- 1Department of Blood Transfusion, the First Affiliated Hospital of Fujian Medical University, Fuzhou, Fujian, China
- 2Department of Blood Transfusion, National Regional Medical Center, Binhai Campus of the First Affiliated Hospital, Fujian Medical University, Fuzhou, Fujian, China
- 3The Center of Information, the First Affiliated Hospital of Fujian Medical University, Fuzhou, Fujian, China
- 4The Center of Information, National Regional Medical Center, Binhai Campus of the First Affiliated Hospital, Fujian Medical University, Fuzhou, Fujian, China
Background: Diabetes mellitus (DM) stands as the most prevalent endocrine abnormality affecting the physiological systems and organs and impairing the male reproductive functions. Type 2 Diabetes Mellitus (T2DM), accounting for about 90-95% of DM, is closely associated with male infertility. However, the magnitude of the causal relationships between T2DM and male infertility remains unclear. The current investigation was to explore the causal relationship between T2DM and male infertility utilizing the Mendelian Randomization (MR) analysis.
Methods: A two-sample MR (2SMR) analysis was conducted to investigate the causal relationship between T2DM and male infertility in the European population from the genome-wide association study (GWAS) summary data that was publicly accessible. GWAS for T2DM and male infertility were extracted from the IEU Open GWAS Project database, with the resulting data encompassing 680 cases and 72,799 controls as the outcome data. Five MR methods were employed for the 2SMR analyses, namely the MR-Egger, weighted median estimation (WME), weighted mode (WM), inverse-variance weighted (IVW), and simple mode. The primary analytical technique utilized in this study was the IVW method, and a multivariate MR analysis was executed to examine the potential mediating influences of T2DM on male infertility.
Results: Following were the odds ratios (ORs) and associated 95% CIs derived from IVW (fixed effects), MR-Egger, WM, WME, and simple mode approaches: 0.824 (95% CI 0.703-0.966), 0.726 (95% CI 0.527-1.001), 0.827 (95% CI 0.596-1.150), 0.841 (95% CI 0.654-1.082), and 0.875 (95% CI 0.544-1.405), respectively. The outcomes of the heterogeneity tests were P=0.378 and P=0.384, respectively, implying no heterogeneity. Egger-intercept outcomes were P=0.374, highlighting the absence of pleiotropy. The stability of the results was affirmed through the leave-one-out analysis. Notably, all F-values surpassed 10, indicating the absence of weak bias attributed to instrument variables(IVs).
Conclusions: This research furnishes evidence supporting a causal association between T2DM and male infertility. These insights offer a foundation for future investigations aiming to establish the association between genetically predicted T2DM and male infertility. These outcomes suggest the significance of active monitoring and proactive measures for preventing infertility in male individuals with T2DM. Furthermore, careful consideration is required for individuals of reproductive age to prevent and treat T2DM.
1 Introduction
Diabetes mellitus (DM) has emerged as a significant global health challenge marked by elevated morbidity and mortality rates. The prevalence of DM is steadily rising, impacting a growing number of young and middle-aged males. Presently, DM affects approximately 422 million individuals globally and is reported to contribute to 1.5 million fatalities annually (1, 2). DM has reached epidemic proportions in the past. The prevalence of DM has witnessed a substantial escalation, escalating from 108 million cases in 1980 to 537 million cases by the year 2021. Predictions suggest a further increase, estimating that 643 million individuals will have DM by 2030 and 783 million will develop this disease by 2045 (3). Diabetes can be classified into four types based on etiology and pathology, including type 1 diabetes mellitus (T1DM; approximately 5-10% of the DM cases), type 2 diabetes mellitus (T2DM; approximately 90-95% of the DM cases), “other” causes of DM (<5% of the DM cases), and gestational diabetes mellitus (GDM). Recent estimates indicate that the prevalence of DM in the general population of the United States is 9.7%, with T2DM and T1DM constituting 8.5% and 0.5% of the cases, respectively (3). DM, particularly T2DM, exhibits a higher prevalence among males compared to females (4). T2DM exerts a considerable impact on numerous physiological systems and tissues, including the male reproductive organs. As the age of individuals diagnosed with T2DM has been progressively decreasing in recent years, an escalating number of young and middle-aged males are struggling with this disease during their reproductive years.
According to the World Health Organization (WHO), infertility has progressively evolved into a public health concern, presenting a prevalence of around 10%-15%. Notably, the male factor contributes to approximately 40% of infertility cases (5), impacting around one in ten couples within the reproductive age range (6). Declining world fertility rates may have a serious negative impact on social development. Predictions suggest that the worldwide population is expected to reach around 9.7 billion by the year 2064. Nevertheless, it will decrease to approximately 8.8 billion in 2100 (7). Pure or mixed male factor has been recognized in approximately half of the infertility cases, with estimates indicating that up to 12% of males experience fertility issues (6, 8). The etiology of male infertility may be associated with congenital or acquired conditions, spanning a range of factors encompassing pretesticular, testicular, or post-testicular causes (6, 8). Among the different causes of infertility, DM, severe ejaculatory disorders, and erectile dysfunction were considered pretesticular causes of infertility (6). The prevalence of DM in infertile males is estimated to be 0.7%-1.4%. However, some studies have reported that the prevalence of infertility in males with DM ranges from approximately 35%-51%. DM in males appears to exert a discernible adverse impact on the fertility of couples, wherein the status of being childless or subfertile in males may be associated with an elevated risk of developing DM (9). Recent studies have brought to light a substantial correlation between DM and sexual dysfunctions (3, 10), specifically, erectile (3, 11) and ejaculatory dysfunctions (12) as well as hypogonadism (11). Available data underscores the evident roles that DM plays in impairing male reproductive organs, thereby influencing overall couple fertility (9, 13). Therefore, it is well known that the low fertility rate of DM patients in humans.
T2DM is the main type of DM cases. An extensive body of research, spanning both clinical observations and studies involving animals, has dedicated attention to exploring and elucidating the impact of T2DM on various aspects of sperm quality and its associated parameters (10, 14). Generally, the pathophysiological mechanisms of becoming infertile in T2DM is caused by an inflammatory condition with increased oxidative stress resulting in decreased sperm vitality and increased sperm DNA fragmentation (10). There is evidence that prevalence of younger patients with T2DM is estimated at 31% in 10-19 years (15). Nonetheless, findings from a Mendelian randomization (MR) study suggest that the elevated risk of spermatozoa abnormality in male Europeans may not be explained solely by T2DM (16). Subsequent studies necessitate larger sample sizes to elucidate the relationship and potential underlying mechanisms between T2DM and male infertility.
Several studies, encompassing both clinical observations and animal research, have substantiated the relationship between T2DM and male infertility (10, 14, 17). Nonetheless, the conclusion drawn from these studies regarding the causal relationship between T2DM and male infertility exhibited inconsistencies. The identified correlation risk in their findings fell short of offering a comprehensive account, leaving gaps in addressing potential confounding factors, such as socioeconomic status and diverse lifestyles. Consequently, an MR study was executed to examine the possible underlying causal relationship between T2DM and male infertility. MR analysis has emerged as a widely adopted tool for assessing the causal relationship between risk factors and outcomes. This sophisticated analytical approach capitalizes on genetic variants stemming from meiosis, effectively leveraging them as a natural experiment (18–20). Considering the random distribution of genetic variants at conception, MR analysis is less prone to bias from potential reverse causality and confounding compared to observational studies (21, 22).
In cases where data on exposure and outcome are measured in separate samples, an MR study can estimate causal effects through a two-sample MR (2SMR) approach (23). Due to the random classification of genotypes at conception, confounding and bias in 2SMR are limited (24). Hence, a 2SMR analysis was performed in this investigation to examine the causal association between T2DM as the exposure and male infertility as the outcome utilizing summary datasets from the genome-wide association studies (GWAS).
2 Methodology
2.1 Data sources
T2DM and male infertility data were retrieved from the IEU Open GWAS Project database (https://gwas.mrcieu.ac.uk/) to identify the most relevant GWAS summary data. Individuals of European ancestry were specifically selected for the cohort to mitigate potential errors arising from stratification effects related to factors like ancestry and population. Furthermore, in this study, a preference was given to GWAS data with a larger sample size, encompassing more single nucleotide polymorphisms (SNPs). The study selected SNPs associated with T2DM in Europeans from a GWAS analysis (GWAS-ID: finn-b-E4_DM2), comprising 32,469 patients with T2DM and 183,185 healthy controls of European ancestry (Table 1). In this research, genetically and statistically plausible SNPs meeting a genome-wide significance threshold of P < 5e-08 were selected. In this context, the genetic variants exhibited a strong association with T2DM in our 2SMR analysis. The F-statistic was utilized to examine the potential for weak instrumental bias and the statistical power of individual SNPs. To ensure the exclusion of weak instrument bias, an F-statistic cutoff value of F < 10 was applied (25). Genetic association data for male infertility in individuals of European ancestry were sourced from the IEU Open GWAS Project database. This dataset comprised a total of 680 individuals with male infertility and 72,799 country-matched non-DM participants of European ancestry (Table 1). This study determined the β coefficients and standard errors for overall male infertility associated with each retrieved SNP of T2DM from the GWAS summary statistics in the European population.
2.2 Instrumental variables
To ensure the effectiveness of instrumental variables (IVs), the SNPs used as IVs in the 2SMR analysis must satisfy the subsequent conditions (1): demonstrate a strong association with T2DM (2), exhibit no association with any confounding factors related to both T2DM and male infertility and (3) affect male infertility solely through the pathway of T2DM (Figure 1). Hence, the selection criteria to identify the effective SNPs were as follows (1): SNPs chosen as potential IVs were linked to each genus at the locus-wide significance threshold (P <5×10-8) (2). The linkage disequilibrium (LD) between the SNPs was calculated utilizing 1000 Genomes Project data for the European population as the reference panel. Out of these, SNPs exhibiting an r2<0.001 (clumping window size=10,000 kb) were retained, including only those with the most minimal P-values (3). SNPs with minor allele frequency (MAF) ≤0.01 were excluded, and in cases where palindromic SNPs were identified, the inferring of forward strand alleles was made utilizing allele frequency information.
2.3 Statistical analysis
2.3.1 Linkage disequilibrium assessment
Genetic variants employed as IVs in most MR methods must be independent of confounding factors, prohibiting the existence of LD. According to the hypothesis, the correlation LD between the chosen SNPs and potential confounding factors was assessed.
2.3.2 Main MR analysis
In our MR study, five approaches employing a multiplicative random effects model were utilized. These methods include Mendelian randomization-Egger regression (MR-Egger), weighted median estimation (WME), inverse-variance weighted (IVW), weighted mode (WM), and simple mode. Notably, the random effects IVW method stands out as the most extensively employed and accepted approach in MR analysis. This is attributed to its capability to consider a large number of SNPs and address the substantive observed heterogeneity during the analysis of causality (26, 27). The MR-Egger method offers a robust measure of causal effects that adjust for horizontal pleiotropy. This is achieved by pooling a single SNP-specific Wald ratio utilizing adaptive Egger regression (28, 29). The WME method offers a consistent estimate of causal effects by utilizing the weighted median of Wald under the condition that at least 50% of variants adhere to the criteria of a valid IV for the exclusion restrictions. Utilizing the estimation of individual proportions, the WM method categorizes SNPs based on their similarity and computes the counter-variance weighted count of SNPs in each group. Ultimately, it derives a causal estimate according to the group of SNPs by the largest weighted number (30). The simple mode method provides consistent estimates of causal effects if at least 50% of SNPs are valid (31).
2.3.3 Sensitivity and heterogeneity analysis
A leave-one-out sensitivity analysis was performed to examine the effect of individual SNPs on causal estimates. The examination of heterogeneity involved the utilization of Cochran’s Q statistic and the related P-values to ascertain the consistency of causal relationships across all SNPs. The consideration of smaller heterogeneity is deemed indicative of more reliable MR estimates, affirming the robustness and reliability of the causal inferences drawn from the analysis.
2.3.4 MR Pleiotropy Residual Sum and Outlier (MR-PRESSO) analysis
The MR-PRESSO analysis was employed to assess the pleiotropy effects of outlier SNPs and correct abnormal findings attributable to such outliers (32). This method involves regressing SNP outcomes on SNP exposure and utilizing the square of residuals to identify outliers. Firstly, the MR-PRESSO global test was utilized to determine heterogeneity and outliers. Then, the MR-PRESSO outlier test was used to correct for pleiotropy by eliminating outlier SNPs. Ultimately, the MR-PRESSO distortion test analyzed the causality difference before and after outlier SNP removal (32). All the analyses were performed utilizing the R “TwoSampleMR” (v0.5.7, Stephen Burgess, Chicago, IL, USA) for the 2SMR analysis between T2DM and male infertility.
3 Results
3.1 MR analysis
To investigate the role of T2DM in the risk of male infertility, 2SMR methods were employed to identify relevant genetic variants in this study. 62 SNPs among European ancestry participants were associated with T2DM at the significance level of P <5×10-8. Then 62 independent SNPs (F >10) surpassed the limited value (r2 <0.001) in LD analysis. Detailed information on the same of SNPs is also summarized Table 1.
The outcomes of the five 2SMR methods employed in this study are detailed in Table 2 and illustrated in Figure 2. Notably, the IVW method served as the primary approach for estimating the causal effects of T2DM on the risk of male infertility. The study results revealed a substantial causal association between T2DM and the risk of male infertility in the European population (IVW fixed effects method: OR 0.824, 95% CI 0.703-0.966; P=0.017) (Table 2 and Figure 2).
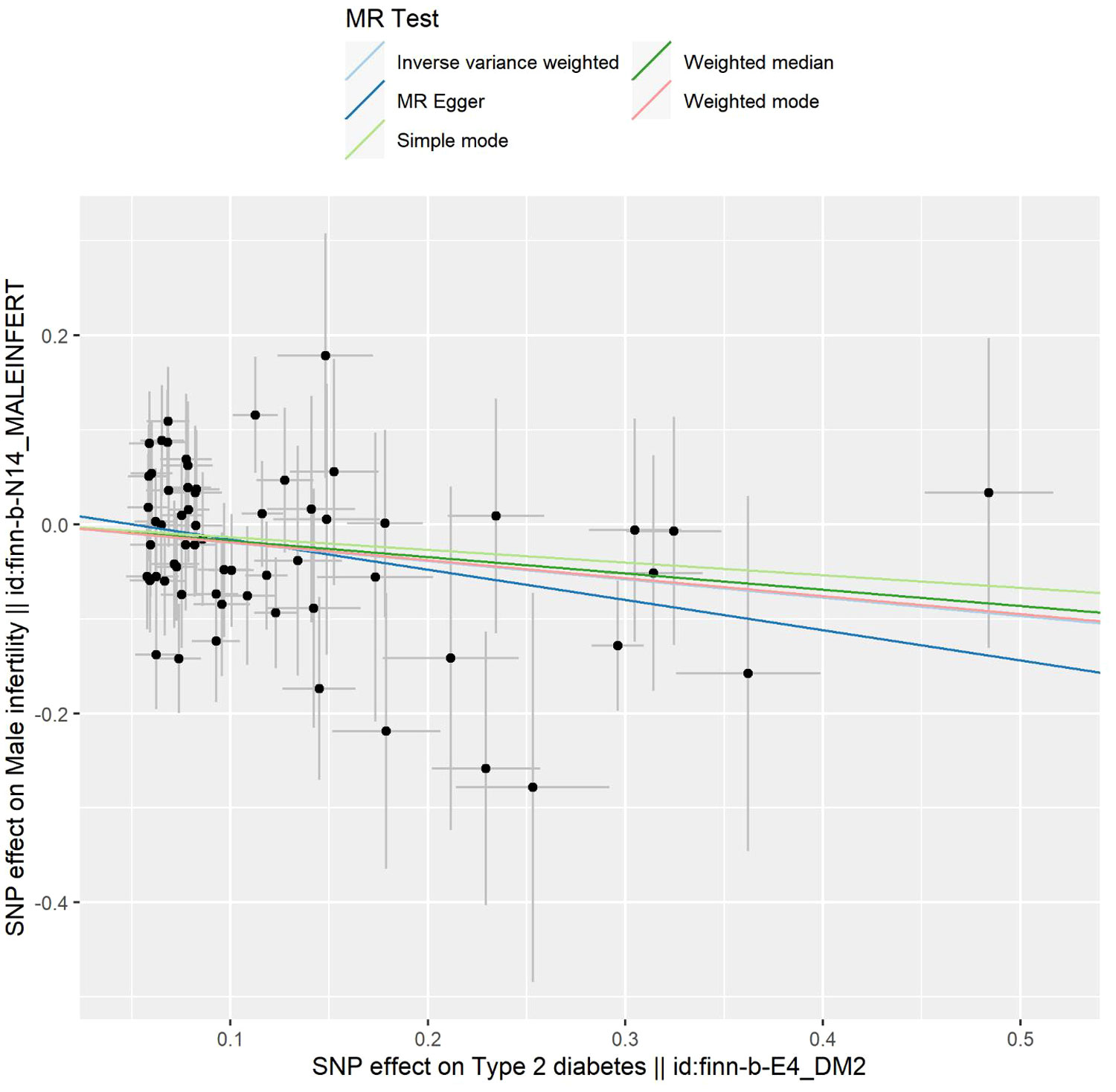
Figure 2 Scatter plot depicting the distribution of individual ratio estimates of type 2 diabetes mellitus with male infertility as the outcome. Trend lines generated from five different 2SMR methods are incorporated in all scatter plots to depict cause and effect.
3.2 Sensitivity and heterogeneity analysis
Sensitivity analysis was executed to validate the reliability of the outcomes obtained from the IVW method. The findings of the IVW and MR-Egger test for heterogeneity analysis indicated the absence of statistically significant heterogeneity between T2DM and male infertility in all five 2SMR analysis methods (P >0.05) (Table 3).
3.3 Further validation of MR results
In this study, the selection of SNPs adhered to the genome-wide significance level criterion of P <5×10–8, aligning with the first condition—the locus-wide significance threshold. As demonstrated by the leave-one-out analysis (Figure 3), individual SNPs were observed to potentially influence the results of the IVW analysis. Consequently, further verification of the IVW method results was undertaken. Furthermore, the results of the MR-Egger regression intercept analysis did not reveal any substantial directional horizontal pleiotropy (P >0.05) (Table 4). The MR-PRESSO analysis verified the absence of marked horizontal pleiotropy and outliers in this research(P>0.05) (Table 5), further affirming the validity of the MR findings. Overall, the outcomes of this research demonstrate a substantial causal relationship between T2DM and the risk of male infertility in the European population.
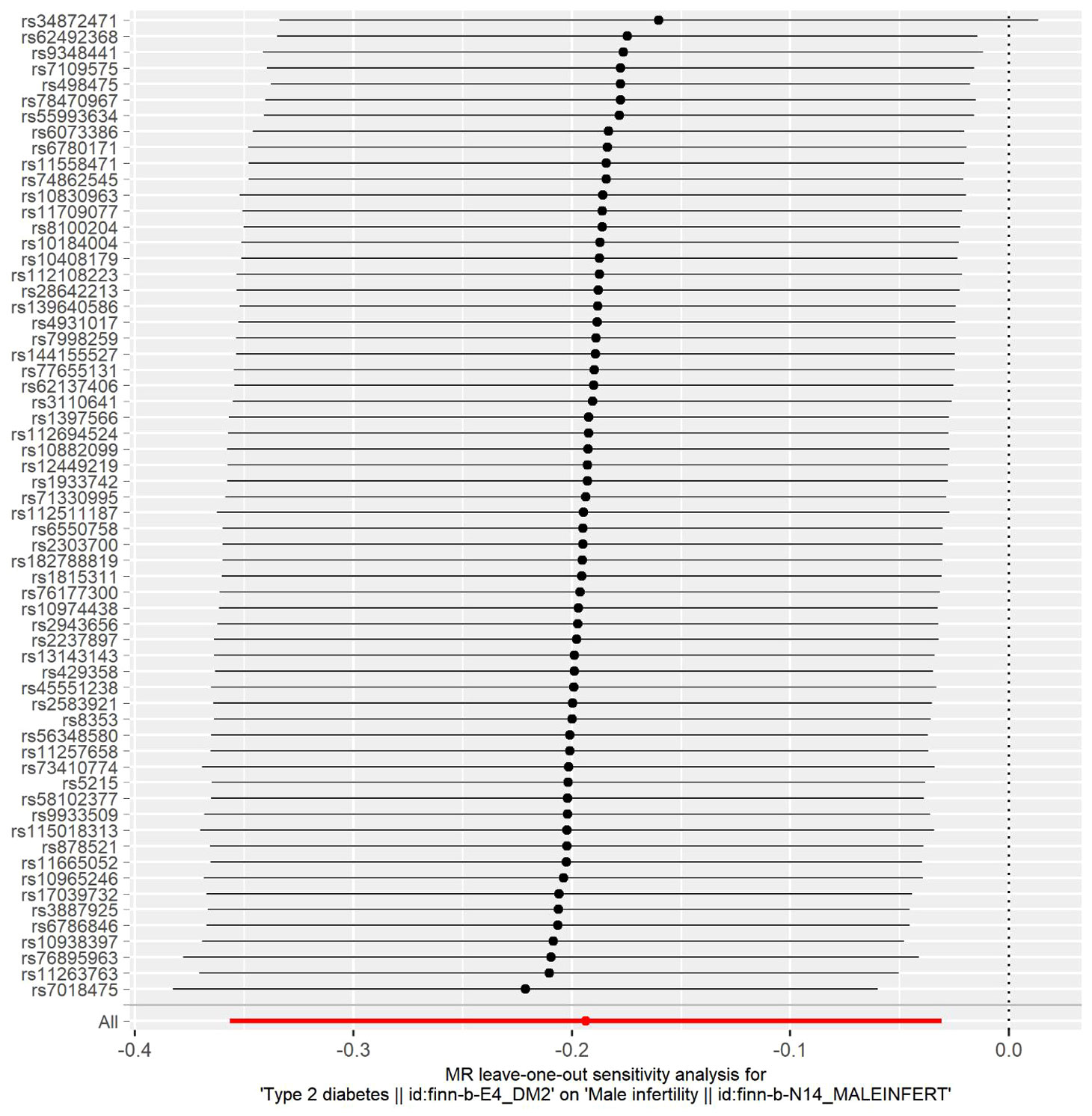
Figure 3 Leave-one-out sensitivity analysis for type 2 diabetes mellitus on male infertility. The dark dots in the visualization represent effect measures derived through IVW-MR analysis, with the exclusion of specific SNPs. Red lines denote the pooled analysis, incorporating all SNPs through the IVW-MR method, and are plotted for the purpose of comparison.
4 Discussion
In the present investigation, a 2SMR analysis was conducted using publicly available GWAS summary statistics data. The objective was to examine the causal relationship between T2DM and male infertility in the European population. As per the currently available literature, the present research appears to represent the initial attempt to examine and unveil a causal relationship between genetically predicted T2DM and the risk of male infertility in the European population. This contribution is deemed significant in providing insights into the mechanisms underlying the association between T2DM and male infertility.
DM can inflict permanent damage on multiple physiological systems and various organs, inclusive of the reproductive organs, potentially resulting in dysfunction or failure of these systems (33). The mechanism of diabetic testicular tissue damage includes glucose and lipid metabolism, oxidative stress, inflammatory response, endoplasmic reticulum stress, autophagy and so on. Eventually, the inflammatory infiltration of the testicular cells, the number of sperm, the decrease of energy, the obstruction of ejaculation, the decrease of male fertility. Presently, the rapid increase in the incidence of T2DM among adolescents, particularly males, is anticipated to significantly increase the prevalence of reproductive dysfunctions in males (34). In a retrospective analysis study, a 51% prevalence of subfertility was identified among individuals diagnosed with T2DM (35). It was reported that around 1.2% of infertile males had T2DM among a cohort of over 500 male partners of infertile couples (36). The prevalence of infertility in the male population with T2DM reached 35.1%, representing a significant increase compared to the normal population (37).
DM patients had higher risk of becoming male infertility and the mechanisms of damage reproduction were different in T1DM and T2DM (10). T1DM caused low ejaculate volume and mitochondrial damage resulting in decreased sperm motility. T2DM caused an inflammatory condition with increased oxidative stress, resulting in decreased sperm vitality and increased sperm DNA fragmentation (10). In addition, various inflammatory signaling pathways and cell growth signaling molecular mechanisms also affect the proliferation, differentiation and death of testicular cells. T2DM may lead to dysregulated spermatogenesis, impairment of erectile function and ejaculation disorders, thereby impairing male fertility (38, 39). Furthermore, numerous studies have shown that DM affects male fertilization by inducing reactive oxygen species (ROS), which negatively affects sperm development (40–42). However, the role of uncoupling proteins (UCPs) as key regulators of redox homeostasis and ROS production in the pathophysiology of diabesity, as well as their potential involvement in diabesity-induced male infertility, remains a subject of debate (43). In addition, the treatment of rats in T2DM restored steroidogenesis in their testes, leading to improve spermatogenesis (44). Other studies also showed that treatment of T2DM increased sperm survival in pigs and improved the quality of frozen sperm in dogs (45). However, in vivo studies from a variety of animal models were found that inconsistent effects of T2DM treatment on sperm count, concentration, morphology, viability and survival (46). The exact molecular mechanism of male infertility in diabetes is unknown, and no specific drug is available to treat it.
The acknowledgment of potential confounding factors in previous studies prompted our approach to utilize MR analysis in this study. Recognizing the primary advantage of MR in removing the impact of confounding factors, this research aimed to enhance the reliability of the outcomes (47). Nevertheless, the findings from an MR study indicated that the relatively elevated risk of abnormal spermatozoa in the European population cannot be solely explained by T2DM (16). Given the inconsistent findings observed in previous studies, it becomes imperative to conduct subsequent investigations with larger sample sizes to elucidate the relationship between T2DM and male infertility. Consequently, this research structured a 2SMR study to unveil the causal relationship between T2DM and the risk of male infertility in the European population. Multiple studies conducted in animals and humans have consistently highlighted the adverse effects of DM on male reproductive functions (34). The present research were consistent with previous research.
Nonetheless, the findings of the present research contrast the MR study (16) that analyzed the causal relationship between T2DM and abnormal spermatozoa in the European population. The differences between the two analyses arise from distinct exposures and outcomes, variations in data sources, and differences in the number of cases and SNPs considered. Firstly, the analyses focused on different outcomes, contributing to the divergent results. Secondly, dissimilar datasets were utilized in the two analyses. Thirdly, there was a discrepancy in the number of cases and SNPs between the studies. The prior analysis identified 17 SNPs associated with T2DM, and 9 independent SNPs related to the abnormal spermatozoa surpassed the limited value in LD analysis (16). However, this study identified 62 SNPs associated with T2DM, and among them, 62 independent SNPs related to male infertility surpassed the limited value. In general, the outcomes of the current research align with numerous studies analyzing the effects of T2DM.
This study possesses several strengths. The implementation of a 2SMR analysis provided a robust framework for the investigation of the causal association between T2DM and male infertility. This methodological approach served to exclude the interference of confounding factors and reverse causation on causal inference, thereby enhancing the validity of causal inferences. Moreover, the genetic variants linked to T2DM were sourced from the most extensive and comprehensive GWAS summary data available, ensuring the robustness and strength of the instruments employed in the MR analysis. Moreover, in this study, a thorough examination was conducted through leave-one-out sensitivity analysis and heterogeneity analysis. Horizontal pleiotropy, a potential confounding factor, was identified and addressed utilizing MR-PRESSO and MR-Egger regression intercept analyses. It is noteworthy that no evidence of horizontal pleiotropy was noted in this study. The adoption of a 2SMR approach, coupled with the utilization of nonoverlapping exposure and outcome summary-level data, was a strategic measure employed to avoid bias and enhance the overall robustness of the findings of the study (48).
Nevertheless, it is crucial to acknowledge various limitations in this research when interpreting the outcomes. Firstly, the study participants are exclusively of European ancestry, limiting the generalizability of the findings to the broader population. Secondly, potential confounding factors, including age, gender, and environmental influences, may introduce variability in the MR analysis. Furthermore, this research exclusively determines the causal associations between T2DM as the exposure and male infertility as the outcome. Future research will explore the reverse causal associations, examining male infertility as the exposure and T2DM as the outcome.
To conclude, this research offers novel insights into the genetic basis of the causal relationship between T2DM and male infertility, offering valuable guidance for future research endeavors.
Data availability statement
The original contributions presented in the study are included in the article/supplementary material. Further inquiries can be directed to the corresponding author.
Ethics statement
Ethical approval was not required for the study involving humans in accordance with the local legislation and institutional requirements. Written informed consent to participate in this study was not required from the participants or the participants’ legal guardians/next of kin in accordance with the national legislation and the institutional requirements.
Author contributions
CF: Conceptualization, Data curation, Formal Analysis, Funding acquisition, Investigation, Methodology, Project administration, Writing – original draft. JZ: Data curation, Formal Analysis, Investigation, Methodology, Project administration, Software, Supervision, Validation, Visualization, Writing – review & editing. DQ: Investigation, Supervision, Validation, Writing – review & editing.
Funding
The author(s) declare that financial support was received for the research, authorship, and/or publication of this article. This study was supported by the Scientific Research Project from the Education Department of Fujian Province (No. JAT220099).
Acknowledgments
The authors would like to acknowledge the contributors to the GWAS summary data used in this study. We thank Bullet Edits Limited for the linguistic editing and proofreading of the manuscript.
Conflict of interest
The authors declare that the research was conducted in the absence of any commercial or financial relationships that could be construed as a potential conflict of interest.
The reviewer ZC declared a shared affiliation with the author(s) to the handling editor at the time of review.
Publisher’s note
All claims expressed in this article are solely those of the authors and do not necessarily represent those of their affiliated organizations, or those of the publisher, the editors and the reviewers. Any product that may be evaluated in this article, or claim that may be made by its manufacturer, is not guaranteed or endorsed by the publisher.
References
1. World Health Organization. Diabetes (2022). Available online at: https://www.who.int/health-topics/diabetes#tab=tab_1 (Accessed August 2022).
2. American Diabetes Association Professional Practice Committee. Classification and diagnosis of diabetes: standards of medical care in diabetes-2022. Diabetes Care. (2022) 45:S17–38. doi: 10.2337/dc22-S002
3. Bommer C, Kuo S, Boyko EJ, Magliano DJ. IDF Diabetes Atlas: Global, regional and country-level diabetes prevalence estimates for 2021 and projections for 2045. Diabetes Res Clin Pract. (2022) 183:109119–32. doi: 10.1016/j.diabres.2021.109119
4. Xu G, Liu B, Sun Y, Du Y, Snetselaar LG, Hu FB, et al. Prevalence of diagnosed type 1 and type 2 diabetes among US adults in 2016 and 2017: population based study. BMJ. (2018) 362:k1497. doi: 10.1136/bmj.k1497
5. Zegers-Hochschild F, Adamson GD, Dyer S, Racowsky C, de Mouzon J, Sokol R, et al. The international glossary on infertility and fertility care, 2017. Fertil steril. (2017) 108:393–406. doi: 10.1016/j.fertnstert.2017.06.005
6. Lotti F, Maggi M. Sexual dysfunction and male infertility. Nat Rev Urol. (2018) 15:287–307. doi: 10.1038/nrurol.2018.20
7. Vollset SE, Goren E, Yuan CW, Cao J, Smith AE, Hsiao T, et al. Fertility, mortality, migration, and population scenarios for 195 countries and territories from 2017 to 2100: A forecasting analysis for the global burden of disease study. Lancet. (2020) 396:1285–306. doi: 10.1016/S0140-6736(20)30677-2
8. Lotti F, Frizza F, Balercia G, Barbonetti A, Behre HM, Calogero AE, et al. The European Academy of Andrology (EAA) ultrasound study on healthy, fertile men: clinical, seminal and biochemical characteristics. Andrology. (2020) 8:1005–20. doi: 10.1111/andr.12808
9. Lotti F, Maggi M. Effects of diabetes mellitus on sperm quality and fertility outcomes: Clinical evidence. Andrology. (2023) 11:399–416. doi: 10.1111/andr.13342
10. Condorelli RA, La Vignera S, Mongioi LM, Alamo A, Calogero AE. Diabetes mellitus and infertility: Different pathophysiological effects in type 1 and type 2 on sperm function. Front Endocrinol. (2018) 9:268. doi: 10.3389/fendo.2018.00268
11. Long L, Qiu H, Cai B, Chen N, Lu X, Zheng S, et al. Hyperglycemia induced testicular damage in type 2 diabetes mellitus rats exhibiting microcirculation impairments associated with vascular endothelial growth factor decreased Via Pi3k/Akt pathway. Oncotarget. (2018) 9:5321–36. doi: 10.18632/oncotarget.23915
12. He Z, Yin G, Li QQ, Zeng Q, Duan J. Diabetes mellitus causes Male reproductive dysfunction: A review of the evidence and mechanisms. In Vivo. (2021) 35:2503–11. doi: 10.21873/invivo.12531
13. Maresch CC, Stute DC, Alves MG, Oliveira PF, de Kretser DM, Linn T. Diabetes-induced hyperglycemia impairs Male reproductive function: A systematic review. Hum Reprod Update. (2018) 24:86–105. doi: 10.1093/humupd/dmx033
14. Chen X, Lin Q, Wen J, Lin W, Liang J, Huang H, et al. Whole genome bisulfite sequencing of human spermatozoa reveals differentially methylated patterns from type 2 diabetic patients. J Diabetes Invest. (2020) 11:856–64. doi: 10.1111/jdi.13201
15. Lascar N, Brown J, Pattison H, Barnett AH, Bailey CJ, Bellary S. Type 2 diabetes in adolescents and young adults. Lancet Diabetes Endocrinol. (2018) 6:69–80. doi: 10.1016/S2213-8587(17)30186-9
16. Dai M, Guo W, Zhu S, Gong G, Chen M, Zhong Z, et al. Type 2 diabetes mellitus and the risk of abnormal spermatozoa: A Mendelian randomization study. Front Endocrinol (Lausanne). (2022) 13:1035338. doi: 10.3389/fendo.2022.1035338
17. Facondo P, Di Lodovico E, Delbarba A, Anelli V, Pezzaioli LC, Filippini E, et al. The impact of diabetes mellitus type 1 on male fertility: Systematic review and meta-analysis. Andrology. (2022) 10:426–40. doi: 10.1111/andr.13140
18. Burgess S, Butterworth A, Thompson SG. Mendelian randomization ysis with multiple genetic variants using summarized data. Genet Epidemiol. (2013) 37:658–65. doi: 10.1002/gepi.21758
19. Davey Smith G, Hemani G. Mendelian randomization: genetic anchors for causal inference in epidemiological studies. Hum Mol Genet. (2014) 23:R89–98. doi: 10.1093/hmg/ddu328
20. Davies NM, Holmes MV, Davey Smith G. Reading Mendelian randomisation studies: a guide, glossary, and checklist for clinicians. BMJ. (2018) 362:k601. doi: 10.1136/bmj.k601
21. Palmer TM, Sterne JA, Harbord RM, Lawlor DA, Sheehan NA, Meng S, et al. Instrumental variable estimation of causal risk ratios and causal odds ratios in Mendelian randomization analyses. Am J Epidemiol. (2011) 173:1392–403. doi: 10.1093/aje/kwr026
22. Verduijn M, Siegerink B, Jager KJ, Zoccali C, Dekker FW. Mendelian randomization: use of genetics to enable causal inference in observational studies. Nephrol Dial Transplant. (2010) 25:1394–8. doi: 10.1093/ndt/gfq098
23. Zheng J, Baird D, Borges MC, Bowden J, Hemani G, Haycock P, et al. Recent developments in mendelian randomization studies. Curr Epidemiol Rep. (2017) 4:330–45. doi: 10.1007/s40471-017-0128-6
24. Yarmolinsky J, Bonilla C, Haycock PC, Langdon RJQ, Lotta LA, Langenberg C, et al. Circulating selenium and prostate cancer risk: A mendelian randomization analysis. J Natl Cancer Institute. (2018) 110:1035–8. doi: 10.1093/jnci/djy081
25. Burgess S, Small DS, Thompson SG. A review of instrumental variable estimators for mendelian randomization. Stat Methods Med Res. (2017) 26:2333–55. doi: 10.1177/0962280215597579
26. Burgess STS. Mendelian randomization: methods for using genetic variants in causal estimation. 1st Edition. New York: Boca Raton, FL: CRC Press (2015).
27. Burgess S, Butterworth A, Thompson SG. Mendelian randomization analysis with multiple genetic variants using summarized data. Genet Epidemiol. (2013) 37:658–65. doi: 10.1002/gepi.21758
28. De Silva K, Demmer RT, Jonsson D, Mousa A, Teede H, Forbes A, et al. Causality of anthropometric markers associated with polycystic ovarian syndrome:Findings of a mendelian randomization study. PloS One. (2022) 17:e0269191. doi: 10.1371/journal.pone.0269191
29. Burgess S, Thompson SG. Interpreting findings from Mendelian randomization using the MR-Egger method. Eur J Epidemiol. (2017) 32:377–89. doi: 10.1007/s10654-017-0255-x
30. Hwang LD, Lawlor DA, Freathy RM, Evans DM, Warrington NM. Using a two-sample mendelian randomization design to investigate a possible causal effect of maternal lipid concentrations on offspring birth weight. Int J Epidemiol. (2019) 48:1457–67. doi: 10.1093/ije/dyz160
31. Bowden J, Smith GD, Haycock PC, Burgess S. Consistent estimation in mendelian randomization with some invalid instruments using a weighted median estimator. Genet Epidemiol. (2016) 40:304–14. doi: 10.1002/gepi.21965
32. Verbanck M, Chen CY, Neale B, Do R. Detection of widespread horizontal pleiotropy in causal relationships inferred from Mendelian randomization between complex traits and diseases. Nat Genet. (2018) 50:693–8. doi: 10.1038/s41588-018-0099-7
33. Ding GL, Liu Y, Liu ME, Pan JX, Guo MX, Sheng JZ, et al. The effects of diabetes on Male fertility and epigenetic regulation during spermatogenesis. Asian J Androl. (2015) 17:948–53. doi: 10.4103/1008-682X.150844
34. Gandhi J, Dagur G, Warren K, Smith NL, Sheynkin YR, Zumbo A, et al. The role of diabetes mellitus in sexual and reproductive health: An overview of pathogenesis, evaluation, and management. Curr Diabetes Rev. (2017) 13:573–81. doi: 10.2174/1573399813666161122124017
35. La Vignera S, Condorelli R, Vicari E, D’Agata R, Calogero AE. Diabetes mellitus and sperm parameters. J Androl. (2012) 33:145–53. doi: 10.2164/jandrol.111.013193
36. Delfino M, Imbrogno N, Elia J, Capogreco F, Mazzilli F. Prevalence of diabetes mellitus in Male partners of infertile couples. Minerva urologica enefrologica = Ital J Urol Nephrol. (2007) 59:131–5. doi: 10.1038/s41598-018-32813-4
37. Imani M, Talebi AR, Fesahat F, Rahiminia T, Seifati SM, Dehghanpour F. Sperm parameters, DNA integrity, and protamine expression in patients with type ii diabetes mellitus. J obstetrics gynaecol J Institute Obstetrics Gynaecol. (2021) 41:439–46. doi: 10.1080/01443615.2020.1744114
38. Singh S, Malini T, Rengarajan S, Balasubramanian K. Impact of experimental diabetes and insulin replacement on epididymal secretory products and sperm maturation in albino rats. J Cell Biochem. (2009) 108:1094–101. doi: 10.1002/jcb.22337
39. Shi GJ, Zheng J, Wu J, Qiao HQ, Chang Q, Niu Y, et al. Beneficial effects of lycium barbarum polysaccharide on spermatogenesis by improving antioxidant activity and inhibiting apoptosis in streptozotocin-induced diabetic Male mice. Food Funct. (2017) 8:1215–26. doi: 10.1039/C6FO01575A
40. Campbell JM, Lane M, Owens JA, Bakos HW. Paternal obesity negatively affects Male fertility and assisted reproduction outcomes: A systematic review and meta-analysis. Reprod biomed Online. (2015) 31:593–604. doi: 10.1016/j.rbmo.2015.07.012
41. Matough FA, Budin SB, Hamid ZA, Alwahaibi N, Mohamed J. The role of oxidative stress and antioxidants in diabetic complications. Sultan Qaboos Univ Med J. (2012) 12:5–18. doi: 10.12816/0003082
42. Palmer NO, Bakos HW, Fullston T, Lane M. Impact of obesity on Male fertility, sperm function and molecular composition. Spermatogenesis. (2012) 2:253–63. doi: 10.4161/spmg.21362
43. Monteiro BS, Freire-Brito L, Carrageta DF, Oliveira PF, Alves MG. Mitochondrial uncoupling proteins (UCPs) as key modulators of ROS homeostasis: A crosstalk between diabesity and male infertility? Antioxidants (Basel). (2021) 10:1746. doi: 10.3390/antiox10111746
44. Derkach KV, Bakhtyukov AA, Romanova IV, Zorina II, Bayunova LV, Bondareva VM, et al. The effect of metformin treatment on the basal and gonadotropin-stimulated steroidogenesis in Male rats with type 2 diabetes mellitus. Andrologia. (2020) 52:e13816. doi: 10.1111/and.13816
45. de Llera AH, Martin-Hidalgo D, Gil MC, Garcia-Marin LJ, Bragado MJ. Amp-activated kinase ampk is expressed in boar spermatozoa and regulates motility. PloS One. (2012) 7:38840–50. doi: 10.1371/journal.pone.0038840
46. Faure M, Bertoldo MJ, Khoueiry R, Bongrani A, Brion F, Giulivi C, et al. Metformin in reproductive biology. Front Endocrinol. (2018) 9:675. doi: 10.3389/fendo.2018.00675
47. Emdin CA, Khera AV, Kathiresan S. Mendelian randomization. Jama. (2017) 318:1925–6. doi: 10.1001/jama.2017.17219
Keywords: T2DM, male infertility, causal relationship, Mendelian randomization, GWAS
Citation: Fan C, Zhang J and Qiu D (2024) Causal relationship between genetically predicted type 2 diabetes mellitus and male infertility. Front. Endocrinol. 15:1357279. doi: 10.3389/fendo.2024.1357279
Received: 17 December 2023; Accepted: 26 February 2024;
Published: 11 March 2024.
Edited by:
Eytan R. Barnea, BioIncept, LLC, United StatesReviewed by:
Gennaro Lettieri, University of Naples Federico II, ItalyZhaolei Cui, Fujian Medical University, China
Kuancan Liu, Xiamen University, China
Copyright © 2024 Fan, Zhang and Qiu. This is an open-access article distributed under the terms of the Creative Commons Attribution License (CC BY). The use, distribution or reproduction in other forums is permitted, provided the original author(s) and the copyright owner(s) are credited and that the original publication in this journal is cited, in accordance with accepted academic practice. No use, distribution or reproduction is permitted which does not comply with these terms.
*Correspondence: Jiandong Zhang, NTM1MTQ4NzBAcXEuY29t