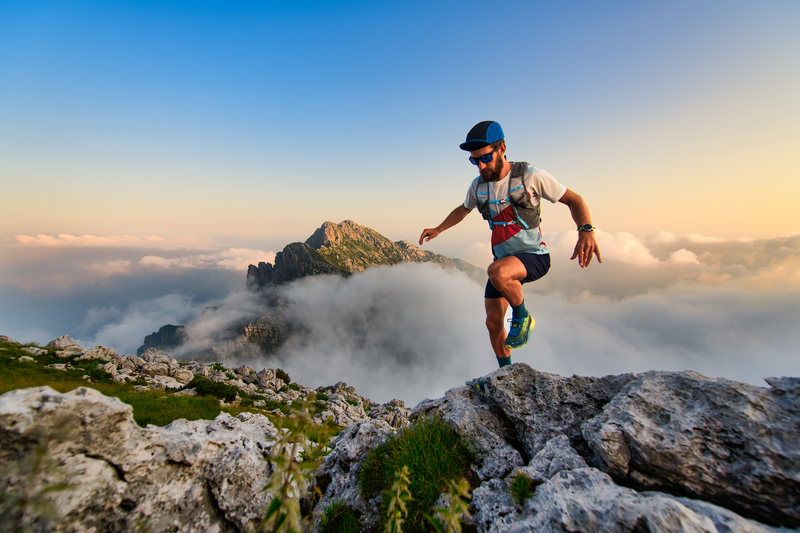
95% of researchers rate our articles as excellent or good
Learn more about the work of our research integrity team to safeguard the quality of each article we publish.
Find out more
ORIGINAL RESEARCH article
Front. Endocrinol. , 06 June 2024
Sec. Cancer Endocrinology
Volume 15 - 2024 | https://doi.org/10.3389/fendo.2024.1321622
Background: The triglyceride glucose (TyG) index has been associated with an increased risk in breast cancer. However, this association remains unclear among the Chinese population. This study aimed to investigate whether the TyG index is associated with the risk of prevalent breast cancer in Chinese women.
Methods: This cross-sectional study included 142,184 women from the REACTION (Risk Evaluation of Cancers in Chinese Diabetic Individuals: A Longitudinal) Study, which recruited adults aged 40 years or older from 25 centers across mainland China between 2011 and 2012. The TyG index was calculated according to the formula: Ln (fasting triglycerides [mg/dL] × fasting glucose [mg/dL]/2). Multivariable-adjusted logistic regression models were used to evaluate odds ratios (ORs) and 95% confidence intervals (CIs) regarding the associations between the TyG index and breast cancer.
Results: Multivariable-adjusted logistic regression analysis showed that compared with the lowest quartile of the TyG index, the highest quartile of the TyG index was significantly associated with an increased risk of prevalent breast cancer, with an OR (95% CI) of 1.61 (1.19–2.17). In the stratified analysis, the association of each 1 SD increase in the TyG index with risk of prevalent breast cancer was more dominant in individuals with menarche at age 13–17, those who were postmenopausal, those with a history of breastfeeding, and those who had two to four children, with the ORs (95% CIs) of 1.35 (1.09–1.68), 1.27 (1.05–1.54), 1.26 (1.05–1.52), and 1.32 (1.08–1.62), respectively. Moreover, among those without discernible insulin resistance (homeostatic model assessment-insulin resistance [HOMA-IR] ≥2.5), hyperglycemia and dyslipidemia, each 1 SD increase in the TyG index was associated with a 1.36-fold increase in breast cancer risk, with an OR (95% CI) of 2.36 (1.44–3.87).
Conclusion: The TyG index is significantly associated with the prevalent breast cancer risk among middle-aged and elderly Chinese women.
Breast cancer is the most popular cancer among women worldwide, with an estimated 2.3 million new cases (11.7%) of all the estimated 19.3 million new cancer cases in 2020, according to the latest 2020 global cancer statistics from the International Agency for Research on Cancer (IARC) (1). In China, female breast cancer claimed the unfortunate distinction of being the most common cancer, contributing to 16.7% of all newly diagnosed cancer cases (2). Identifying factors or related indices associated with the development of breast cancer is of great societal impact.
Insulin resistance (IR) plays a key role in the pathophysiology of breast cancer (3). IR, which leads to glucose intolerance, elevated homeostatic model assessment-insulin resistance (HOMA-IR), and compensatory hyperinsulinemia, is thought to be a central cause of obesity-related cancer onset and associated with unfavorable prognosis in postmenopausal women with breast cancer (4, 5). Previous studies have investigated the association between breast cancer risk and IR markers; however, these studies mostly relied on glucose, insulin, C-peptide, or HOMA-IR as related markers, and the association remains inconsistent (5–7). The triglyceride glucose (TyG) index is a reliable biochemical marker to assess IR, which has inexpensive and easy-to-use properties, compared with traditional hyperinsulinemic-euglycemic clamp (HIEC) (8). Currently, the population-based studies on the association of the TyG index with breast cancer risk are limited. A recent study has reported a non-linear dose–response relationship between TyG index and breast cancer among Indonesians (9). However, no significant association was observed in the European population (10). In addition, there is a lack of evidence in the Chinese population, and the relationship between TyG index and the risk of breast cancer is not yet clear.
Therefore, our study set out to explore the association between TyG index and breast cancer in a cross-sectional study of the general Chinese population. Additionally, we aimed to determine whether the TyG index maintains its association with breast cancer risk in a population without IR as defined by the HOMA-IR.
The REACTION study was a population-based multicenter study, in which a total of 259,657 Chinese adults (aged ≥40 years) from 25 communities across mainland China were recruited to participate in the baseline survey during 2011 to 2012. The detailed design and methods of the study cohort have been described previously (11–13). Briefly, eligible participants were identified from the local residence registration records and was approached by trained community workers using a door-to-door invitation in the baseline survey during 2011 to 2012. There were no gender or race restrictions during the recruitment period, which ended up with 169,628 women. The current analysis was a cross-sectional design, provided only baseline data from the REACTION study baseline survey, and included only women. After excluding those who had missing data for fasting glucose or fasting triglyceride (n = 3,168), had missing data for history of cancer (n = 3,768), use lipid-lowering drugs (n = 1,474) and glucose-lowering drugs (n = 12,015), or had chronic liver and kidney diseases (n = 7,019), a total of 142,184 women were included in the final analysis (Figure 1).
The study conformed to the Institutional Review Board of the Ruijin Hospital, Shanghai Jiao Tong University School of Medicine. All participants provided written informed consent.
The details of the data collection have been described previously (11). Trained investigators at each study center collected sociodemographic characteristics, lifestyle factors, and medical and family histories from a standardized questionnaire through personal interviews. Anthropometric measurements such as weight and height were performed by trained nurses according to a standard protocol. Women who had smoked one cigarette per day or seven per week regularly during the past 6 months were defined as current smokers, and those who had consumed alcohol once per week regularly during the past 6 months were defined as current drinkers. The type and frequency of smoking and alcohol consumption were recorded. Educational levels were divided into high school or above versus less than high school. The International Physical Activity Questionnaire was used to estimate intensity, duration, and frequency of physical activities by using the metabolic equivalent time per week. The validated dietary questionnaire was designed to collected participants’ dietary habits, frequency, and quantity information over the past 12 months, including red meat, fruits, vegetables, and dairy of typical food items. Women were asked about their reproductive history, including age at menarche, menopausal status, number of childbirths, and breastfeeding status.
Blood sampling was performed in the morning after an overnight fast of at least 10 h, and the samples were stored in dry ice at −80°C for transport to the central laboratory located at the Shanghai Institute of Endocrine and Metabolic Diseases that was certified by the College of American Pathologists. Glycated hemoglobin (HbA1c) was assayed by means of high-performance liquid chromatography method (Variant II and D-10 Systems; Bio-Rad, Hercules, CA). Fasting insulin (FINS) was measured with chemiluminescent immunoassay (i2000SR system, ARCHITECT ci16200 analyzer; Abbott Laboratories). Total cholesterol (TC), triglycerides (TG), high-density lipoprotein cholesterol (HDL-C), and low-density lipoprotein cholesterol (LDL-C) were measured on an autoanalyzer (c16000 system, ARCHITECT ci16200 analyzer; Abbott Laboratories, Lake Bluff, IL) in the central laboratory. In addition, all participants underwent an oral glucose tolerance test (OGTT). Plasma samples were obtained at 0 h and 2 h during the test, and blood glucose levels were assessed by glucose oxidase or hexokinase assay. HOMA-IR was calculated using the mathematical formula as follows: homeostatic model assessment of insulin resistance (HOMA-IR) = fasting plasma glucose (FPG) (mmol/L) × FINS (µU/mL)/22.5 (14).
The TyG index was calculated according to the formula that was previously published: TyG index = Ln [fasting TG (mg/dL) × FPG (mg/dL)/2] (10). Body mass index (BMI) was calculated as body weight in kilograms divided by body height squared in meters (kg/m2). Diagnosis of breast cancer was self-reported and further validated by reviewing medical records or pathology reports.
Data on the basic characteristics are presented as means ± standard deviations (SD) for normally distributed continuous variables or median (interquartile range) for continuous variables with a skewed distribution, and frequency (proportion) for categorical variables. Comparison of continuous variables was analyzed using one-way analysis of variance (ANOVA) and χ2 tests for categorical variables. Correlations between metabolic factors and TyG index were assessed using Spearman’s correlation analysis. Due to the cross-sectional nature of this study, there was a lack of follow-up time for breast cancer and Cox analysis could not be performed. Therefore, multivariable adjusted logistic regression analysis was used to evaluate the odds ratio (OR) and 95% confidence interval (CI) of TyG index and breast cancer. The multivariable model was adjusted for age, BMI, smoking status (current smoker or not), drinking status (current drinker or not), physical activity (moderate to vigorous or none to mild), family history of breast cancer, healthy diet (yes or no), 2-h plasma glucose (2h-PG), HbA1c and HDL-C in model 1 and further adjusted for age at menarche, menopausal status (yes or no), breastfeeding (yes or no), and number of childbirths in model 2. The TyG index entered the model either as a continuous variable with each 1 SD increase or as a categorical variable that was divided into quartiles, with the lowest quartile (Q1) as the reference group. Univariate logistic regression models were built to identify the associations between TyG index vs. TG, HbA1c, and FPG with breast cancer. P for trend across quartiles was calculated using ordinal values in separate models. Sensitivity analysis was conducted using Poisson regression with robust standard errors to compute prevalence ratios (PRs) and 95% CIs for associations between TyG index and breast cancer, adjusting for confounders.
Subgroup analysis was further performed to evaluate the potential effect of metabolic and reproductive factors on the association of TyG index each 1 SD increase and breast cancer. A likelihood ratio test was used to calculate the P value for interaction by comparing models with and without the interaction terms. In addition, several sensitivity analyses were conducted to test the role of TyG index on breast cancer in different metabolic states. We repeated the analyses excluding individuals with IR (HOMA-IR ≥2.5), hyperglycemia (FPG ≥6.1 mmol/L or 2h-PG ≥7.8 mmol/L or diagnosed with type 1 or type 2 diabetes or pre-diabetes), and dyslipidemia (TC ≥6.2 mmol/L or LDL-C ≥4.1 mmol/L or HDL-C <1.0 mmol/L or TG ≥2.3 mmol/L), respectively. In addition, to further explore whether this association is still significant in women with relatively healthy metabolic status, individuals with discernible IR, hyperglycemia, and dyslipidemia at the same time were also excluded. All statistical analyses were performed using SAS (version 9.4, SAS Institute Inc, Cary, NC). A two-tailed P < 0.05 was considered statistically significant.
We also used R (v 4.2.1) to construct restricted cubic spline (RCS) analysis using four knots to assess the non-linear dose–response relationship between TyG index and breast cancer after full adjustment.
Among the 142,184 women included, the mean (SD) age of the study population was 56.37 ± 9.30 years old and 809 (0.57%) individuals had breast cancer. Table 1 presents the baseline characteristics of the study population according to the quartile of the TyG index. On average, those with a lower TyG index were much younger and more educated, more likely to have ideal physical activity and healthy dietary habits, and tended to have lower levels of BMI, plasma glucose, TG, TC, and FINS (all P for trend < 0.0005). The characteristics of the participants with and without breast cancer are shown in Supplementary Table 1.
Table 1 Baseline characteristics of the study population according to the quartile of the TyG index.
Spearman correlation analysis was conducted to estimate the potential metabolic factors that influence the TyG index. It showed that the TyG index was positively correlated with 2h-PG, HbA1c, LDL-C and TC, FINS, and HOMA-IR (r ≤ 0.5) but negatively correlated with HDL-C (r = −0.3, Table 2).
The prevalence of breast cancer for women across quartile of the TyG index was 0.39%, 0.61%, 0.56%, and 0.72%, respectively. The association of the TyG index by quartile and the risk of breast cancer is given in Table 3. In unadjusted and age-adjusted models, both higher TyG index was associated with increased risk of breast cancer. After adjusting for potential covariates (multivariable-adjusted model 1), the ORs (95% CIs) of breast cancer in higher quartiles versus the lowest quartiles were 1.53 (1.20–1.95), 1.43 (1.11–1.83), and 1.73 (1.34–2.23), respectively. These associations persisted after further adjusting for reproductive factors; the ORs (95% CIs) of breast cancer in higher quartiles versus the lowest quartiles were 1.51 (1.14–2.00), 1.44 (1.08–1.93), and 1.61 (1.19–2.17), respectively. The results even remained significant after further adjusting for diabetes duration (Supplementary Table 2). The trends across quartiles were significant for the TyG index (P for trend < 0.0001). Each 1 SD increment of the TyG index was associated with a 29% increase in the risk of breast cancer in the fully adjusted model. The RCS analysis results showed that there was a non-linear relationship between the TyG index and breast cancer (P for non-linear < 0.05, Figure 2). In addition, when comparing the ORs of the TyG index, TG, HbA1c, and FPG, the ORs of higher levels of the TyG index stood out the most, indicating that the TyG index may have a superior discriminative ability for breast cancer (Supplementary Table 3). The PR (95% CI) of breast cancer associated with quartiles of the TyG index was similar (Supplementary Table 4). Per SD increasement of the TyG index was associated with a 16% increased prevalence of breast cancer (adjusted PR, 1.16; 95% CI, 1.05–1.28; Supplementary Table 4).
Figure 2 Non-linear dose–response relationship between the TyG index and breast cancer. The solid lines represent a fitted relationship, and the shadows represent the 95% confidence interval. TyG index, triglyceride glucose index.
In the stratification analysis (Figure 3), the association was stronger in women aged 50–59 years, with a BMI of lower than 24 kg/m2, age at menarche of 13–17 years old, those who were without moderate to vigorous physical activity and healthy diet, and those who were postmenopausal, breastfeeding, and have given birth to two to four children. There was no evidence of statistical interaction between risk factors and the TyG index (all P for interaction > 0.05). In addition, it seems that the association was significant among those without family history of breast cancer, rather than those with family history of breast cancer. However, no interaction between family history of breast cancer and TyG index was observed (P for interaction = 0.52, Supplementary Table 5).
Figure 3 Association of TyG index per 1 SD increase with breast cancer risk stratified by potential risk factors. TyG index, triglyceride glucose index; OR, odds ratio; 95% CI, 95% confidence interval; BMI, body mass index.
In sensitivity analysis, the results were remained significantly when participants with IR, hyperglycemia, or dyslipidemia were excluded, when participants had both IR and hyperglycemia were excluded, and when participants had both IR and dyslipidemia were excluded. In individuals without IR, hyperglycemia, and dyslipidemia, each 1 SD increase in the TyG index is associated with a 1.36-fold increase in breast cancer risk (OR = 2.36; 95% CI 1.44–3.87), after adjustment for confounders (Table 4).
Table 4 Sensitivity analyses of association between every per-unit TyG index value increase and risk of prevalent breast cancer.
In this study, we found that the TyG index was significantly associated with prevalent risk of breast cancer, even in those without discernible IR, hyperglycemia, and dyslipidemia. Our findings provide the first evidence of the association between the TyG index and breast cancer in a large Chinese middle-aged and elderly population.
Preclinical and clinical studies suggested complex associations between diabetes, especially type 2 diabetes with breast cancer (15). Our results showed that patients with breast cancer have significantly higher insulin levels than those without breast cancer, which is consistent with the previous evidence (7). As part of the important causes of diabetes, hyperinsulinemia and IR have been shown to be risk factors for breast cancer (4–7, 16). A study reported that compared with the lowest quartile, the highest quartile of insulin and HOMA-IR was associated with the greatest risk of breast cancer among 5,064 Chinese women (7). In addition, two studies from the Women’s Health Initiative (WHI) suggested that elevated levels of insulin and HOMA-IR may be a risk factor for postmenopausal breast cancer (5, 6), whereas glucose levels showed no association with this risk (6). A post genome-wide gene–environment interaction study among 11,109 postmenopausal women from the WHI identified single-nucleotide polymorphisms of HOMA-IR in combination with lifestyle as synergistic factors for breast cancer risk (4). However, a cohort study among 7,894 women from the Atherosclerosis Risk in Communities (ARIC) showed that there is no association between fasting insulin level and breast cancer incidence (17). This indicates that single indicators such as insulin or glucose may not be a good indicator for breast cancer in different populations, whereas the association between HOMA-IR and breast cancer suggests that it may be more beneficial to use multifactor indicators.
TyG index, a composite indicator based on TG and FPG, was reported to be an excellent surrogate indicator for IR that is economical and practical. Recently, a multicenter case–control study among 432 women from Indonesia showed that TyG index >8.87 was associated with risk of breast cancer (9). A cross-sectional Chinese study also found that the increasing TyG index was positively correlated with the heightening risk of breast cancer, but they used patients with breast disease rather than the general population (18). Furthermore, a hospital-based study among 510 Turkey patients with benign breast lesions or breast cancer indicated the predictive effect of the TyG index (cutoff was 8.628) in distinguishing benign and malignant lesions of the breast (19). However, in a large 17-year prospective study of 510,471 individuals from six European cohorts, the highest quintile of TyG index (>9.1) was not associated with the risk of postmenopausal breast cancer, compared with the lowest quintile (10). In our analysis, the prevalence of breast cancer in the highest quartile (>9.02) was nearly twice as high as in the lowest quartile (0.72% vs. 0.39%). Moreover, those in the highest quartile showed a significant association between TyG index and breast cancer, compared with the lowest quartile, and these risks remained mostly significant after adjustment for confounding factors. This result suggested that further examinations may be prioritized in women with a TyG index greater than 9.02. Our study firstly confirmed the association of the TyG index with breast cancer in a large Chinese general population more than 140,000 women, which provides powerful new evidence.
Reproductive risk factors, such as menstruation, number of births, and breastfeeding, have been previously reported to be associated with breast cancer risk (20). However, these conclusions remain controversial. The number of births can modulate breast cancer risk, and with some reduction with breastfeeding (21). In contrast, other studies have pointed out that the relationships between reproductive risk factors and breast cancer etiology are complex. Age at diagnosis of breast cancer appears to modify the effect of number of births; the elevated risk is not observed in women under 25 years of age (22). Moreover, the risk of breastfeeding on breast cancer varies among different populations (23, 24). In our analysis, when we further adjusted for age at menarche, menopausal status, number of childbirths, and breastfeeding, the association of the TyG index with breast cancer was attenuated but still significant. This suggested that the association between TyG index and breast cancer could not affected by these factors. In addition, we did not observe interactions between these risk factors and the TyG index for breast cancer risk. This finding is partially in line with several studies that found a null association of reproductive risk factors with breast cancer (9, 24). However, we found that the TyG index each 1 SD increase is associated with higher risk of breast cancer in postmenopausal women, in contrast to previous European reports that there is no association between TyG index and breast cancer (10). It could be partially due to the racial and population differences, which suggested that the association may be influenced by ethnic background. Additionally, higher risks were also observed in those with menarche at age 13–17, with breastfeeding, and had two to four children. This suggests that the TyG index might account at least partly for the additional risk despite these reproductive factors. Thus, TG or glucose level lowering appears as an additional target in women at high reproductive risk.
A previous study has reported that low physical activity is also a risk factor for breast cancer (20). Our findings supported this view because we found that the association of the TyG index with breast cancer was more predominant in women with low physical activity compared with women with moderate or vigorous physical activity, and the proportion of low physical activity was highest in the highest quartile of the TyG index. Due to the lack of relevant information, some other risk factors were not addressed in our study, including pathological type, genetic mutations, and exposure to steroid hormones (20). There is a long debate on whether oral contraceptives increase the risk of breast cancer; however, hormonal therapy for climacteric symptoms has been shown to increase the risk of breast cancer (25). A population-based study from Turkey confirmed the predictive effect of the TyG index in distinguishing benign and malignant lesions of the breast, which suggested that the TyG index may have greater predictive value for malignant breast lesions (19). The predictive role of the TyG index in distinguishing breast cancer gene mutation subtypes needs to be explored in future studies.
Specially, given that the cross-sectional study design could not confirm the causal relationship between TyG index and breast cancer, we further examined this association in those who were without IR, which is defined as HOMA-IR < 2.5 (26). We found that this association remained significant in women with HOMA-IR < 2.5. It is known that there are many risk factors for breast cancer in addition to IR. As a part of the TyG index, TG was also associated with breast cancer (27). Therefore, in the non-IR population defined by HOMA-IR, the risk of breast cancer may also be high. Our results suggested that the TyG index could be a potential marker for breast cancer risk among Chinese women and even perform better than HOMA-IR. Specially, the TyG index each 1 SD increase was significantly associated with a 2.36-fold greater risk of breast cancer among women without discernible IR, hyperglycemia, or dyslipidemia, after adjustment for confounders. It is worth noting that in addition to our study, there is still lack of evidence for this association in populations with a relatively healthy metabolic status. The reason for this association becoming stronger may be due to the fact that individuals with IR, hyperglycemia, and dyslipidemia are those who typically have more metabolic risk factors (28), which would obscure the association of the TyG index in the risk of breast cancer. Moreover, tumor cells required more energy compared with normal cells; several oncoproteins in breast cancer could promote the glycolytic process to provide substrates to highly proliferative cancer cells (29), which may lower glucose levels in patients with more advanced breast cancer. Importantly, this means that the TyG index, as a composite indicator, has the potential to serve as a warning marker for early prevention of breast cancer in relatively healthy women.
Mechanisms linking diabetes, especially type 2 diabetes, and breast cancer have been reported in previous studies (30, 31). These associations include the biological effects of type 2 diabetes on breast cancer risk and progression. At present, there are three potential mechanisms of association between type 2 diabetes and breast cancer, including the insulin pathway, insulin-like growth factor (IGF) pathway, and sex-hormone regulation (15). The influence of insulin on breast cancer is mainly based on the effect of IR or hyperinsulinemia, which activates the extracellular-related-kinase cascade and the AKT pathway through activation of the insulin receptor or the IGF receptor in breast cancer (15). The high risk of cancer in insulin-resistant individuals may also be due to overproduction of reactive oxygen species (ROS) (32). The increased production of mitochondrial ROS may affect the metastasis and recurrence pathway of breast cancer (33). In addition, overexpression of insulin receptor induces malignant transformation of mammary epithelial cell lines. Diabetes could also cause high plasma-free estrogen concentrations by increasing the production of sex hormones, which in turn activate the estrogen receptor (15). As part of the TyG index, TG also influences breast cancer through the AKT pathway via the G protein-coupled receptor (34). In addition, recent evidence has highlighted the importance of chronic inflammation in breast cancer pathobiology, such as NLRP3 inflammasome and cytokine oncostatin M, which are involved in breast cancer signaling and reprogramming the tumor microenvironment (35, 36).
The main strength of our study is the large sample size of more than 140,000 women from the 25-region community-based population, which represents the distribution of different regions in China. Nevertheless, there are some limitations in our study. Firstly, given the cross-sectional study design, we could not evaluate the causal relationship and directly compare the TyG index with HOMA-IR or other IR indices. However, we discerned an association between the TyG index and breast cancer in women without insulin resistance, as defined by HOMA-IR, and observed robust results. Further prospective studies are needed to identify the causal relationship between TyG index and breast cancer in larger Chinese population. Secondly, we were not able to categorize the pathological types of breast cancer. Thirdly, as our study was conducted in middle-aged and elderly women, we cannot generalize the results to younger women.
In conclusion, this is the first study that confirmed the association between the TyG index and the prevalent risk of breast cancer within a cohort exceeding 140,000 Chinese women. Moreover, our findings reveal that an elevated TyG index remains correlated with breast cancer among women without discernible IR, hyperglycemia, and dyslipidemia.
The original contributions presented in the study are included in the article/Supplementary material. Further inquiries can be directed to the corresponding authors.
The studies involving humans were approved by Institutional Review Board of the Ruijin Hospital, Shanghai Jiao Tong University School of Medicine. The studies were conducted in accordance with the local legislation and institutional requirements. The participants provided their written informed consent to participate in this study.
JL: Writing – review & editing, Validation, Supervision, Funding acquisition. XW: Writing – original draft, Visualization, Formal analysis, Data curation, Conceptualization. SYW: Writing – original draft. LL: Writing – original draft. XJ: Writing – review & editing, Data curation. CH: Writing – review & editing, Data curation. HQ: Writing – review & editing, Data curation. HL: Writing – review & editing, Investigation, Data curation. RZ: Writing – review & editing, Investigation, Data curation. ML: Writing – review & editing, Investigation, Data curation. YX: Writing – review & editing, Investigation, Data curation. MX: Writing – review & editing, Investigation, Data curation. LLC: Writing – review & editing, Data curation. TZ: Writing – review & editing, Data curation. RH: Writing – review & editing, Data curation. ZY: Writing – review & editing, Data curation. LS: Writing – review & editing, Data curation. QS: Writing – review & editing, Data curation. XY: Writing – review & editing, Data curation. LY: Writing – review & editing, Data curation. TW: Writing – review & editing, Investigation, Data curation. ZZ: Writing – review & editing, Investigation, Data curation. JZ: Writing – review & editing, Investigation, Data curation. GQ: Writing – review & editing, Data curation. QW: Writing – review & editing, Data curation. GC: Writing – review & editing, Data curation. MD: Writing – review & editing, Investigation, Data curation. XT: Writing – review & editing, Data curation. ZG: Writing – review & editing, Data curation. FS: Writing – review & editing, Data curation. XG: Writing – review & editing, Data curation. ZL: Writing – review & editing, Data curation. YQ: Writing – review & editing, Data curation. LC: Writing – review & editing, Data curation. XH: Writing – review & editing, Data curation. YH: Writing – review & editing, Data curation. QL: Writing – review & editing, Data curation. GW: Writing – review & editing, Data curation. YZ: Writing – review & editing, Data curation. CL: Writing – review & editing, Data curation. YW: Writing – review & editing, Data curation. SLW: Writing – review & editing, Data curation. TY: Writing – review & editing, Data curation. HD: Writing – review & editing, Data curation. JJZ: Writing – review & editing, Data curation. YM: Writing – review & editing, Data curation. GN: Writing – review & editing, Validation, Project administration, Funding acquisition. WW: Writing – review & editing, Supervision, Project administration, Funding acquisition. YB: Writing – review & editing, Supervision, Funding acquisition. YC: Writing – review & editing, Supervision, Funding acquisition.
The author(s) declare financial support was received for the research, authorship, and/or publication of this article. This study was supported by the Ministry of Science and Technology of the People’s Republic of China (Grant No. 2022YFC2505202 and 2023YFC2506704), the National Natural Science Foundation of China (Grant No. 82370810, 91857205, 82088102, 82170819, 82372347 and 82200998), Science and Technology Committee of Shanghai (Grant No. 22Y31900300, 23JS1400900 and 23Y11908400), Clinical Research Project of Shanghai Municipal Health Commission (Grant No. 20224Y0087 and 20214Y0002), Shanghai Committee of Science and Technology, and Innovative Research Team of High-Level Local Universities in Shanghai.
The authors thank all the participants of the REACTION study.
The authors declare that the research was conducted in the absence of any commercial or financial relationships that could be construed as a potential conflict of interest.
The reviewers YM and PB declared a shared parent affiliation with the authors JL, XW, SW, LL, XJ, CH, HQ, HL, RZ, ML, YX, MX, TW, ZZ, JZ, MD, GN, WW, YB, YC, YZ, and QS and the reviewer JY declared a shared parent affiliation with the author LY to the handling editor at the time of review.
All claims expressed in this article are solely those of the authors and do not necessarily represent those of their affiliated organizations, or those of the publisher, the editors and the reviewers. Any product that may be evaluated in this article, or claim that may be made by its manufacturer, is not guaranteed or endorsed by the publisher.
The Supplementary Material for this article can be found online at: https://www.frontiersin.org/articles/10.3389/fendo.2024.1321622/full#supplementary-material
1. Sung H, Ferlay J, Siegel RL, Laversanne M, Soerjomataram I, Jemal A, et al. Global cancer statistics 2020: GLOBOCAN estimates of incidence and mortality worldwide for 36 cancers in 185 countries. CA Cancer J Clin. (2021) 71:209–49. doi: 10.3322/caac.21660
2. Zheng R, Zhang S, Zeng H, Wang S, Sun K, Chen R, et al. Cancer incidence and mortality in China, 2016. J Natl Cancer Center. (2022) 2:1–9. doi: 10.1016/j.jncc.2022.02.002
3. Zhang F, Liu S. Mechanistic insights of adipocyte metabolism in regulating breast cancer progression. Pharmacol Res. (2020) 155:104741. doi: 10.1016/j.phrs.2020.104741
4. Jung SY, Papp JC, Sobel EM, Yu H, Zhang ZF. Breast cancer risk and insulin resistance: post genome-wide gene-environment interaction study using a random survival forest. Cancer Res. (2019) 79:2784–94. doi: 10.1158/0008-5472.Can-18-3688
5. Pan K, Chlebowski RT, Mortimer JE, Gunter MJ, Rohan T, Vitolins MZ, et al. Insulin resistance and breast cancer incidence and mortality in postmenopausal women in the Women's Health Initiative. Cancer. (2020) 126:3638–47. doi: 10.1002/cncr.33002
6. Kabat GC, Kim M, Caan BJ, Chlebowski RT, Gunter MJ, Ho GY, et al. Repeated measures of serum glucose and insulin in relation to postmenopausal breast cancer. Int J Cancer. (2009) 125:2704–10. doi: 10.1002/ijc.24609
7. Zhu Y, Wang T, Wu J, Huang O, Zhu L, He J, et al. Biomarkers of insulin and the insulin-like growth factor axis in relation to breast cancer risk in chinese women. Onco Targets Ther. (2020) 13:8027–36. doi: 10.2147/ott.S258357
8. Sánchez-García A, Rodríguez-Gutiérrez R, Mancillas-Adame L, González-Nava V, Díaz González-Colmenero A, Solis RC, et al. Diagnostic accuracy of the triglyceride and glucose index for insulin resistance: A systematic review. Int J Endocrinol. (2020) 2020:4678526. doi: 10.1155/2020/4678526
9. Panigoro SS, Sutandyo N, Witjaksono F, Siregar NC, Ramli R, Hariani R, et al. The association between triglyceride-glucose index as a marker of insulin resistance and the risk of breast cancer. Front Endocrinol (Lausanne). (2021) 12:745236. doi: 10.3389/fendo.2021.745236
10. Fritz J, Bjørge T, Nagel G, Manjer J, Engeland A, Häggström C, et al. The triglyceride-glucose index as a measure of insulin resistance and risk of obesity-related cancers. Int J Epidemiol. (2020) 49:193–204. doi: 10.1093/ije/dyz053
11. Du R, Zheng R, Xu Y, Zhu Y, Yu X, Li M, et al. Early-life famine exposure and risk of cardiovascular diseases in later life: findings from the REACTION study. J Am Heart Assoc. (2020) 9:e014175. doi: 10.1161/jaha.119.014175
12. Zhong F, Guan Q, Zhang H, Zhang X, Zhao M, Yuan Z, et al. Association of longitudinal changes in serum lipids with the natural history of subclinical hypothyroidism: A retrospective cohort study using data from the REACTION study. EClinicalMedicine. (2022) 53:101629. doi: 10.1016/j.eclinm.2022.101629
13. Hu C, Zhang Y, Zhang J, Huo Y, Wan Q, Li M, et al. Age at menarche, ideal cardiovascular health metrics, and risk of diabetes in adulthood: Findings from the REACTION study. J Diabetes. (2021) 13:458–68. doi: 10.1111/1753-0407.13128
14. Matthews DR, Hosker JP, Rudenski AS, Naylor BA, Treacher DF, Turner RC. Homeostasis model assessment: insulin resistance and beta-cell function from fasting plasma glucose and insulin concentrations in man. Diabetologia. (1985) 28:412–9. doi: 10.1007/bf00280883
15. Wolf I, Sadetzki S, Catane R, Karasik A, Kaufman B. Diabetes mellitus and breast cancer. Lancet Oncol. (2005) 6:103–11. doi: 10.1016/s1470-2045(05)01736-5
16. Capasso I, Esposito E, Pentimalli F, Montella M, Crispo A, Maurea N, et al. Homeostasis model assessment to detect insulin resistance and identify patients at high risk of breast cancer development: National Cancer Institute of Naples experience. J Exp Clin Cancer Res. (2013) 32:14. doi: 10.1186/1756-9966-32-14
17. Mink PJ, Shahar E, Rosamond WD, Alberg AJ, Folsom AR. Serum insulin and glucose levels and breast cancer incidence: the atherosclerosis risk in communities study. Am J Epidemiol. (2002) 156:349–52. doi: 10.1093/aje/kwf050
18. Zhang J, Yin B, Xi Y, Bai Y. Triglyceride-glucose index is a risk factor for breast cancer in China: a cross-sectional study. Lipids Health Dis. (2024) 23:29. doi: 10.1186/s12944-024-02008-0
19. Alkurt EG, Özkan MB, Turhan VB. Predictive value of triglyceride/glucose index (TyG) in predicting breast cancer in patients with breast mass. Eur Rev Med Pharmacol Sci. (2022) 26:4671–6. doi: 10.26355/eurrev_202207_29191
20. Loibl S, Poortmans P, Morrow M, Denkert C, Curigliano G. Breast cancer. Lancet. (2021) 397:1750–69. doi: 10.1016/s0140-6736(20)32381-3
21. Ambrosone CB, Higgins MJ. Relationships between breast feeding and breast cancer subtypes: lessons learned from studies in humans and in mice. Cancer Res. (2020) 80:4871–7. doi: 10.1158/0008-5472.Can-20-0077
22. Nichols HB, Schoemaker MJ, Cai J, Xu J, Wright LB, Brook MN, et al. Breast cancer risk after recent childbirth: A pooled analysis of 15 prospective studies. Ann Intern Med. (2019) 170:22–30. doi: 10.7326/m18-1323
23. Fortner RT, Sisti J, Chai B, Collins LC, Rosner B, Hankinson SE, et al. Parity, breastfeeding, and breast cancer risk by hormone receptor status and molecular phenotype: results from the Nurses' Health Studies. Breast Cancer Res. (2019) 21:40. doi: 10.1186/s13058-019-1119-y
24. Tan MM, Ho WK, Yoon SY, Mariapun S, Hasan SN, Lee DS, et al. A case-control study of breast cancer risk factors in 7,663 women in Malaysia. PloS One. (2018) 13:e0203469. doi: 10.1371/journal.pone.0203469
25. Collaborative Group on Hormonal Factors in Breast Cancer. Type and timing of menopausal hormone therapy and breast cancer risk: individual participant meta-analysis of the worldwide epidemiological evidence. Lancet. (2019) 394:1159–68. doi: 10.1016/S0140-6736(19)31709-X
26. Tam CS, Xie W, Johnson WD, Cefalu WT, Redman LM, Ravussin E. Defining insulin resistance from hyperinsulinemic-euglycemic clamps. Diabetes Care. (2012) 35:1605–10. doi: 10.2337/dc11-2339
27. Katzke VA, Sookthai D, Johnson T, Kühn T, Kaaks R. Blood lipids and lipoproteins in relation to incidence and mortality risks for CVD and cancer in the prospective EPIC-Heidelberg cohort. BMC Med. (2017) 15:218. doi: 10.1186/s12916-017-0976-4
28. Ozkan B, Ndumele CE. Addressing cardiovascular risk in diabetes: it's more than the sugar. Circulation. (2023) 147:1887–90. doi: 10.1161/circulationaha.123.065090
29. Kansara S, Singh A, Badal AK, Rani R, Baligar P, Garg M, et al. The emerging regulatory roles of non-coding RNAs associated with glucose metabolism in breast cancer. Semin Cancer Biol. (2023) 95:1–12. doi: 10.1016/j.semcancer.2023.06.007
30. Biello F, Platini F, D'Avanzo F, Cattrini C, Mennitto A, Genestroni S, et al. Insulin/IGF axis in breast cancer: clinical evidence and translational insights. Biomolecules. (2021) 11(1):125. doi: 10.3390/biom11010125
31. Chou PC, Choi HH, Huang Y, Fuentes-Mattei E, Velazquez-Torres G, Zhang F, et al. Impact of diabetes on promoting the growth of breast cancer. Cancer Commun (Lond). (2021) 41:414–31. doi: 10.1002/cac2.12147
32. Arcidiacono B, Iiritano S, Nocera A, Possidente K, Nevolo MT, Ventura V, et al. Insulin resistance and cancer risk: an overview of the pathogenetic mechanisms. Exp Diabetes Res. (2012) 2012:789174. doi: 10.1155/2012/789174
33. Romani P, Nirchio N, Arboit M, Barbieri V, Tosi A, Michielin F, et al. Mitochondrial fission links ECM mechanotransduction to metabolic redox homeostasis and metastatic chemotherapy resistance. Nat Cell Biol. (2022) 24:168–80. doi: 10.1038/s41556-022-00843-w
34. Sekine Y, Koike H, Nakano T, Nakajima K, Suzuki K. Remnant lipoproteins stimulate proliferation and activate MAPK and Akt signaling pathways via G protein-coupled receptor in PC-3 prostate cancer cells. Clin Chim Acta. (2007) 383:78–84. doi: 10.1016/j.cca.2007.04.016
35. Faria SS, Costantini S, de Lima VCC, de Andrade VP, Rialland M, Cedric R, et al. NLRP3 inflammasome-mediated cytokine production and pyroptosis cell death in breast cancer. J BioMed Sci. (2021) 28:26. doi: 10.1186/s12929-021-00724-8
Keywords: insulin resistance, triglyceride, glucose, breast cancer, Chinese population
Citation: Wu X, Wang S, Lin L, Jia X, Hu C, Qi H, Lin H, Zheng R, Li M, Xu Y, Xu M, Chen L, Zeng T, Hu R, Ye Z, Shi L, Su Q, Yu X, Yan L, Wang T, Zhao Z, Zheng J, Qin G, Wan Q, Chen G, Dai M, Tang X, Gao Z, Shen F, Gu X, Luo Z, Qin Y, Chen L, Hou X, Huo Y, Li Q, Wang G, Zhang Y, Liu C, Wang Y, Wu S, Yang T, Deng H, Zhao J, Mu Y, Ning G, Wang W, Bi Y, Chen Y and Lu J (2024) Association between triglyceride glucose index and breast cancer in 142,184 Chinese adults: findings from the REACTION study. Front. Endocrinol. 15:1321622. doi: 10.3389/fendo.2024.1321622
Received: 14 October 2023; Accepted: 21 May 2024;
Published: 06 June 2024.
Edited by:
Javad Sharifi-Rad, University of Azuay, EcuadorReviewed by:
Jinhua Yan, Third Affiliated Hospital of Sun Yat-sen University, ChinaCopyright © 2024 Wu, Wang, Lin, Jia, Hu, Qi, Lin, Zheng, Li, Xu, Xu, Chen, Zeng, Hu, Ye, Shi, Su, Yu, Yan, Wang, Zhao, Zheng, Qin, Wan, Chen, Dai, Tang, Gao, Shen, Gu, Luo, Qin, Chen, Hou, Huo, Li, Wang, Zhang, Liu, Wang, Wu, Yang, Deng, Zhao, Mu, Ning, Wang, Bi, Chen and Lu. This is an open-access article distributed under the terms of the Creative Commons Attribution License (CC BY). The use, distribution or reproduction in other forums is permitted, provided the original author(s) and the copyright owner(s) are credited and that the original publication in this journal is cited, in accordance with accepted academic practice. No use, distribution or reproduction is permitted which does not comply with these terms.
*Correspondence: Yufang Bi, YnlmMTA3ODRAcmpoLmNvbS5jbg==; Yuhong Chen, Y2hlbnloNzBAMTI2LmNvbQ==; Jieli Lu, amllbGlsdUBob3RtYWlsLmNvbQ==
†These authors have contributed equally to this work
Disclaimer: All claims expressed in this article are solely those of the authors and do not necessarily represent those of their affiliated organizations, or those of the publisher, the editors and the reviewers. Any product that may be evaluated in this article or claim that may be made by its manufacturer is not guaranteed or endorsed by the publisher.
Research integrity at Frontiers
Learn more about the work of our research integrity team to safeguard the quality of each article we publish.