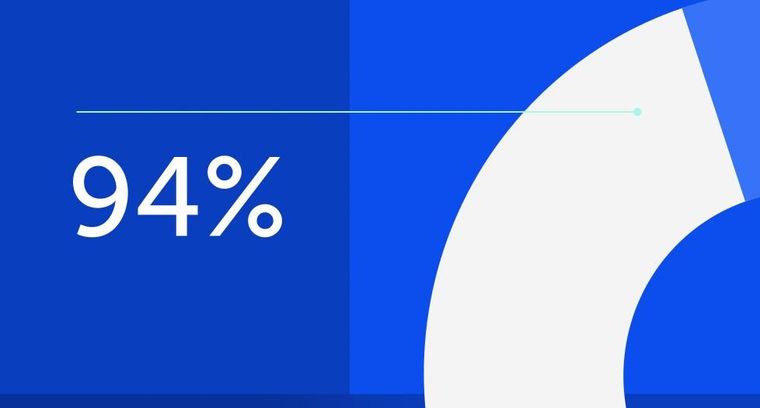
94% of researchers rate our articles as excellent or good
Learn more about the work of our research integrity team to safeguard the quality of each article we publish.
Find out more
SYSTEMATIC REVIEW article
Front. Endocrinol., 21 March 2024
Sec. Clinical Diabetes
Volume 15 - 2024 | https://doi.org/10.3389/fendo.2024.1286827
This article is part of the Research TopicThe Underlying Mechanisms and Novel Approaches for Diabetes and its Related ComplicationsView all 36 articles
Aim: The comparative effectiveness of basal insulins has been examined in several studies. However, current treatment algorithms provide a list of options with no clear differentiation between different basal insulins as the optimal choice for initiation.
Methods: A comprehensive search of MEDLINE, Embase, Cochrane Library, ISI, and Scopus, and a reference list of retrieved studies and reviews were performed up to November 2023. We identified phase III randomized controlled trials (RCTs) comparing the efficacy and safety of basal insulin regimens. The primary outcomes evaluated were HbA1c reduction, weight change, and hypoglycemic events. The revised Cochrane ROB-2 tool was used to assess the methodological quality of the included studies. A random-effects frequentist network meta-analysis was used to estimate the pooled weighted mean difference (WMD) and odds ratio (OR) with 95% confidence intervals considering the critical assumptions in the networks. The certainty of the evidence and confidence in the rankings was assessed using the GRADE minimally contextualized approach.
Results: Of 20,817 retrieved studies, 44 RCTs (23,699 participants) were eligible for inclusion in our network meta-analysis. We found no significant difference among various basal insulins (including Neutral Protamine Hagedorn (NPH), ILPS, insulin glargine, detemir, and degludec) in reducing HbA1c. Insulin glargine, 300 U/mL (IGlar-300) was significantly associated with less weight gain (mean difference ranged from 2.9 kg to 4.1 kg) compared to other basal insulins, namely thrice-weekly insulin degludec (IDeg-3TW), insulin degludec, 100 U/mL (IDeg-100), insulin degludec, 200 U/mL (IDeg-200), NPH, and insulin detemir (IDet), but with low to very low certainty regarding most comparisons. IDeg-100, IDeg-200, IDet, and IGlar-300 were associated with significantly lower odds of overall, nocturnal, and severe hypoglycemic events than NPH and insulin lispro protamine (ILPS) (moderate to high certainty evidence). NPH was associated with the highest odds of overall and nocturnal hypoglycemia compared to others. Network meta-analysis models were robust, and findings were consistent in sensitivity analyses.
Conclusion: The efficacy of various basal insulin regimens is comparable. However, they have different safety profiles. IGlar-300 may be the best choice when weight gain is a concern. In contrast, IDeg-100, IDeg-200, IDet, and IGlar-300 may be preferred when hypoglycemia is the primary concern.
Diabetes Mellitus (DM) is a chronic and progressive metabolic disorder that affects 9.3% of the world population (463 million people), with increasing incidence and prevalence (1). Type-2 diabetes mellitus (T2D) accounts for 90-95% of all cases and is caused by progressive insulin resistance and relative insulin deficiency (1). T2D can initially be treated with diet, increased physical activity, and oral glycemia-lowering medications (2, 3). However, as the disease progresses, many patients require and benefit from additional medications, including insulin, due to the progressive and gradual loss of insulin-producing cells in the pancreas over 5 to 10 years (4). Insulin initiation and intensification are required when lifestyle modifications, oral glucose-lowering drugs (OGLDs), Glucagon-like peptide 1 receptor agonists (GLP-1RAs), and SGLT2 inhibitors fail to provide adequate glycemic control (5, 6).
Although insulin was introduced nearly a century ago, and its use has prolonged survival and has saved the lives of countless people with diabetes, significant unmet needs remain (7–11). The proportion of people with T2D who achieve their glycemic goals with hypoglycemic events remains high (7, 9–11). This study focuses on various long-acting (basal) insulin preparations, their usage, efficacy, and side effects.
Neutral Protamine Hagedorn (NPH) insulin, a long-acting basal insulin preparation, has been considered the standard treatment for many years, and a large number of insulin analogs have been produced in recent years and are increasingly used in the treatment of diabetes (11). Despite the fundamental advances in their design, pharmacokinetics, and pharmacodynamics, hypoglycemia and weight gain remain two major problems with their use (8, 10). Significant improvements have been made with the introduction of long-acting insulin analogs regarding glycemic variability and risk of hypoglycemia (7, 11). Furthermore, new generations of basal insulin analogs have been developed to improve their efficacy and safety (11–15).
Several studies have compared first and second-generation basal insulins regarding glycemic control, weight gain, and hypoglycemic events; however, their results have been inconsistent and largely compared to insulin glargine and NPH (14–20). In the absence of adequate data on direct comparisons, network meta-analysis can synthesize evidence from direct and indirect comparisons of multiple interventions to determine the best available treatment option (21, 22).
The results of the present comprehensive systematic review and network meta-analysis address the challenging question regarding selecting the “best” treatment alternative (preferred or with a high priority to choose) among basal insulins. The purposes of the study included: determining the benefits (efficacy) and risks (safety) of basal insulins in T2D and determining the best treatment alternative (preferred or with a high priority to choose) among basal insulins in terms of glycemic control, weight gain, and hypoglycemic events. This can help clinicians, patients, and policymakers decide the best treatment options with optimal balance for increased efficacy and less harm.
The PRISMA-NMA guideline was followed to report the present systematic review and network meta-analysis (23). The protocol was registered in the International Prospective Register of Systematic Reviews, PROSPERO (CRD42022325625).
A comprehensive search of online databases, including MEDLINE, Embase, Cochrane Library, ISI, and Scopus, was performed through November 2023, using the MeSH terms ‘basal’ or ‘basal insulin’ or ‘neutral protamine Hagedorn’ or ‘NPH’ or ‘isophane’ or ‘insulin lispro protamine suspension’ or ‘ILPS’ or ‘human insulin’ or ‘glargine’ or ‘lantus’ or ‘long-acting insulin’ or ‘ultra long-acting insulin’ or ‘insulin analogue’ or ‘detemir’ or ‘levemir’ or ‘Toujeo’ or ‘degludec’ or ‘Tresiba’ AND ‘efficacy’ or ‘safety’ or ‘benefit’ or ‘risk’ or ‘glycemic’ or ‘glycated hemoglobin’ or ‘fasting plasma glucose’ or ‘fasting blood sugar’ or ‘body weight’ or ‘weigh gain’ or ‘hypoglycemia’ AND ‘diabetes mellitus.’ Also, meeting abstracts, ClinicalTrials.gov, and the annual meeting abstract books of the ADA and EASD were searched. The time and language of publications were not restricted. In addition, we contacted experts and other researchers in the field for ongoing studies and additional data using Email. We reviewed the reference lists of retrieved publications and relevant reviews for further pertinent studies. Duplicate publications were removed. Two authors (MD and MS) independently screened the studies based on titles and abstracts, and finally, the full texts were reviewed in case the studies met the inclusion criteria based on the title and abstract. Conflicts were resolved by consensus.
All phase III randomized controlled trials (RCTs) that compared basal insulins (long and ultra-long-acting insulins) with each other for study outcomes (glycemic control, weight gain, and hypoglycemia) in adults with T2D were included in the present systematic review. The population of interest was defined as adults with T2D, including those who were newly initiating insulin (insulin-naïve and on OGLDs), as well as individuals who were already exposed to insulin. This encompassed a diverse range of patients, reflecting both those at the initiation stage of insulin therapy and those with prior exposure to insulin treatments. Our decision to include both subgroups is supported by the findings of Freemantle et al. (24), who performed subgroup analysis specifically on studies assessing outcomes in insulin-naïve patients. Remarkably, their results indicated that the estimates remained largely unchanged even after including all studies, irrespective of the pretreatment status. Regarding the assessed interventions among RCTs, studies were considered eligible as long as the treatment regimens of the study arms contained at least two distinct basal insulins, including all intermediate/long and ultra-long-acting basal insulins including NPH, ILPS, glargine, 100 U/mL (IGlar-100); glargine, 300 U/mL (IGlar-300); detemir (IDet); degludec, 100 U/mL (IDeg-100); degludec, 200 U/mL (IDeg-200); and thrice-weekly degludec (IDeg-3TW). When the interventions comprised basal-bolus insulin regiments, the RCTs were included only when the short-acting components of the treatment regimens in various arms were precisely the same. Studies on Premixed insulin preparations and insulin peglispro were excluded (25). HbA1C, FPG, Body weight, Overall, Nocturnal, and severe hypoglycemia were all considered primary outcomes of the present study. RCTs were included when they assessed and compared these outcomes in T2D patients between different intervention arms. A minimum follow-up time of 12 weeks was also deemed an inclusion criterion. Inhaled insulins were also excluded. Glargine biosimilars (including MK-1293, MYL-1501D, LY2963016, etc.) were also excluded due to their similar efficacy and safety profile and similar pharmacokinetic and pharmacodynamics properties with the reference product (glargine). An adequate and standard randomization process in the RCTs was considered a vital inclusion criterion. The studies were included in the review if the two treatment arms were similar regarding main baseline variables, namely diabetes duration, mean age of the participants, HbA1c level, and body mass index. In addition, patients’ antidiabetic treatments prior to study initiation were assessed. In studies evaluating patients with different treatment backgrounds, ensuring that the two arms did not differ regarding the pretreatment medications or that pretreatments were considered during the randomization and stratification of patients was crucial.
The following studies were excluded: those with no control group, non-randomized trials, post-hoc or pooled analyses, real-world data, OGLDs/placebo/unknown comparison groups, unknown treatment period, follow-up period less than 12 weeks, data registries, non-standard randomized clinical trial, incorrect, insufficient or incomplete statistical analyses. In addition, studies including participants with cardiovascular or renal diseases or people with known risk factors for cardiovascular diseases were excluded. Figure 1 shows the process of identifying, screening, and selecting randomized controlled trials to include in the systematic review and final analyses.
The main characteristics that were extracted from eligible studies were as follows: author name, publication year, name of the country, gender, age, body weight, body mass index, fasting blood sugar, HbA1c, study setting and population, duration of follow-up, type of diabetes, diabetes duration, number of patients in each treatment group, treatment regimens, insulin dosage, study outcomes (change in HbA1c from baseline, change in fasting plasma glucose (FPG) from baseline, change in body weight from baseline, the number of patients experiencing any/overall, nocturnal, or severe hypoglycemia events, effect size estimates (odds ratio or mean difference) and their 95% confidence intervals. Two reviewers (MD and MS) extracted the data independently, and the observed differences were resolved by consensus of review team members.
To assess the risk of bias or methodological quality of included RCTs, the new and revised Cochrane risk of bias tool (ROB-2) was used (26). The tool consists of five domains: bias based on the randomization process, deviations from intended interventions, missing outcome data, measurement of the outcome, and selection of the reported results. Each domain contains several questions, and the answer to each question has five options (yes, probably yes, probably no, no, no information). At the end of each domain, a risk of bias judgment is made for the domain as either low risk of bias, some concerns, or high risk of bias. Finally, an overall judgment of the risk of bias is made for each study, which summarizes all five domains (26). Two reviewers (MD and MS) assessed the risk of bias in studies, and three (HRB, LJ, and FB) verified it.
Basal insulin regimens were compared in all RCTs that were included. Treatment effects were estimated as mean differences (MDs) with 95% confidence intervals in HbA1c, fasting plasma glucose, and body weight (continuous outcomes), or odds ratios (ORs) with 95% confidence intervals (CIs) for any/overall, nocturnal, and severe hypoglycemia. Random-effects network meta-analyses within frequentist general linear mixed model framework were performed for data analyses. Network plots containing all basal insulin treatments were drawn for each outcome (27).
Extended forest plots were used to show pairwise comparisons of treatments using direct evidence. To assess the uncertainty in the estimated treatment effects, which includes the extent of heterogeneity, the predictive interval (PrI) plot was also used. Estimated treatment effects in a random-effects network meta-analysis for pairwise comparisons of all evidence in the network (both direct and indirect evidence) with 95% CIs were displayed using a league table. Diagonal cells contain the names of competing treatments in the network in the league table.
The assumption of heterogeneity was examined both qualitatively and quantitatively using study and participant characteristics across all eligible trials and the magnitude of tau2 (between study variance). We assessed the transitivity assumption by considering the distributions of potential effect modifiers (age, diabetes duration, baseline HbA1c level, and baseline body mass index) across pairwise comparisons. A design-by-treatment approach was used to check the consistency assumption in the entire analytical network (28). A loop-specific approach was applied to evaluate the presence of inconsistency locally in each closed loop (29). Inconsistency factor (IF) for continuous outcomes and the ratio of two odds ratios (ROR) for dichotomous outcomes in each closed loop and the ifplot was used for this purpose. The node-splitting method was also used to assess the inconsistency of the model by separating evidence on particular comparisons into direct and indirect evidence (30).
Sensitivity analyses were conducted by restricting the analyses to studies with a sample size of more than 100 participants in each treatment arm of the trial, only trials that had a low risk of bias, and trials with a parallel design (excluding the crossover designs) to assess the generalizability and the robustness of the network meta-analyses findings for each outcome. If any differences were observed in the distribution of effect modifiers (participants’ baseline characteristics) across the studies, subgroup analyses and, in particular, meta-regression were performed. The results before and after the adjustment with the effect modifiers were evaluated to derive clinically valid conclusions.
To rank the basal insulins for an outcome, we used mean rank and surface under the cumulative ranking (SUCRA) cumulative probabilities that express (as a percentage) the efficacy or safety of every intervention relative to an imaginary intervention that is always the best without uncertainty. Consequently, larger SUCRA scores might indicate a more effective or safer intervention. The presence of small-study effects in a network meta-analysis was assessed by comparison-adjusted funnel plots (31). All network meta-analyses were performed using Stata version 17.0 (Stata Corp., College Station, TX, USA) with a multivariate meta-analysis model using the mvmeta command and routines in Stata and R version 3.3.0 using the netmeta package.
We used the approach suggested by the GRADE (Grading of Recommendations Assessment, Development, and Evaluation) working group for rating the certainty of evidence for each network estimate (32–35). This approach ranks the overall certainty in effect estimates from very low to high (4 levels).
We initially rated the certainty of direct estimates for each pair-wise comparison. At this stage, since all the evidence was from RCTs, we started at high certainty in effect estimates and rated down in cases of serious concerns regarding the risk of bias, publication bias, indirectness, and inconsistency. Then, we rated the certainty in indirect estimates, focusing on the dominant lowest-order loop that contributed to the indirect estimate. The indirect estimate would be further rated down for concerns regarding intransitivity. The main effect-modifying factors that were considered to cause conceptual transitivity were baseline BMI, baseline HbA1c, duration of follow-up, and duration of DM. Cut-offs for decision-making were set based on the standard minimally clinically important differences as suggested by previous studies in patients with T2D.
To judge the overall certainty in the network estimates, we selected the certainty in the estimate that contributed more to the network estimate between the direct and indirect estimates as a baseline. We then considered rating down the certainty of the network estimate if there was incoherence (local inconsistency) between the contributing direct and indirect estimates. The certainty of the network estimate was subsequently assessed for serious or very serious imprecision that would further downgrade the overall certainty in the effect estimate according to the GRADE guidance articles (33, 34).
Using a minimally contextualized approach, we then chose the intervention with the highest number of studies (IGlar-100) as the reference and categorized the interventions for each outcome into three colored groups relative to this reference: 1) Among the most effective/least harmful (Green), 2) Not convincingly different from standard treatment (Yellow), and 3) Among the most harmful/least effective (Red). We further categorized each of these groups based on certainty about the effect estimate of the intervention relative to IGlar-100 as obtained from the GRADE approach, coloring the “very low” to “low” certainty evidence with light colors and “moderate” to “high” certainty evidence in dark colors (36, 37).
We identified 20,817 records through a comprehensive systematic search of databases. One thousand two hundred-and-thirty-three (1,254) records remained after removing duplicates and studies unrelated to the research question. After excluding the studies that did not meet the eligibility criteria, 226 full texts were assessed and selected 67 RCTs in the systematic review based on the defined criteria. In our network meta-analyses, we conducted separate analyses for changes in HbA1c (44 RCTs included), changes in body weight (38 RCTs included), overall hypoglycemia (43 RCTs included), and nocturnal hypoglycemia (40 RCTs included). Inclusion criteria were based on methodological, clinical, and baseline homogeneity, as illustrated in Figure 1.
Supplementary Table 1 demonstrates the main characteristics of the 44 randomized controlled clinical trials included in this analysis (14, 17, 18, 38–78). The included trials were published between 2000 and 2021. Most trials were multicenter and multinational from most to all continents and with relatively large sample sizes. Only five studies had a total sample size of smaller than 100 participants, i.e., less than 50 patients in each group (31, 45, 61, 70, 71), and about 80% of trials had included more than 100 patients in each study arm (Supplementary Table 1). Three studies were conducted as a crossover clinical trial design (39, 42, 61), and the rest were parallel clinical trials. We handled these crossover trials similarly to parallel trials by only extracting data from the first period (79). Regarding the sample size and design of trials, sensitivity analyses were performed to assess the robustness of the findings.
Both men and women were included in all studies. The mean age of patients with T2D was 58.7 years (range 54 to 66 years). The mean treatment period (follow-up period) was 38 weeks, and the mean duration of T2D was 10.8 (range 6.8 to 16) years. The mean HbA1c level and body mass index (BMI) at baseline were 8.5% (range 7.1% to 9.5%) and 30.2 (range 24.6 to 36.6), respectively. Moreover, the qualitative synthesis revealed a diverse range of pretreatment backgrounds among patients initiating basal insulins in included studies, with 27 RCTs specifically comparing efficacy and/or safety in insulin-naive patients (solely using oral anti-diabetic therapy), six assessing those already using basal insulin, three examining a population comprised of both insulin-naive individuals and those already using basal insulins, and eight RCTs focusing on patients utilizing a basal-bolus regimen before the commencement of the trial.
All 44 randomized clinical trials were assessed for methodological quality, the results of which are presented in detail in Supplementary Table 2. Based on the assessment, two RCTs (4.5% of studies) were at high risk of bias, nine RCTs (19.5% of studies) posed some concerns at risk of bias, and 35 RCTs (76% of the studies) were placed in the low risk of bias category. More than two-thirds of the studies had a low risk of bias because of their standard design, conduct, analysis, and reporting. Most of the studies suffered from two domains: deviations from intended interventions and the measurement of the outcome. Deviation from the intervention was almost balanced among the groups in most of the studies, and the outcome assessors were aware of the type of insulin received by the patients in some studies. Sensitivity analysis was also conducted by excluding studies with a high risk of bias to examine the robustness of the model findings.
Network plots compare basal insulins regarding the effect on HbA1c level, FPG, body weight, any/overall, nocturnal, and severe hypoglycemia outcomes (six networks) (Figure 2). Basal insulins in the main analyses included IGlar-100, IGlar-300, IDet, IDeg-100, IDeg-200, IDeg-3TW, NPH, and ILPS. Among all comparisons, IGlar-100 was the most commonly used treatment (more participants were assigned in the trials). The number of RCTs comparing IGlar-100 against NPH and IDeg-100 was more than the other comparators in all networks.
Figure 2 Network plots comparing basal insulins for change in HbA1c level (A), FPG (B), body weight (C), overall/any hypoglycemia (D), nocturnal hypoglycemia (E), and severe hypoglycemia (F) in people with T2DM. Each node (circle) represents a basal insulin regimen, and its size is proportional to the number of participants randomly assigned to each treatment. The width of the line joining two nodes is proportional to the number of trials that directly compare the two respective treatments. Glargine, 100 U/mL (IGlar-100); Glargine, 300 U/mL (IGlar-300); Detemir (IDet); Degludec, 100 U/mL (IDeg-100); Degludec, 200 U/mL (IDeg-200); Thrice-weekly degludec (IDeg-3TW).
The distribution of potential effect modifiers, including baseline HbA1c level and body weight as well as age and duration of T2D, were examined qualitatively; they were relatively the same across included studies in all six networks, so the transitivity assumption was accepted. Heterogeneity was assessed qualitatively by examining participants’ clinical and methodological characteristics across trials. Heterogeneity was also examined visually through the predictive interval plot. This plot assessed the uncertainty in the estimated treatment effects, which also includes the extent of heterogeneity (tau2 for HbA1c=0.01, for FPG=0.17, for body weight=0.23, for overall hypoglycaemia=0.02, for nocturnal hypoglycaemia=0.01, and for severe hypoglycaemia=0.001 indicating low heterogeneity among the studies in all networks).
Local consistency was evaluated using a loop-specific approach and confirmed (except for 2 of the 8 closed loops in HbA1c, 3 of the 8 closed loops for FPG, 2 of the 8 closed loops for body weight, 2 of the 8 closed loops for overall hypoglycemia, and 3 of the 8 closed loops for nocturnal hypoglycemia). In each network, most of the studies with small sample sizes and larger estimated standard errors caused local inconsistency. After excluding these trials from the networks (seven studies in the FPG network, four studies in body weight, three studies in overall hypoglycemia, and four studies in nocturnal hypoglycemia network), local consistency was established in each closed loop and led to more global consistency in some networks. Local consistency was also confirmed by comparing direct and indirect estimates through the node-splitting method (none of the p-values were statistically significant). Global consistency through the design-by-treatment interaction model showed no inconsistency in the networks (p-values for consistency ranged from 0.43 to 0.98 in networks of outcomes). The qualitative and quantitative assessments indicated that the network meta-analyses’ consistency was logical.
The league table shows the random-effects network meta-analysis estimates (relative treatment effects, pooled mean difference) for comparison of basal insulins to change HbA1c level (Table 1). The findings of 44 RCTs (28 direct and indirect comparisons) indicated that IDeg-200 (pooled MD: -0.21% with 95% CI: -0.12% to -0.30%), IDeg-100 (Pooled MD:-0.15% with 95% CI: -0.02% to -0.27%), IDeg-3TW (Pooled MD: -0.27% with 95% CI: -0.08% to -0.45%), IDet (Pooled MD: -0.23% with 95% CI: -0.10% to -0.36%), and NPH (Pooled MD: -0.16% with 95% CI: -0.05% to -0.27%) are statistically significant effective than IGlar-100 in reducing HbA1c level. No other significant differences were identified in comparing basal insulins regarding changes in HbA1c levels. It seems that the observed difference between these insulins is not clinically significant, and therefore it can be said that almost all of them are equally effective in reducing the HbA1c level and do not have any particular preference over each other. Consistent results were observed when the included studies were restricted to RCTs with sample sizes of more than 100 patients in each study arm (excluding nine trials), parallel trials (excluding three trials), and RCTs with low risk of bias in separate sensitivity analyses. Visual inspection of the funnel plot did not reveal evidence of small-study effects (likely no publication bias).
Table 1 Random-effects network meta-analyses estimate for all direct and indirect evidence comparing basal insulins regarding the defined outcomes (A-F) for T2DM.
Forty RCTs that compared eight basal insulins were included in the network meta-analysis. Based on an extended forest plot for direct evidence and a predictive interval plot using direct and indirect evidence, except for a few comparisons, there is no clinically significant difference between basal insulins regarding the effect on FPG level in T2D.
Estimates from network meta-analysis for all 28 direct and indirect comparisons (league Table, Table 1) showed that IDeg-3TW and IDeg-100 compared to IGlar-300 (Pooled MD:-13.8 mg/dL with 95% CI: -22.7 to -4.8 and -12.4 mg/dL with 95% CI: -20.3 to -4.5 respectively), IGlar-100 (Pooled MD: -10.8 mg/dL with 95% CI: -17.05 to -4.5 and -9.9 mg/dL with 95% CI: -14.3 to -4.5 respectively), IDet (Pooled MD: -8.6 with 95% CI: -13.6 to -3.6 and -7.3 mg/dL with 95% CI: -11.2 to -3.4 respectively), ILPS (Pooled MD: -10.1 with 95% CI: -17.1 to -3.0 and -8.6 mg/dL with 95% CI: -14.5 to -2.8 respectively), and NPH (Pooled MD: -9.9 with 95% CI: -15.6 to -4.3 and -8.6 mg/dL with 95% CI: -12.8 to -4.4 respectively) were slightly more effective in reducing FPG level, but this magnitude of difference was not clinically important. Results were consistent in sensitivity analyses when included studies were restricted to RCTs with a low risk of bias and a sample size of more than 100 patients in each treatment arm. Inspection of the funnel plot indicated no evidence of publication bias, implying no evidence of small-study effects in the network.
Data on the changes in body weight from baseline were available from 36 RCTs. The league table findings from the network meta-analysis for all 28 direct and indirect comparisons of eight basal insulins indicated that patients with T2D treated with IGlar-300 were significantly associated with less body weight gain compared to IDeg- 3TW (pooled MD: -4.12 kg with 95% CI: -0.87 kg to -7.36 kg), IDeg-100 (pooled MD: -2.96 kg with 95% CI: -0.06 kg to -5.8 kg), IDeg- 200 (Pooled MD: -3.65 kg with 95% CI: -0.85 kg to -6.45 kg), NPH (Pooled MD: -3.66 kg with 95% CI: -0.72 kg to -6.61 kg), and IDet (Pooled MD: -3.1 kg with 95% CI: -0.14 kg to -6.08 kg) (Table 1). The weight gain by IGlar-300 compared to other basal insulins is considered important from a clinical point of view. It might represent this insulin as the preferred choice regarding the less harmful effect on body weight (where the weight gain is of lower magnitude). Sensitivity analyses demonstrated the consistency of the results, indicating that the network meta-analysis model was robust. The comparison-adjusted funnel plot showed publication bias was unlikely.
Forty-three randomized clinical trials were considered in the network meta-analysis to compare the effect of eight basal insulins on hypoglycemia. Overall (any) hypoglycemia was defined as typical symptoms associated with hypoglycemia with or without a plasma glucose level (3.9 mmol/L or less) or a confirmed plasma glucose level of 3.9 mmol/L or less. In general, findings from the extended forest plot for direct evidence and the predictive interval plot using direct and indirect evidence showed a significant difference in the incidence of overall/any hypoglycemia between some comparisons.
The findings from the network meta-analysis of 43 RCTs (league table) indicated that the use of all basal insulins except IDeg-3TW was associated with significantly lower odds of hypoglycemia compared to NPH (ranging from 25% (IGlar-100) to 48% (IDeg-100)). The odds of any hypoglycemia in patients with T2D treated with IDeg-200 (pooled odds ratio: 0.78 with 95% CI: 0.66 to 0.93) and IDeg-100 (pooled OR: 0.69 with 95% CI: 0.51 to 0.92) were significantly lower than IGlar-100. IDet was also associated with lower odds of overall hypoglycemia compared to IGlar-100. No significant differences were observed for other treatment comparisons (Table 1). Both findings of relative ranking and network meta-analysis estimates showed that IDeg-100, IDeg-200, and IDet could be preferred choices, and IDeg-3TW and NPH should be the least favored options for attaining less hypoglycemia. Small-study effects were unlikely in assessing comparison-adjusted funnel plots in the network.
To compare basal insulins to investigate their effect on nocturnal hypoglycemia, 40 RCTs contributed to the network. All trials included in the network meta-analysis defined nocturnal hypoglycemia as any hypoglycemic event between bedtime and waking (usually between 12:00 PM and 6:00 AM). Extended forest and predictive interval plots showed significant differences in the occurrence of nocturnal hypoglycemia in some comparisons among basal insulins.
Administration of all basal insulins except IDeg-3TW compared to NPH and ILPS significantly reduced the odds of nocturnal hypoglycemia (Table 1). IGlar-100 and IGlar-300 significantly increased the odds of nocturnal hypoglycemia compared to IDet (pooled OR: 1.32 with 95% CI: 1.11 to 1.56; and pooled OR: 1.30 with 95% CI: 1.01 to 1.67 respectively). Patients with T2D treated with IDeg-100 had significantly lower odds of nocturnal hypoglycemia compared to other basal insulins [except IDeg-200) (ranging from 28% (against IDet) to 68% (against NPH)]. As with overall hypoglycemia, it can be stated that IDeg-100, IDeg-200, and IDet could be the first choices for obtaining less nocturnal hypoglycemia, and the use of NPH and IDeg-3TW should be the last priority in terms of prevention of nocturnal hypoglycemia. Results were consistent in sensitivity analyses when only low-risk-of-bias trials, parallel designs, and studies with more than 100 patients per arm were included. There was no evidence of publication bias.
Thirty-five RCTs were included in the network meta-analysis to assess basal insulins’ effect on severe hypoglycemic events. All studies consistently defined severe hypoglycemia as an event that required third-person assistance. Considerable difference in the occurrence of severe hypoglycemia was observed in some comparisons among basal insulins based on extended forest plots using direct evidence and predictive interval plots using direct and indirect evidence.
Treatment with IDeg-100, IDeg-200, and IGlar-300 was associated with significantly reduced odds of severe hypoglycemia compared to NPH and ILPS. IDeg-100 significantly resulted in lower odds of severe hypoglycemia compared to IGlar-100, IDet, ILPS, and NPH (pooled OR: 0.38 with 95% CI: 0.20 to 0.75; pooled OR: 0.41 with 95% CI: 0.23 to 0.70; pooled OR: 0.15 with 95% CI: 0.04 to 0.52; and pooled OR: 0.28 with 95% CI: 0.13 to 0.59) respectively (Table 1). Both relative ranking and league table findings indicated that the first choices that result in less severe hypoglycemia could be IDeg-100, IDeg-200, and IGlar-300, and the last options are NPH, ILPS, and IDeg-3TW. The network meta-analysis model was robust, and findings were consistent in sensitivity analyses restricted to large trials and studies with low risk of bias. Small-study effects were unlikely in assessing comparison-adjusted funnel plots in the network.
To achieve accurate and reliable findings, network meta-regressions were performed considering HbA1c, body mass index, and age of patients at baseline. However, due to the homogeneity among studies and consistency of the networks, no significant differences were observed in the estimated treatment effects (data not shown).
Despite the statistically significant difference in the reduction of HbA1c among specific network comparisons, these differences did not reach the minimal clinically important differences (more than 0.5%). Although the evidence is relatively uncertain for this outcome (most comparisons had low to very low certainty), the current effect estimates differ by such a small magnitude that future studies are unlikely to change this conclusion. Similarly, the effects of different basal insulins on the reduction of FPG were comparable, with statistically significant differences ranging between 3 to 13 mg/dl.
Regarding the effect of insulins on patients’ weight, IGlar-300 and IGlar-100 may be associated with less weight gain than other basal insulins. However, these differences were slight (3-4 kg), and the effect estimates were imprecise (low to very low-quality evidence).
Moderate to high certainty evidence showed that IDeg-100 and IDeg-200 were the safest options when considering hypoglycemia-related outcomes, followed by IDet for nocturnal and overall hypoglycemia. The next safe option regarding nocturnal and overall hypoglycemia was IDeg-3TW (low-certainty evidence). Based on moderate-quality evidence, ILPS, IGlar-100, and IGlar-300 have similar profiles concerning overall hypoglycemia. However, ILPS was associated with higher rates of nocturnal hypoglycemia than IGlar-100 (moderate-certainty evidence). The intermediate-acting insulin NPH was associated with the highest odds of overall and nocturnal hypoglycemia (moderate-certainty evidence). Table 2 presents the summary of findings using the GRADE minimally-contextualized framework.
Table 2 The summary of findings table with the certainty of the rankings using the GRADE minimally-contextualized framework.
The current study’s findings enable patients and healthcare practitioners to make informed choices to individualize insulin therapy, depending on whether weight gain or hypoglycemia is the most significant concern in the shared decision-making process. The control and management of T2D are always complex and require careful consideration of patient-related factors such as capability and desire for self-care, co-morbidities, and costs (80). Type 2 diabetes is a progressive disease, and many patients require injectable medications, including insulin, at some point in the course of the disease (81, 82). As a result, decision-makers and clinicians must rely mainly on research evidence and clinical judgment in choosing a specific basal insulin among all available basal insulins. By conducting a network meta-analysis of the current evidence, we try to address these issues and provide evidence for decision-makers and clinicians to select the most appropriate option for their patients. For instance, older, frail patients who are at the highest risk of hypoglycemia may choose to prioritize insulins that have a lower risk of hypoglycemia. Conversely, insulins that have a lower propensity to cause weight gain may be the preferred option in patients who are concerned about the complications of becoming overweight or obese.
We combined direct and indirect evidence to compare the effects of basal insulin regimens on HbA1c reduction, weight gain, and hypoglycemic events. Regarding glycemic control, subtle yet statistically significant differences were observed when comparing the efficacy of distinct basal insulin regimens in reducing HbA1C. It is crucial to emphasize that relying solely on the interpretations derived from a network meta-analysis may not align perfectly with clinical practice objectives. Various guidelines, including the recent National Institute for Health and Care Excellence (NICE) recommendations for diabetes management, have established a more than 0.5% change in HbA1C as the minimal clinically significant threshold (83). Our analysis demonstrated that neither the crude calculated differences nor their associated confidence intervals surpassed this threshold. Consequently, despite statistical significance, we deemed these observed differences clinically insignificant.
Regarding changes in body weight, IGlar-300 was associated with the least weight gain compared to insulin degludec, detemir, and NPH. Treatment with NPH was associated with the highest odds of overall and nocturnal hypoglycemia compared to all other basal insulins, while patients treated with IDeg-100, IDeg-200, IDet, and IGlar-300 were less likely to experience overall and nocturnal hypoglycemia than the patients treated with other basal insulin regimens. Treatment regimens that consisted of NPH and ILPS were more likely to cause severe hypoglycemia, whereas treatment with IDeg-100 was associated with a significant reduction in the odds of severe hypoglycemia compared to IGlar-100 and IDet, NPH, and ILPS. NPH insulin exhibits lower efficacy in weight management and has a higher propensity to induce hypoglycemic events compared to other basal insulins. These observations are consistent with each other since the increased weight gain associated with NPH may be partially attributed to the overconsumption of food triggered by the hypoglycemic episodes elicited by this drug.
Choosing the optimal treatment among intermediate, long, and ultra-long-acting basal insulins in people with T2D should be based on the effects of insulins on clinically relevant parameters, including unmet needs (84). The present study’s findings indicate that newer-generation basal insulins do not seem to improve glycemic control compared to first-generation basal insulins such as insulin glargine-100, insulin detemir, or even NPH insulin. However, where less weight gain is a priority, insulin glargine-300 is the superior choice. Also, considering nocturnal hypoglycemia, administration of insulin degludec-100, insulin degludec-200, or insulin glargine-300 is associated with the lowest incidence odds.
We did not find a significant difference between IDeg-100 and IDeg-200 in the present study. Therefore, both could be considered potential clinical practice options when indicated (24). Challenges remain regarding the clinical use of ILPS, as it is associated with an increased risk of severe and nocturnal hypoglycemia without relative beneficial effects on blood glucose control or body weight. It is also recommended to consider patients’ compliance with treatment and cardiovascular safety in deciding on the best treatment options (85–87).
To our knowledge, cardiovascular outcomes have not been compared in patients receiving different basal insulins. However, a recent meta-analysis by Rados et al. demonstrated that basal insulins, regardless of their type, are not associated with an increased risk of either cardiovascular events incidence or all-time cardiovascular mortality compared to other potential T2D treatments (88). Further investigation and comparison of different basal insulin effects on cardiovascular outcomes can aid physicians in devising personalized treatment plans best suited to individual patients’ unique needs.
Patients’ adherence significantly affects the efficacy of glucose-lowering agents. Although treatments demonstrate comparable effectiveness in randomized controlled trials, it’s crucial to recognize that trial participants are a selectively motivated and highly adherent group. As a result, findings from clinical trials may not consistently represent the broader characteristics of the general population (89, 90).
Experience from real-world data suggests that T2D treatment adherence is greatly influenced by the ease of drug administration, dosing frequency, treatment complexity, out-of-pocket costs, and incident hypoglycemia (91). For example, non-adherence is more significant with injectable drugs than with oral medications, as drug injections are more challenging and can be inconvenient for patients (92). Similarly, adherence is better for insulin pens than for insulin administered by vial and syringe (91, 93). Hence, when comparing ease of use between medication groups, differences between insulin administered by vial and syringe versus insulin done through the pen should be considered.
As T2D advances, individuals on NPH insulin may require more than one injection per day (5), a factor that could compromise treatment adherence. Considering its suboptimal performance in various aspects of efficacy and safety, the utilization of NPH insulin is recommended primarily in situations where cost considerations weigh significantly on the patient’s decision-making process.
In a similar design to the current study, Madenidou et al. conducted a network meta-analysis on RCTS assessing the efficacy and safety of basal insulins in T2D patients in 2018. They indicated that the difference in efficacy among basal insulin analogs is minimal (94). The present study’s findings suggest the same regarding the efficacy of basal insulins. They also observed that IDet was associated with less weight gain than other basal insulins, and IGlar-300 had a more favorable weight gain profile than IDeg-100, IDeg-200, Ideg-3TW, and IGlar-100. Similarly, we report less weight gain associated with IGlar 300 than IDeg-100, IDeg-200, and Ideg-3TW. However, our network meta-analysis showed the superiority of IGlar 300 to IDet regarding weight gain. Moreover, Madenidou et al. indicated that the incidence of severe hypoglycemia did not differ between basal insulins, except for ILPS, which was associated with a higher risk of hypoglycemia compared to other basal insulin regimens. It should be noted that the researchers included Glargine biosimilars and did not include NPH insulin in their review. In contrast, the present network meta-analysis included NPH insulin as well as larger randomized clinical trials reported in recent years that are expected to further contribute to the external validity or generalizability of the findings in the current study. Insulin glargine and glargine biosimilars were similar in terms of efficacy and safety related to the reduction of HbA1c level, effect on body weight, or occurrence of hypoglycemia in various reported studies as well as in a comprehensive systematic review (94, 95); hence, glargine biosimilars were not included in our review and analyses. In 2016, Freemantle et al. conducted a network meta-analysis on randomized clinical trials comparing different basal insulins (including IGlar-300, IGlar-100, NPH, Detemir, and Degludec) and premixed insulins with each other in T2D patients (96). They reported the superiority of IGlar-300 over NPH and premixed insulins in reducing hypoglycemic events. They also stated that the safety of other basal insulins is comparable. In line with their findings, we also report the superiority of IGlar-300 over NPH. However, the results of the current meta-analysis suggest the superiority of IDeg-100 and IDeg-200 in reducing the odds of nocturnal hypoglycemia. This might be due to the fact that the findings of more recent large sample RCTs comparing Degludec and Glargine insulins have been released since they conducted their network meta-analysis. It is also worth mentioning that Freemantle et al. included premixed insulins in their network. In contrast, our analysis focused exclusively on basal insulins, contributing to a more targeted investigation of this specific insulin category.
There are some limitations in the present study. The findings of the network meta-analysis should be interpreted with caution in the context of limitations of the available data. The comparative effectiveness of newer basal insulins has been based mainly on indirect comparisons. Therefore, any inferences about the favorable effect of second-generation basal insulins should be interpreted with caution. Differences in the use of background glucose-lowering medications among some trials are important to consider. Although we tried to maintain homogeneity among the participants, the efforts did not eliminate heterogeneity ideally. Measurement of specific outcomes between intervention groups has been similar in the reviewed studies. Still, in some cases, the outcome assessors were aware of the type of insulin received by the patients. Therefore, the outcome measurement may be affected by the knowledge of the type of insulin received. Also, the variation in daily insulin dose plans among the trials included in the analyses should be considered. Also, the present network meta-analysis has the same limitations and common challenges present in other network meta-analyses (97), indicating that the findings should be interpreted cautiously. We took steps to minimize these limitations by applying methods proposed by GRADE (32–37) and presented the findings’ certainty and the effect estimates to avoid misinterpretation of the results.
The present study also has some strengths. Publication bias was most unlikely in our systematic review because we have searched multiple sources, including abstracts and trial registries. The favorable quality of the included studies (76% of the trials had a low risk of bias) and the multinational and multicenter nature of the majority of trials with a considerable number of participants strengthen the validity and confidence of the research findings. The low heterogeneity of the participants (especially in terms of the main and important baseline variables), study designs, and the clinical characteristics of the patients led to the acceptable consistency of the networks and relatively realistic findings.
Findings of the combination of direct and indirect evidence with acceptable quality indicate that basal insulin regimens are comparable in glycemic control in people with T2D. Insulin glargine, 300 U/mL, may be associated with a slightly less severe weight gain than other basal insulins. Insulin degludec 100 U/mL, degludec 200 U/mL, detemir, and glargine 300 U/mL are preferred options when hypoglycemia is the primary concern.
The original contributions presented in the study are included in the article/Supplementary Material, further inquiries can be directed to the corresponding author/s.
MD: Writing – original draft, Visualization, Software, Methodology, Investigation, Data curation, Writing – review & editing, Conceptualization. MS: Writing – original draft, Writing – review & editing, Software, Methodology, Investigation, Formal analysis, Data curation. FB: Writing – review & editing, Visualization, Investigation, Formal analysis. ZM: Writing – review & editing, Supervision, Methodology. LJ: Writing – review & editing, Supervision, Formal analysis. SM: Writing – review & editing, Supervision, Methodology. YL: Writing – review & editing, Methodology. FI: Writing – review & editing, Writing – original draft, Supervision, Methodology. HB: Writing – review & editing, Supervision, Conceptualization. MK: Writing – review & editing, Writing – original draft, Supervision, Methodology, Investigation, Conceptualization.
The author(s) declare that no financial support was received for the research, authorship, and/or publication of this article.
The authors would like to thank Ms. Roya Vesal Azad, an information specialist, for technical assistance in the comprehensive systematic search of the databases.
The authors declare that the research was conducted in the absence of any commercial or financial relationships that could be construed as a potential conflict of interest.
All claims expressed in this article are solely those of the authors and do not necessarily represent those of their affiliated organizations, or those of the publisher, the editors and the reviewers. Any product that may be evaluated in this article, or claim that may be made by its manufacturer, is not guaranteed or endorsed by the publisher.
The Supplementary Material for this article can be found online at: https://www.frontiersin.org/articles/10.3389/fendo.2024.1286827/full#supplementary-material
1. Saeedi P, Petersohn I, Salpea P, Malanda B, Karuranga S, Unwin N, et al. Global and regional diabetes prevalence estimates for 2019 and projections for 2030 and 2045: Results from the International Diabetes Federation Diabetes Atlas. Diabetes Res Clin Pract. (2019) 157:107843. doi: 10.1016/j.diabres.2019.107843
2. ElSayed NA, Aleppo G, Aroda VR, Bannuru RR, Brown FM, Bruemmer D, et al. Facilitating positive health behaviors and well-being to improve health outcomes: standards of Care in Diabetes—2023. Diabetes Care. (2023) 46:S68–96. doi: 10.2337/dc23-S005
3. ElSayed NA, Aleppo G, Aroda VR, Bannuru RR, Brown FM, Bruemmer D, et al. Classification and diagnosis of diabetes: standards of care in diabetes—2023. Diabetes Care. (2023) 46:S19–40. doi: 10.2337/dc23-S002
4. Burrows NR, Li Y, Gregg EW, Geiss LS. Declining rates of hospitalization for selected cardiovascular disease conditions among adults aged≥35 years with diagnosed diabetes, U.S., 1998e2014. Diabetes Care. (2018) 41:293e302. doi: 10.2337/dc17-1259
5. ElSayed NA, Aleppo G, Aroda VR, Bannuru RR, Brown FM, Bruemmer D, et al. Pharmacologic approaches to glycemic treatment: standards of care in diabetes—2023. Diabetes Care. (2023) 46:S140–57. doi: 10.2337/dc23-S009
6. De Block CE, Van Cauwenberghe J, Bochanen N, Dirinck E. Rapid-acting insulin analogues: Theory and best clinical practice in type 1 and type 2 diabetes. Diabetes Obes Metab. (2022) 24:63–74. doi: 10.1111/dom.14713
7. Cahn A, Miccoli R, Dardano A, Del Prato S. New forms of insulin and insulin therapies for the treatment of type 2 diabetes. Lancet Diabetes Endocrinol. (2015) 3:638–52. doi: 10.1016/S2213-8587(15)00097-2
8. DeFronzo RA, Ferrannini E, Zimmet P, Alberti KGMM eds. International Textbook of Diabetes Mellitus. 4th ed. Sussex, England: John Wiley and Sons (2015) 3–1224.
9. Owens DR, Matfin G, Monnier L. Basal insulin analogues in the management of diabetes mellitus: What progress have we made? Diabetes Metab Res Rev. (2014) 30:104–19. doi: 10.1002/dmrr.2469
10. Owens DR, Rosenstock J. New forms of insulin analogs in development: the quest for a better basal insulin. In: Umpierrez GE, editor. Therapy for Diabetes Mellitus and Related Disorders, 6th ed. American Diabetes Association, Alexandria, VA (2014). p. 480–507.
11. Black JE, Harris SB, Ryan BL, Zou G, Ratzki-Leewing A. Real-world effects of second-generation versus earlier intermediate/basal insulin analogues on rates of hypoglycemia in adults with type 1 and 2 diabetes (iNPHORM, US). Diabetes Ther. (2023) 3:1–9. doi: 10.1007/s13300-023-01423-3
12. Becker RH, Dahmen R, Bergmann K, Lehmann A, Jax T, Heise T. New insulin glargine 300 units · mL provides a more even activity profile and prolonged glycemic control at steady state compared with insulin glargine 100 units · mL. Diabetes Care. (2015) 38:637–43. doi: 10.2337/dc14-0006
13. Jonassen I, Havelund S, Hoeg-Jensen T, Steensgaard DB, Wahlund PO, Ribel U. Design of the novel protraction mechanism of insulin degludec, an ultra-long-acting basal insulin. Pharm Res. (2012) 29:2104–14. doi: 10.1007/s11095-012-0739-z
14. Zinman B, Fulcher G, Rao PV. Insulin degludec, ultra-long-acting basal insulin, once a day or three times a week versus insulin glargine once a day in patients with type 2 diabetes: a 16-week, randomised, open-label, phase 2 trial. Lancet. (2011) 377:924–31. doi: 10.1016/S0140-6736(10)62305-7
15. Shimoda S, Sakamoto W, Hokamura A, Matsuo Y, Sekigami T, Ichimori S, et al. Comparison of the efficacy and safety of once-daily insulin degludec/insulin aspart (IDegAsp) and long-acting second-generation basal insulin (insulin degludec and insulin glargine 300 units/mL) in insulin-naïve Japanese adults with type 2 diabetes: a pilot, randomized, controlled study. Endocr J. (2019) 66:745–52. doi: 10.1507/endocrj.EJ19-0179
16. Yale JF, Aroda VR, Charbonnel B, Sinclair AJ, Trescoli C, Cahn A, et al. Glycaemic control and hypoglycaemia risk with insulin glargine 300 U/mL versus glargine 100 U/mL: A patient-level meta-analysis examining older and younger adults with type 2 diabetes. Diabetes Metab. (2020) 46:110–8. doi: 10.1016/j.diabet.2018.10.002
17. Ji L, Kang ES, Dong X, Li L, Yuan G, Shang S, et al. Efficacy and safety of insulin glargine 300 U/mL versus insulin glargine 100 U/mL in Asia Pacific insulin-naïve people with type 2 diabetes: The EDITION AP randomized controlled trial. Diabetes Obes Metab. (2020) 22:612–21. doi: 10.1111/dom.13936
18. Philis-Tsimikas A, Klonoff DC, Khunti K, Bajaj HS, Leiter LA, Hansen MV, et al. CONCLUDE Study Group. Risk of hypoglycaemia with insulin degludec versus insulin glargine U300 in insulin-treated patients with type 2 diabetes: the randomised, head-to-head CONCLUDE trial. Diabetologia. (2020) 63:698–710. doi: 10.1007/s00125-019-05080-9
19. Wheeler MD, Barrientos-Perez M, Lo FS, Liang B, Lunsford A, Thórisdóttir Ó, et al. A 26-week, randomized trial of insulin detemir versus NPH insulin in children and adolescents with type 2 diabetes (iDEAt2). Eur J Pediatr. (2018) 177:1497–503. doi: 10.1007/s00431-018-3205-z
20. Russell-Jones D, Gall MA, Niemeyer M, Diamant M, Del Prato S. Insulin degludec results in lower rates of nocturnal hypoglycaemia and fasting plasma glucose vs. insulin glargine: A meta-analysis of seven clinical trials. Nutr Metab Cardiovasc Dis. (2015) 25:898–905. doi: 10.1016/j.numecd.2015.06.005
21. Riley RD, Jackson D, Salanti G, Burke DL, Price M, Kirkham J, et al. Multivariate and network meta-analysis of multiple outcomes and multiple treatments: rationale, concepts, and examples. BMJ. (2017) 13:358. doi: 10.1136/bmj.j3932
22. Salanti G. Indirect and mixed-treatment comparison, network, or multiple-treatments meta-analysis: many names, many benefits, many concerns for the next generation evidence synthesis tool. Res Synth Methods. (2012) 3:80–97. doi: 10.1002/jrsm.1037
23. Hutton B, Salanti G, Caldwell DM, Chaimani A, Schmid CH, Cameron C, et al. The PRISMA extension statement for reporting of systematic reviews incorporating network meta-analyses of health care interventions: checklist and explanations. Ann Intern Med. (2015) 162:777–84. doi: 10.7326/M14-2385
24. Bode BW, Chaykin LB, Sussman AM, Warren ML, Niemeyer M, Rabøl R, et al. Efficacy and safety of insulin degludec 200 U/mL and insulin degludec 100 U/mL in patients with type 2 diabetes (Begin: Compare). Endocr Pract. (2014) 20:785–91. doi: 10.4158/EP13411.OR
25. Lilly ends basal insulin peglispro development program. PR Newswire, USA. (2015). Available at: www.prnewswire.com/newsreleases/lilly-ends-basal-insulin-peglispro-development-program300188076.html.
26. Sterne JA, Savović J, Page MJ, Elbers RG, Blencowe NS, Boutron I, et al. RoB 2: a revised tool for assessing risk of bias in randomised trials. BMJ. (2019) 28:366. doi: 10.1136/bmj.l4898
27. Chaimani A, Higgins JP, Mavridis D, Spyridonos P, Salanti G. Graphical tools for network meta-analysis in STATA. PloS One. (2013) 3; 8:e76654. doi: 10.1371/journal.pone.0076654
28. White IR, Barrett JK, Jackson D, Higgins JP. Consistency and inconsistency in network meta-analysis: model estimation using multivariate meta-regression. Res Synth Methods. (2012) 3:111–25. doi: 10.1002/jrsm.1045
29. Veroniki AA, Vasiliadis HS, Higgins JP, Salanti G. Evaluation of inconsistency in networks of interventions. Int J Epidemiol. (2013) 42:332–45. doi: 10.1093/ije/dys222
30. Yu-Kang T. Node-Splitting generalized linear mixed models for evaluation of inconsistency in network meta- analysis. Value Health. (2016) 19:957–63. doi: 10.1016/j.jval.2016.07.005
31. Salanti G, Ades AE, Ioannidis JP. Graphical methods and numerical summaries for presenting results from multiple-treatment meta-analysis: an overview and tutorial. J Clin Epidemiol. (2011) 64:163–71. doi: 10.1016/j.jclinepi.2010.03.016
32. Puhan MA, Schünemann HJ, Murad MH, Li T, Brignardello-Petersen R, Singh JA, et al. A GRADE Working Group approach for rating the quality of treatment effect estimates from network meta-analysis. BMJ. (2014) 349:g5630. doi: 10.1136/bmj.g5630
33. Brignardello-Petersen R, Mustafa RA, Siemieniuk RA, Murad MH, Agoritsas T, Izcovich A, et al. GRADE approach to rate the certainty from a network meta-analysis: addressing incoherence. J Clin Epidemiol. (2019) 108:77–85. doi: 10.1016/j.jclinepi.2018.11.025
34. Brignardello-Petersen R, Guyatt GH, Mustafa RA, Chu DK, Hultcrantz M, Schünemann HJ, et al. GRADE guidelines 33: addressing imprecision in a network meta-analysis. J Clin Epidemiol. (2021) 139:49–56. doi: 10.1016/j.jclinepi.2021.07.011
35. Yepes-Nuñez JJ, Li SA, Guyatt G, Jack SM, Brozek JL, Beyene J, et al. Development of the summary of findings table for network meta-analysis. J Clin Epidemiol. (2019) 115:1–3. doi: 10.1016/j.jclinepi.2019.04.018
36. Brignardello-Petersen R, Florez ID, Izcovich A, Santesso N, Hazlewood G, Alhazanni W, et al. GRADE approach to drawing conclusions from a network meta-analysis using a minimally contextualised framework. BMJ. (2020) 371:m3900. doi: 10.1136/bmj.m3900
37. Zeng L, Brignardello-Petersen R, Hultcrantz M, Mustafa RA, Murad MH, Iorio A, et al. GRADE Guidelines 34: Updated GRADE guidance for imprecision rating using a minimally contextualized approach. J Clin Epidemiol. (2022) 150:216–24. doi: 10.1016/j.jclinepi.2022.07.014
38. Ling J, Poon EW, Yang A, Yeung T, Loo K, Ozaki R, et al. Glycemic variability and time in range during self-titration of once daily insulin glargine 300 U/ml versus neutral protamine hagedorn insulin in insulin-naïve Chinese type 2 diabetes patients. Diabetes Ther. (2021) 12:1399–413. doi: 10.1007/s13300-021-01046-6
39. Goldenberg RM, Aroda VR, Billings LK, Christiansen AS, Meller Donatsky A, Parvaresh Rizi E, et al. Effect of insulin degludec versus insulin glargine U100 on time in range: SWITCH PRO, a crossover study of basal insulin-treated adults with type 2 diabetes and risk factors for hypoglycaemia. Diabetes Obes Metab. (2021) 23:2572–81. doi: 10.1111/dom.14504
40. Rosenstock J, Cheng A, Ritzel R, Bosnyak Z, Devisme C, Cali AM, et al. More similarities than differences testing insulin glargine 300 units/mL versus insulin degludec 100 units/mL in insulin-naive type 2 diabetes: the randomized head-to-head BRIGHT trial. Diabetes Care. (2018) 41:2147–54. doi: 10.2337/dc18-0559
41. Ritzel R, Harris SB, Baron H, Florez H, Roussel R, Espinasse M, et al. A randomized controlled trial comparing efficacy and safety of insulin glargine 300 units/mL versus 100 units/mL in older people with type 2 diabetes: results from the SENIOR study. Diabetes Care. (2018) 41:1672–80. doi: 10.2337/dc18-0168
42. Wysham C, Bhargava A, Chaykin L, de la Rosa R, Handelsman Y, Troelsen LN, et al. Effect of insulin degludec vs insulin glargine U100 on hypoglycemia in patients with type 2 diabetes: the SWITCH 2 randomized clinical trial. Jama. (2017) 318:45–56. doi: 10.1001/jama.2017.7117
43. Terauchi Y, Koyama M, Cheng X, Sumi M, Riddle MC, Bolli GB, et al. Glycaemic control and hypoglycaemia with insulin glargine 300 U/mL compared with glargine 100 U/mL in Japanese adults with type 2 diabetes using basal insulin plus oral anti-hyperglycaemic drugs (EDITION JP 2 randomised 12-month trial including 6-month extension). Diabetes Metab. (2017) 43:446–52. doi: 10.1016/j.diabet.2017.03.001
44. Bolli GB, Riddle MC, Bergenstal RM, Wardecki M, Goyeau H, Home PD. Glycaemic control and hypoglycaemia with insulin glargine 300 U/mL versus insulin glargine 100 U/mL in insulin-naïve people with type 2 diabetes: 12-month results from the EDITION 3 trial. Diabetes Metab. (2017) 43:351–8. doi: 10.1016/j.diabet.2017.04.007
45. Aso Y, Suzuki K, Chiba Y, Sato M, Fujita N, Takada Y, et al. Effect of insulin degludec versus insulin glargine on glycemic control and daily fasting blood glucose variability in insulin-naïve Japanese patients with type 2 diabetes: I’D GOT trial. Diabetes Res Clin Pract. (2017) 130:237–43. doi: 10.1016/j.diabres.2017.06.007
46. Pan C, Gross JL, Yang W, Lv X, Sun L, Hansen CT, et al. A multinational, randomized, open-label, treat-to-target trial comparing insulin degludec and insulin glargine in insulin-naive patients with type 2 diabetes mellitus. Drugs R&D. (2016) 16:239–49. doi: 10.1007/s40268-016-0134-z
47. Yki-Järvinen H, Bergenstal RM, Bolli GB, Ziemen M, Wardecki M, Muehlen-Bartmer I, et al. Glycaemic control and hypoglycaemia with new insulin glargine 300 U/ml versus insulin glargine 100 U/ml in people with type 2 diabetes using basal insulin and oral antihyperglycaemic drugs: the EDITION 2 randomized 12-month trial including 6-month extension. Diabetes Obes Metab. (2015) 17:1142–9. doi: 10.1111/dom.12532
48. Riddle MC, Yki-Järvinen H, Bolli GB, Ziemen M, Muehlen-Bartmer I, Cissokho S, et al. One-year sustained glycaemic control and less hypoglycaemia with new insulin glargine 300 U/ml compared with 100 U/ml in people with type 2 diabetes using basal plus meal-time insulin: the EDITION 1 12-month randomized trial, including 6-month extension. Diabetes Obes Metab. (2015) 17:835–42. doi: 10.1111/dom.12472
49. Home PD, Bolli GB, Mathieu C, Deerochanawong C, Landgraf W, Candelas C, et al. Modulation of insulin dose titration using a hypoglycaemia-sensitive algorithm: insulin glargine versus neutral protamine Hagedorn insulin in insulin-naïve people with type 2 diabetes. Diabetes Obes Metab. (2015) 17:15–22. doi: 10.1111/dom.12329
50. Rosenstock J, Fonseca V, Schinzel S, Dain MP, Mullins P, Riddle M. Reduced risk of hypoglycemia with once-daily glargine versus twice-daily NPH and number needed to harm with NPH to demonstrate the risk of one additional hypoglycemic event in type 2 diabetes: evidence from a long-term controlled trial. J Diabetes Complicat. (2014) 28:742–9. doi: 10.1016/j.jdiacomp.2014.04.003
51. Bode B, Chaykin L, Sussman A, Warren M, Niemeyer M, Rabøl R, et al. Efficacy and safety of insulin degludec 200 U/ml and insulin degludec 100 U/ml in patients with Type 2 diabetes (Begin: Compare). Endocr Pract. (2014) 20:785–91. doi: 10.4158/EP13411.OR
52. Arakaki RF, Blevins TC, Wise JK, Liljenquist DR, Jiang HH, Jacobson JG, et al. Comparison of insulin lispro protamine suspension versus insulin glargine once daily added to oral antihyperglycaemic medications and exenatide in type 2 diabetes: a prospective randomized open-label trial. Diabetes Obes Metab. (2014) 16:510–8. doi: 10.1111/dom.12242
53. Zinman B, DeVries JH, Bode B, Russell-Jones D, Leiter LA, Moses A, et al. Efficacy and safety of insulin degludec three times a week versus insulin glargine once a day in insulin-naive patients with type 2 diabetes: results of two phase 3, 26 week, randomised, open-label, treat-to-target, non-inferiority trials. Lancet Diabetes Endocrinol. (2013) 1:123–31. doi: 10.1016/S2213-8587(13)70013-5
54. Rodbard HW, Cariou B, Zinman B, Handelsman Y, Philis-Tsimikas A, Skjøth TV, et al. Comparison of insulin degludec with insulin glargine in insulin-naive subjects with Type 2 diabetes: a 2-year randomized, treat-to-target trial. Diabetic Med. (2013) 30:1298–304. doi: 10.1111/dme.12303
55. Onishi Y, Iwamoto Y, Yoo SJ, Clauson P, Tamer SC, Park S. Insulin degludec compared with insulin glargine in insulin-naïve patients with type 2 diabetes: A 26-week, randomized, controlled, P an-A sian, treat-to-target trial. J Diabetes Invest. (2013) 4:605–12. doi: 10.1111/jdi.12102
56. Meneghini L, Kesavadev J, Demissie M, Nazeri A, Hollander P. Once-daily initiation of basal insulin as add-on to metformin: a 26-week, randomized, treat-to-target trial comparing insulin detemir with insulin glargine in patients with type 2 diabetes. Diabetes Obes Metab. (2013) 15:729–36. doi: 10.1111/dom.12083
57. Meneghini L, Atkin SL, Gough SC, Raz I, Blonde L, Shestakova M, et al. The efficacy and safety of insulin degludec given in variable once-daily dosing intervals compared with insulin glargine and insulin degludec dosed at the same time daily: a 26-week, randomized, open-label, parallel-group, treat-to-target trial in individuals with type 2 diabetes. Diabetes Care. (2013) 36:858–64. doi: 10.2337/dc12-1668
58. Gough SC, Bhargava A, Jain R, Mersebach H, Rasmussen S, Bergenstal RM. Low-volume insulin degludec 200 units/ml once daily improves glycemic control similarly to insulin glargine with a low risk of hypoglycemia in insulin-naive patients with type 2 diabetes: a 26-week, randomized, controlled, multinational, treat-to-target trial: the BEGIN LOW VOLUME trial. Diabetes Care. (2013) 36:2536–42. doi: 10.2337/dc12-2329
59. Garber AJ, King AB, Del Prato S, Sreenan S, Balci MK, Muñoz-Torres M, et al. NN1250-3582 (BEGIN BB T2D) Trial Investigators. Insulin degludec, an ultra-longacting basal insulin, versus insulin glargine in basal-bolus treatment with mealtime insulin aspart in type 2 diabetes (BEGIN Basal-Bolus Type 2): a phase 3, randomised, open-label, treat-to-target non-inferiority trial. Lancet. (2012) 379:1498–507. doi: 10.1016/S0140-6736(12)60205-0
60. Koivisto V, Cleall S, Pontiroli AE, Giugliano D. Comparison of insulin lispro protamine suspension versus insulin glargine once daily in basal-bolus therapies with insulin lispro in type 2 diabetes patients: a prospective randomized open-label trial. Diabetes Obes Metab. (2011) 13:1149–57. doi: 10.1111/j.1463-1326.2011.01484.x
61. Fadini GP, De Kreutzenberg SV, Mariano V, Boscaro E, Bertolini F, Mancuso P, et al. Optimized glycaemic control achieved with add-on basal insulin therapy improves indexes of endothelial damage and regeneration in type 2 diabetic patients with macroangiopathy: a randomized crossover trial comparing detemir versus glargine. Diabetes Obes Metab. (2011) 13:718–25. doi: 10.1111/j.1463-1326.2011.01396.x
62. Swinnen SG, Dain MP, Aronson R, Davies M, Gerstein HC, Pfeiffer AF, et al. A 24-week, randomized, treat-to-target trial comparing initiation of insulin glargine once-daily with insulin detemir twice-daily in patients with type 2 diabetes inadequately controlled on oral glucose-lowering drugs. Diabetes Care. (2010) 33:1176–8. doi: 10.2337/dc09-2294
63. Strojek K, Shi C, Carey MA, Jacober SJ. Addition of insulin lispro protamine suspension or insulin glargine to oral type 2 diabetes regimens: a randomized trial. Diabetes Obes Metab. (2010) 12:916–22. doi: 10.1111/j.1463-1326.2010.01257.x
64. Fogelfeld L, Dharmalingam M, Robling K, Jones C, Swanson D, Jacober S. A randomized, treat-to-target trial comparing insulin lispro protamine suspension and insulin detemir in insulin-naive patients with Type 2 diabetes. Diabetic Med. (2010) 27:181–8. doi: 10.1111/j.1464-5491.2009.02899.x
65. Raskin P, Gylvin T, Weng W, Chaykin L. Comparison of insulin detemir and insulin glargine using a basal-bolus regimen in a randomized, controlled clinical study in patients with type 2 diabetes. Diabetes/Metab Res Rev. (2009) 25:542–8. doi: 10.1002/dmrr.989
66. Rosenstock J, Davies M, Home PD, Larsen J, Koenen C, Schernthaner G. A randomised, 52-week, treat-to-target trial comparing insulin detemir with insulin glargine when administered as add-on to glucose-lowering drugs in insulin-naive people with type 2 diabetes. Diabetologia. (2008) 51:408–16. doi: 10.1007/s00125-007-0911-x
67. Hollander P, Cooper J, Bregnhøj J, Pedersen CB. A 52-week, multinational, open-label, parallel-group, noninferiority, treat-to-target trial comparing insulin detemir with insulin glargine in a basal-bolus regimen with mealtime insulin aspart in patients with type 2 diabetes. Clin Ther. (2008) 30:1976–87. doi: 10.1016/j.clinthera.2008.11.001
68. Esposito K, Ciotola M, Maiorino MI, Gualdiero R, Schisano B, Ceriello A, et al. Addition of neutral protamine lispro insulin or insulin glargine to oral type 2 diabetes regimens for patients with suboptimal glycemic control: a randomized trial. Ann Internal Med. (2008) 149:531–9. doi: 10.7326/0003-4819-149-8-200810210-00005
69. Pan CY, Sinnassamy P, Chung KD, Kim KW. Insulin glargine versus NPH insulin therapy in Asian type 2 diabetes patients. Diabetes Res Clin Pract. (2007) 76:111–8. doi: 10.1016/j.diabres.2006.08.012
70. Yokoyama H, Tada J, Kamikawa F, Kanno S, Yokota Y, Kuramitsu M. Efficacy of conversion from bedtime NPH insulin to morning insulin glargine in type 2 diabetic patients on basal-prandial insulin therapy. Diabetes Res Clin Pract. (2006) 73:35–40. doi: 10.1016/j.diabres.2005.12.009
71. Yki-Järvinen H, Kauppinen-Mäkelin R, Tiikkainen M, Vähätalo M, Virtamo H, Nikkilä K, et al. Insulin glargine or NPH combined with metformin in type 2 diabetes: the LANMET study. Diabetologia. (2006) 49:442–51. doi: 10.1007/s00125-005-0132-0
72. Hermansen K, Davies M, Derezinski T, Martinez Ravn G, Clauson P, Home P, et al. A 26-week, randomized, parallel, treat-to-target trial comparing insulin detemir with NPH insulin as add-on therapy to oral glucose-lowering drugs in insulin-naive people with type 2 diabetes. Diabetes Care. (2006) 29:1269–74. doi: 10.2337/dc05-1365
73. Eliaschewitz FG, Calvo C, Valbuena H, Ruiz M, Aschner P, Villena J, et al. Therapy in type 2 diabetes: insulin glargine vs. NPH insulin both in combination with glimepiride. Arch Med Res. (2006) 37:495–501. doi: 10.1016/j.arcmed.2005.10.015
74. Haak T, Tiengo A, Draeger E, Suntum M, Waldhäusl W. Lower within-subject variability of fasting blood glucose and reduced weight gain with insulin detemir compared to NPH insulin in patients with type 2 diabetes. Diabetes Obes Metab. (2005) 7:56–64. doi: 10.1111/j.1463-1326.2004.00373.x
75. Riddle MC, Rosenstock J, Gerich J, Insulin Glargine 4002 Study Investigators. The treat-to-target trial: randomized addition of glargine or human NPH insulin to oral therapy of type 2 diabetic patients. Diabetes Care. (2003) 26:3080–6. doi: 10.2337/diacare.26.11.3080
76. Benedetti MM, Humburg E, Dressler A, Ziemen M, null for the 3002 Study Group. A one-year, randomised, multicentre trial comparing insulin glargine with NPH insulin in combination with oral agents in patients with type 2 diabetes. Hormone Metab Res. (2003) 35:189–96. doi: 10.1055/s-2003-39080
77. Fritsche A, Schweitzer MA, Hring HU, 4001 Study Group. Glimepiride combined with morning insulin glargine, bedtime neutral protamine hagedorn insulin, or bedtime insulin glargine in patients with type 2 diabetes: a randomized, controlled trial. Ann Internal Med. (2003) 138:952–9. doi: 10.7326/0003-4819-138-12-200306170-00006
78. Yki-Järvinen HA, Dressler A, Ziemen M, HOE 901/300s Study Group. Less nocturnal hypoglycemia and better post-dinner glucose control with bedtime insulin glargine compared with bedtime NPH insulin during insulin combination therapy in type 2 diabetes. HOE 901/3002 Study Group. Diabetes Care. (2000) 23:1130–6. doi: 10.2337/diacare.23.8.1130
79. Elbourne DR, Altman DG, Higgins JP, Curtin F, Worthington HV, Vail A. Meta-analyses involving cross-over trials: methodological issues. Int J Epidemiol. (2002) 31:140–9. doi: 10.1093/ije/31.1.140
80. Ismail-Beigi F, Moghissi E, Tiktin M, Hirsch IB, Inzucchi SE, Genuth S. Individualizing glycemic targets in type 2 diabetes mellitus: implications of recent clinical trials. Ann Internal Med. (2011) 154:554–9. doi: 10.7326/0003-4819-154-8-201104190-00007
81. Inzucchi SE, Bergenstal RM, Buse JB, Diamant M, Ferrannini E, Nauck M, et al. Management of hyperglycaemia in type 2 diabetes: a patient-centered approach. Position statement of the American Diabetes Association (ADA) and the European Association for the Study of Diabetes (EASD). Diabetologia. (2019) 55:1577–96. doi: 10.1007/s00125-012-2534-0
82. MaChado-Alba JE, MaChado-Duque ME, Moreno-Gutierrez PA. Time to and factors associated with insulin initiation in patients with type 2 diabetes mellitus. Diabetes Res Clin Pract. (2015) 107:332–7. doi: 10.1016/j.diabres.2015.01.018
83. Type 2 diabetes in adults: management. London: National Institute for Health and Care Excellence (NICE (2022). Available at: https://www.ncbi.nlm.nih.gov/books/NBK553486/. NICE Guideline, No. 28.
84. Inzucchi SE, Bergenstal RM, Buse JB, Diamant M, Ferrannini E, Nauck M, et al. Management of hyperglycemia in type 2 diabetes, 2015: a patient-centered approach: update to a position statement of the American Diabetes Association and the European Association for the Study of Diabetes. Diabetes Care. (2015) 38:140–9. doi: 10.2337/dc14-2441
85. Marso SP, McGuire DK, Zinman B, Poulter NR, Emerson SS, Pieber TR, et al. Efficacy and safety of degludecversusglargineintype2diabetes. N Engl J Med. (2017) 377:72332. doi: 10.1056/NEJMoa1615692
86. ORIGIN Trial Investigators. Cardiovascular and other outcomes postintervention with insulin glargine and omega-3 fatty acids (ORIGINALE). Diabetes Care. (2016) 39:709–16. doi: 10.2337/dc15-1676
87. Gerstein HC, Bosch J, Dagenais GR, Dı´az R, Jung H, Maggioni AP, et al. ORIGIN Trial Investigators. Basal insulin and cardiovascular and other outcomes in dysglycemia. N Engl J Med. (2012) 367:31928. doi: 10.1056/NEJMoa1203858
88. Rados DV, Falcetta MR, Pinto LC, Leitao CB, Gross JL. All-cause mortality and cardiovascular safety of basal insulin treatment in patients with type 2 diabetes mellitus: a systematic review with meta-analysis and trial sequential analysis. Diabetes Res Clin Pract. (2021) 173:108688. doi: 10.1016/j.diabres.2021.108688
89. Jenkins D, Czachorowski M, Bujkiewicz S, Dequen P, Jonsson P, Abrams KR. Evaluation of methods for the inclusion of real-world evidence in network meta-analysis - A case study in multiple sclerosis. Value Health. (2014) 17:A576. doi: 10.1016/j.jval.2014.08.1941
90. Efthimiou O, Mavridis D, Debray TP, Samara M, Belger M, Siontis GC, et al. GetReal Work Package 4. Combining randomized and non-randomized evidence in network meta-analysis. Stat Med. (2017) 36:1210–26. doi: 10.1002/sim.7223
91. Polonsky WH, Henry RR. Poor medication adherence in type 2 diabetes: recognizing the scope of the problem and its key contributors. Patient Prefer Adherence. (2016) 10:1299–307. doi: 10.2147/PPA
92. Garcia-Perez LE, Alvarez M, Dilla T, Gil-Guillen V, Orozco-Beltran D. Adherence to therapies in patients with type 2 diabetes. Diabetes Ther. (2013) 12:175–94. doi: 10.1007/s13300-013-0034-y
93. Davies MJ, Gagliardino JJ, Gray LJ, Khunti K, Mohan V, Hughes R. Real-world factors affecting adherence to insulin therapy in patients with Type 1 or Type 2 diabetes mellitus: a systematic review. Diabetes Med. (2013) 30:512–24, 5. doi: 10.1111/dme.12128
94. Madenidou AV, Paschos P, Karagiannis T, Katsoula A, Athanasiadou E, Kitsios K, et al. Comparative benefits and harms of basal insulin analogues for type 2 diabetes: a systematic review and network meta-analysis. Ann Intern Med. (2018) 169:165–74. doi: 10.7326/M18-0443
95. Rosenstock J, Hollander P, Bhargava A, Ilag LL, Pollom RK, Zielonka JS, et al. Similar efficacy and safety of LY2963016 insulin glargine and insulin glargine (Lantus®) in patients with type 2 diabetes who were insulin-naı¨ve or previously treated with insulin glargine: a randomized, double-blind controlled trial (the ELEMENT 2 study). Diabetes Obes Metab. (2015) 17:734–41. doi: 10.1111/dom.12482
96. Freemantle N, Chou E, Frois C, Zhuo D, Lehmacher W, Vlajnic A, et al. Safety and efficacy of insulin glargine 300 u/mL compared with other basal insulin therapies in patients with type 2 diabetes mellitus: a network meta-analysis. BMJ Open. (2016) 6:e009421. doi: 10.1136/bmjopen-2015-009421
Keywords: basal insulin, blood glucose, body weight, diabetes treatment, hypoglycemia, network meta-analysis
Citation: Dehghani M, Sadeghi M, Barzkar F, Maghsoomi Z, Janani L, Motevalian SA, Loke YK, Ismail-Beigi F, Baradaran HR and Khamseh ME (2024) Efficacy and safety of basal insulins in people with type 2 diabetes mellitus: a systematic review and network meta-analysis of randomized clinical trials. Front. Endocrinol. 15:1286827. doi: 10.3389/fendo.2024.1286827
Received: 31 August 2023; Accepted: 22 February 2024;
Published: 21 March 2024.
Edited by:
Qi Pan, Peking University, ChinaReviewed by:
Sudhanshu Kumar Bharti, Patna University, IndiaCopyright © 2024 Dehghani, Sadeghi, Barzkar, Maghsoomi, Janani, Motevalian, Loke, Ismail-Beigi, Baradaran and Khamseh. This is an open-access article distributed under the terms of the Creative Commons Attribution License (CC BY). The use, distribution or reproduction in other forums is permitted, provided the original author(s) and the copyright owner(s) are credited and that the original publication in this journal is cited, in accordance with accepted academic practice. No use, distribution or reproduction is permitted which does not comply with these terms.
*Correspondence: Hamid Reza Baradaran, YmFyYWRhcmFuLmhyQGl1bXMuYWMuaXI=; Mohammad E. Khamseh, a2hhbXNlaC5tQGl1bXMuYWMuaXI=
†These authors have contributed equally to this work and share first authorship
Disclaimer: All claims expressed in this article are solely those of the authors and do not necessarily represent those of their affiliated organizations, or those of the publisher, the editors and the reviewers. Any product that may be evaluated in this article or claim that may be made by its manufacturer is not guaranteed or endorsed by the publisher.
Research integrity at Frontiers
Learn more about the work of our research integrity team to safeguard the quality of each article we publish.