu L, et al. Healthy lifestyle behaviors, mediating biomarkers, and risk of microvascular complications among individuals with type 2 diabetes: A cohort study. PloS Med. (2023) 20:e1004135. doi: 10.1371/journal.pmed.1004135
9. Lustman PJ, Anderson RJ, Freedland KE, de Groot M, Carney RM, Clouse RE. Depression and poor glycemic control: a meta-analytic review of the literature. Diabetes Care. (2000) 23:934–42. doi: 10.2337/diacare.23.7.934
10. Okura T, Heisler M, Langa KM. Association between cognitive function and social support with glycemic control in adults with diabetes mellitus. J Am Geriatr Soc. (2009) 57:1816–24. doi: 10.1111/j.1532-5415.2009.02431.x
11. Dinges DF. Napping patterns and effects in human adults. In: Sleep and alertness: chronobiological, behavioral and medical aspects of napping. Raven Press, New York (1989). p. 171–204.
12. Liu J, Richmond RC, Bowden J, Barry C, Dashti HS, Daghlas I, et al. Assessing the causal role of sleep traits on glycated hemoglobin: A mendelian randomization study. Diabetes Care. (2022) 45:772–81. doi: 10.2337/dc21-0089
13. Broughton R. D.D. Napping: a ubiquitous enigma. In: Dinges DF, Broughton RJ, editors. Sleep and alertness: chronobiological, behavioral and medical aspects of napping. Raven Press, New York (1989). p. 1–7.
14. Duggan KA, McDevitt EA, Whitehurst LN, Mednick SC. To nap, perchance to DREAM: A factor analysis of college students’ Self-reported reasons for napping. Behav Sleep Med. (2018) 16:135–53. doi: 10.1080/15402002.2016.1178115
15. Du J, Wang Y, Xu S, Huang Y, Zhang R, Xiao L, et al. Structural model of napping motivation among chinese college students based on self-rating: evidence from an exploratory factor analysis. Nat Sci Sleep. (2022) 14:843–53. doi: 10.2147/nss.S349013
16. Lee SWH, Ng KY, Chin WK. The impact of sleep amount and sleep quality on glycemic control in type 2 diabetes: A systematic review and meta-analysis. Sleep Med Rev. (2017) 31:91–101. doi: 10.1016/j.smrv.2016.02.001
17. Wang C, Bangdiwala SI, Rangarajan S, Lear SA, AlHabib KF, Mohan V, et al. Association of estimated sleep duration and naps with mortality and cardiovascular events: a study of 116 632 people from 21 countries. Eur Heart J. (2019) 40:1620–9. doi: 10.1093/eurheartj/ehy695
18. Gozashti MHM, Eslami NM, Radfar MHM, Pakmanesh HM. Sleep pattern, duration and quality in relation with glycemic control in people with type 2 diabetes mellitus. Iran J Med Sci. (2016) 41:531–8.
19. Makino S, Hirose S, Kakutani M, Fujiwara M, Nishiyama M, Terada Y, et al. Association between nighttime sleep duration, midday naps, and glycemic levels in Japanese patients with type 2 diabetes. Sleep Med. (2018) 44:4–11. doi: 10.1016/j.sleep.2017.11.1124
20. Bawadi H, Al Sada A, Al Mansoori N, Al Mannai S, Hamdan A, Shi Z, et al. Sleeping duration, napping and snoring in association with diabetes control among patients with diabetes in Qatar. Int J Environ Res Public Health. (2021) 18(8):4017. doi: 10.3390/ijerph18084017
21. Suárez-Torres I, García-García F, Morales-Romero J, Melgarejo-Gutiérrez M, Demeneghi-Marini VP, Luna-Ceballos RI, et al. Poor quality of sleep in Mexican patients with type 2 diabetes and its association with lack of glycemic control. Prim Care Diabetes. (2023) 17:155–60. doi: 10.1016/j.pcd.2023.01.011
22. Izci Balserak B, Jackson N, Ratcliffe SA, Pack AI, Pien GW. Sleep-disordered breathing and daytime napping are associated with maternal hyperglycemia. Sleep Breath. (2013) 17:1093–102. doi: 10.1007/s11325-013-0809-4
23. Fang W, Li Z, Wu L, Cao Z, Liang Y, Yang H, et al. Longer habitual afternoon napping is associated with a higher risk for impaired fasting plasma glucose and diabetes mellitus in older adults: results from the Dongfeng-Tongji cohort of retired workers. Sleep Med. (2013) 14:950–4. doi: 10.1016/j.sleep.2013.04.015
24. Saylor J, Ji X, Calamaro CJ, Davey A. Does sleep duration, napping, and social jetlag predict hemoglobin A1c among college students with type 1 diabetes mellitus? Diabetes Res Clin Pract. (2019) 148:102–9. doi: 10.1016/j.diabres.2019.01.007
25. WHO. Definition, diagnosis and classification of diabetes mellitus and its complications: report of a WHO consultation. In: Part 1. Diagnosis and classification of diabetes mellitus. World Health Organization, Geneva, Switzerland (1999).
26. Mayega RW, Guwatudde D, Makumbi FE, Nakwagala FN, Peterson S, Tomson G, et al. Comparison of fasting plasma glucose and haemoglobin A1c point-of-care tests in screening for diabetes and abnormal glucose regulation in a rural low income setting. Diabetes Res Clin Pract. (2014) 104:112–20. doi: 10.1016/j.diabres.2013.12.030
27. Lovato N, Lack L, Wright H. The napping behaviour of Australian university students. PloS One. (2014) 9:e113666. doi: 10.1371/journal.pone.0113666
28. Liu J, Feng R, Ji X, Cui N, Raine A, Mednick SC. Midday napping in children: associations between nap frequency and duration across cognitive, positive psychological well-being, behavioral, and metabolic health outcomes. Sleep. (2019) 42(9):zsz126. doi: 10.1093/sleep/zsz126
29. Yamada T, Shojima N, Yamauchi T, Kadowaki T. J-curve relation between daytime nap duration and type 2 diabetes or metabolic syndrome: A dose-response meta-analysis. Sci Rep. (2016) 6:38075. doi: 10.1038/srep38075
30. Häusler N, Marques-Vidal P, Haba-Rubio J, Heinzer R. Does sleep predict next-day napping or does napping influence same-day nocturnal sleep? Results of a population-based ecological momentary assessment study. Sleep Med. (2019) 61:31–6. doi: 10.1016/j.sleep.2019.04.014
31. Botonis PG, Koutouvakis N, Toubekis AG. The impact of daytime napping on athletic performance - A narrative review. Scand J Med Sci Sports. (2021) 31:2164–77. doi: 10.1111/sms.14060
32. Bastien CH, Vallières A, Morin CM. Validation of the Insomnia Severity Index as an outcome measure for insomnia research. Sleep Med. (2001) 2:297–307. doi: 10.1016/s1389-9457(00)00065-4
33. Morin CM, Belleville G, Bélanger L, Ivers H. The Insomnia Severity Index: psychometric indicators to detect insomnia cases and evaluate treatment response. Sleep. (2011) 34:601–8. doi: 10.1093/sleep/34.5.601
34. Li Enze LW, Xi Z, Zhang B. Psychometric property of the Insomnia Severity Index in students of a commercial school. J Neurosci Ment Health. (2019) 19:268–72. doi: 10.3969/j.issn.1009-6574.2019.03.012
35. Ai S, Zhang J, Zhao G, Wang N, Li G, So HC, et al. Causal associations of short and long sleep durations with 12 cardiovascular diseases: linear and nonlinear Mendelian randomization analyses in UK Biobank. Eur Heart J. (2021) 42:3349–57. doi: 10.1093/eurheartj/ehab170
36. Nasreddine ZS, Phillips NA, Bédirian V, Charbonneau S, Whitehead V, Collin I, et al. The Montreal Cognitive Assessment, MoCA: a brief screening tool for mild cognitive impairment. J Am Geriatr Soc. (2005) 53:695–9. doi: 10.1111/j.1532-5415.2005.53221.x
37. Lu J, Li D, Li F, Zhou A, Wang F, Zuo X, et al. Montreal cognitive assessment in detecting cognitive impairment in Chinese elderly individuals: a population-based study. J Geriatr Psychiatry Neurol. (2011) 24:184–90. doi: 10.1177/0891988711422528
38. Kroenke K, Spitzer RL, Williams JBW. The PHQ-9: Validity of a brief depression severity measure. J Gen Internal Med. (2001) 16:606–13. doi: 10.1046/j.1525-1497.2001.016009606.x
39. Zuo P, Shi J, Yan J, Yang L, Liu C, Yuan L, et al. Effects of insulin therapy and oral hypoglycemic agents on glycemic control for type 2 diabetes mellitus patients in China-A case control study. Exp Clin Endocrinol Diabetes. (2021) 129:374–8. doi: 10.1055/a-0881-9611
40. Shibabaw YY, Dejenie TA, Tesfa KH. Glycemic control and its association with sleep quality and duration among type 2 diabetic patients. Metabol Open. (2023) 18:100246. doi: 10.1016/j.metop.2023.100246
41. Lan TY, Lan TH, Wen CP, Lin YH, Chuang YL. Nighttime sleep, Chinese afternoon nap, and mortality in the elderly. Sleep. (2007) 30:1105–10. doi: 10.1093/sleep/30.9.1105
42. Yao X, Lu F, Wang Z, Miao Y, Feng Q, Zhang Y, et al. Association of sleep behaviors, insulin resistance surrogates, and the risk of hypertension in Chinese adults with type 2 diabetes mellitus. Front Endocrinol (Lausanne). (2023) 14:1212878. doi: 10.3389/fendo.2023.1212878
43. Gilbert SS, van den Heuvel CJ, Ferguson SA, Dawson D. Thermoregulation as a sleep signalling system. Sleep Med Rev. (2004) 8:81–93. doi: 10.1016/s1087-0792(03)00023-6
44. Andrei VT, Rofail SR. Adaptation to marine climate. In: Marine climate of Russian coastal territories, vol. 37. Springer, Switzerland (2023).
45. Evans FJ, Cook MR, Cohen HD, Orne EC, Orne MT. Appetitive and replacement naps: EEG and behavior. Science. (1977) 197:687–9. doi: 10.1126/science.17922
46. Myllyntausta S, Salo P, Kronholm E, Pentti J, Oksanen T, Kivimäki M, et al. Does removal of work stress explain improved sleep following retirement? The Finnish Retirement and Aging study. Sleep. (2019) 42(8):zsz109. doi: 10.1093/sleep/zsz109
47. Herring SJ, Nelson DB, Pien GW, Homko C, Goetzl LM, Davey A, et al. Objectively measured sleep duration and hyperglycemia in pregnancy. Sleep Med. (2014) 15:51–5. doi: 10.1016/j.sleep.2013.07.018
48. Zhou R, Chen HW, Huang YN, Zhong Q, Li FR, Huang RD, et al. The association between daytime napping and risk of type 2 diabetes is modulated by inflammation and adiposity: Evidence from 435 342 UK-Biobank participants. J Diabetes. (2023) 15:496–507. doi: 10.1111/1753-0407.13387
49. Xue P, Tang X, Tan X, Benedict C. Self-reported regular daytime napping is associated with indicators of poor type 2 diabetes control: A cohort study. Diabetes Obes Metab. (2022) 24:737–41. doi: 10.1111/dom.14619
50. Liu M, Liu M, Wang S, Sun Y, Zhou F, Sun H. Relationship between daytime napping with the occurrence and development of diabetes: a systematic review and meta-analysis. BMJ Open. (2023) 13:e068554. doi: 10.1136/bmjopen-2022-068554
51. Lee SI, Nishi T, Takahashi M, Higuchi S. Effects of 2-hour nighttime nap on melatonin concentration and alertness during 12-hour simulated night work. Ind Health. (2021) 59:393–402. doi: 10.2486/indhealth.2020-0245
52. Heo JI, Yoon DW, Yu JH, Kim NH, Yoo HJ, Seo JA, et al. Melatonin improves insulin resistance and hepatic steatosis through attenuation of alpha-2-HS-glycoprotein. J Pineal Res. (2018) 65:e12493. doi: 10.1111/jpi.12493
53. Xu Q, Song Y, Hollenbeck A, Blair A, Schatzkin A, Chen H. Day napping and short night sleeping are associated with higher risk of diabetes in older adults. Diabetes Care. (2010) 33:78–83. doi: 10.2337/dc09-1143
54. Cai Z, Yang Y, Zhang J, Liu Y. The relationship between daytime napping and obesity: a systematic review and meta-analysis. Sci Rep. (2023) 13:12124. doi: 10.1038/s41598-023-37883-7
55. Boye KS, Lage MJ, Thieu V, Shinde S, Dhamija S, Bae JP. Obesity and glycemic control among people with type 2 diabetes in the United States: A retrospective cohort study using insurance claims data. J Diabetes Complications. (2021) 35:107975. doi: 10.1016/j.jdiacomp.2021.107975
56. Feinberg I, Maloney T, March JD. Precise conservation of NREM period 1 (NREMP1) delta across naps and nocturnal sleep: implications for REM latency and NREM/REM alternation. Sleep. (1992) 15:400–3. doi: 10.1093/sleep/15.5.400
57. Rea EM, Nicholson LM, Mead MP, Egbert AH, Bohnert AM. Daily relations between nap occurrence, duration, and timing and nocturnal sleep patterns in college students. Sleep Health. (2022) 8:356–63. doi: 10.1016/j.sleh.2022.05.002
58. Owens JF, Buysse DJ, Hall M, Kamarck TW, Lee L, Strollo PJ, et al. Napping, nighttime sleep, and cardiovascular risk factors in mid-life adults. J Clin Sleep Med. (2010) 6:330–5. doi: 10.5664/jcsm.27873
59. Ye L, Hutton Johnson S, Keane K, Manasia M, Gregas M. Napping in college students and its relationship with nighttime sleep. J Am Coll Health. (2015) 63:88–97. doi: 10.1080/07448481.2014.983926
60. Spiegel K, Leproult R, Van Cauter E. Impact of sleep debt on metabolic and endocrine function. Lancet. (1999) 354:1435–9. doi: 10.1016/s0140-6736(99)01376-8
61. Bes F, Jobert M, Schulz H. Modeling napping, post-lunch dip, and other variations in human sleep propensity. Sleep. (2009) 32:392–8. doi: 10.1093/sleep/32.3.392
62. Faraut B, Boudjeltia KZ, Dyzma M, Rousseau A, David E, Stenuit P, et al. Benefits of napping and an extended duration of recovery sleep on alertness and immune cells after acute sleep restriction. Brain Behav Immun. (2011) 25:16–24. doi: 10.1016/j.bbi.2010.08.001
63. Kägi D, Ho A, Odermatt B, Zakarian A, Ohashi PS, Mak TW. TNF receptor 1-dependent beta cell toxicity as an effector pathway in autoimmune diabetes. J Immunol. (1999) 162:4598–605.
64. Ehses JA, Perren A, Eppler E, Ribaux P, Pospisilik JA, Maor-Cahn R, et al. Increased number of islet-associated macrophages in type 2 diabetes. Diabetes. (2007) 56:2356–70. doi: 10.2337/db06-1650
65. Faraut B, Nakib S, Drogou C, Elbaz M, Sauvet F, De Bandt JP, et al. Napping reverses the salivary interleukin-6 and urinary norepinephrine changes induced by sleep restriction. J Clin Endocrinol Metab. (2015) 100:E416–426. doi: 10.1210/jc.2014-2566
Keywords: diabetes, nap, sleep, glycemic control, HbA1c
Citation: Yuan J, Wang J, Chen Y, Zhang M, Zhao A, Du J, Zhang J, Liu F, Wang Y, Chen P and Zhu B (2024) The relationship between daytime napping and glycemic control in people with type 2 diabetes. Front. Endocrinol. 15:1361906. doi: 10.3389/fendo.2024.1361906
Received: 27 December 2023; Accepted: 19 February 2024;
Published: 05 March 2024.
Edited by:
Shinya Makino, Osaka Gyomeikan Hospital, JapanReviewed by:
Haitham Jahrami, Arabian Gulf University, BahrainCopyright © 2024 Yuan, Wang, Chen, Zhang, Zhao, Du, Zhang, Liu, Wang, Chen and Zhu. This is an open-access article distributed under the terms of the Creative Commons Attribution License (CC BY). The use, distribution or reproduction in other forums is permitted, provided the original author(s) and the copyright owner(s) are credited and that the original publication in this journal is cited, in accordance with accepted academic practice. No use, distribution or reproduction is permitted which does not comply with these terms.
*Correspondence: Bingqian Zhu, emh1YnFAc2hzbXUuZWR1LmNu
†These authors have contributed equally to this work and share first authorship
‡ORCID: Bingqian Zhu, orcid.org/0000-0003-3997-7882
Disclaimer: All claims expressed in this article are solely those of the authors and do not necessarily represent those of their affiliated organizations, or those of the publisher, the editors and the reviewers. Any product that may be evaluated in this article or claim that may be made by its manufacturer is not guaranteed or endorsed by the publisher.
Research integrity at Frontiers
Learn more about the work of our research integrity team to safeguard the quality of each article we publish.