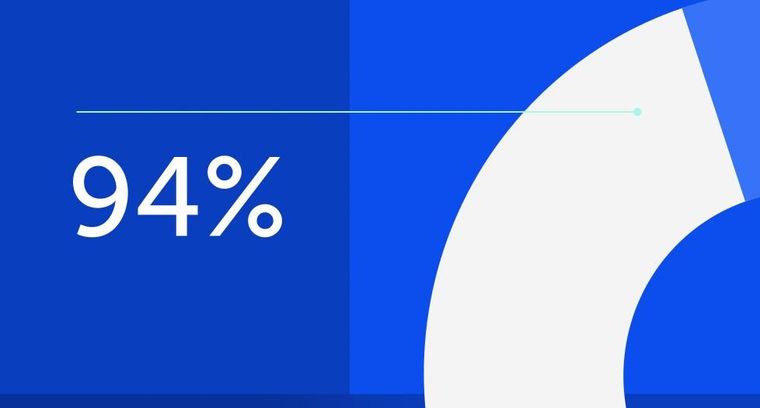
94% of researchers rate our articles as excellent or good
Learn more about the work of our research integrity team to safeguard the quality of each article we publish.
Find out more
ORIGINAL RESEARCH article
Front. Endocrinol., 08 February 2024
Sec. Renal Endocrinology
Volume 15 - 2024 | https://doi.org/10.3389/fendo.2024.1285872
Aims: Previous Mendelian randomization (MR) of obesity and diabetic nephropathy (DN) risk used small sample sizes or focused on a single adiposity metric. We explored the independent causal connection between obesity-related factors and DN risk using the most extensive GWAS summary data available, considering the distribution of adiposity across childhood and adulthood.
Methods: To evaluate the overall effect of each obesity-related exposure on DN (Ncase = 3,676, Ncontrol = 283,456), a two-sample univariate MR (UVMR) analysis was performed. The independent causal influence of each obesity-related feature on DN was estimated using multivariable MR (MVMR) when accounting for confounding variables. It was also used to examine the independent effects of adult and pediatric obesity, adjusting for their interrelationships. We used data from genome-wide association studies, including overall general (body mass index, BMI) and abdominal obesity (waist-to-hip ratio with and without adjustment for BMI, i.e., WHR and WHRadjBMI), along with childhood obesity (childhood BMI).
Results: UVMR revealed a significant association between adult BMI (OR=1.24, 95%CI=1.03-1.49, P=2.06×10-2) and pediatric BMI (OR=1.97, 95%CI=1.59-2.45, P=8.55×10-10) with DN risk. At the same time, adult WHR showed a marginally significant increase in DN (OR =1.27, 95%CI = 1.01-1.60, P=3.80×10-2). However, the outcomes were adverse when the influence of BMI was taken out of the WHR (WHRadjBMI). After adjusting for childhood BMI, the causal effects of adult BMI and adult abdominal obesity (WHR) on DN were significantly attenuated and became nonsignificant in MVMR models. In contrast, childhood BMI had a constant and robust independent effect on DN risk(adjusted for adult BMI: IVW, OR=1.90, 95% CI=1.60-2.25, P=2.03×10-13; LASSO, OR=1.91, 95% CI=1.65-2.21, P=3.80×10-18; adjusted for adult WHR: IVW, OR=1.80, 95% CI=1.40-2.31, P=4.20×10-6; LASSO, OR=1.90, 95% CI=1.56-2.32, P=2.76×10-10).
Interpretation: Our comprehensive analysis illustrated the hazard effect of obesity-related exposures for DN. In addition, we showed that childhood obesity plays a separate function in influencing the risk of DN and that the adverse effects of adult obesity (adult BMI and adult WHR) can be substantially attributed to it. Thus, several obesity-related traits deserve more attention and may become a new target for the prevention and treatment of DN and warrant further clinical investigation, especially in childhood obesity.
Diabetic nephropathy (DN), a severe microvascular complication associated with diabetes, is characterized by a decline in estimated glomerular filtration rate (eGFR) or the presence of albuminuria. DN is widely recognized as the primary factor behind end-stage renal disease (ESRD) globally (1), exacerbating the occurrence and progression of cardiovascular disease and mortality (2). Around 30% to 40% of people with diabetes will eventually develop DN (3). Furthermore, the financial load of DN and ESKD is immense (4). Therefore, it is vital to identify the potential causative factor for the prevention and management of DN.
It is well-known that obesity poses a unique risk for the onset and progression of DN (5). The main subtypes of obesity are general obesity and abdominal obesity. Consistent findings from conventional epidemiologic research have consistently shown an increased likelihood of DN associated with adult BMI (a measure of overall adiposity in adults). In contrast, the results for adult WHR (a measure of abdominal adiposity in adults) have been inconclusive (6–8).
In addition, while childhood obesity has been a concern for decades, it is now an undeniable public health crisis (9), with evidence from observational studies linking early-life obesity to a greater risk of chronic conditions such as type 2 diabetic mellitus (10–13). DN has a more extended latency period, and obese children are more likely than children with a standard body mass index (BMI) to develop end-stage renal disease (ESRD) or chronic kidney disease (CKD) (14). Hence, it is reasonable to suggest that the early stages of life, including childhood and adolescence, play a significant role in shaping adults’ susceptibility to DN through adiposity. Because of the inconsistent findings in observational research regarding the link between abdominal obesity and DN, as well as the lack of sufficient evidence regarding the connection between early-life adiposity and the risk of DN. Due to the complexity of these relationships, it is essential to understand how different obesity-related characteristics interact to increase the risk of DN.
Mendelian randomization (MR) employs genetic variations in the reproductive cell line as substitutes to enable causal deduction between a specific exposure and a result (15). Due to the random distribution and stable characteristics of genetic variations, In comparison to conventional observational analysis, MR analyses are anticipated to be less susceptible to conventional confounding and reverse causality (16).
Furthermore, multivariable MR enables the evaluation of distinct impacts of various exposures (e.g., childhood and adult BMI) on health outcomes (17–19). Univariable MR can estimate the general impact of body size in early life on DN (20, 21). However, independent of adult body size, multivariable MR can assess the precise impact of childhood obesity on DN risk. This approach has recently been used to investigate whether body size in childhood influences the risk of developing diseases like breast and colorectal cancer later in life. It also explores if adult body size impacts this influence (22, 23).
There have been limited publications on the relationship between obesity and DN using Mendelian randomization due to the absence of genome-wide association study (GWAS) data on DN in type 2 diabetes. Only one obesity-related measure, BMI, has been evaluated for a causal relationship with DN (24). In addition, MR studies of abdominal obesity and childhood obesity remain empty.
The intrinsic link between obesity and DN has been better understood because of earlier MR investigations, but there are still a lot of unanswered questions. Furthermore, due to the increasing sample size of genome-wide association studies (GWAS) and the continuous accumulation of data, it is crucial to thoroughly research the relationship between obesity and DN utilizing an MR approach.
In this study, we utilized an extensively expanded collection of IVs derived from the most comprehensive exposure and outcome GWAS conducted to date (25) to (1) assess the overall influence of characteristics related to obesity (general and abdominal obesity, adult and childhood obesity) on DN; (2) Determine the separate causal influence of each characteristic related to obesity while accounting for the confounding influences of glycemic traits, hypertension, and three other noteworthy risk factors; (3) To assess the separate influence of obesity in adulthood and during childhood on DN, while considering their correlation.
The Genetic Investigation of Anthropometric Traits (GIANT) consortium and the UK Biobank (UKBB) worked together to conduct the largest GWAS study of adults with general body obesity (BMI) and abdominal obesity (WHR and WHRadjBMI) in 2019. It included an estimated 700,000 people of European ancestry (25). Standard techniques were used to measure anthropometric information such as height, weight, waist, and hip circumference. By dividing the circumferences of the hips and the waist, the Waist-to-Hip Ratio (WHR) was calculated. In contrast, Body Mass Index (BMI) was computed by dividing weight by the square of height. Regressing WHR on BMI and adding BMI as a second independent variable led to the creation of WHRadjBMI. The most recent and comprehensive genome-wide association study (GWAS) on childhood BMI was carried out in 2020 by the Early Growth Genetics (EGG) consortium (26). This study included a combined dataset from 41 studies, comprising 39,620 children between the ages of 6 and 10, all of European ancestry.
For DN, we defined the outcome as the presence of glomerular damage in patients with diabetes mellitus based on the ICD-10 criterion (code: N08.3*). To obtain the necessary summary statistics, we used data from the FinnGen biobank, which included 3,676 cases and 283,456 controls of European ancestry (27).
Our MR study now includes five key risk factors: fasting insulin, Homeostasis Model Assessment of Insulin Resistance (HOMA-IR), high blood pressure, circulating CRP levels, and smoking. This inclusion provides a more comprehensive perspective. The summary-level data for glycemic traits, specifically fasting insulin (N=153,525) and HOMA-IR (N=37,037), were sourced from the Meta-analyses of Glucose and Insulin-related Traits Consortium (MAGIC) (28, 29). We obtained the GWAS summary statistics from the IEU Open GWAS project download for high blood pressure, including 124,227 European cases and 337,653 European controls. We used information from a massive GWAS meta-analysis of 88 studies (involving 204,402 individuals) (30) to determine levels of circulating CRP. This GWAS meta-analysis identified 58 significant genetic loci across the entire genome for circulating CRP levels, accounting for up to 7.0% of the variation in circulating CRP levels (30). To analyze smoking, we utilized information released in 2019 by the GSCAN (GWAS & Sequencing Consortium of Alcohol and Nicotine), involving 1,232,091 individuals of European descent, explicitly focusing on smoking initiation (31).
We extracted IVs from exposed GWAS that reached genome-wide significance. A total of 85109 SNPs were shown to be independently associated with BMI, 39709 with WHR, 54367 with WHRadjBMI, and 1353 with pediatric BMI (all P value< 5×10-8).
After conducting the clumping process (R2<0.001, window size=10,000kb), the instrumental variables were disentangled from linkage disequilibrium (LD) to ensure their autonomy. Besides, to meet the exclusivity assumption in Mendelian Randomization, we rigorously excluded SNP data with strong associations (P<5×10-5) to diabetic nephropathy. This ensures the integrity of our instrumental variable selection. In addition, performing F-statistics to validate significant effects for all IV-SNPs (overall F-statistic value>10) was necessary for the validity of the results.
These SNPs were carefully matched up with the results of the GWAS for DN. See Supplementary Table 2 for details. We used the following equation to figure out the strength of the device (Table 1). The F-statistic of an instrument is considered to be strong enough if it is greater than 10 (32). R2 shows the phenotypic diversity that can be explained by genetic tools. These tools are based on raw GWAS data or can be calculated using the genetic association of an SNP with an exposure (β) and the minor allele frequency (MAF). The following equation was used to figure out R2.
To assess the potential causal relationship between obesity-related exposures (BMI, WHR, WHRadjBMI, childhood BMI) and DN, we conducted a comprehensive two-sample (MR) analysis. In Figure 1, a schematic diagram is shown to illustrate the method of MR analysis.
Figure 1 Overview of the MR design assumptions and study methodology. Assumption 1 states that the genetic variants proposed as instrumental variables should be strongly associated with the relevant risk factor; assumption 2 states that the genetic variants used should not be associated with potential confounders; and assumption 3 states that the selected genetic variants should only influence outcome risk through the relevant risk factor and not through other possible pathways. BMI, body mass index; WHR, waist-to-hip ratio; WHRadjBMI, waist-to-hip ratio adjusted for body mass index; UVMR, Univariable Mendelian randomization analysis; MVMR, Multivariable Mendelian randomization analysis.
We used UVMR as our primary analysis method to examine the overall impact of each obesity-related characteristic on DN. We first applied the inverse variance weighted (IVW) approach in a random effects model. Regressing the outcome coefficient on the exposure coefficient in this manner yields an estimate of the causal effect without including an intercept term (33). We supplemented the IVW analysis with MR-Egger regression to account for potential bias due to horizontal pleiotropy (34).
MR-Egger regression is similar to IVW but includes an intercept term in the regression model to capture the presence of directional pleiotropy, thereby providing insight into potential bias due to pleiotropic effects. In addition, we used the weighted median approach (35), which is known to be more robust to the inclusion of invalid instruments than IVW and MR-Egger regression.
Furthermore, after locating and eliminating all discovered outlying SNPs, we further employed MR-PRESSO to determine whether horizontal pleiotropy existed and to re-estimate the causal effect (36). By detecting and removing outlying SNPs, we aimed to obtain a more reliable causal effect estimate while accounting for potential horizontal pleiotropy.
We carried out various sensitivity analyses to ensure our results’ robustness. First, we conducted an analysis with IVs that excluded palindromic SNPs with strand ambiguity. The analysis was done to address potential issues related to ambiguous strand orientation that could introduce bias into the analysis. In order to determine if a single SNP had a significant impact on the MR estimate, we also conducted a leave-one-out study. In this analysis, we sequentially left out one SNP at a time and assessed the effect on the results. This approach helped us to identify potential sources of heterogeneity and assess the robustness of the MR estimate. In addition, we also performed Steiger filtering to ensure the directionality of the association between obesity and DN (37).
To investigate the independence of the causative effects of childhood and adult obesity on DN, we used a multivariable MR analysis. By adopting this approach, we were able to investigate whether the causal effects of childhood and adult obesity on diabetic nephropathy (DN) are distinct from each other. This provided vital insights into the development of DN in relation to obesity during different life stages.
We included childhood BMI as an additional component to each adult obesity trait (BMI, WHR, and WHRadjBMI) to account for the intercorrelation between adult and childhood obesity in order to assess the independent effects of each adult obesity characteristic on DN. To create composite instrumental variables (IVs), we used linkage disequilibrium clustering with an R2 threshold greater than 0.001 (38). Three sets of SNPs were used to create composite IVs: 510 SNPs for BMI and childhood BMI, 327 SNPs for WHR and childhood BMI, and 266 SNPs for WHRadjBMI and childhood BMI. These composite IVs allowed us to calculate the direct effect of childhood adiposity on DN while accounting for the effect of adult BMI or vice versa. In our multivariable MR analysis, we implemented IVW, MR-LASSO, and Weighted Median methods to address instrumental variable collinearity and integrated MR-PRESSO to control pleiotropic biases, thereby enhancing our study’s accuracy and interpretability.
In addition, to further explore the independent association between childhood obesity and DN, five risk factors (fasting insulin, HOMA-IR, hypertension, circulating CRP levels, and smoking) considered as important confounders were included. After taking into account the confounding factors, one at a time as well as concurrently to calculate the independent impact of childhood obesity on DN. Odds ratios (ORs) and their 95% confidence intervals (CIs) are used to present the results, which provide a relative risk estimate of DN produced by each increase in standard deviation (SD) of each obesity-related trait examined in this study. P-values were changed into q-values in our MR analysis to take the false discovery rate (FDR) in multiple testing into consideration. FDR-adjusted P-values less than 0.05 were used to define robust statistical significance, and crude P-values less than 0.05 and FDR-adjusted P-values greater than 0.05 were used to define marginal significance. All statistical analyses use R v4.0.0 and the “TwoSampleMR” package (39).
Employing UVMR, genetically predicted BMI showed a statistically significant association with an elevated risk of DN (OR=1.24, 95%CI=1.03-1.49, P=2.06×10-2), as shown in Figure 2, which also survived FDR correction. Adult WHR showed a marginally significant increase in DN (OR=1.27, 95%CI=1.01-1.60, P=3.80×10-2). When the effect of BMI was removed from WHR (WHRadjBMI), the results were negative. Regarding childhood BMI, there is convincing evidence that it has a statistically significant hazard effect on DN (OR=1.97, 95%CI= 1.59-2.45, P=8.55×10-10). The weighted median method and MR-Egger regression provided additional support for the results of IVW as mentioned above, with estimates that were all in the same general direction.
Figure 2 Estimation of the total effect of life course adiposity on the risk of DN using univariable Mendelian randomization. Boxes denote the point estimates of causal effects, and error bars denote 95% confidence intervals. Asterisks (※) denote statistical significance survived false discovery rate (FDR) correction (PFDR <0.05). BMI, body mass index; WHR, waist-to-hip ratio; WHRadjBMI, waist-to-hip ratio adjusted for body mass index; No. of SNP, number of instrumental variables; OR, odds ratio; 95%CI, 95% confidence interval.
In contrast to childhood BMI, the Q statistics of the SNP instruments for the other lifelong adiposity measures (BMI, WHR, WHRadjBMI) were all less than 0.05, indicating significant heterogeneity among the genetic instruments. To account for this heterogeneity and ensure our results’ robustness, we used a random effects model for these adiposity measures in the analysis.
In addition, MR-Egger regression analysis revealed an intercept centered at zero, indicating no strong evidence for substantial horizontal pleiotropy (MR-Egger intercept>0.05). This suggests that asymmetric pleiotropic effects were less likely to influence the causal estimates generated. However, the significant global test p-value 0.05 of the MR-PRESSO study demonstrated pleiotropy for adult BMI and WHR. Notably, the results remained statistically significant after outlier-corrected filtering, demonstrating the robustness of our findings even after accounting for potential pleiotropic effects.
The funnel plot of the life course adiposity instruments showed a proportional distribution of effect estimates, indicating no significant publication bias. Leave-one-out histogram analysis also failed to identify any specific SNP that had a disproportionate effect on the association between the risk of DN and the overall association. These results demonstrate that the reported associations are legitimate and robust and that the influence of the genetic tools, rather than any particular SNP, is responsible for the overall results. Furthermore, Steiger filtering did not reveal a causal effect of DN on any of the obesity exposures, further assuring directionality (Supplementary Table 2).
Although previous evidence has shown that both childhood and adult obesity are associated with an increased risk of DN, it is still uncertain whether their effects are independent of each other. To address this question, we performed a series of MVMR analyses (Table 2). Of note, the causal effects of adult BMI and adult abdominal obesity (WHR) on DN were significantly attenuated. They became nonsignificant in MVMR models after accounting for childhood BMI as a covariate. It suggests that the presence of childhood obesity influences the effect of adult general and abdominal obesity on DN. These results imply that childhood obesity may mediate the association between adult obesity and the risk of DN.
In contrast, a constant and robust independent effect of childhood BMI on the risk of DN was found even after controlling for each adult adiposity characteristic. The effect of childhood BMI was still substantial and high after adjusting for adult BMI[IVW, OR=1.90, 95% CI =1.60-2.25, P=2.03×10-13; LASSO, OR=1.91, 95% CI =1.65-2.21, P=3.80×10-18], the independent effect of childhood BMI on DN persisted after adjustment for adult WHR [IVW, OR=1.80, 95% CI=1.40-2.31, P=4.20×10-6; LASSO, OR=1.90, 95% CI =1.56-2.32, P=2.76×10-10]. These consistent findings across both IVW and MR-Lasso analyses, with p-values significantly less than 0.001, reinforce the independent and substantial impact of childhood BMI on the risk of DN. Furthermore, the implementation of MR-PRESSO, especially after outlier correction, highlighted significant differences, affirming that the exposure effects on DN outcomes are statistically meaningful and robust even when adjusting for pleiotropy. This underscores the reliability and strength of our conclusions.
Lastly, in our MVMR analysis, adjustments for confounders such as fasting insulin, HOMA-IR, high blood pressure, circulating CRP levels, and smoking were meticulously made. These adjustments are crucial for accurately assessing the link between childhood obesity and DN, considering the potential modifying effects of various risk factors. After controlling for covariates, the magnitude and direction of the impact of childhood obesity on DN were all sustained across repeated testing corrections (Figure 3).
Figure 3 After correcting for each confounder separately and applying MVMR, the effects of genetically predicted childhood obesity on the risk of DN are independent. The y-axis details the genetically predicted confounder(s) for which adjustment was made, and the x-axis details the ORs and 95%Cls per 1-standard deviation (SD) increase in exposure. Asterisks (※) denote statistical significance survived false discovery rate (FDR) correction (PFDR<0.05). BMI, body mass index; DN, Diabetic nephropathy; CRP, circulating CRP levels; IVW, Inverse-variance weighted approach; NO.SNP, number of instrumental variables; OR, odds ratio; 95%CI, 95% confidence interval.
Using data from the biggest GWAS(s) carried out to date for each variable, our MR investigation evaluated the causative involvement of numerous obesity-related features in the development of DN. We successfully identified the significant deleterious effects of genetically predicted adult BMI, adult WHR, and childhood BMI on DN. Integrating these obesity-related traits, we observed that the impact of adult BMI on DN was mainly mediated by childhood BMI, which also held true for adult WHR. On the contrary, when compared to adult measures, childhood BMI consistently exhibited a distinct and independent risk factor for DN.
Multiple studies have employed an MR method to identify links between genetic susceptibility to overall obesity and DN (24, 40). We additionally investigated the utilization of MVMR to regulate the impact of childhood BMI and discovered a moderation in the impact of adult BMI, indicating that the presumed causal association between adult BMI and DN is primarily due to elevated childhood BMI. When considering these traits collectively, these findings indicate an intricate interaction that underlies various obesity-related characteristics throughout one’s lifetime, emphasizing the significance of simultaneously taking these traits into account.
A meta-analysis of 14 cross-sectional studies indicated that abdominal obesity parameters were associated with increased odds of DN (6), While Man et al. (8) found that people with abdominal obesity did not have DN in T2DM. Despite the above discrepancies, there is no MR study on the association between abdominal obesity and DN.
Our UVMR revealed a significant detrimental effect of DN using an enlarged collection of IVs that included 330 WHR-associated SNPs. After accounting for childhood BMI, our MVMR also noticed that this protective impact of WHR practically vanished, suggesting that the established putative causality of adult WHR and DN is mainly due to high childhood BMI. There are several possible interpretations of this discrepancy: First, these studies were limited by their observational nature and lack of randomization, prospective design, and blinding. They primarily consisted of observational studies or meta-analyses based on observational data. The discrepancies in the results could be attributed to the inherent limitations of the non-randomized comparative study design itself (41). Second, the association between abdominal obesity and DN identified in observational studies may be due to insidious confounding variables. Despite adjusting for various confounders such as age and diabetes mellitus in numerous studies, there may still be underlying confounders that remain unaccounted for. Hence, further research is warranted to investigate the genetic link between DN and abdominal obesity.
Moreover, our research emphasizes the critical contribution of childhood BMI to DN. Based on the findings from our UVMR analysis, it was evident that childhood obesity posed a significant threat to the development of DN. Conversely, our MVMR results offered robust evidence supporting a direct link between childhood obesity and DN, which remained unaffected by adult metrics or other variables.
In recent times, multiple systematic reviews and meta-analyses have consistently demonstrated a significant association between high childhood BMI and an elevated risk of developing adult diabetes, with indications of its potential persistence into adulthood (42–44). Therefore, DN, one of the most common microvascular complications of diabetes, may have potential mechanisms, including long-term childhood obesity status, that directly affect renal function by altering intrarenal hemodynamics, causing oxidative stress, and increasing pro-inflammatory adipokines and cytokines (8), such as insulin resistance, hypertension, and impaired glucose and lipid metabolism, which damage the kidney. Unfortunately, there was not enough data from observational studies to prove the significant association between childhood obesity and DN; therefore, additional experimental studies are necessary to elucidate the precise molecular mechanism behind this discovery.
The current study has several advantages, as we utilized extensive MR to creatively evaluate the distinct impact of various interconnected obesity characteristics on DN. Notably, our findings indicate that childhood obesity has a detrimental effect on DN later in life. However, we also need to acknowledge several limitations. Initially, the presence of pleiotropy caused by undetected confounders may introduce bias to the causal estimates. Nevertheless, we made every effort to minimize such prejudice. We applied the MR-Steiger filtering method separately in UVMR to eliminate SNPs that might suggest reverse causality during the research process. Furthermore, we conducted MVMR analysis by incorporating exposure with confounders individually to prevent pleiotropy. In addition, due to the linear assumption underlying the two-sample MR approach, it was not possible for us to investigate the non-linear relationship between obesity and DN using GWAS summary statistics. Therefore, it may be necessary to conduct future one-sample MR studies utilizing semiparametric methods (45) to address this issue. Given the restricted sample size of DN, it may be necessary to have a more extensive GWAS database for DN to further confirm causality.
In conclusion, we have clarified the increasing correlation between obesity and the risk of DN. Additionally, we discovered that childhood obesity was substantially responsible for the overall impact of adult general obesity and adult abdominal obesity on DN. Finally, we showed that childhood BMI has a largely independent influence on DN, independent of adult measurements. Our findings highlight the importance of childhood obesity in the development of DN and underscore the need to consider the complex interactions that underlie related exposures. Further observational studies or pathophysiological mechanisms of childhood obesity are needed in the future to provide new targets for the prevention and treatment of DN.
The original contributions presented in the study are included in the article/Supplementary Material. Further inquiries can be directed to the corresponding author.
HZ: Writing – original draft, Writing – review & editing. QZ: Writing – review & editing. YS: Writing – review & editing. LW: Writing – review & editing. MC: Writing – review & editing. JB: Writing – review & editing. QY: Writing – review & editing.
The author(s) declare that no financial support was received for the research, authorship, and/or publication of this article.
The authors would like to express our gratitude to the coalition organizations mentioned in the data availability statement.
The authors declare that the research was conducted in the absence of any commercial or financial relationships that could be construed as a potential conflict of interest.
The reviewer YP declared a shared parent affiliation with the authors to the handling editor at the time of review.
All claims expressed in this article are solely those of the authors and do not necessarily represent those of their affiliated organizations, or those of the publisher, the editors and the reviewers. Any product that may be evaluated in this article, or claim that may be made by its manufacturer, is not guaranteed or endorsed by the publisher.
The Supplementary Material for this article can be found online at: https://www.frontiersin.org/articles/10.3389/fendo.2024.1285872/full#supplementary-material
1. Association, A.D. Nephropathy in diabetes. Diabetes Care (2004) 27(suppl_1):s79–83. doi: 10.2337/diacare.27.2007.S79
2. Barkoudah E, Skali H, Uno H, Solomon SD, Pfeffer MA. Mortality rates in trials of subjects with type 2 diabetes. J Am Heart Assoc (2012) 1(1):e000059. doi: 10.1161/JAHA.111.000059
3. Deshpande AD, Harris-Hayes M, Schootman M. Epidemiology of diabetes and diabetes-related complications. Phys Ther (2008) 88(11):1254–64. doi: 10.2522/ptj.20080020
4. Golestaneh L, Alvarez PJ, Reaven NL, Funk SE, McGaughey KJ, Romero A, et al. All-cause costs increase exponentially with increased chronic kidney disease stage. Am J Managed Care (2017) 23(10 Suppl):S163–72.
5. Wahba IM, Mak RH. Obesity and obesity-initiated metabolic syndrome: mechanistic links to chronic kidney disease. Clin J Am Soc Nephrol (2007) 2(3):550–62. doi: 10.2215/CJN.04071206
6. Zhao Q, Yi X, Wang Z. Meta-analysis of the relationship between abdominal obesity and diabetic kidney disease in type 2 diabetic patients. Obes Facts (2021) 14(4):338–45. doi: 10.1159/000516391
7. Kanakamani J, Ammini AC, Gupta N, Dwivedi SN. Prevalence of microalbuminuria among patients with type 2 diabetes mellitus—a hospital-based study from north India. Diabetes Technol Ther (2010) 12(2):161–6. doi: 10.1089/dia.2009.0133
8. Man REK, Gan ATL, Fenwick EK, Gupta P, Wong MYZ, Wong TY, et al. The relationship between generalized and abdominal obesity with diabetic kidney disease in type 2 diabetes: a multiethnic asian study and meta-analysis. Nutrients (2018) 10(11):1685. doi: 10.3390/nu10111685
9. Diabetes, T.L. Childhood obesity: a growing pandemic. Lancet Diabetes Endocrinol (2022) 10(1):1. doi: 10.1016/S2213-8587(21)00314-4
10. Tabarés Seisdedos R. Health effects of overweight and obesity in 195 countries over 25 years. New Engl J Med (2017) 377(1):13–27. doi: 10.1056/NEJMoa1614362
11. Bibbins-Domingo K, Coxson P, Pletcher MJ, Lightwood J, Goldman L. Adolescent overweight and future adult coronary heart disease. New Engl J Med (2007) 357(23):2371–9. doi: 10.1056/NEJMsa073166
12. Hannon TS, Rao G, Arslanian SA. Childhood obesity and type 2 diabetes mellitus. Pediatrics (2005) 116(2):473–80. doi: 10.1542/peds.2004-2536
13. Xue F, Rosner B, Eliassen H, Michels KB. Body fatness throughout the life course and the incidence of premenopausal breast cancer. Int J Epidemiol (2016) 45(4):1103–12. doi: 10.1093/ije/dyw149
14. Rodríguez MM, Gómez AH, Abitbol CL, Chandar JJ, Duara S, Zilleruelo GE. Histomorphometric analysis of postnatal glomerulogenesis in extremely preterm infants. Pediatr Dev Pathol (2004) 7(1):17–25. doi: 10.1007/s10024-003-3029-2
15. Davey Smith G, Ebrahim S. ‘Mendelian randomization’: can genetic epidemiology contribute to understanding environmental determinants of disease? Int J Epidemiol (2003) 32(1):1–22. doi: 10.1093/ije/dyg070
16. Lawlor DA, Harbord RM, Sterne JA, Timpson N, Davey Smith G, et al. Mendelian randomization: using genes as instruments for making causal inferences in epidemiology. Stat Med (2008) 27(8):1133–63. doi: 10.1002/sim.3034
17. Burgess S, Thompson SG. Multivariable Mendelian randomization: the use of pleiotropic genetic variants to estimate causal effects. Am J Epidemiol (2015) 181(4):251–60. doi: 10.1093/aje/kwu283
18. Sanderson E, Davey Smith G, Windmeijer F, Bowden J. An examination of multivariable Mendelian randomization in the single-sample and two-sample summary data settings. Int J Epidemiol (2019) 48(3):713–27. doi: 10.1093/ije/dyy262
19. Sanderson E, Richardson TG, Morris TT, Tilling K, Davey Smith G. Estimation of causal effects of a time-varying exposure at multiple time points through multivariable mendelian randomization. PloS Genet (2022) 18(7):e1010290. doi: 10.1371/journal.pgen.1010290
20. Richardson TG, Sanderson E, Elsworth B, Tilling K, Davey Smith G. Use of genetic variation to separate the effects of early and later life adiposity on disease risk: mendelian randomisation study. Bmj (2020) 369. doi: 10.1136/bmj.m1203
21. Brandkvist M, Bjørngaard JH, Ødegård RA, Åsvold BO, Smith GD, Brumpton B, et al. Separating the genetics of childhood and adult obesity: a validation study of genetic scores for body mass index in adolescence and adulthood in the HUNT Study. Hum Mol Genet (2020) 29(24):3966–73. doi: 10.1093/hmg/ddaa256
22. Hao Y, Xiao J, Liang Y, Wu X, Zhang H, Xiao, et al. Reassessing the causal role of obesity in breast cancer susceptibility: a comprehensive multivariable Mendelian randomization investigating the distribution and timing of exposure. Int J Epidemiol (2023) 52(1):58–70. doi: 10.1093/ije/dyac143
23. Papadimitriou N, Bull CJ, Jenab M, Hughes DJ, Bell JA, Sanderson E, et al. Separating the effects of early and later life adiposity on colorectal cancer risk: a Mendelian randomization study. BMC Med (2023) 21(1):5. doi: 10.1186/s12916-022-02702-9
24. Lu J, Liu X, Jiang S, Kan S, An Y, Zheng C, et al. Body mass index and risk of diabetic nephropathy: a Mendelian randomization study. J Clin Endocrinol Metab (2022) 107(6):1599–608. doi: 10.1210/clinem/dgac057
25. Pulit SL, Stoneman C, Morris AP, Wood AR, Glastonbury CA, Tyrrell J, et al. Meta-analysis of genome-wide association studies for body fat distribution in 694 649 individuals of European ancestry. Hum Mol Genet (2019) 28(1):166–74. doi: 10.1093/hmg/ddy327
26. Vogelezang S, Bradfield JP, Ahluwalia TS, Curtin JA, Lakka TA, Grarup N, et al. Novel loci for childhood body mass index and shared heritability with adult cardiometabolic traits. PloS Genet (2020) 16(10):e1008718. doi: 10.1371/journal.pgen.1008718
27. Kurki MI, Karjalainen J, Palta P, Sipilä TP, Kristiansson K, et al. FinnGen: Unique genetic insights from combining isolated population and national health register data. MedRxiv (2022), 2022.03.03.22271360. doi: 10.1101/2022.03.03.22271360
28. Chen J, Spracklen CN, Marenne G, Varshney A, Corbin LJ, Luan J, et al. The trans-ancestral genomic architecture of glycemic traits. Nat Genet (2021) 53(6):840–60. doi: 10.1038/s41588-021-00852-9
29. Manning AK, Hivert MF, Scott RA, Grimsby JL, Bouatia-Naji N, Chen H, et al. A genome-wide approach accounting for body mass index identifies genetic variants influencing fasting glycemic traits and insulin resistance. Nat Genet (2012) 44(6):659–69. doi: 10.1038/ng.2274
30. Ligthart S, Vaez A, Võsa U, Stathopoulou MG, de Vries PS, Prins BP, et al. Genome analyses of> 200,000 individuals identify 58 loci for chronic inflammation and highlight pathways that link inflammation and complex disorders. Am J Hum Genet (2018) 103(5):691–706. doi: 10.1016/j.ajhg.2018.09.009
31. Liu M, Jiang Y, Wedow R, Li Y, Brazel DM, Chen F, et al. Association studies of up to 1.2 million individuals yield new insights into the genetic etiology of tobacco and alcohol use. Nat Genet (2019) 51(2):237–44. doi: 10.1038/s41588-018-0307-5
32. Burgess S, Thompson SG, C.C.G. Collaboration. Avoiding bias from weak instruments in Mendelian randomization studies. Int J Epidemiol (2011) 40(3):755–64. doi: 10.1093/ije/dyr036
33. Burgess S, Butterworth A, Thompson SG. Mendelian randomization analysis with multiple genetic variants using summarized data. Genet Epidemiol (2013) 37(7):658–65. doi: 10.1002/gepi.21758
34. Bowden J, Davey Smith G, Burgess S. Mendelian randomization with invalid instruments: effect estimation and bias detection through Egger regression. Int J Epidemiol (2015) 44(2):512–25. doi: 10.1093/ije/dyv080
35. Bowden J, Davey Smith G, Haycock PC, Burgess S. Consistent estimation in Mendelian randomization with some invalid instruments using a weighted median estimator. Genet Epidemiol (2016) 40(4):304–14. doi: 10.1002/gepi.21965
36. Verbanck M, Chen CY, Neale B, Do R. Detection of widespread horizontal pleiotropy in causal relationships inferred from Mendelian randomization between complex traits and diseases. Nat Genet (2018) 50(5):693–8. doi: 10.1038/s41588-018-0099-7
37. Hemani G, Tilling K, Davey Smith G. Orienting the causal relationship between imprecisely measured traits using GWAS summary data. PloS Genet (2017) 13(11):e1007081. doi: 10.1371/journal.pgen.1007081
38. Burgess S, Zuber V, Valdes-Marquez E, Sun BB, Hopewell JC. Mendelian randomization with fine-mapped genetic data: choosing from large numbers of correlated instrumental variables. Genet Epidemiol (2017) 41(8):714–25. doi: 10.1002/gepi.22077
39. Hemani G, Zheng J, Elsworth B, Wade KH, Haberland V, Baird D, et al. The MR-Base platform supports systematic causal inference across the human phenome. elife (2018) 7:e34408. doi: 10.7554/eLife.34408
40. Todd JN, Dahlström EH, Salem RM, Sandholm N, Forsblom C, FinnDiane Study Group, et al. Genetic evidence for a causal role of obesity in diabetic kidney disease. Diabetes (2015) 64(12):4238–46. doi: 10.2337/db15-0254
41. Liu Y, Xu H, Zhao Z, Dong Y, Wang X, Niu J. No evidence for a causal link between Helicobacter pylori infection and nonalcoholic fatty liver disease: A bidirectional Mendelian randomization study. Front Microbiol (2022) 13:1018322. doi: 10.3389/fmicb.2022.1018322
42. Llewellyn A, Simmonds M, Owen CG, Woolacott N. Childhood obesity as a predictor of morbidity in adulthood: a systematic review and meta-analysis. Obes Rev (2016) 17(1):56–67. doi: 10.1111/obr.12316
43. Reilly JJ, Kelly J. Long-term impact of overweight and obesity in childhood and adolescence on morbidity and premature mortality in adulthood: systematic review. Int J Obes (2011) 35(7):891–8. doi: 10.1038/ijo.2010.222
44. Park MH, Falconer C, Viner RM, Kinra S. The impact of childhood obesity on morbidity and mortality in adulthood: a systematic review. Obes Rev (2012) 13(11):985–1000. doi: 10.1111/j.1467-789X.2012.01015.x
Keywords: Mendelian randomization, diabetic nephropathy, life course adiposity, childhood obesity, obesity
Citation: Zhang H, Zhang Q, Song Y, Wang L, Cai M, Bao J and Yu Q (2024) Separating the effects of life course adiposity on diabetic nephropathy: a comprehensive multivariable Mendelian randomization study. Front. Endocrinol. 15:1285872. doi: 10.3389/fendo.2024.1285872
Received: 30 August 2023; Accepted: 19 January 2024;
Published: 08 February 2024.
Edited by:
John D. Imig, University of Arkansas for Medical Sciences, United StatesReviewed by:
Yu Pan, Shanghai Jiao Tong University, ChinaCopyright © 2024 Zhang, Zhang, Song, Wang, Cai, Bao and Yu. This is an open-access article distributed under the terms of the Creative Commons Attribution License (CC BY). The use, distribution or reproduction in other forums is permitted, provided the original author(s) and the copyright owner(s) are credited and that the original publication in this journal is cited, in accordance with accepted academic practice. No use, distribution or reproduction is permitted which does not comply with these terms.
*Correspondence: Qing Yu, eXVxaW5nXzEyNTJtQDE2My5jb20=
Disclaimer: All claims expressed in this article are solely those of the authors and do not necessarily represent those of their affiliated organizations, or those of the publisher, the editors and the reviewers. Any product that may be evaluated in this article or claim that may be made by its manufacturer is not guaranteed or endorsed by the publisher.
Research integrity at Frontiers
Learn more about the work of our research integrity team to safeguard the quality of each article we publish.