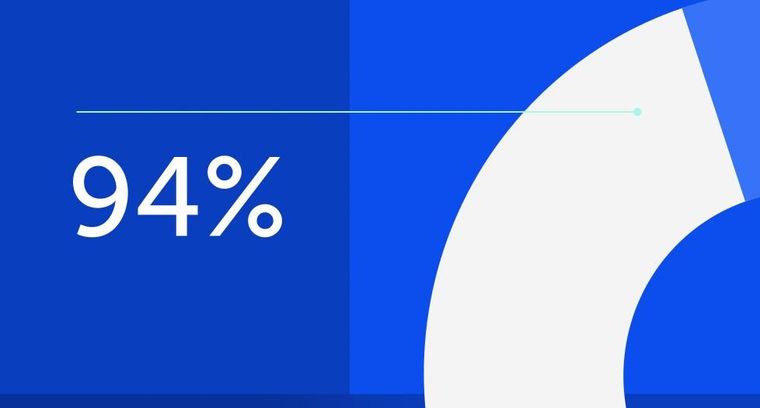
94% of researchers rate our articles as excellent or good
Learn more about the work of our research integrity team to safeguard the quality of each article we publish.
Find out more
ORIGINAL RESEARCH article
Front. Endocrinol., 02 February 2024
Sec. Diabetes: Molecular Mechanisms
Volume 15 - 2024 | https://doi.org/10.3389/fendo.2024.1313597
Background: Metformin is a drug with a long history of providing benefits in diabetes management and beyond. The mechanisms of action of metformin are complex, and continue to be actively debated and investigated. The aim of this study is to identify metabolic signatures associated with metformin treatment, which may explain the pleiotropic mechanisms by which metformin works, and could lead to an improved treatment and expanded use.
Methods: This is a cross-sectional study, in which clinical and metabolomic data for 146 patients with type 2 diabetes were retrieved from Qatar Biobank. Patients were categorized into: Metformin-treated, treatment naïve, and non-metformin treated. Orthogonal partial least square discriminate analysis and linear models were used to analyze differences in the level of metabolites between the metformin treated group with each of the other two groups.
Results: Patients on metformin therapy showed, among other metabolites, a significant increase in 3-hydroxyoctanoate and 3-hydroxydecanoate, which may have substantial effects on metabolism.
Conclusions: This is the first study to report an association between 3-hydroxy medium chain fatty acids with metformin therapy in patients with type 2 diabetes. This opens up new directions towards repurposing metformin by comprehensively understanding the role of these metabolites.
Metformin is undisputedly a milestone in the treatment of type 2 diabetes (T2D). The well-known advantages of metformin include its efficacy and safety profile, success in combination therapy, associated modest body weight reduction, and low cost (1). Although metformin has been utilized for several decades, the complete understanding of its mechanism of action and its effects on metabolism is still lacking. Indeed, the mechanism of action of metformin is complex, and continues to be actively debated and investigated (2). The main mechanisms of metformin are summarized in Table 1.
The repurposing of metformin has been expanded to include many pathophysiological conditions including cancer, cardiovascular diseases, and polycystic ovary syndrome. Moreover, recent studies demonstrated antioxidant and antiaging, renoprotective, and anti-inflammatory and immunomodulatory effects of metformin (2).
Metabolomics involves the comprehensive analysis of metabolites in biological systems, providing insights into complex biochemical pathways and the molecular mechanisms underlying drug effects (10). It has been utilized in various studies to investigate the impacts of drugs on cellular processes, identify potential biomarkers for disease and treatment response, and improve the chemical substance synthesis upstream of later development steps (11). This holistic approach has been particularly useful in understanding the underlying molecular pathways of drug effects, enabling researchers to develop more targeted and effective therapies (11, 12).
Greater insight into the underlying metabolic pathways influenced by metformin can provide a comprehensive understanding of its multifaceted mechanisms of action. This knowledge can pave the way for the development of specialized tools and strategies to enhance metformin therapy in the future. In this a cross-sectional study, our objective is to identify blood metabolites associated with metformin treatment in a cohort of 146 samples from type 2 diabetes patients. We analyzed the metabolic profiles of metformin-treated T2D patients in comparison to drug-naïve T2D patients, and then validated our findings by comparing the profiles of metformin-treated T2D patients with patients treated with other anti-diabetic drugs.
The study collected data from participants through the Qatar Biobank (QBB), which maintains a comprehensive dataset of Qatari nationals or long-term residents (those residing in Qatar for ≥15 years) aged 18 years and older. The QBB database encompasses various aspects of participant information, including questionnaires that capture details on diabetes history, medication usage, and laboratory results for a wide range of clinically significant metabolic traits. These traits include physical measurements such as blood pressure and body mass index (BMI), clinical chemistry parameters, endocrinology test results, as well as extensive metabolomics data comprising information on over 3000 metabolites. All clinical parameters were measured at the central laboratory of Hamad Medical Corporation in Qatar, accredited by the College of American Pathologists.
Out of the total participants, a cohort of 146 individuals diagnosed with type 2 diabetes, and possessing accessible metabolic data was carefully chosen. The diagnostic criteria for T2D, in accordance with the guidelines set forth by the American Diabetes Association (ADA), were meticulously followed during participant selection (13). Patients with incomplete or inconsistent medication records were excluded from the study. The research was approved by the Institutional Review Boards of the Qatar Biobank (QF-QBB-RES-ACC- 00125). Informed consent was obtained from all participants. Patients were categorized into three groups for this study. The first group consisted of patients who were undergoing treatment with metformin monotherapy, with daily doses ranging from 1000 to 2000 mg. The second group included patients who had not previously received any pharmacological treatment for their condition; these individuals were either newly diagnosed with T2D or had been managing their diabetes solely through lifestyle modifications. The third group comprised patients who were not prescribed metformin, but were instead receiving other anti-diabetic drugs, mainly gliclazide, sitagliptin, or a combination of both.
All participant serum samples were subjected to untargeted metabolomics using established methods. A detailed explanation of the protocols has already been provided (14). Internal standards and quality checks have been previously published (15). Quality control samples were employed to monitor the stability and consistency of the process over time. A systematic approach was adopted for managing pre-analytical aspects of the samples, such as collection, storage, and preparation, to minimize variability and ensure sample integrity.
The metabolomics data were inverse rank normalized. Multivariate analysis including unsupervised (principal component analysis) PCA and supervised (orthogonal partial least square-discriminant analysis) OPLS-DA were conducted using the software SIMCA® (version 18.0.0). Linear models for each metabolite (as the response variable) versus ‘Group 1’ vs ‘Group 2’, then ‘Group 1’ vs ‘Group 3’ (as the explanatory variables) were performed using R (version 4.2.1). The model also included the following confounders: Age, gender, BMI and principal components 1 and 2. The nominal p-values were adjusted using the multiple testing correction method (False Discovery Rate, FDR). Statistical significance was set as FDR < 0.05.
Table 2 provides an overview of the general characteristics of participants in this study, grouped by their treatment regimens for T2D. Notably, several parameters exhibited significant differences among the groups. Gender distribution differed significantly between the metformin-treated group (G1) and Drug-naïve (G2) group, while age was notably distinct in (G1) compared to (G2). Metformin-treated individuals (G1) showed significant differences in insulin levels and homeostatic model assessment of insulin resistance (HOMA-IR) when compared to the non-metformin-treated (G3) group. Additionally, variations were observed in total cholesterol (TC), LDL cholesterol, systolic blood pressure, pulse rate, albumin, alkaline phosphatase, total iron, Free triiodothyronine, ferritin, total dihydroxyvitamin D, and other parameters across different groups.
Non-targeted metabolomics analysis was conducted to explore the metabolic profiles of 146 T2D patients, classified into three distinct groups. The OPLS-DA method, depicted in Figure 1, was employed to identify key discriminating components between metformin-treated individuals (Group 1) and drug-naïve patients (Group 2), as well as between Group 1 and non-metformin-treated patients (Group 3). Scatter plots in Figures 1A, B clearly exhibit the distinct separation of group 1 from group 2, and group 1 from group 3, respectively. Furthermore, Figures 1C, D display the corresponding loading plots, revealing the primary metabolic pathways responsible for distinguishing group 1 from group 2, and group 1 from group 3, respectively. These include phosphatidylethanolamine, primary bile acids and sphingomyelins for group 1 vs group 2, and amino fatty acid, monohydroxy FA, primary bile acids and sphingomyelins for group 1 vs group 3.
Figure 1 Score and loading plots from OPLS-DA. (A, B) Score plots showing predictive component (x-axis) and orthogonal component (y-axis) between metformin-treated T2D vs drug-naïve T2D and metformin treated T2D vs non-metformin treated T2D respectively. (C, D) Corresponding loading plots depicting the enriched pathways for each OPLS-DA respectively. trt, treated; FA, Fatty acid.
The linear model analysis revealed several significant changes with false discovery rate (FDR) adjustments between the metformin-treated group and the drug-naïve group, as illustrated in Table 3. Notably, the metformin-treated group exhibited an increase in 3-hydroxydecanoate, 3-hydroxyoctanoate, and ethyl alpha-glucopyranoside. Conversely, a decrease was observed in citrulline, gamma-glutamylcitrulline, N6,N6,N6-trimethyllysine, as well as various sphingomyelins.
Table 3 Metabolites differentiating metformin-treated T2D vs drug-naïve T2D after correcting for age, gender, BMI and principal components 1 and 2.
The linear model analysis also revealed several significant changes with FDR adjustments between the metformin-treated group and the non-metformin-treated group, as presented in Table 4. Notably, the metformin-treated group exhibited an increase in 3-hydroxydecanoate, 3-hydroxyoctanoate, betonicine, and 2-aminooctanoate. Conversely, a decrease was observed in citrulline, gamma-glutamylcitrulline, as well as various secondary bile acid metabolites.
Table 4 Metabolites differentiating metformin treated T2D vs non-metformin treated T2D after correcting for age, gender, BMI and principal components 1 and 2.
To identify metabolite changes specifically associated with metformin treatment, we analyzed the common metabolites that significantly differentiated between group 1 vs. group 2 and group 1 vs. group 3 (Figure 2). These changes included higher levels of 3-hydroxydecanoate, and 3-hydroxyoctanoate (Figures 2A, B) in group 1 compared to groups 2 and 3, and lower levels of citrulline, gamma-glutamylcitrulline, and taurochenodeoxycholic acid 3-sulfate (Figures 2C–E) in group 1 compared to groups 2 and 3.
Figure 2 Raincloud plots showing the novel metabolites (A, B), and other metabolites (C–E) associated with metformin-treated T2D individuals compared to drug-naïve T2D and non-metformin treated T2D from the linear regression analysis.
The pathogenesis of T2D is characterized by a complex interplay of various metabolic abnormalities, including reduced peripheral glucose utilization, impaired beta-cell function, increased lipolysis, elevated free fatty acid levels, and gut dysbiosis (16). Obesity, ethnicity, physical activity, and socioeconomic status are risk factors that contribute to the development and progression of T2D (17). Metformin is currently the first-line medication to treat T2D in most guidelines. The mechanisms underlying the therapeutic action of metformin are complex and are still not fully understood (2). Pharmacometabolomics is a research field aimed at achieving a comprehensive and systemic understanding of drug mechanisms.
In this study, we employed an untargeted metabolomics approach to investigate the metabolic changes associated with metformin treatment in patients with T2D. Our emerging data revealed two novel metabolites, namely 3-hydroxyoctanoate and 3-hydroxydecanoate, which have not been previously reported in association with metformin treatment. While previous studies have reported lipid alterations linked to metformin treatment (18, 19), our findings are in line with earlier research (20, 21), demonstrating that citrulline and gamma-glutamylcitrulline are associated with metformin intake. The observed increase in 3-hydroxyoctanoate and 3-hydroxydecanoate levels in the metformin-treated group, when compared to the drug-naïve group, was further supported by comparisons with the non-metformin treated group. These metabolites will be the primary focus of our discussion.
3-hydroxyoctanoate and 3-hydroxydecanoate are primary metabolites that belong to the class of organic compounds known as 3-hydroxy medium-chain fatty acid (MCFA). These are hydroxy saturated fatty acids with 6 to 12 carbon atoms long side chain. MCFAs are natural compounds present in both animal and plant tissues that participate in cell metabolism. Like short-chain fatty acids, MCFAs play an important role in intracellular signaling and contribute to the regulation of cell metabolism. MCFAs have metabolic properties that are distinct from those of long-chain fatty acids, which make them readily oxidized by cells, provide a very efficient source of energy production, and impact postprandial energy expenditure positively (22). 3-hydroxy MCFAs are intermediates of mitochondrial β-oxidation (23), and of microbial origin (24).
Mitochondria play a pivotal role in the pharmacodynamics of metformin (25). Metformin promotes mitochondrial fatty acid oxidation (26, 27). It activates AMP-activated protein kinase (AMPK) which reduces the activity of Acetyl-CoA carboxylase 1 and 2 (ACC1/2), thus reducing malonyl-CoA levels, leading to an increase in fatty acid oxidation in the mitochondria (19). Metformin also leads to an increase in the expression of peroxisome proliferator-activated receptor gamma coactivator 1-alpha (PGC1-α) in various cell types, including hepatocytes and skeletal muscle cells (28). PGC1-α stimulates fatty acid targeting for mitochondrial β-oxidation thus diminishing the rate of fatty acid storage, the synthesis of lipotoxic derivatives, and triacylglycerol storage and secretion (29).
In line with our results, Walcott et al. reported a metabolic signature of increased fatty acid beta-oxidation and 3-hydroxy fatty acids among Li-Fraumeni syndrome (LFS) patients upon metformin treatment (6). However, our emerging data showed only the increase of 3-hydroxy medium chain fatty acids in the metformin-treated group when compared to each of the two other groups. No significant changes in other beta oxidation intermediates were shown. This suggests that the rate of beta oxidation was reduced at a certain step that favors the accumulation of these particular metabolites. This is consistent with many studies that reported the production of 3-hydroxy fatty as a result of incomplete beta-oxidation (30, 31).
In fact, the enzyme 3-hydroxyacyl-CoA dehydrogenase catalyzes the penultimate reaction of the mitochondrial β-oxidation cascade, the NAD+ dependent conversion of L-3-hydroxyacyl-CoA to 3-ketoacyl-CoA (32). No medium-chain 3-L-hydroxyacyl-coA dehydrogenase has been identified in humans; however, the human mitochondrial trifunctional protein (MTP) complex that possesses 3-hydroxyacyl-coA dehydrogenase activity is active with substrates of acyl-chain length from C6 to C16 (33). Interestingly, 3-hydroxyacyl-coA dehydrogenase donates electrons directly to complex I of the electron transport chain (ETC) (34); moreover, the MTP complex has been demonstrated to interact with the NADH-binding domain of complex I of the ETC (35), yet the exact nature of this relationship and its implications for cellular metabolism are not yet fully understood. Taking into consideration that metformin inhibits the complex I of ETC (36), the inhibition of this complex may lead to a decrease in the activity of 3-hydroxyacyl-coA dehydrogenase, and the increase in the levels of 3-hydroxy medium-chain fatty acids.
Another plausible explanation is through the mitochondrial enzyme deacetylase Sirtuin 3 (SIRT3). SIRT3 is essential for maintaining mitochondrial function, and it has been verified to regulate aging, neurodegeneration, liver and heart diseases, and other metabolic diseases (37). Sirt3 was shown to mediate, at least partly, the metformin-induced AMPK activation and cardioprotection (3). SIRT3 regulates fatty acid oxidation (38) via enzyme deacetylation. Interestingly, 3-hydroxyacyl-CoA dehydrogenase is a highly probable substrate for SIRT3 deacetylation (39). On the other hand, metformin reduces hepatic expression of SIRT3 and mitochondrial SIRT3 protein levels (40). This reduction of SIRT3, could ultimately lead to the inhibition of 3-hydroxyacyl-CoA dehydrogenase, and increase in the 3-hydroxy medium chain fatty acids.
Intriguingly, SIRT3 directly deacetylates and regulates the activity of ornithine transcarbamoylase (OTC), the enzyme that catalyzes the formation of citrulline from ornithine (39). The reduction of SIRT3 activity by metformin and subsequent inhibition of ornithine transcarbamoylase could explain the significant decrease in the levels of citrulline and its metabolite gamma-glutamylcitrulline in the metformin-treated group when compared to each of the two other groups. Nevertheless, it is undeniable that more future research studies are still needed to fully elucidate the interaction mechanism between mitochondria and metformin.
Microbial metabolism could be one of the most relevant source of 3-hydroxy medium chain fatty acids (41). Sjögren et al. reported the production of several 3-hydroxy fatty acids, including 3-hydroxydecanoic acid, from Lactobacillus plantarum (42), which belongs to the phylum Firmicutes, one of the two major phyla that dominate the gut microbiota (43). Moreover, Le Roy et al. showed that yogurt consumption was associated with reduced visceral fat mass, changes in gut microbiome, and importantly, an increase of 3-hydroxyoctanoic acid among other metabolites (44). However, according to Mikkelsen et al., 3-hydroxydecanoate is not produced by the gut microbiota, but it may still regulate its levels (45). The crosstalk between the metabolism of gut microbiota and metformin is now well ingrained (46). Increasing the production of short-chain fatty acids is among the mechanisms by which metformin exerts part of its hypoglycemic effects via the gut microbiota (7, 47). Though more validation studies are needed, we hypothesize that metformin-induced gut microbiota metabolites can also include 3-hydroxy MCFAs.
3-hydroxy MCFAs act as agonists of the hydroxycarboxylic acid receptor 3 (HCA3), also known as GPR109B (48). HCA3 is a G-protein-coupled receptor (also called metabolite-sensing G protein-coupled receptor), which regulates metabolism and immune functions (49). It is exclusively expressed in humans and higher primates (23), and this is presumably the reason why the receptor is still insufficiently studied because of lack of accessible animal models. In humans, HCA3 is expressed in adipocytes, immune cells, and intestinal epithelium; however, its expression has not been studied in-depth (50). Compared with other endogenous ligands which have low potency, 3-hydroxyoctanoic acid is highly specific to HCA3 (51), and activates it at micromolar concentrations (52). 3-hydroxy MCFAs act also as agonists to G-protein coupled receptor 84 (GPR84) which is primarily expressed in myeloid cells, and has remarkably increased expression in adipocytes when encountering acute inflammatory stimuli (53), and by hyperglycemia and hypercholesterolemia (54). 3-hydroxydecanoic acid is a common agonist of HCA3 and GPR84 (49). Strikingly, both HCA3 and GPR84 couple to G proteins resulting in the inhibition of adenylyl cyclase, and subsequent decrease of intracellular cyclic AMP (cAMP) levels (49), consistent with the ability of metformin to reduce cAMP levels resulting in decreased cAMP-dependent protein kinase (PKA) activity and reduced phosphorylation of critical PKA target proteins, and ultimate suppression of glucagon-dependent glucose output from hepatocytes (55).
Ahmed et al. demonstrated that 3-hydroxyoctanoic acid exerts an anti-lipolytic activity via HCA3 in situations of increased fatty acid oxidation and inhibits free fatty acid release (51). This is likely, at least in part, to explain the observed reduction in FFA after metformin treatment. Noteworthily, a partial inhibition of lipolysis in adipocytes may improve insulin sensitivity (56). The improvement of insulin sensitivity is considered a consequence of the changes induced by metformin in lipid metabolism (19). This corroborates our emerging data showing a significant reduction in HOMA-IR in metformin-treated group when compared to the two other groups, indicating an improvement in insulin sensitivity along with the increase in 3-hydroxy MCFAs.
Additionally, Stäubert et al. provided evidence that HCA3 is essential for fatty acid metabolism control in breast cancer cells (57). Moreover, HCA3 is an important regulator of inflammation in adipose tissue and may potentially be a target for the treatment of metabolic disorders (30). HCA3 activation inhibits the production of reactive oxygen species, and increases anti-inflammatory interleukin 10 secretion (58). Activation of HCA3 reduced the production of proinflammatory cytokines in adipocytes and macrophages (59). This is consistent with the anti-oxidative and anti-inflammatory effects of metformin. On the other hand, Recio et al. showed that GPR84 activation triggered increased phagocytosis in macrophages (54). Relatedly, studies reported metformin-induced shift in myeloid cells from classical to nonclassical monocytes, and increased phagocytosis activity (60). Promoting phagocytosis of macrophages is one of anti-tumor strategies (61), and metformin is a very promising drug to treat cancer (62). However, whether metformin promotes phagocytosis by indirect activation of GPR84 through 3-hydroxy MCFA is to be confirmed by in-depth future studies. Interestingly, Peters et al. (63) demonstrated that metabolites of lactic acid bacteria, permanently colonizing the human intestine, are highly potent agonists of HCA3. Additionally, Sakura et al. reported the production of HCA3 ligands in vitro by Bifidobacterium, which is one of the major components of the human gut microbiota (64).
Taken together, our results provide evidence that metformin increases the production of 3-hydroxy fatty acids via beta oxidation and/or gut microbiota. Subsequently, these metabolites have the potential to activate the metabolite-sensing G protein-coupled receptors, and thus mediating the pleiotropic beneficial effects of metformin. Although not statistically significant, the metformin-treated group showed better glycemic control and lipid profile compared to the two other groups, suggesting an underlying beneficial role of 3-hydroxy medium chain fatty acids. Yet, other studies hold a conclusion against our findings. Mikkelsen et al. (45) reported that 3-hydroxydecanoate is enriched in the circulation of obese individuals with T2D compared with healthy controls. Al-Sulaiti et al. (65) showed that 3-hydroxyoctanoate and 3-hydroxydecanoate were elevated in patients with T2D when compared to obese insulin resistant and obese insulin sensitive participants. However, these two studies didn’t take into consideration the treatment of the patients with diabetes. Very probably, a significant number of these patients could be on metformin therapy, and the elevation of 3-hydroxydecanoate and/or 3-hydroxydecanoate could be beneficial and attributed to metformin treatment rather than the diabetes condition.
To our knowledge, we report for the first time an association between 3-hydroxyoctanoate and 3-hydroxydecanoate with metformin treatment in patients with T2D. This could provide insights into the underlying mechanisms of metformin, and help to identify new therapeutic applications of this drug. 3-hydroxy medium chain fatty acids can originate from an increased but incomplete mitochondrial beta oxidation, or produced by the gut microbiota.3-hydroxyoctanoate and 3-hydroxydecanoate are activators of two important receptors, namely hydroxycarboxylic acid receptor 3 and G-protein coupled receptor 84.
While our results were replicated when comparing metformin-treated group with each of the two other groups, we acknowledge that the small sample size is a limitation in this study. Another limitation is the cross-sectional design of the study, which does not allow for the assessment of changes of these metabolites over time. Future research could involve larger cohorts of patients, including different ethnic groups and populations, to validate the findings and explore any potential differences in metabolic signatures.
Qatar biobanks owns the intellectual property rights of the data. Requests to access these datasets should be directed to dGFrZXBhcnRAcWF0YXJiaW9iYW5rLm9yZy5xYQ==.
The studies involving humans were approved by Institutional Review Boards of the Qatar Biobank (QF-QBB-RES-ACC-00125). The studies were conducted in accordance with the local legislation and institutional requirements. The participants provided their written informed consent to participate in this study.
KN: Writing – original draft. NA: Software, Writing – original draft. AM: Writing – review & editing. ME: Funding acquisition, Project administration, Supervision, Writing – review & editing.
The author(s) declare financial support was received for the research, authorship, and/or publication of this article. This research was funded by Qatar National Research Fund (QNRF), grant number NPRP13S-1230-190008.
Authors would like to acknowledge Qatar Biobank for providing the data.
The authors declare that the research was conducted in the absence of any commercial or financial relationships that could be construed as a potential conflict of interest.
All claims expressed in this article are solely those of the authors and do not necessarily represent those of their affiliated organizations, or those of the publisher, the editors and the reviewers. Any product that may be evaluated in this article, or claim that may be made by its manufacturer, is not guaranteed or endorsed by the publisher.
T2D, Type 2 diabetes; MCFA, Medium-chain fatty acid; HCA3, Hydroxycarboxylic acid receptor 3; GPR84, G-protein coupled receptor 84; AMPK, Adenosine monophosphate-activated protein kinase; GLP-1, Glucagon-like peptide-1; HbA1C, Glycated hemoglobin; HOMA-IR, Homeostatic model assessment of insulin resistance; HDL, High-density lipoprotein; LDL, Low-density lipoprotein; ALT, Alanine transaminase; AST, Aspartate aminotransferase; CRP, C-reactive protein; GGT, Gamma-glutamyl transferase; TSH, Thyroid stimulating hormone.
1. Giaccari A, Solini A, Frontoni S, Del Prato S. Metformin benefits: another example for alternative energy substrate mechanism? Diabetes Care (2021) 44(3):647–54. doi: 10.2337/dc20-1964
2. Foretz M, Guigas B, Viollet B. Metformin: update on mechanisms of action and repurposing potential. Nat Rev Endocrinol (2023), 1–17. doi: 10.1038/s41574-023-00833-4
3. Zhu H, Jia Z, Li YR, Danelisen I. Molecular mechanisms of action of metformin: latest advances and therapeutic implications. Clin Exp Med (2023), 1–11. doi: 10.1007/s10238-023-01051-y
4. Wu T, Xie C, Wu H, Jones KL, Horowitz M, Rayner CK. Metformin reduces the rate of small intestinal glucose absorption in type 2 diabetes. Diabetes Obes Metab (2017) 19(2):290–3. doi: 10.1111/dom.12812
5. Kaneto H, Kimura T, Obata A, Shimoda M, Kaku K. Multifaceted mechanisms of action of metformin which have been unraveled one after another in the long history. Int J Mol Sci (2021) 22(5):2596. doi: 10.3390/ijms22052596
6. Walcott FL, Annunziata CM, Sotomayor EM, Fojo AT. Effect of metformin chemoprevention on metabolomics profiles in Li-Fraumeni Syndrome (LFS). J Clin Oncol (2017) 35(15_suppl):1556–. doi: 10.1200/JCO.2017.35.15_suppl.1556
7. Zhang Q, Hu N. Effects of metformin on the gut microbiota in obesity and type 2 diabetes mellitus. Diabetes Metab Syndr Obes (2020) 13:5003–14. doi: 10.2147/DMSO.S286430
8. Mueller NT, Differding MK, Zhang M, Maruthur NM, Juraschek SP, Miller ER 3rd, et al. Metformin affects gut microbiome composition and function and circulating short-chain fatty acids: A randomized trial. Diabetes Care (2021) 44(7):1462–71. doi: 10.2337/dc20-2257
9. van Stee MF, de Graaf AA, Groen AK. Actions of metformin and statins on lipid and glucose metabolism and possible benefit of combination therapy. Cardiovasc Diabetology. (2018) 17(1):94.
10. Tounta V, Liu Y, Cheyne A, Larrouy-Maumus G. Metabolomics in infectious diseases and drug discovery. Mol Omics. (2021) 17(3):376–93. doi: 10.1039/D1MO00017A
11. Danzi F, Pacchiana R, Mafficini A, Scupoli MT, Scarpa A, Donadelli M, et al. To metabolomics and beyond: a technological portfolio to investigate cancer metabolism. Signal Transduct Target Ther (2023) 8(1):137. doi: 10.1038/s41392-023-01380-0
12. Gonzalez-Covarrubias V, Martinez-Martinez E, Del Bosque-Plata L. The potential of metabolomics in biomedical applications. Metabolites (2022) 12(2). doi: 10.3390/metabo12020194
13. ElSayed NA, Aleppo G, Aroda VR, Bannuru RR, Brown FM, Bruemmer D, et al. 2. Classification and diagnosis of diabetes: standards of care in diabetes-2023. Diabetes Care (2023) 46(Suppl 1):S19–s40.
14. Al-Khelaifi F, Diboun I, Donati F, Botrè F, Alsayrafi M, Georgakopoulos C, et al. A pilot study comparing the metabolic profiles of elite-level athletes from different sporting disciplines. Sports Med Open (2018) 4(1):2. doi: 10.1186/s40798-017-0114-z
15. Evans A, Bridgewater B, Liu Q, Mitchell M, Robinson R, Dai H, et al. High resolution mass spectrometry improves data quantity and quality as compared to unit mass resolution mass spectrometry in high-throughput profiling metabolomics. Metabolomics (2014) 4. doi: 10.4172/2153-0769.1000132
16. Galicia-Garcia U, Benito-Vicente A, Jebari S, Larrea-Sebal A, Siddiqi H, Uribe KB, et al. Pathophysiology of type 2 diabetes mellitus. Int J Mol Sci (2020) 21(17). doi: 10.3390/ijms21176275
17. Weisman A, Fazli GS, Johns A, Booth GL. Evolving trends in the epidemiology, risk factors, and prevention of type 2 diabetes: A review. Can J Cardiol (2018) 34(5):552–64. doi: 10.1016/j.cjca.2018.03.002
18. Dahabiyeh LA, Mujammami M, AlMalki RH, Arafat T, Benabdelkamel H, Alfadda AA, et al. Lipids alterations associated with metformin in healthy subjects: an investigation using mass spectrometry shotgun approach. Int J Mol Sci (2022) 23(19). doi: 10.3390/ijms231911478
19. He L. Metformin and systemic metabolism. Trends Pharmacol Sci (2020) 41(11):868–81. doi: 10.1016/j.tips.2020.09.001
20. Adam J, Brandmaier S, Leonhardt J, Scheerer MF, Mohney RP, Xu T, et al. Metformin effect on nontargeted metabolite profiles in patients with type 2 diabetes and in multiple murine tissues. Diabetes (2016) 65(12):3776–85. doi: 10.2337/db16-0512
21. Rotroff DM, Oki NO, Liang X, Yee SW, Stocker SL, Corum DG, et al. Pharmacometabolomic assessment of metformin in non-diabetic, African Americans. Front Pharmacol (2016) 7:135. doi: 10.3389/fphar.2016.00135
22. Yuan T, Wang L, Jin J, Mi L, Pang J, Liu Z, et al. Role medium-chain fatty acids in the lipid metabolism of infants. Front Nutr (2022) 9:804880. doi: 10.3389/fnut.2022.804880
23. Ahmed K. Biological roles and therapeutic potential of hydroxy-carboxylic acid receptors. Front Endocrinol (2011) 2. doi: 10.3389/fendo.2011.00051
24. Kutschera A, Dawid C, Gisch N, Schmid C, Raasch L, Gerster T, et al. Bacterial medium-chain 3-hydroxy fatty acid metabolites trigger immunity in Arabidopsis plants. Science (2019) 364(6436):178–81. doi: 10.1126/science.aau1279
25. Feng J, Wang X, Ye X, Ares I, Lopez-Torres B, Martínez M, et al. Mitochondria as an important target of metformin: The mechanism of action, toxic and side effects, and new therapeutic applications. Pharmacol Res (2022) 177:106114. doi: 10.1016/j.phrs.2022.106114
26. Wang C, Liu F, Yuan Y, Wu J, Wang H, Zhang L, et al. Metformin suppresses lipid accumulation in skeletal muscle by promoting fatty acid oxidation. Clin Lab (2014) 60(6):887–96. doi: 10.7754/Clin.Lab.2013.130531
27. Tokubuchi I, Tajiri Y, Iwata S, Hara K, Wada N, Hashinaga T, et al. Beneficial effects of metformin on energy metabolism and visceral fat volume through a possible mechanism of fatty acid oxidation in human subjects and rats. PloS One (2017) 12(2):e0171293. doi: 10.1371/journal.pone.0171293
28. Aatsinki SM, Buler M, Salomäki H, Koulu M, Pavek P, Hakkola J. Metformin induces PGC-1α expression and selectively affects hepatic PGC-1α functions. Br J Pharmacol (2014) 171(9):2351–63. doi: 10.1111/bph.12585
29. Cheng CF, Ku HC, Lin H. PGC-1α as a pivotal factor in lipid and metabolic regulation. Int J Mol Sci (2018) 19(11). doi: 10.3390/ijms19113447
30. Duncan EM, Vita L, Dibnah B, Hudson BD. Metabolite-sensing GPCRs controlling interactions between adipose tissue and inflammation. Front Endocrinol (Lausanne). (2023) 14:1197102. doi: 10.3389/fendo.2023.1197102
31. Madu UL, Ogundeji AO, Pohl CH, Albertyn J, Sebolai OM. Elucidation of the role of 3-hydroxy fatty acids in cryptococcus-amoeba interactions. Front Microbiol (2017) 8. doi: 10.3389/fmicb.2017.00765
32. Schulz N, Himmelbauer H, Rath M, van Weeghel M, Houten S, Kulik W, et al. Role of medium- and short-chain L-3-hydroxyacyl-CoA dehydrogenase in the regulation of body weight and thermogenesis. Endocrinology (2011) 152(12):4641–51. doi: 10.1210/en.2011-1547
33. Adeva-Andany MM, Carneiro-Freire N, Seco-Filgueira M, Fernández-Fernández C, Mouriño-Bayolo D. Mitochondrial β-oxidation of saturated fatty acids in humans. Mitochondrion (2019) 46:73–90. doi: 10.1016/j.mito.2018.02.009
34. Schönfeld P, Wojtczak L. Short- and medium-chain fatty acids in energy metabolism: the cellular perspective. J Lipid Res (2016) 57(6):943–54. doi: 10.1194/jlr.R067629
35. Wang Y, Palmfeldt J, Gregersen N, Makhov AM, Conway JF, Wang M, et al. Mitochondrial fatty acid oxidation and the electron transport chain comprise a multifunctional mitochondrial protein complex. J Biol Chem (2019) 294(33):12380–91. doi: 10.1074/jbc.RA119.008680
36. Fontaine E. Metformin-induced mitochondrial complex I inhibition: facts, uncertainties, and consequences. Front Endocrinol (Lausanne). (2018) 9:753. doi: 10.3389/fendo.2018.00753
37. Zhang J, Xiang H, Liu J, Chen Y, He RR, Liu B. Mitochondrial Sirtuin 3: New emerging biological function and therapeutic target. Theranostics (2020) 10(18):8315–42. doi: 10.7150/thno.45922
38. Hirschey MD, Shimazu T, Goetzman E, Jing E, Schwer B, Lombard DB, et al. SIRT3 regulates mitochondrial fatty-acid oxidation by reversible enzyme deacetylation. Nature (2010) 464(7285):121–5. doi: 10.1038/nature08778
39. Hallows WC, Yu W, Smith BC, Devries MK, Ellinger JJ, Someya S, et al. Sirt3 promotes the urea cycle and fatty acid oxidation during dietary restriction. Mol Cell (2011) 41(2):139–49. doi: 10.1016/j.molcel.2011.01.002
40. Buler M, Aatsinki SM, Izzi V, Hakkola J. Metformin reduces hepatic expression of SIRT3, the mitochondrial deacetylase controlling energy metabolism. PloS One (2012) 7(11):e49863. doi: 10.1371/journal.pone.0049863
41. Xiao K, Yue XH, Chen WC, Zhou XR, Wang L, Xu L, et al. Metabolic engineering for enhanced medium chain omega hydroxy fatty acid production in escherichia coli. Front Microbiol (2018) 9:139. doi: 10.3389/fmicb.2018.00139
42. Sjögren J, Magnusson J, Broberg A, Schnürer J, Kenne L. Antifungal 3-hydroxy fatty acids from Lactobacillus plantarum MiLAB 14. Appl Environ Microbiol (2003) 69(12):7554–7. doi: 10.1128/AEM.69.12.7554-7557.2003
43. Le B, Yang SH. Efficacy of Lactobacillus plantarum in prevention of inflammatory bowel disease. Toxicol Rep (2018) 5:314–7. doi: 10.1016/j.toxrep.2018.02.007
44. Le Roy CI, Kurilshikov A, Leeming ER, Visconti A, Bowyer RCE, Menni C, et al. Yoghurt consumption is associated with changes in the composition of the human gut microbiome and metabolome. BMC Microbiol (2022) 22(1):39.
45. Mikkelsen RB, Arora T, Trošt K, Dmytriyeva O, Jensen SK, Meijnikman AS, et al. Type 2 diabetes is associated with increased circulating levels of 3-hydroxydecanoate activating GPR84 and neutrophil migration. iScience (2022) 25(12):105683. doi: 10.1016/j.isci.2022.105683
46. Wu H, Esteve E, Tremaroli V, Khan MT, Caesar R, Mannerås-Holm L, et al. Metformin alters the gut microbiome of individuals with treatment-naive type 2 diabetes, contributing to the therapeutic effects of the drug. Nat Med (2017) 23(7):850–8. doi: 10.1038/nm.4345
47. Forslund K, Hildebrand F, Nielsen T, Falony G, Le Chatelier E, Sunagawa S, et al. Disentangling type 2 diabetes and metformin treatment signatures in the human gut microbiota. Nature (2015) 528(7581):262–6. doi: 10.1038/nature15766
48. Ahmed K, Tunaru S, Offermanns S. GPR109A, GPR109B and GPR81, a family of hydroxy-carboxylic acid receptors. Trends Pharmacol Sci (2009) 30(11):557–62. doi: 10.1016/j.tips.2009.09.001
49. Peters A, Rabe P, Krumbholz P, Kalwa H, Kraft R, Schöneberg T, et al. Natural biased signaling of hydroxycarboxylic acid receptor 3 and G protein-coupled receptor 84. Cell Commun Signal (2020) 18(1):31. doi: 10.1186/s12964-020-0516-2
50. Offermanns S. Hydroxy-carboxylic acid receptor actions in metabolism. Trends Endocrinol Metab (2017) 28(3):227–36. doi: 10.1016/j.tem.2016.11.007
51. Ahmed K, Tunaru S, Langhans CD, Hanson J, Michalski CW, Kölker S, et al. Deorphanization of GPR109B as a receptor for the beta-oxidation intermediate 3-OH-octanoic acid and its role in the regulation of lipolysis. J Biol Chem (2009) 284(33):21928–33. doi: 10.1074/jbc.M109.019455
52. Davenport AP, Alexander SP, Sharman JL, Pawson AJ, Benson HE, Monaghan AE, et al. International Union of Basic and Clinical Pharmacology. LXXXVIII. G protein-coupled receptor list: recommendations for new pairings with cognate ligands. Pharmacol Rev (2013) 65(3):967–86.
53. Zhang Q, L-h C, Yang H, Fang Y-C, Wang S-W, Wang M, et al. GPR84 signaling promotes intestinal mucosal inflammation via enhancing NLRP3 inflammasome activation in macrophages. Acta Pharmacologica Sinica. (2022) 43(8):2042–54. doi: 10.1038/s41401-021-00825-y
54. Recio C, Lucy D, Purvis GSD, Iveson P, Zeboudj L, Iqbal AJ, et al. Activation of the immune-metabolic receptor GPR84 enhances inflammation and phagocytosis in macrophages. Front Immunol (2018) 9:1419. doi: 10.3389/fimmu.2018.01419
55. Kim YD, Park KG, Lee YS, Park YY, Kim DK, Nedumaran B, et al. Metformin inhibits hepatic gluconeogenesis through AMP-activated protein kinase-dependent regulation of the orphan nuclear receptor SHP. Diabetes (2008) 57(2):306–14. doi: 10.2337/db07-0381
56. Girousse A, Tavernier G, Valle C, Moro C, Mejhert N, Dinel AL, et al. Partial inhibition of adipose tissue lipolysis improves glucose metabolism and insulin sensitivity without alteration of fat mass. PloS Biol (2013) 11(2):e1001485. doi: 10.1371/journal.pbio.1001485
57. Stäubert C, Broom OJ, Nordström A. Hydroxycarboxylic acid receptors are essential for breast cancer cells to control their lipid/fatty acid metabolism. Oncotarget (2015) 6(23):19706–20. doi: 10.18632/oncotarget.3565
58. Peters A, Rabe P, Liebing A-D, Krumbholz P, Nordström A, Jäger E, et al. Hydroxycarboxylic acid receptor 3 and GPR84 – Two metabolite-sensing G protein-coupled receptors with opposing functions in innate immune cells. Pharmacol Res (2022) 176:106047. doi: 10.1016/j.phrs.2021.106047
59. Mandrika I, Tilgase A, Petrovska R, Klovins J. Hydroxycarboxylic acid receptor ligands modulate proinflammatory cytokine expression in human macrophages and adipocytes without affecting adipose differentiation. Biol Pharm Bull (2018) 41(10):1574–80. doi: 10.1248/bpb.b18-00301
60. Feng X, Chen W, Ni X, Little PJ, Xu S, Tang L, et al. Metformin, macrophage dysfunction and atherosclerosis. Front Immunol (2021) 12:682853. doi: 10.3389/fimmu.2021.682853
61. Li SY, Guo YL, Tian JW, Zhang HJ, Li RF, Gong P, et al. Anti-tumor strategies by harnessing the phagocytosis of macrophages. Cancers (Basel) (2023) 15(10).
62. Wu H, Huang D, Zhou H, Sima X, Wu Z, Sun Y, et al. Metformin: A promising drug for human cancers. Oncol Lett (2022) 24(1):204.
63. Peters A, Krumbholz P, Jäger E, Heintz-Buschart A, Çakir MV, Rothemund S, et al. Metabolites of lactic acid bacteria present in fermented foods are highly potent agonists of human hydroxycarboxylic acid receptor 3. PloS Genet (2019) 15(5):e1008145.
64. Sakurai T, Horigome A, Odamaki T, Shimizu T, Xiao JZ. Production of hydroxycarboxylic acid receptor 3 (HCA(3)) ligands by bifidobacterium. Microorganisms (2021) 9(11).
Keywords: metformin, metabolomics, 3-hydroxy medium chain fatty acids, type 2 diabetes, anti-diabetic drugs
Citation: Naja K, Anwardeen N, Malki AM and Elrayess MA (2024) Metformin increases 3-hydroxy medium chain fatty acids in patients with type 2 diabetes: a cross-sectional pharmacometabolomic study. Front. Endocrinol. 15:1313597. doi: 10.3389/fendo.2024.1313597
Received: 10 October 2023; Accepted: 18 January 2024;
Published: 02 February 2024.
Edited by:
Rajakrishnan Veluthakal, City of Hope National Medical Center, United StatesReviewed by:
Daniele Vergara, University of Salento, ItalyCopyright © 2024 Naja, Anwardeen, Malki and Elrayess. This is an open-access article distributed under the terms of the Creative Commons Attribution License (CC BY). The use, distribution or reproduction in other forums is permitted, provided the original author(s) and the copyright owner(s) are credited and that the original publication in this journal is cited, in accordance with accepted academic practice. No use, distribution or reproduction is permitted which does not comply with these terms.
*Correspondence: Mohamed A. Elrayess, bS5lbHJheWVzc0BxdS5lZHUucWE=
Disclaimer: All claims expressed in this article are solely those of the authors and do not necessarily represent those of their affiliated organizations, or those of the publisher, the editors and the reviewers. Any product that may be evaluated in this article or claim that may be made by its manufacturer is not guaranteed or endorsed by the publisher.
Research integrity at Frontiers
Learn more about the work of our research integrity team to safeguard the quality of each article we publish.