- 1Department of Traditional Chinese Medicine (TCM) Gynecology, Hangzhou Hospital of Traditional Chinese Medicine Affiliated to Zhejiang Chinese Medical University, Hangzhou, China
- 2College of Pharmaceutical Science, Zhejiang Chinese Medical University, Hangzhou, China
- 3The First Clinical Medical College of Nanjing University of Chinese Medicine, Nanjing, China
Unexplained recurrent spontaneous abortion (URSA) is a severe challenge to reproductive females worldwide, and its etiology and pathogenesis have not yet been fully clarified. Abnormal intercellular communication between macrophages (Mφ) and decidual stromal cells (DSCs) or trophoblasts has been supposed to be the key to URSA. However, the exact molecular mechanisms in the crosstalk are not yet well understood. This study aimed to explore the potential molecule mechanism that may be involved in the communication between Mφ and DSC or trophoblast cells and determine their diagnostic characteristics by using the integrated research strategy of bioinformatics analysis, machine learning and experiments. First, microarrays of decidual tissue (GSE26787, GSE165004) and placenta tissue (GSE22490) in patients with URSA, as well as microarrays involving induced decidualization (GSE94644) and macrophage polarization in vitro (GSE30595) were derived from the gene expression omnibus (GEO) database. And 721 decidua-differentially expressed genes (DEGs), 613 placenta-DEGs, 510 Mφ polarization DEGs were obtained in URSA by differential expression analysis. Then, the protein-protein interaction (PPI) network was constructed, and the hub genes were identified by CytoHubba in Cytoscape software and validated by real-time PCR assay. Subsequently, immune enrichment analysis on decidua-DEGs and placenta-DEGs by ClueGO verified their regulation effects on Mφ. Besides, functional enrichment analysis was performed on Mφ polarization DEGs and the essential module genes derived from the weighted gene co-expression network analysis (WGCNA) to uncover the biological function that were related to abnormal polarization of Mφ. Furthermore, we screened out 29, 43 and 22 secreted protein-encoding genes from DSC-DEGs, placenta-DEGs and Mφ polarization DEGs, respectively. Besides, the hub secreted-protein-encoding genes were screened by CytoHubba. Moreover, we conducted functional enrichment analysis on these genes. And spearman correlation analysis between hub secreted-protein-encoding genes from donor cells and hub genes in recipient cells was performed to further understand the molecular mechanism of intercellular communication further. Moreover, signature genes with diagnostic value were screened from secreted protein-encoding genes by machine learning and validated by immunofluorescence co-localization analysis with clinical samples. Finally, three biomarkers of DSCs (FGF9, IL1R2, NID2) and three biomarkers of Mφ (CFB, NID2, CXCL11) were obtained. In conclusion, this project provides new ideas for understanding the mechanism regulatory network of intercellular communication involving macrophages at the maternal-fetal interface of URSA. Also, it provides innovative insights for the diagnosis and treatment of URSA.
1 Introduction
Recurrent spontaneous abortion (RSA) is defined as two or more consecutive miscarriages before 20 weeks of gestation with the same partner (1, 2). Approximately 1-5% reproductive women are afflicted with RSA, and 40-50% of cases remain unclear, which is defined as unexplained recurrent spontaneous abortion (URSA) (3). Accumulated evidence has profiled that disturbed regulatory mechanisms in the maternal-fetal interface are mainly responsible for URSA (4). Maternal-fetal interface is a crucial site for mutual recognition and information transfer between mothers and fetus, which mainly comprises decidual stromal cells (DSCs), decidual immune cells (DICs) and trophoblasts (5). DSCs are differentiated from endometrial stromal cells, i.e., decidualization, under the influence of hormones or embryo implantation signals (6). The decidualized phenotype contributes to precisely timed adaptations in endometrium for fetal growth (7). Trophoblasts, the main component of the placenta, are responsible for invading and reshaping the endometrial stroma and blood vessels in early pregnancy. What’s more, multiple researchers have suggested that the cellular abundance, phenotypes, and functions of DICs can be finely adjusted by DSCs and trophoblasts, the active contributors to the homeostatic and tolerogenic properties of maternal-fetal interface (8, 9). In turn, DICs can mediate maternal-derived DSC and fetal-derived trophoblasts (9). Once the complex and sophisticated cell-cell crosstalk is disrupted, recurrent embryo loss may occur. Therefore, exploring the mechanism of intercellular communication between DSCs, trophoblasts and DICs is conducive to further deciphering the pathological mechanisms of URSA.
Currently, various investigations have focused on the role of Mφ in intercellular communication at the maternal-fetal interface in URSA. As the second largest leukocytes at the maternal-fetal interface, Mφ account for 20-30% of DICs, and plays pivotal roles in regulating trophoblast invasion, remodeling spiral arteries, and maintenance of immune homeostasis (10, 11). Based on cellular phenotype and function, Mφ can be broadly categorized into classically activated subtypes (M1) and alternatively activated subtypes (M2) (12, 13), in which M1-polarized Mφ can efficiently clear antigens and switch T lymphocyte responses to T helper-1 (Th1) immune responses, thus inducing embryo rejection; while M2-polarized Mφ exhibit immunosuppressive properties and contribute to tissue remodeling, promoting Th2 immune response and maternal immune tolerance to embryos (14). Besides, neighboring cells, such as DSCs and trophoblasts, are thought to be critical in regulating Mφ polarization. Furthermore, recent studies have shed light on the dysregulation of DSCs as well as trophoblasts on macrophage polarization in URSA (15–17). However, the results are heterogeneous, mainly because traditional molecular biology methods are insufficient to provide a comprehensive landscape of molecule mechanism on intercellular communication. Nowadays, the revolutionary development of microarray techniques and bioinformatics make up for the current shortcomings and greatly facilitate in-depth insights into the characterization and pathogenesis of disease from both molecular and system levels.
In this study, we integrated and analyzed microarrays involving decidua and placental tissue of URSA, along with the mRNA expression profiles of in vitro decidualization and Mφ polarization from the gene expression omnibus (GEO) database, to obtain differentially expressed genes (DEGs) derived from decidua, placenta, and decidual stromal cells (DSCs) as well as DEGs related to Mφ polarization in URSA. We then performed protein-protein interaction (PPI) network analysis, hub gene screening and functional analysis by GeneMANIA for DSC-derived DEGs, placenta-derived DEGs and macrophage polarization related DEGs in RSA, respectively. After that, we further performed immune enrichment analysis on decidual and placental-derived DEGs by ClueGO to identify their regulation effects on Mφ. Moreover, functional enrichment analysis was conducted on Mφ polarization related DEGs as well as critical modules of macrophage polarization-related genes through weighted gene co-expression network analysis (WGCNA) to uncover the abnormal molecular mechanisms of Mφ polarization in URSA and the intercellular communication in which these molecules were involved in. Furthermore, we creatively focused on screening secreted protein-encoding genes from the above DEGs and performed PPI network analysis, hub gene screening and functional enrichment analysis on these secreted protein-encoding genes. In addition, we performed spearman correlation analysis on secreted protein-encoding genes and hub genes in receptor cell so as to further understand intercellular communication. Last but not least, biomarkers from secreted proteins were screened by machine learning and experiments based on clinical samples. Herein, our study deciphers the molecular mechanism of intercellular communication at the maternal-fetal interface of URSA, provides ideas for novel diagnostic strategies and targeted therapies for URSA, and also lays the foundation for subsequent studies. The workflow chart of the study is shown in Figure 1.
2 Materials and methods
2.1 Searching and screening microarray data
All the microarray datasets were retrieved from the GEO database (https://www.ncbi.nlm.nih.gov/geo/) (18). Firstly, GSE26787 and GSE165004 that respectively, involved the mRNA expression profiles of decidual tissues, and GSE22490 involved the mRNA expression profiles of villus tissues from URSA and health control samples were included, in which patients with URSA experienced at least two miscarriages, and health control had at least one live birth without a history of adverse pregnancy. Besides, dataset of GSE30595 was included, which involved mRNA expression profiles of Mφ polarization from M0 macrophage to M1 and M2 types in vitro. Finally, the dataset of GSE94644, which identified the transcription profiles of induced decidualization, was downloaded. The detailed information on each microarray was shown in Table 1.
2.2 Data preprocessing, normalizing and screening of DEGs
The raw data derived from GEO were preprocessed and normalized by R statistical software (version 4.1.2, https://www.r-project.org/) and Bioconductor analysis tools (http://www.bioconductor.org/). The robust multiarray averaging (RMA) and FPKM (Fragments Per Kilobase per Million) algorithms were utilized to implement background correction and quantile normalization. And the effect of batch correction was verified with the “ggplot2” package in R software, as shown in (Supplementary Figure 1). After that, DEGs were screened out by using the “limma” package, with the filtering condition of P value < 0.05 and |log2FC| >0.5. Besides, the volcano map and heat map of DEGs were respectively drawn by the “ggplot2” package and the “pheatmap” package. Then, Venn diagram was conducted to screen out the overlapped genes through the online software: jvenn (http://www.bioinformatics.com.cn/) (19).
2.3 Construction of PPI network and identification of hub genes
The STRING database (https://string-db.org/) was applied to perform PPI network analysis (20). CytoHubba, a Cytoscape software (version 3.7.1 plugin (https://cytoscape.org/) (21) was utilized to construct a PPI network of the interaction between DEGs with criteria were confidence score >0.9. And the top 15 genes were identified as the hub genes by Maximal Clique Centrality (MCC) algorithm (22). Furthermore, we also analyzed the functions and networks of these hub genes through GeneMANIA (http://genemania.org) (23).
2.4 Function enrichment analysis on decidua-DEGs, placenta-DEGs and Mφ polarization DEGs
To preliminary explore the regulation effects of decidua-DEGs and placenta-DEGs on Mφ, we performed immune enrichment analysis on decidua-DEGs and placenta-DEGs by a Cytoscape plugin “ClueGO” under the criterion of P<0.05. ClueGO can classify non-redundant Gene Ontology (GO) terms and visualize the functionally related genes in a clustered network (24). Besides, to comprehend the biological processes that Mφ polarization DEGs were involved in, we performed Kyoto Encyclopedia of Genes and Genomes (KEGG), rectome and Wiki pathway enrichment analysis on the Webgestalt website (http://www.webgestalt.org/) (25) under the criterion of P<0.05.
2.5 Construction of co-expression network and hub module identification by WGCNA
The WGCNA is widely used to uncover critically interacted genetic modules and essential genes by linking gene networks to clinical traits. In this study, the WGCNA co-expression system was established to investigate the modules that were highly associated with URSA by using the “WGCNA” package in R software based on genes related to Mφ polarization. First, genes with variation higher than 25% across samples in the combined dataset were selected as the input data set for the subsequent WGCNA. Then, the outlier cases were removed by hierarchical clustering analysis with the “goodSamplesGenes” function. After that, the appropriate soft threshold was determined by using the pickSoftThreshold function and validated by the correlation between k and p (k). Subsequently, the correlation matrix was converted into an adjacency matrix, which was further processed into a topological overlap matrix (TOM). The dynamic tree cutting approach was performed to identify various modules. The relationship between these modules and URSA was investigated. Finally, a module with the greatest Pearson correlation coefficient was picked for further investigation.
2.6 Screening secreted protein-encoding DEGs from total DEGs
Secreted proteins are potential signaling molecules for intercellular communication. The DSCs-derived and trophoblast-derived secreted proteins may be the possible active molecules for regulating Mφ polarization in the maternal-fetal interface. And therefore, we focused on secreted protein-encoding genes in DSC derived-DEGs and trophoblasts derived-DEGs in URSA. In this part, we retrieved the Human Protein Atlas website (https://www.proteinatlas.org/) to download the dataset of secreted protein-encoding genes. And then, the overlapped genes of secreted protein-encoding genes and decidua-DEGs, placenta-DEGs and Mφ polarization DEGs were separately obtained by using the Venn diagram tool.
2.7 PPI analysis, hub gene identification and physicochemical property analysis on secreted protein-encoding DEGs
First, PPI analysis and hub genes screening based on the secreted protein-encoding DEGs were performed as mentioned above. The top 10 under the MCC algorithm were defined as hub secreted protein-encoding genes. Then, to further unravel the physicochemical properties of hub secreted protein-encoding genes, we acquired their multiple physicochemical property parameters through the online tool ProtParam website (https://web.expasy.org/protparam/, accessed on 06/13/2022) (26), including molecular weight, theoretical pI, extinction coefficient, instability index, lipid index, and hydrophilic grand average (GRAVY).
2.8 Function enrichment analysis
Besides, to annotate the functions of genes in hub module identification by WGCNA as well as secreted protein-encoding genes, we conducted GO and KEGG pathway analysis by Webgestalt. GO was extensively applied to comprehensively describe the properties of genes and gene products in organisms, including biological process (BP), cell components (CC), and molecular function (MF). KEGG is available for systematic analysis of gene functions, link genome information and functions, and is able to build modules through computer processing of biological processes. In the above analysis, the species was selected as “Homo sapiens”, and the reference set was selected as genome protein-coding. GO terms and pathways with a threshold of P value <0.05 were filtered, and the top 10 terms (with the highest degree values) were displayed in a bubble plot by the “ggplot2” package in R software.
2.9 Diagnostic value of the secreted protein-encoding genes for URSA
Machine learning provides optimal methods to improve the accuracy of diagnosis models. In this study, the least absolute shrinkage and selection operator (LASSO) regression analysis and support vector machine recursive feature elimination (SVM-RFE) were performed to identify secreted protein-encoding genes with diagnostic value, namely signature genes. LASSO regression analysis is a refined model by constructing a penalty function based on a linear regression model, which can improve the stability of the model and the classification efficacy. The “glmne” package in R software was utilized for LASSO regression analysis (27). Besides, SVM-RFE is a support vector machine based on an optimal feature selection algorithm that ranks features on the basis of a recursive feature deletion sequence (28). R package “e1071” was utilized to construct the SVM-RFE classifier and analyze the candidate biomarkers in RSA. What’s more, to evaluate the diagnostic value of these genes, receiver operating characteristic (ROC) curve was applied, and the area under the curve (AUC) was calculated via the pROC R package. In addition, “rms” package in R software was also utilized to establish the nomogram prediction model. Each secreted protein-encoding gene with diagnostic value was converted into an assessment point system in the model. And the total score determined the final risk assessment value. Besides, the performance of the nomogram was assessed by calibration and discrimination, which was respectively evaluated by a visual calibration plot and ROC through the “rms” and “pROC” packages in R software.
2.10 Validation of hub genes and signature genes in clinical samples
Decidua and villus tissues were collected from healthy women required for induced abortion for unwanted pregnancies and patients with URSA in Hangzhou Hospital of Traditional Chinese Medicine Affiliated to Zhejiang Chinese Medical University from December 2022 to March 2023. Women with the following criteria were excluded from the study: (a) symptoms of endocrine or metabolic disease, (b) abnormal karyotype analysis, (c) uterine abnormalities or other identifiable causes of miscarriage.
For the validation of hub genes, total RNA from decidua and villus tissues were extracted by Trizol method, reverse transcription was performed by HiFiScript cDNA Synthesis Kit (CW2569M; CWBIO), and real time PCR was performed by SYBR Green Pro Taq HS premixed qPCR kit (AG11701; ACCURATE BIOLOGY). Finally, two-step PCR reaction procedure was used to perform the reaction, and then the computed CT values were calculated by 2−(ΔΔCT) method. The primer sequences used for quantitative real-time PCR (qRT-PCR) were as follows:
MX1: Forward primer (5′-3′) CGGTTCTGGGTCGGAGGCTAC, Reverse primer (5′-3′) CTGG ATGGCGGCGTTCTTCAC; IFI27: Forward primer (5′-3′) TCACTGGGAGCAACTGGACTC TC, Reverse primer (5′-3′) TCGCAATGACAGCCGCAATGG; IFIT3: Forward primer (5′-3′) GGAGAATGGCGTGAACCTGGAAG, Reverse primer (5′-3′) TTGAGATGGAGTCTTGCTC TGTTGC; LYN: Forward primer (5′-3′) TGAAGAGCGATGAAGGTGGCAAAG, Reverse primer (5′-3′) GTGACTCGGAGACCAGAACATTAGC; NNMT: Forward primer (5′-3′)AAG GGCTGAACTGATGGAAGGAATG, Reverse primer (5′-3′) CACTTCTGTACCACTGGAGC ACTG; SNCA: Forward primer (5′-3′) GTGGCAACAGTGGCTGAGAAGAC, Reverse primer (5′-3′) TACTGCTGTCACACCCGTCACC; IFIT1: Forward primer (5′-3′) CGAGAGCAGCCTT GGCAATGG, Reverse primer (5′-3′) TCCAGCAGTCCACTCACCTCAG; OAS2: Forward primer (5′-3′) CCAAAGAAGCGGGTGCCAGAC, Reverse primer (5′-3′) GAGAGCGAGTCC AGGGTAGAAGG.
As for the validation of signature genes decidua tissues were fixed with 4% paraformaldehyde and embedded in paraffin for immunofluorescence analysis, which was conducted following previously described methods (29). In this study, Vimentin representative decidual stromal cell and CX3CR1 representative macrophage. Digital pathological section (fluorescence) scanning analyzer (VS120-S6-W, OLYMPUS) was used to observe and photograph the images. Pearson correlation coefficient of FGF9-Vimentin, IL1R2-Vimentin, NID2-Vimentin, CFB-CX3CR1, CXCL11-CX3CR1 and NID2-CX3CR1 were performed using Image J software (version 1.53v). All samples were collected with the informed consent of patients, and all relevant procedures were approved by the Internal review and Ethics Committee of Hangzhou Hospital of Traditional Chinese Medicine affiliated to Zhejiang Chinese Medical University (NO. 2022LH007).
3 Results
3.1 Differential expression analysis to screen decidua-DEGs, placenta-DEGs, and Mφ polarization DEGs of URSA
After preprocessing and normalization of the included datasets, the limma package in R software was utilized to find the DEGs of URSA in decidual tissue (decidua-DEGs) as well as in placenta tissue (placenta-DEGs), with the screening conditions of P value <0.05 and |log2FC|>0.5. A total of 721 decidua-DEGs and 613 placenta-DEGs were obtained. Besides, 1532 genes related to induced decidualization derived from GSE94644 were intersected with decidua-DEGs, and 106 overlapped genes were acquired, which were taken as DEGs of DSCs in URSA, namely DSCs-DEGs. The DEGs mentioned above were separately visualized using a heatmap and volcano map, as shown in Figures 2A-G.
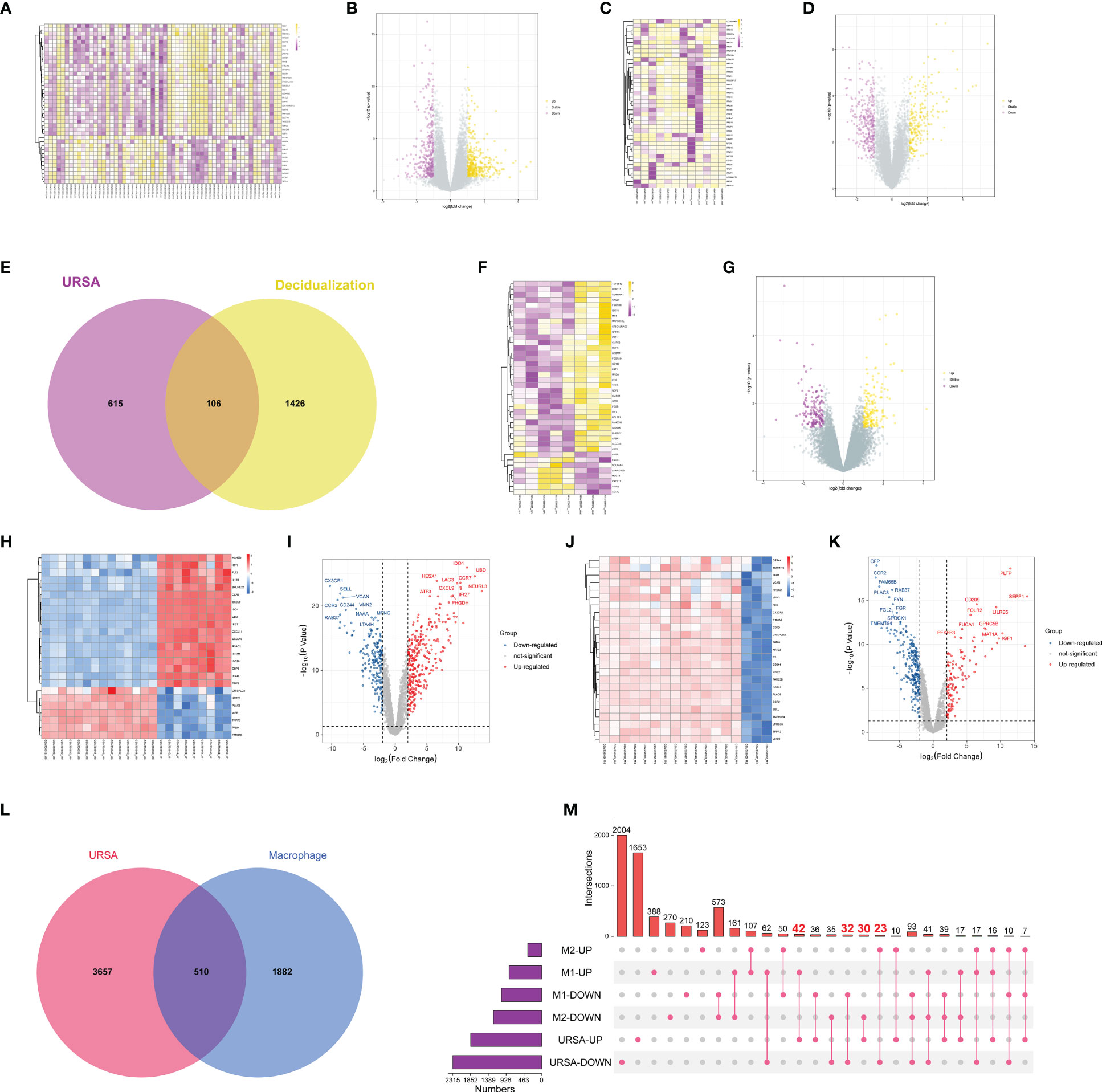
Figure 2 Differential expression analysis on genes at maternal-fetal interface of URSA. (A) Heat map and (B) volcano plot visualized the decidua-DEGs of URSA. (C) Heat map and (D) volcano plot visualized the decidualization related genes. (E) Venn diagram showed DSCs-DEGs by intersecting decidua-DEGs and decidualization related genes. (F) Heat map and (G) volcano plot visualized the placenta-DEGs of URSA. (H) Heat map and (I) volcano plot visualized the DEGs screened from the M0 and M1 macrophages. (J) Heat map and (K) volcano plot visualized the DEGs screened from the M0 and M2 macrophages. Venn diagram (L) and upset diagram (M) showed the intersection of decidua-DEGs with macrophage polarization related genes and decidua-DEGs, namely macrophage polarization DEGs. In the heatmap, each row represented a DEG, each column represents a sample, and in the volcano plot, each dot represents a gene, in which yellow and red plot points represented upregulated genes, and purple plot and blue points represent down-regulated genes.
Furthermore, we obtained 1901 M1 polarization-related DEGs and 1622 M2 polarization- related DEGs from the GSE30595 data set with the same filter conditions as mentioned above. The heat map and volcano map were shown in Figures 2H-L. And there were 510 overlapped genes after intersecting the Mφ polarization-related DEGs with decidua-DEGs, namely Mφ polarization DEGs. And then, the upset diagram was plotted based on M1 and M2 polarization-related genes with decidua-DEGs, and the results manifested that 42 genes promoting M1 polarization were upregulated in URSA and 32 genes inhibiting M1 polarization were down-regulated in URSA. Besides, 23 genes promoting M2 polarization were downregulated in URSA, and 30 genes suppressing M2 polarization were up-regulated in URSA (Figure 2M).
3.2 Construction of PPI network and identification of hub genes
Proteins are the primary carriers of biological activity. To understand how proteins encoded by these DEGs interact, DSCs-DEGs, placenta-DEGs, and Mφ polarization DEGs were uploaded to the STRING website to construct the PPI network, respectively, as shown in Figures 3A-C. Subsequently, hub genes in networks were detected by the MCC algorithm (Cytoscape, CytoHubba) (30). Hub genes in DSCs included NNMT, LYN, SNCA. Hub genes derived from the placenta contained IFIT1, IFIT3, OAS2, etc. Decidual Mφ derived hub genes involved IFIT3, IFI27, MX1, etc. (Table 2). After that, the hub genes were converged for PPI analysis, which exhibited close interactions between these hub genes (Figure 3D). Furthermore, we also analyzed the functions and networks of these hub genes through GeneMANIA, and the result suggested that these genes were closely linked both in localization and functions and were mainly involved in the regulation of immune activity, including response to type I interferon, toll-like receptor signaling pathway, regulation of B cell activation and so on (Figure 3E). Finally, we verified the obtained hub genes with clinical samples by qRT-PCR. The results showed that for DSCs-hub DEGs, LYN, NNMT and SNCA significantly decreased in URSA group (P<0.05) (Figure 3F), for placenta-hub DEGs, IFIT1 and IFIT3 significantly increased in URSA group (P<0.05) (Figure 3G), while OAS2 tends to be elevated in URSA (P=0.058). As for Mφ polarization associated hub DEGs, IFIT3, IFI27 and MX1 were significantly increased in URSA group (for IFIT3 and IFI27, P<0.05; for MX1, P<0.01) (Figure 3H).
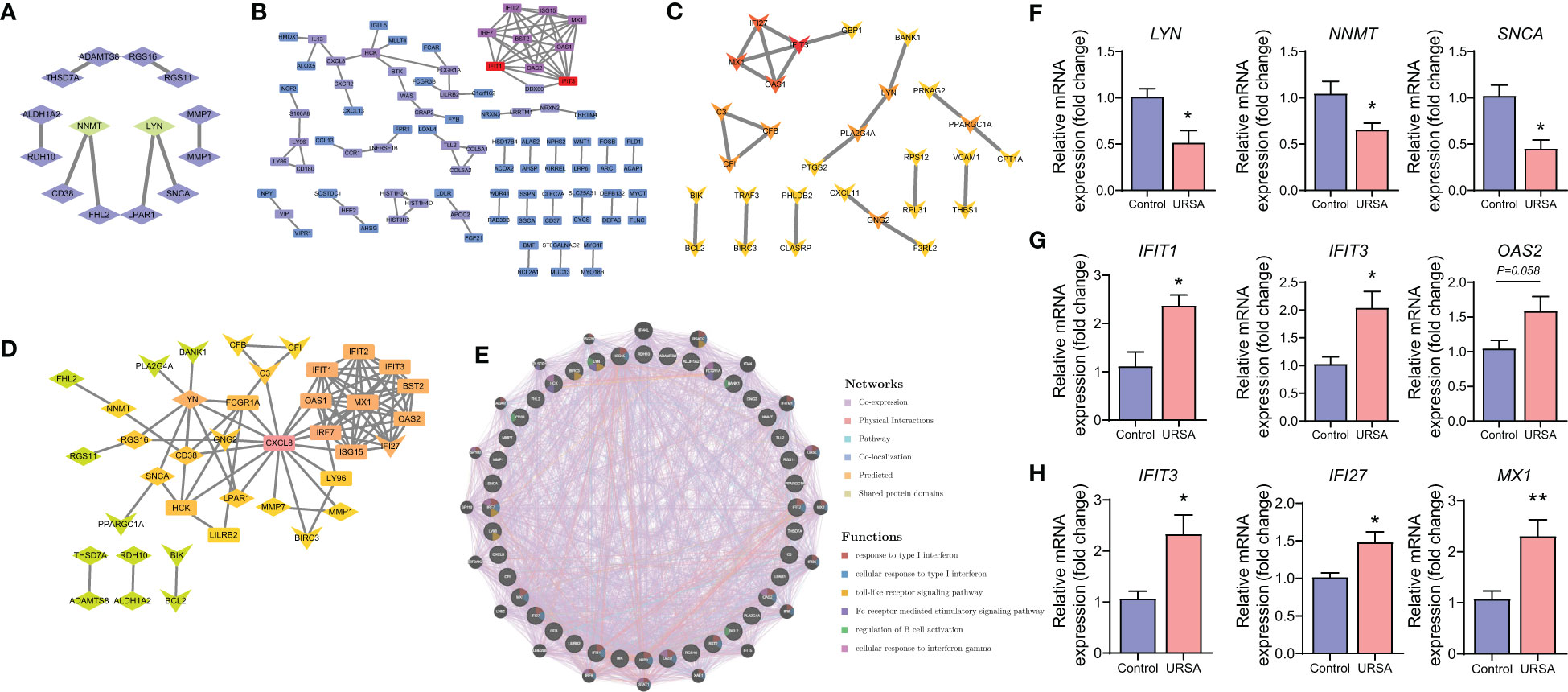
Figure 3 Protein-protein interaction analysis on DEGs at maternal-fetal interface. PPI network of DSC-DEGs (A), placenta-DEGs (B) and macrophages polarization DEGs (C). The PPI network of hub genes (D), in which the diamonds, the rectangles and the “V”-shape nodes represented hub genes from DSC-DEGs, from macrophages polarization DEGs, and from placenta-DEGs, respectively. The functions of the hub proteins were predicted by the GeneMANIA plug-in in Cytoscape (E). The qRT-PCR results of DSC-DEGs (F), placenta-DEGs (G) and macrophages polarization DEGs (H). * represents P<0.05, **represents P<0.01, compared with the control group.
3.3 Immunoenrichment analysis on decidua-DEGs and placenta-DEGs in URSA
We performed immune enrichment analysis on decidua-DEGs and placenta-DEGs using the “ClueGO” plugin in Cytoscape. The results demonstrated that decidua-DEGs in URSA were mainly enriched in negative regulation of immune response, Mφ tolerance induction, and negative regulation of Mφ antigen processing and presentation. And placenta-DEGs in URSA were mainly enriched in the cellular response to type I interferon, Mφ tolerance induction, regulation of Mφ antigen processing and presentation, Mφ activation, and so on (Figures 4A, B). From the above analysis, we could indicate that DEGs in the decidua and placenta of URSA were involved in various immune processes, and the regulation effects on Mφ were one of its important manifestations.
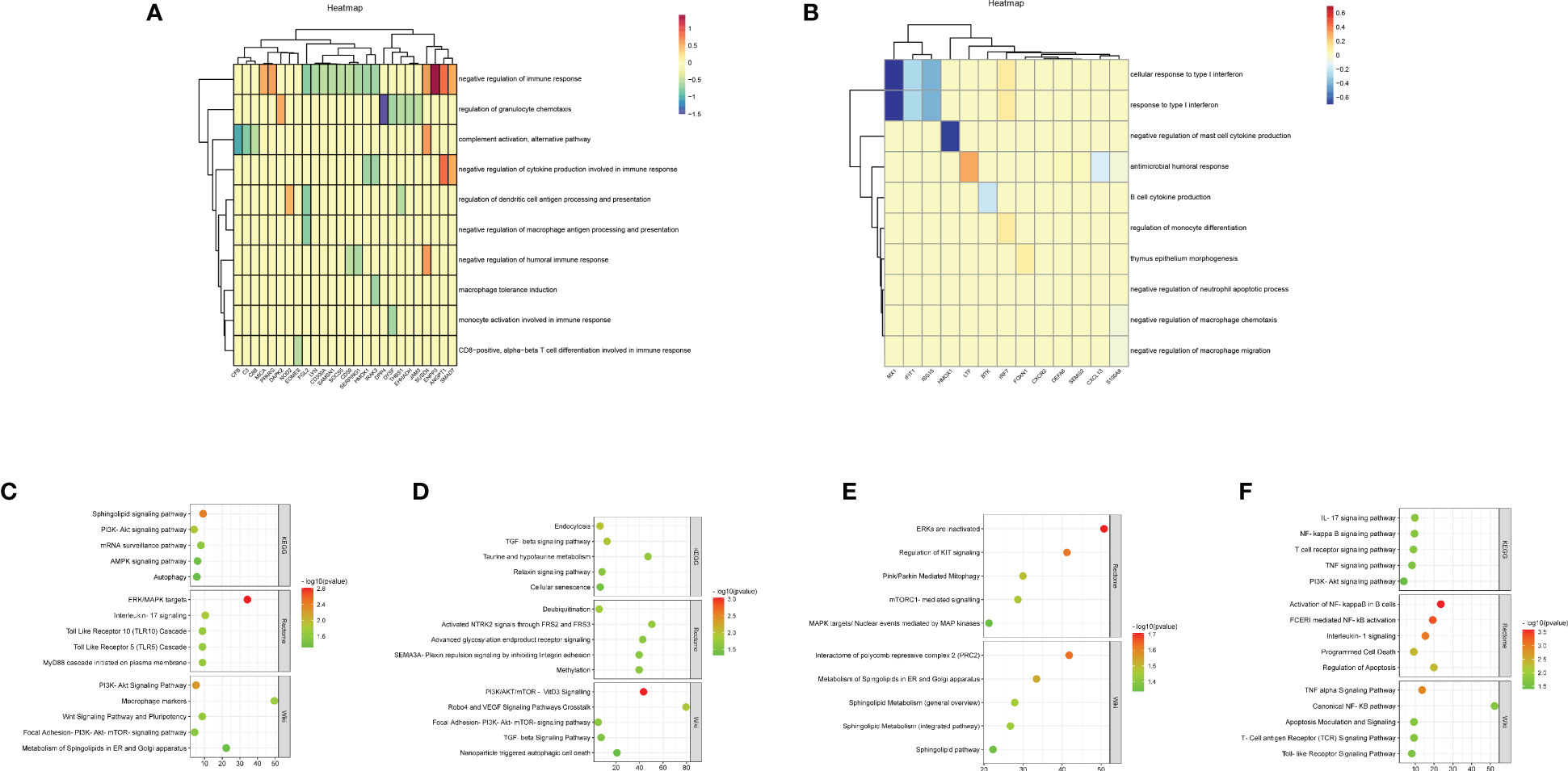
Figure 4 Functional analysis of DEGs to reveal cell-cell interactions at maternal-fetal interface The heatmap visualized immune enrichment analysis of decidua-DEGs (A) and placenta-DEGs (B) by ClueGO plug-in in Cytoscape. The bubble chart displayed the functional enrichment analysis of the upregulated genes that promoted M1 polarization in URSA (C), the downregulated genes in URSA that inhibited M1 polarization (D), the downregulated genes that promoted M2 polarization (E) and the upregulated genes that inhibited M2 polarization (F).
3.4 Functional enrichment analysis of Mφ polarization DEGs in URSA
To comprehend the biological processes that are associated with Mφ polarization DEGs, we performed KEGG, rectome and Wiki pathway enrichment analysis by using Webgestalt. The results indicated that the upregulated genes that promoted M1 polarization in URSA were mainly enriched in Sphingolipid signaling pathway, ERK/MAPK signaling pathway, interleukin-17 signaling pathway, PI3K-Akt signaling pathway, etc.(Figure 4C). The downregulated genes in URSA that inhibited M1 polarization were associated with TGF-β signaling pathway, cellular senescence, deubiquitination, PI3K/AKT/mTOR-VitD3 signaling pathway and TGF-β receptor signaling, etc.(Figure 4D) The downregulated genes that promoted M2 polarization were primarily involved in Pink/Parkin mediated mitophagy, mTORC1 mediated signaling pathway, metabolism of Spingolipids in ER and Golgi apparatus, etc.(Figure 4E). The upregulated genes that inhibited M2 polarization in URSA were enriched in VEGF signaling pathway, apoptosis, activation of NF-kappaB in B cells, and TNF alpha signaling pathway (Figure 4F). From the above results, it can be inferred that DEGs that tend to enhance the M1/M2 ratio in URSA could activate pro-inflammatory responses and regulate intercellular communication and cell fate, etc.
3.5 WGCNA for Mφ polarization related genes and enrichment analysis on genes in critical modules
The co-expression network was conducted with WGCNA package to detect the critically interacted genetic modules based on the mRNA expression profiling of Mφ polarization- related genes. After matching the sample traits with the expression matrix, the sample cluster tree was shown in Figure 5A. The power of β= five was selected as the soft thresholding power to ensure a scale-free network according to the scale independence and mean connectivity values (Figure 5B). In total, four modules were identified by WGCNA (Figure 5C). The specific module information was shown in Table 3. Then heatmaps of module-trait relationships were plotted to assess the correlation between each module and clinical traits (URSA and health control), as shown in Figure 5D. Besides, Figure 5E displayed that genes in the yellow module were significantly positively correlated with URSA modules (cor=0.76, P=4e−12), and genes in the blue module were significantly negatively correlated with URSA (cor=-0.54, P=1e−05) (Figure 5F). Considering that the blue and yellow modules were the most significantly related to URSA among all the modules, genes in the two modules were picked for further functional enrichment analysis.
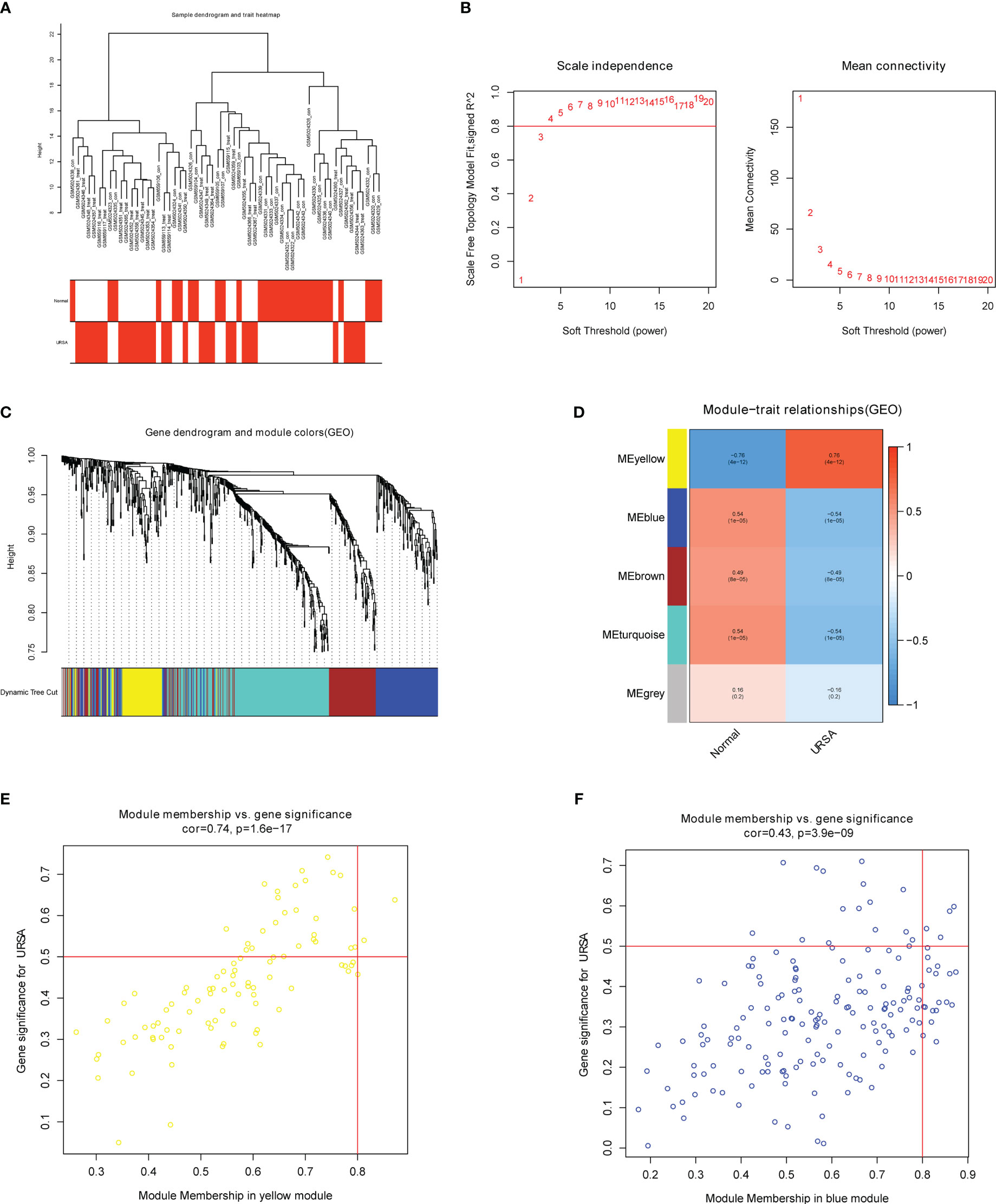
Figure 5 WGCNA for macrophage polarization related genes (A) Clustering dendrogram of samples with trait heatmap. (B) Analysis of the scale-free fit index and the mean connectivity for various soft-thresholding powers. (C) Clustering dendrogram of genes by average linkage hierarchical clustering, in which 4 modules were identified. Each branch represents a gene, and each color underneath the branch represents a co-expression module. (D) Heat map visualizing the correlation between modules and RPL. (E, F) A scatter plot showing the correlationship between MM (X-axis) vs. GS (Y-axis) in the yellow and blue modules.
The BP of GO enrichment analysis illustrated that genes in the blue module were enriched in negative regulation of the immune system process, regulation of Mφ differentiation and Mφ chemotaxis, etc. In KEGG analysis, the enriched pathways of blue module genes were necroptosis, IL-17 signaling pathway, TNF signaling pathway, and autophagy, etc. (Figure 6A, B) Genes in yellow modules are mainly involved in the BP of positive regulation of leukocyte proliferation, activating cell surface receptor signaling pathway, and Ras protein signal transduction, etc. As for KEGG pathway analysis, genes in yellow modules were enriched in T cell receptor signaling pathway, MAPK signaling pathway, Fc gamma R-mediated phagocytosis, and chemokine signaling pathway, etc. (Figures 6C, D) From the above results, it could be assumed that the blue module genes mainly functioned positively for embryonic immune tolerance, while the yellow module genes were chiefly involved in embryonic immune rejection. In addition, we also discovered that Mφ polarization DEGs communicated intracellular and extracellular signaling cascades to achieve information exchange between cells. However, the specific communication mechanism remained to be further explored.
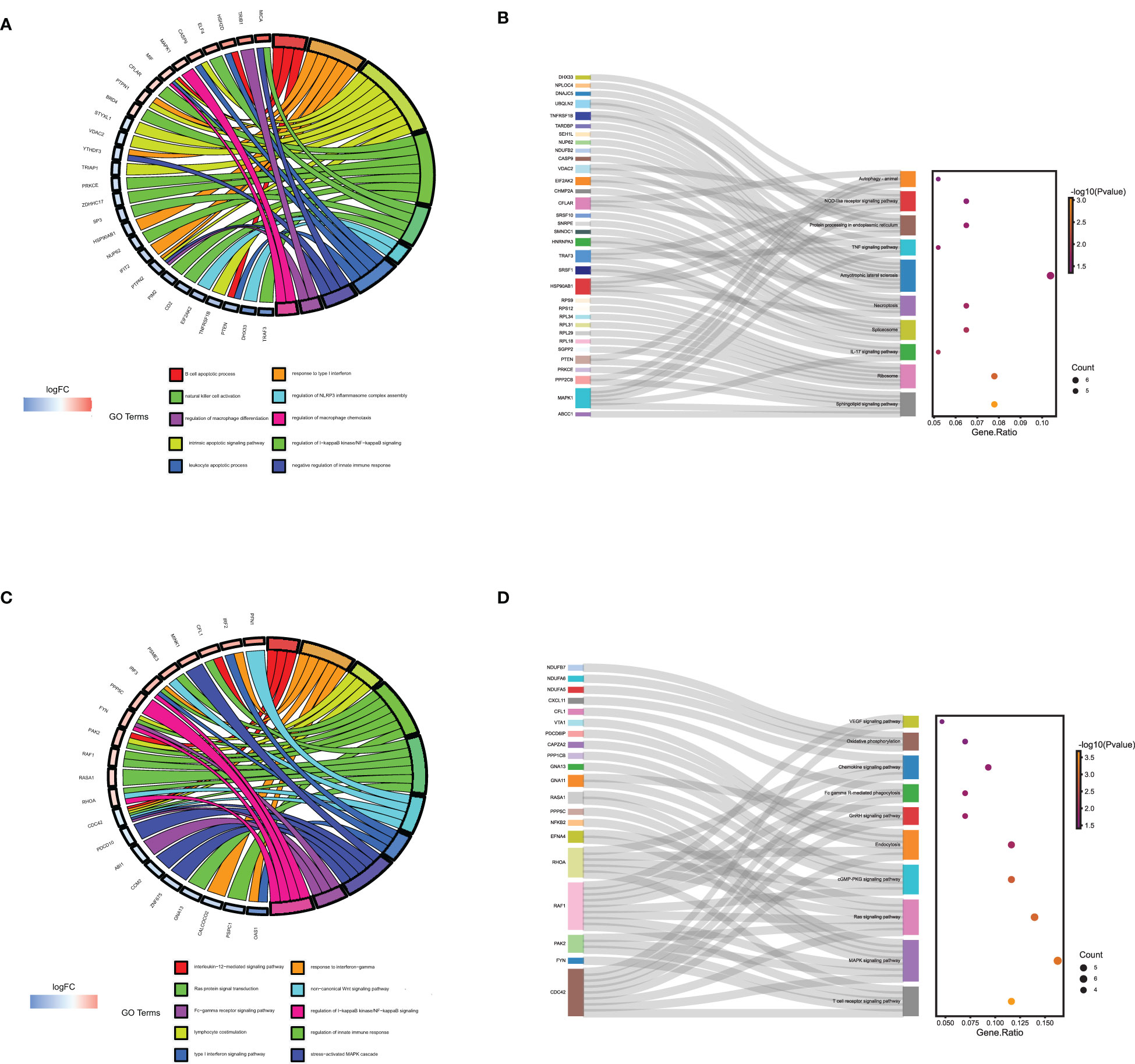
Figure 6 The GO and KEGG enrichment analysis of genes in the yellow and blue module derived from WGNCA. The GO (A) and KEGG enrichment analysis (B) of genes in the yellow module by Webgestalt. The GO (C) and KEGG enrichment analysis (D) of genes in the blue module by Webgestalt.
3.6 Identification of the secreted protein-encoding genes at the maternal-fetal interface in URSA
Secreted proteins are pivotal mediators of intercellular communication. To clarify the intercellular communication at the maternal-fetal interface in RSA, we identified DEGs that could encode secreted proteins from DSCs, placenta, and decidual Mφ, separately. Firstly, a total of 1903 secreted were obtained from the Human Protein Atlas website. Subsequently, the decidua-DEGs, along with the decidualization-related DEGs were intersected with the 1903 secreted proteins, and a total of 29 secreted protein-encoding DEGs of DSCs in URSA were obtained. In addition, we intersected decidua-DEGs, Mφ polarization related genes in the 1903 secreted proteins, and finally obtained 22 decidual Mφ derived secreted proteins encoding DEGs (Figure 7A). Similarly, 43 secreted protein encoding DEGs derived from the placenta were obtained by intersecting placenta DEGs with the 1903 secreted proteins (Figure 7B).
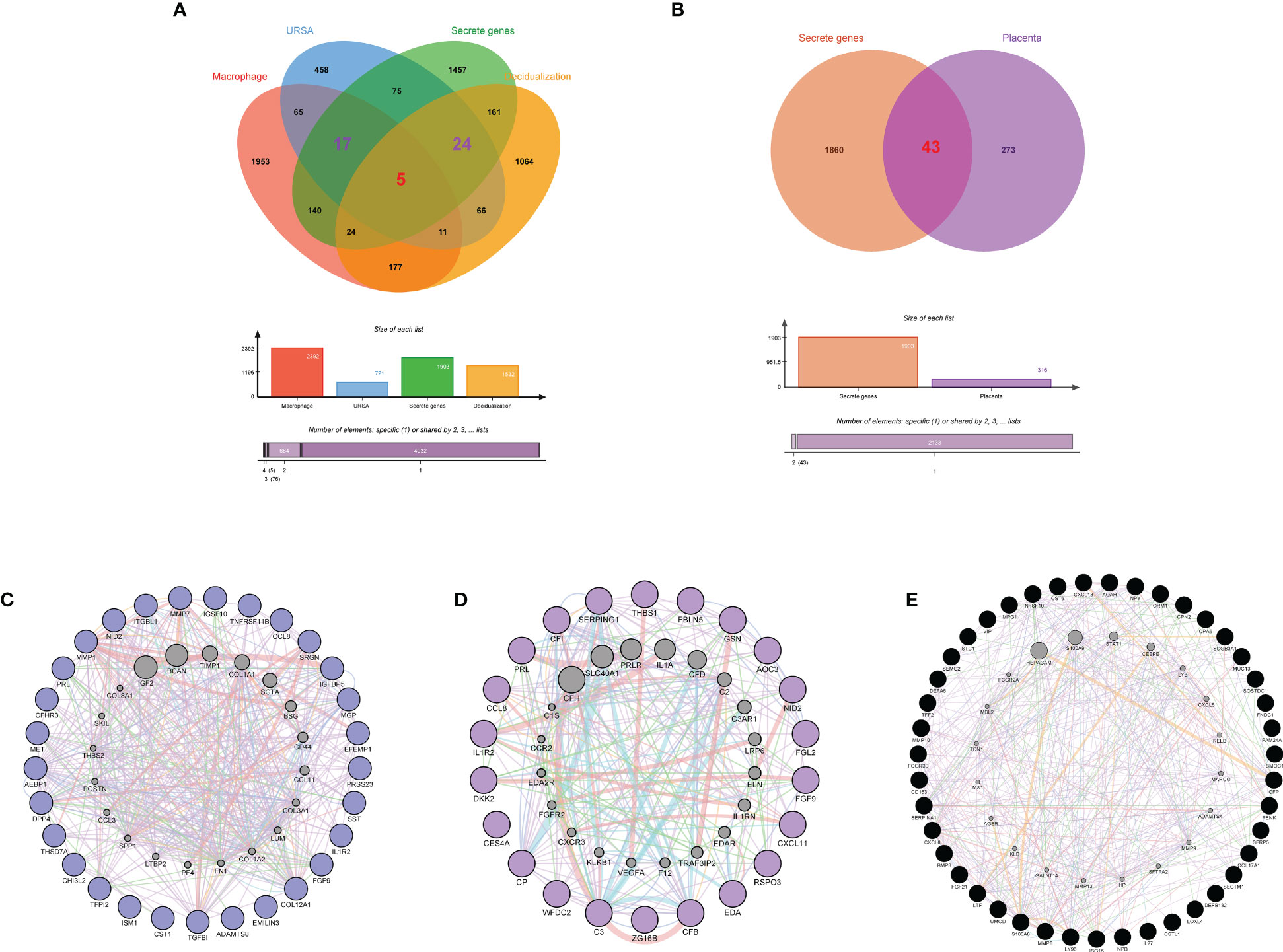
Figure 7 Identification of secreted protein encoding genes at maternal fetal interface in URSA and PPI analysis on them. Venn diagram of secreted protein encoding gene sets, decidua-DEGs, decidualization related genes and macrophages polarization related genes (A). Venn diagram of secreted protein encoding gene sets and placenta-DSCs (B). PPI network of secreted protein encoding DEGs derived from DSCs (C), macrophages (D) and placenta (E).
3.7 PPI network construction, hub gene identification, and physicochemical property analysis of secreted proteins
PPI networks were constructed for secreted proteins encoding genes from DSCs, placenta and decidual Mφ by GeneMANIA, and hub-secreted proteins encoding genes were screened by MCC algorithm in cytoHubba of Cytoscape software. In DSCs, the hub-secreted proteins encoding genes included MMP1, COL12A1, MET, MMP7, and TNFRSF11B, etc. (Figure 7C). The hub-secreted proteins encoding genes of decidual Mφ covered C3, CFB, SERPING1,CP and CFI (Figure 7D). In the placenta, the hub-secreted protein-encoding genes consist of CXCL8, SERPINA1, FCGR3B, S100A8, and LTF, etc. (Figure 7E). Besides, we reported the physicochemical properties of the identified hub-secreted proteins, which is vital for the in-depth investigation of the significant biomolecules. The detailed information about hub-secreted protein-encoding genes was shown in Table 4.
3.8 Functional enrichment analysis of secreted proteins encoding DEGs at maternal-fetal interface in URSA
GO and KEGG enrichment analysis of secreted proteins encoding DEGs of DSCs, placental and decidual Mφ was respectively performed in this analysis. The 29 secreted proteins encoding DEGs of DSCs were mainly distributed in the cellular component of collagen-containing extracellular matrix, basement membrane, integrin complex, etc., with molecular functions such as receptor-ligand activity, integrin binding and transmembrane receptor protein kinase activity. These genes were predominantly involved in BPs of extracellular matrix organization, positive regulation of protein kinase B signaling, and female pregnancy (Figure 8A). KEGG enrichment analysis corroborated that these 29 DEGs were mainly enriched in cytokine-cytokine receptor interaction, PI3K-Akt signaling pathway, Rap1 signaling pathway, and so on (Figure 8B).
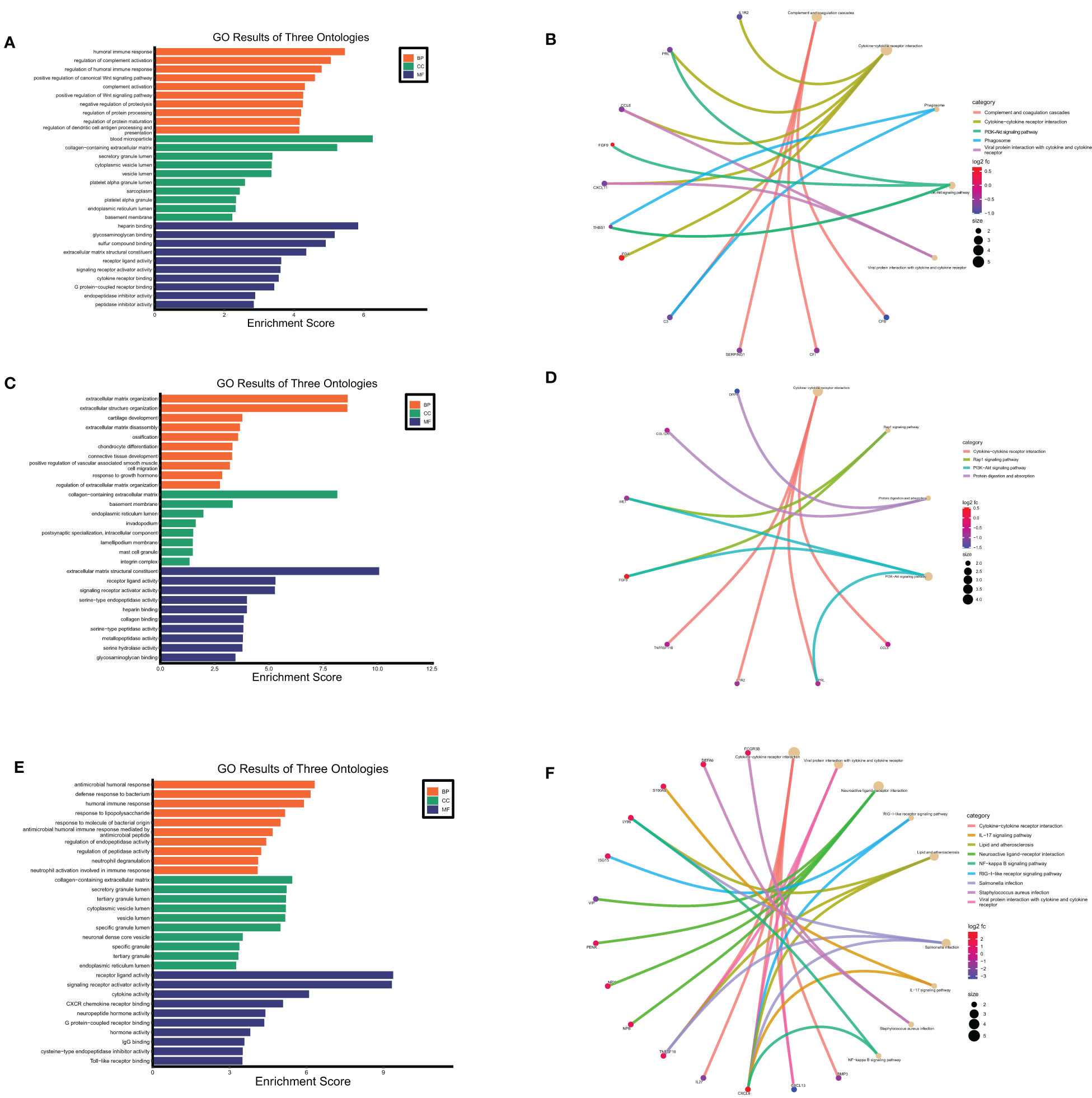
Figure 8 Functional enrichment analysis of secreted proteins encoding DEGs at maternal fetal interface in URSA. The barplot showed the results of GO enrichment analysis on secreted protein encoding DEGs derived from DSCs (A), macrophages (C) and placenta (E). The circular network plot visualized the results of KEGG analysis on secreted protein encoding DEGs derived from DSCs (B), macrophages (D) and placenta (F), in which khaki nodes represented terms, the color from blue to red nodes represented secreted protein encoding DEGs, and connecting lines represented the relationship between terms and genes.
GO enrichment analysis on the 22 secreted-protein encoding DEGs from decidual Mφ implied that these genes were primarily expressed in collagen-containing extracellular matrix, secretory granule lumen and cytoplasmic vesicle lumen, mainly affected receptor ligand activity, cytokine receptor binding and chemokine activity, and involved in humoral immune response, regulation of complement activation, regulation of dendritic cell antigen processing and presentation, etc.(Figure 8C). KEGG analysis on these genes suggested that these genes primarily participated in cytokine-cytokine receptor interaction, PI3K-Akt signaling pathway and positive regulation of Wnt signaling pathway (Figure 8D).
As for the 43 secreted protein encoding DEGs from placenta of URSA, the results of GO-CC analysis verified that DEGs were mainly enriched in collagen-containing extracellular matrix, cytoplasmic vesicle lumen, secretory granule lumen. And GO-BP analysis indicated that DEGs were mainly involved in humoral immune response, response to lipopolysaccharide and neutrophil degranulation, etc. Regarding GO-MF analysis, the 43 DEGs were primarily related to cytokine activity, CXCR chemokine receptor binding and IgG binding, etc.(Figure 8E). The KEGG analysis indicated that the 43 DEGs mainly participated in cytokine-cytokine receptor interaction, IL-17 signaling pathway and NF-kappa B signaling pathway (Figure 8F).
3.9 Correlations between cells at the maternal-fetal interface evaluated by hub secreted protein-encoding genes of donor cells and hub genes in recipient cells
To investigate the cellular communication between DSC, placenta, and Mφ in URSA, we applied Spearman analysis test to detect the correlation between DSC or placenta-derived hub secreted protein-encoding genes and the hub genes of Mφ, along with the correlation between Mφ derived critical secreted proteins and the hub genes of DSC or placenta in URSA samples.
Spearman correlation analysis on hub secreted protein encoding genes of DSCs and hub genes of decidual Mφ demonstrated that EFEMP1 was closely and positively correlated with C3 (r=0.78, P<0.01), followed by EFEMP1 with IFIT3 (r=0.76, P<0.01) and MET with GBP1 (r=0.74, P<0.01). Besides, FGF9 was closely and negatively correlated with GBP1 (r=-0.48, P<0.01), followed by FGF9 with SERPING1 (r=-0.42, P<0.05), TGFBI with OAS1 (r=-0.38, P<0.05) (Figures 9A, B).
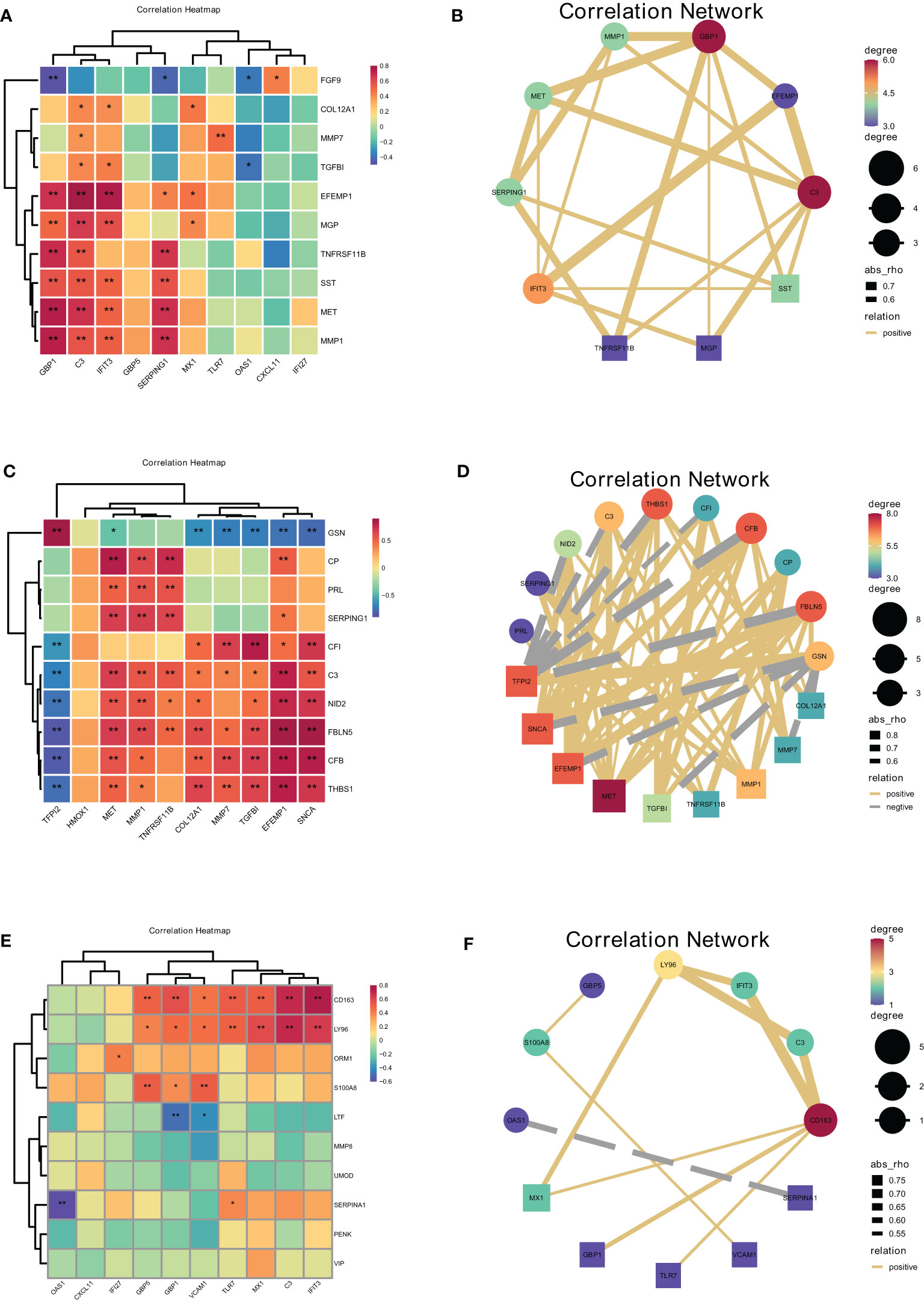
Figure 9 Correlations between cells at the maternal-fetal interface evaluated by hub secreted protein encoding genes of donor cells and hub genes in recipient cells. The heatmap and correlation network visualized the correlation of DSCs-derived secreted encoding DEGs with macrophage polarization DEGs (A, B), macrophage-derived secreted encoding DEGs with DSC-DEGs (C, D), placenta-derived secreted encoding DEGs with macrophage polarization DEGs (E, F). *represents P<0.05, **represents P<0.01.
Furthermore, the correlation analysis between Mφ derived hub secreted-protein-encoding genes and hub genes of DSC confirmed that GSN was closely and positively correlated with TFPI2 (r=0.89, P<0.01), followed by FBLN5 with SNCA (r=0.88, P<0.01), FBLN5 with EFEMP1 (r=0.81, P<0.01), and FBLN5 with TFPI2 (r=-0.85, P<0.01). Besides, FBLN5 was closely negatively correlated with TFPI2 (r=-0.85, P<0.01), followed by CFB with TFPI2 (r=-0.84, P<0.01), and GSN with SNCA (r=-0.78, P<0.01). This result illustrated the close correlation between decidual Mφ and DSC. Heat maps and network plots were plotted to visualize gene correlations (Figures 9C, D).
The correlation analysis of placental derived hub secreted-protein-encoding genes and hub genes of decidual Mφ identified the highest positive correlation coefficient between CD163 and IFIT3 (r=0.75, P<0.01), followed by CD163 and C3, as well as LY96 and C3 (r=0.70, P<0.01). And SERPINA1 was the most negatively correlated with OAS1 (r=-0.61, P<0.01), followed by LTF with GBP1 (r=-0.50, P<0.01) (Figures 9E, F).
3.10 Identifying the diagnostic value of secreted proteins by machine learning and immunofluorescence analysis
Maternal-fetal interface-derived secreted proteins may be candidate biomarkers for the diagnosis of URSA. To determine their diagnostic value, LASSO and SVM-RFE algorithms were conducted and the ROC curves of each hub gene were plotted using R software. In DSCs, 8 genes and 13 genes were respectively obtained by LASSO and SVM-RFE, and there were three overlapped genes, including FGF9, IL1R2, NID2 (Figures 10A–C). Besides, we got 11 genes by LASSO regression and 4 genes by SVM-RFE from 22 secreted proteins encoding genes of decidual Mφ, the intersection of which contains three genes, including CFB, NID2, CXCL11 (Figures 10D–F). Similarly, we obtained 2 genes from 43 URSA placental secreted proteins by LASSO regression and 8 genes by SVM-RFE, with the intersecting genes being SFRP5 and SOSTDC1 (Figures 10G–I). The AUC of all these signature genes were above 0.65, indicating a high diagnostic accuracy (Supplementary Figure 2). In addition, we constructed nomogram with the three DSC-derived and three decidual Mφ-derived genes, respectively. And the calibration curves verified the predictive performance of the model, with high AUC value to verify its robustness (Figures 10J–O). Finally, we verified the obtained signature genes with decidual tissue by immunofluorescence colocalization analysis. The results showed that FGF9, IL1R2 and NID2 colocalized with the DSC marker vimentin. Furthermore, FGF9 were significantly increased in URSA (P<0.05), IL1R2 and NID2 were significantly decreased in URSA (P<0.05). Besides, CFB, CXCL11 and NID2 were colocalized with the Mφ marker CX3CR1, all of which were significantly decreased in URSA (P<0.05) (Figure 11).
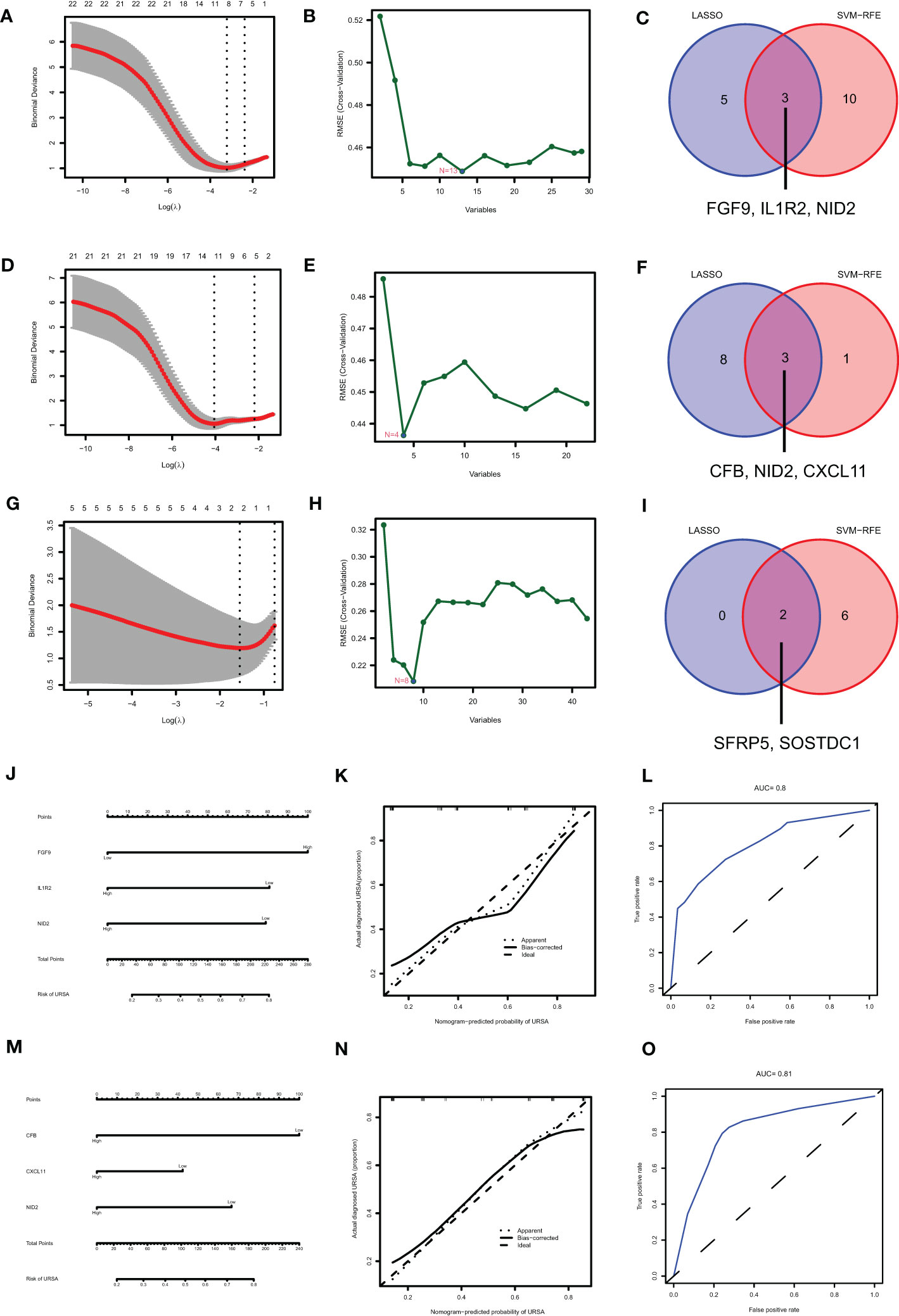
Figure 10 Identifying the diagnostic value of secreted proteins by machine learning. Results of LASSO, SVM-RFE algorithms and the intersection of the two for screening signature genes from DSC-DEGs (A–C), macrophage polarization DEGs (D–F) and placenta-DEGs (G–I). Nomograms of the 3 DSC-derived signature genes (J) and 3 decidual macrophage-derived genes (M), and the corresponding calibration curves (K, N) and ROCs (L, O).
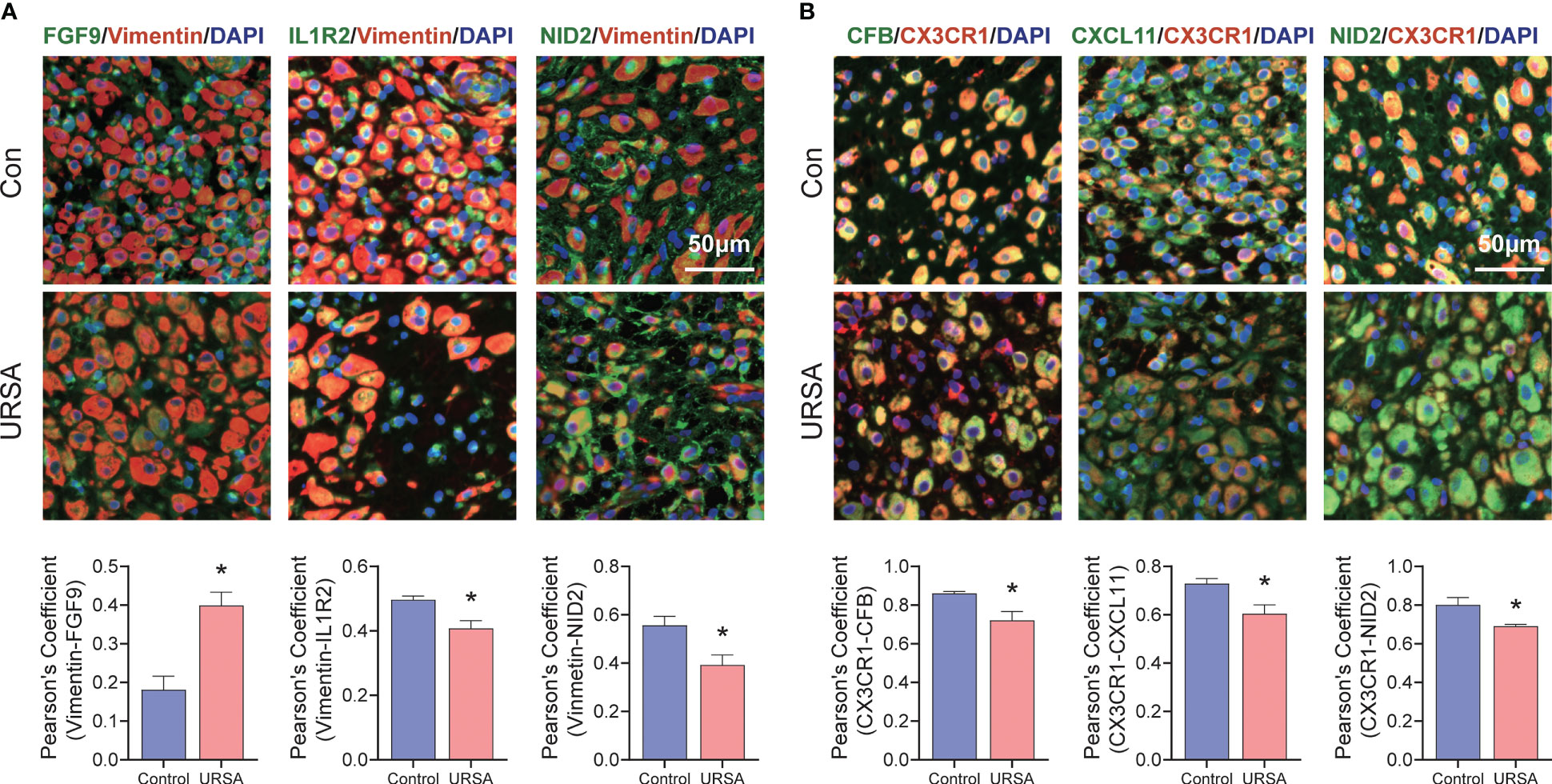
Figure 11 Validation of signature genes in clinical samples. (A) Immunofluorescence results of FGF9-Vimentin, IL1R2-Vimentin and NID2-Vimentin. (B) Immunofluorescence results of CFB-CX3CR1, CXCL11-CX3CR1 and NID2-CX3CR1. *represents P<0.05, compared with the control group; Here, Vimentin representative decidual stromal cell, CX3CR1 representative macrophage; Scale bar: 50μm.
4 Discussion
This study obtained 721 decidua-DEGs, 613 placenta-DEGs, and 510 Mφ polarization DEGs. Besides, hub genes were screened by CytoHubba and validated by qRT-PCR, including LYN, NNMT and SNCA in DSCs; IFIT1, IFIT3 and OAS2 in placenta; IFIT3, IFI27 and MX1 associated with Mφ polarization in URSA. Besides, functional analysis of the hub genes by GeneMANIA suggested that they were closely related to immune regulation. For example, LYN is an non-receptor tyrosine-protein kinase and is an essential regulator of immunoreceptor signaling, initiating both pro-inflammatory and suppressive signaling pathways in myeloid immune cells (e.g. macrophages, neutrophils, dendritic cells and monocytes) (31). Studies found that Toll-like Receptor (TLR) pathways, which trigger inflammatory responses to pathogen-associated molecular patterns (PAMPs) are also regulated by LYN. Downstream of TLRs, the adaptor proteins MyD88 and CARD9 and the transcription factor IRF5 generate hyperactivated signaling in LynKO myeloid cells and drive autoimmunity in LynKO mice. Furthermore, one study found a negative role for LYN in macrophage TLR4 signaling (32). Our study is the first to discover that LYN was decreased in DSCs of URSA, and it might be one of the molecular mechanisms that accounted for Mφ polarization disorder in URSA. Besides, for placental-derived IFIT1, IFIT3 (respectively encoding interferon induced protein with tetratricopeptide repeats 1 and 3) and OAS2 (encoding 2’-5’-Oligoadenylate Synthetase 2), along with IFIT3, IFIT27 and MX1 (encoding MX Dynamin Like GTPase 1) related to Mφ polarization, all these genes belong to the core responsive IFN-inducible genes. They are all closely associated with cytokine signaling in immune system, such as the pro-inflammatory phenotypic transformation of macrophages (33, 34). Currently, these genes are supposed to play an influential role in embryo implantation (35) and infection-related diseases during pregnancy (36). However, in the context of URSA, their expression patterns and biological significance have not been studied. Our results discovered that IFIT1, IFIT3, OAS2, IFIT27 and MX1 are elevated in URSA, which may represent the activation of immune and inflammatory responses in the maternal-fetal interface. This is consistent with the current findings (37). For instance, Wang et al. clarified that decidual stromal cell-derived decorin can polarize decidual macrophages toward the M1 phenotype and results in the occurrence of URSA (38). Gao et al. confirmed that the absence of G-CSF derived from trophoblasts weakened the suppression of trophoblasts against macrophages and may be a key factor in URSA occurrence (39). All these findings reveal that gene expression profile disorders in DSCs and trophoblast cells can disturb the immune landscape at the maternal-fetal interface, especially the communication with macrophages, which is an essential hint to explain the pathological mechanism of URSA. And our findings provide new targets and direction for research in this area.
To further systematically understand the immunomodulatory activities that decidua-DEGs and placenta-DEGs were involved in, we performed immune function enrichment analysis by CluGO, which displayed that these DEGs were mainly closely related to macrophage activity, such as affecting macrophage tolerance induction, regulation of macrophage antigen processing and presentation as well as macrophage activation. It has been confirmed that DSC-conditioned medium (CM) can promote macrophage survival and induce typical phenotype of decidual macrophages, and that DSC-CM also inhibit the pro-inflammatory effects of granulocyte-macrophage colony-stimulating factor (GM-CSF) by upregulating CD14, CD163 and CD209 (40). In addition, scholars have found that the level of nuclear factor-κB ligand (RANKL)/RANK levels at the maternal-fetal interface were abnormally decreased in patients with miscarriage, and RANKL receptor activator, secreted by trophoblasts and DSCs, could polarize dMϕ toward a M2 phenotype (41). Besides, Wu et al. demonstrated that overexpression of miR-410-5p in trophoblasts inhibited the polarization of M2 macrophages (42). Chen et al. discovered that reduced IL-10 derived from placental villi of URSA patients could activate endoplasmic reticulum stress of macrophages, thereby inhibiting M2 polarization (43). From the above studies we could project that DSCs and trophoblasts exerted significant effects on the phenotype and function of decidual macrophage. Combined with the results of differential expression analysis in this study, we hypothesized that DEGs at the maternal-fetal interface in URSA may affect macrophage polarization and be closely associated with pregnancy outcome in URSA.
To further understand the molecular mechanisms of intercellular communication that Mφ was involved in at the maternal-fetal interface, we performed enrichment analysis on Mφ polarization DEGs as well as the key module gene based on macrophage polarization-related genes by WGCNA. The results revealed that these genes regulated immune inflammatory signaling molecules and influenced cell function and fate, which might be an important way for macrophages to participate in intercellular communication at the maternal-fetal interface. Studies have confirmed that plenty of macrophages accumulated rapidly in the superficial stroma of endometrium during the period of “implantation window” period, suggesting that macrophages may involve in endometrial tolerance (44). For example, M2 macrophages are the primary source of decidual retinoic acid regulating regulated gap junction communication and promoting the differentiation of endometrial stromal cells (45). In addition, Sheng et al. uncovered that decidual macrophages could clear apoptotic DSCs through cytoplasmic action under the regulation of the IL-33/ST2 axis, the disorder of which was closely associated with URSA (46, 47). Hao et al. demonstrated that macrophages could affect nitric oxide levels and induce trophoblast apoptosis via protein l-arginine methyltransferase 3 (PRMT3)/asymmetrical dimethylarginine (ADMA), which was considered as a potential cause of recurrent miscarriage (48). According to the above studies, we could intuitively conclude that the regulation of DSCs and trophoblasts by decidual macrophages was essential for physiological pregnancy and that abnormalities in this dialogue mechanism are closely associated with pregnancy loss.
Considering that secreted proteins may play a central role in communicating intercellular signal transduction, we screened the genes encoding secreted proteins from DEGs of URSA. And finally, we obtained 29 secreted protein encoding DEGs in DSC-DEGs, 43 secreted protein encoding DEGs in placenta-DEGs, and 22 secreted proteins encoding DEGs in decidual macrophages. The functional enrichment analysis argued that these genes were mainly enriched in cytokine-cytokine receptor interaction, PI3K-Akt signaling pathway, NF-kappa B signaling pathway, and Wnt signaling pathway, etc. Taking the PI3K-Akt signaling pathway as an example, it is an intracellular signaling pathway that regulates metabolism, cell proliferation, survival, and growth in response to extracellular signals. Several studies have confirmed that the PI3K/Akt signaling pathway at the maternal-fetal interface is involved in the pathology of the URSA mechanism (49). Cui et al. suggested that the abnormal polarization of macrophages in patients with spontaneous abortion was related to the activation of the PI3K/Akt signaling pathway (50). Dai et al. observed that DSC could promote M2-like phenotype polarization by secreting growth arrest-specific factor 6 (rhGAS6) and activating the PI3K/Akt signaling pathway, and this mechanism was disrupted in patients with miscarriage (51). In addition, studies confirmed that G-CSF derived from M2 macrophages could promote the invasion and migration of trophoblasts by activating the PI3K/AKT/Erk1/2 pathway (52). It can be inferred that PI3K/AKT is a pivotal pathway for intercellular communication. Furthermore, multiple hub-secreted protein-encoding genes, such as FGF9, THBS1, MET, etc. were all enriched in the PI3K/Akt signaling pathway. For instance, FGFs (fibroblast growth factors) are pleiotropic growth factors and is able to coordinate cell proliferation and differentiation, which has been proven to activate PI3K/AKT signaling pathways (53). Thrombospondin 1 encoded by THBS1 is an antiproliferative gene that can reduce the levels of PI3K and Akt (54, 55). Thus, we speculated that these secreted proteins communicated between cells at the maternal-fetal interface by activating multiple intracellular signaling cascades of recipient cells, which are the focus of future research.
In addition, Spearman correlation analysis was performed between the hub gene of recipient cells and hub-secreted protein-encoding genes from DSC, decidual macrophages, and the placenta. Results displayed that EFEMP1 and FGF9 derived from DSC were correlated with C3 and GBP1 in decidual macrophages FBLN5 derived from macrophage might be closely associated with TFPI2 in DSC. CD163 and SERPINA1 derived from placenta might be closely related to IFIT3 and OAS1 in decidual macrophages, respectively. Taking negatively correlated FGF9 and GBP1 as an example, FGF9 has been confirmed to promote M2 polarization in a variety of diseases (56, 57), At the same time, GBP1 has been verified to participate in M1 polarization in a variety of infectious diseases (56, 58), and is considered to be capable of regulating the polarity of M1/M2 macrophages in the tumor microenvironment (59). Based on existing studies, we speculated that the abnormal expression profilings of FGF9 secreted by DSCs and GBP1 in macrophages might lead to the imbalance of M1/M2 polarization at the maternal-fetal interface of URSA. However, how FGF9 affects GBP 1 and how they participate in the regulation of macrophage polarization is required to be further studied. In addition, other highly correlated molecules are also worthy of further investigation so as to elucidate the molecular mechanism of cell communication at the maternal-fetal interface of URSA.
As the traditional diagnosis of URSA relies on exclusion diagnosis after extensive screening, exploring new diagnostic strategies is imperative. Considering the potential of exocrine proteins as diagnostic markers, we explored novel diagnostic markers and diagnostic models for URSA by LASSO and SVM-RFE algorithms based on secreted protein-encoding genes in DEGs. Results revealed that 3 secreted protein-encoding genes (FGF9, IL1R2 and NID2) derived from DSC, 3 secreted protein-encoding genes (CFB, NID2 and CXCL11) derived from macrophages and 2 secreted protein-encoding genes (SFRP5 and SOSTDC1) derived from placenta exhibited remarkable diagnostic value which were confirmed by ROC. In addition, nomograms was constructed based on DSC and decidual macrophage-derived biomarkers, and the predictive performance and robustness of the model were also confirmed. Furthermore, we also performed validation in clinical samples by immunofluorescence co-localization analysis, which further confirmed FGF9, IL1R2, CXCL11, CFB and NID2 as biomarkers. Besides, consistent with our findings, CXCL11 and FGF9 have been verified to be valuable for the diagnosis of URSA (60, 61). As for IL1R2, CFB and NID2, studies have confirmed that IL1R2, encoding interleukin 1 receptor type 2 can bind to interleukin 1 and prevent its inflammatory actions, which then inhibit the signaling and influx of the inflammatory cascade (62). And a positive correlation between IL-lβ concentrations and successful pregnancy outcome in IVF have been reported (63). CFB, encoding complement factors B, can activate C3, thereby initiating the alternative pathway, leading to self-injury and is associated with the dysregulation of placental development (64). A study by Xue et al. confirmed that high serum CFB concentration during mid-pregnancy is a potential predictor of the risk of preeclampsia in patients with gestational diabetes mellitus (65). With respect to NID2, encoding idogen-2 may be a biomarker for decidualization initiation and development (66), while decidualization deficiency is an essential pathological feature of URSA. All the above studies have revealed the close relationship between the abnormal expression of these markers and pregnancy complications, however, there is a gap in the studies in URSA. Our study focuses for the first time on the diagnostic value of these molecules in URSA. More large-scale clinical studies are necessary to confirm their diagnostic performance. Taken together, this innovation undoubtedly provides new inspiration for the diagnosis and treatment of URSA.
5 Conclusion
In conclusion, our study is the first time to explore cellular communication between DSCs, macrophages, and placental trophoblasts at the maternal-fetal interface with an integrated research strategies based on bioinformatics analysis and experiments. This study signified that abnormalities in intercellular communication involving macrophages was a potential pathological mechanism of URSA, and the potential specific molecular mechanism is shown in Figure 12. In addition, this is the first time we utilize machine learning and experiment validation to screen URSA diagnostic markers. Taking FGF9, IL1R2, NID2, CFB and CXCL11 as examples, these molecules may be instrumental in the sensitive diagnosis of URSA. Taken together, all our findings confirm the involvement of cellular communication at the maternal-fetal interface in URSA and its close correlation with macrophages, which provide new insights into the pathogenesis, diagnosis and treatment of URSA.
Data availability statement
The datasets presented in this study can be found in online repositories. The names of the repository/repositories and accession number(s) can be found in the article/Supplementary Material.
Author contributions
XZ and HZ: conceptualization. XZ and YJ: data curation. YJ and HZ: data analysis. XZ, SL and YZ: writing-original draft. HZ: reviewed the manuscript. All authors read and approved the final article. All authors contributed to the article.
Funding
This research was funded by TCM Science and Technology Project of Zhejiang Province (2023ZR038); 2022 Research Project of the Affiliated Hospital of Zhejiang Chinese Medical University(2022FSYYZQ16); Hangzhou Health Science and Technology Project (A20230675) to XZ; 2022 Research Project of the Affiliated Hospital of Zhejiang Chinese Medical University (2022FSYYZZ13) to HZ.
Acknowledgments
Special thanks to the researchers who provide the transcriptome microarrays to the GEO website, and to Yuepeng Jiang from Zhejiang Chinese Medical University for his contribution to the data analysis.
Conflict of interest
The authors declare that the research was conducted in the absence of any commercial or financial relationships that could be construed as a potential conflict of interest.
Publisher’s note
All claims expressed in this article are solely those of the authors and do not necessarily represent those of their affiliated organizations, or those of the publisher, the editors and the reviewers. Any product that may be evaluated in this article, or claim that may be made by its manufacturer, is not guaranteed or endorsed by the publisher.
Supplementary material
The Supplementary Material for this article can be found online at: https://www.frontiersin.org/articles/10.3389/fendo.2023.973930/full#supplementary-material
Supplementary Figure 1 | Data preprocessing and normalization. The box plots of the GSE26787 and GSE165004 datasets before (A) and after (B) normalization. Three- dimensional PCA cluster plot of the GSE26787 and GSE165004 datasets before (C) and after (D) normalization.Red nodes represent GSE26787 and yellow nodes represent GSE165004.
Supplementary Figure 2 | The receiver operating characteristic (ROC) curve of genes from the intersection of LASSO derived signature genes and SVM-RFE derived signature genes. (A-C)There signature genes in DSCs. (D-F) There signature genes in decidual Mφ. (G-H) Two signature genes in URSA placental.
Abbreviations
AUC, area under the ROC; DEGs, differentially expressed genes; DICs, decidual immune cells; DSCs, decidua stromal cells; Mφ, macrophages; PPI, protein-protein interaction; RSA, recurrent spontaneous abortion; URSA, unexplained recurrent spontaneous abortion; ROC, receiver operating characteristic curve; SVM-RFE, support vector machine-recursive feature elimination; TFs, transcription factors; WGCNA, weighted gene co-expression network analysis.
References
1. Practice Committee of the American Society for Reproductive Medicine. Evaluation and treatment of recurrent pregnancy loss: a committee opinion. Fertil Steril (2012) 98:1103–11. doi: 10.1016/j.fertnstert.2012.06.048
2. Bender AR, Christiansen OB, Elson J, Kolte AM, Lewis S, Middeldorp S, et al. ESHRE guideline: recurrent pregnancy loss. Hum Reprod Open (2018) 2018:y4. doi: 10.1093/hropen/hoy004
3. Stephenson MD. Frequency of factors associated with habitual abortion in 197 couples. Fertil Steril (1996) 66:24–9. doi: 10.1016/S0015-0282(16)58382-4
4. Li J, Wang L, Ding J, Cheng Y, Diao L, Li L, et al. Multiomics studies investigating recurrent pregnancy loss: an effective tool for mechanism exploration. Front Immunol (2022) 13:826198. doi: 10.3389/fimmu.2022.826198
5. Semmes EC, Coyne CB. Innate immune defenses at the maternal-fetal interface. Curr Opin Immunol (2022) 74:60–7. doi: 10.1016/j.coi.2021.10.007
6. Gellersen B, Brosens IA, Brosens JJ. Decidualization of the human endometrium: mechanisms, functions, and clinical perspectives. Semin Reprod Med (2007) 25:445–53. doi: 10.1055/s-2007-991042
7. Gellersen B, Brosens JJ. Cyclic decidualization of the human endometrium in reproductive health and failure. Endocr Rev (2014) 35:851–905. doi: 10.1210/er.2014-1045
8. Gao L, Xu QH, Ma LN, Luo J, Muyayalo KP, Wang LL, et al. Trophoblast-derived lactic acid orchestrates decidual macrophage differentiation via SRC/LDHA signaling in early pregnancy. Int J Biol Sci (2022) 18:599–616. doi: 10.7150/ijbs.67816
9. Xu L, Li Y, Sang Y, Li DJ, Du M. Crosstalk between trophoblasts and decidual immune cells: the cornerstone of maternal-fetal immunotolerance. Front Immunol (2021) 12:642392. doi: 10.3389/fimmu.2021.642392
10. Zhang YH, He M, Wang Y, Liao AH. Modulators of the balance between M1 and M2 macrophages during pregnancy. Front Immunol (2017) 8:120. doi: 10.3389/fimmu.2017.00120
11. Zhang Y, Ma L, Hu X, Ji J, Mor G, Liao A. The role of the PD-1/PD-L1 axis in macrophage differentiation and function during pregnancy. Hum Reprod (2019) 34:25–36. doi: 10.1093/humrep/dey347
12. True H, Blanton M, Sureshchandra S, Messaoudi I. Monocytes and macrophages in pregnancy: the good, the bad, and the ugly. Immunol Rev (2022) 308:77–92. doi: 10.1111/imr.13080
13. Houser BL, Tilburgs T, Hill J, Nicotra ML, Strominger JL. Two unique human decidual macrophage populations. J Immunol (2011) 186:2633–42. doi: 10.4049/jimmunol.1003153
14. Houser BL. Decidual macrophages and their roles at the maternal-fetal interface. Yale J Biol Med (2012) 85:105–18.
15. Jena MK, Nayak N, Chen K, Nayak NR. Role of macrophages in pregnancy and related complications. Arch Immunol Ther Exp (Warsz) (2019) 67:295–309. doi: 10.1007/s00005-019-00552-7
16. Shang Y, Wu S, Li S, Qin X, Chen J, Ding J, et al. Downregulation of EZH2 in trophoblasts induces decidual M1 macrophage polarization: a potential cause of recurrent spontaneous abortion. Reprod Sci (2022) 29:2820–8. doi: 10.1007/s43032-021-00790-1
17. Ding J, Zhang Y, Cai X, Diao L, Yang C, Yang J. Crosstalk between trophoblast and macrophage at the maternal-fetal interface: current status and future perspectives. Front Immunol (2021) 12:758281. doi: 10.3389/fimmu.2021.758281
18. Barrett T, Wilhite SE, Ledoux P, Evangelista C, Kim IF, Tomashevsky M, et al. NCBI GEO: archive for functional genomics data sets–update. Nucleic Acids Res (2013) 41:D991–95. doi: 10.1093/nar/gks1193
19. Bardou P, Mariette J, Escudié F, Djemiel C, Klopp C. Jvenn: an interactive Venn diagram viewer. BMC Bioinf (2014) 15:293. doi: 10.1186/1471-2105-15-293
20. Szklarczyk D, Morris JH, Cook H, Kuhn M, Wyder S, Simonovic M, et al. The STRING database in 2017: quality-controlled protein-protein association networks, made broadly accessible. Nucleic Acids Res (2017) 45:D362–68. doi: 10.1093/nar/gkw937
21. Shannon P, Markiel A, Ozier O, Baliga NS, Wang JT, Ramage D, et al. Cytoscape: a software environment for integrated models of biomolecular interaction networks. Genome Res (2003) 13:2498–504. doi: 10.1101/gr.1239303
22. Chin CH, Chen SH, Wu HH, Ho CW, Ko MT, Lin CY. cytoHubba: identifying hub objects and sub-networks from complex interactome. BMC Syst Biol (2014) 8 Suppl 4:S11. doi: 10.1186/1752-0509-8-S4-S11
23. Franz M, Rodriguez H, Lopes C, Zuberi K, Montojo J, Bader GD, et al. GeneMANIA update 2018. Nucleic Acids Res (2018) 46:W60–64. doi: 10.1093/nar/gky311
24. Bindea G, Mlecnik B, Hackl H, Charoentong P, Tosolini M, Kirilovsky A, et al. ClueGO: a cytoscape plug-in to decipher functionally grouped gene ontology and pathway annotation networks. Bioinformatics (2009) 25:1091–93. doi: 10.1093/bioinformatics/btp101
25. Liao Y, Wang J, Jaehnig EJ, Shi Z, Zhang B. WebGestalt 2019: gene set analysis toolkit with revamped UIs and APIs. Nucleic Acids Res (2019) 47:W199–205. doi: 10.1093/nar/gkz401
27. Friedman J, Hastie T, Tibshirani R. Regularization paths for generalized linear models via coordinate descent. J Stat Softw (2010) 33:1–22. doi: 10.18637/jss.v033.i01
28. Huang ML, Hung YH, Lee WM, Li RK, Jiang BR. SVM-RFE based feature selection and taguchi parameters optimization for multiclass SVM classifier. ScientificWorldJournal (2014) 2014:795624. doi: 10.1155/2014/795624
29. Zhao X, Jiang Y, Ren J, Wang Y, Zhao Y, Feng X. Deciphering the mechanism of bushen huoxue decotion on decidualization by intervening autophagy via AMPK/mTOR/ULK1: a novel discovery for URSA treatment. Front Pharmacol (2022) 13:794938. doi: 10.3389/fphar.2022.794938
30. Ma H, He Z, Chen J, Zhang X, Song P. Identifying of biomarkers associated with gastric cancer based on 11 topological analysis methods of CytoHubba. Sci Rep (2021) 11:1331. doi: 10.1038/s41598-020-79235-9
31. Brian BT, Freedman TS. The src-family kinase Lyn in immunoreceptor signaling. Endocrinology (2021) 162. doi: 10.1210/endocr/bqab152
32. Keck S, Freudenberg M, Huber M. Activation of murine macrophages via TLR2 and TLR4 is negatively regulated by a Lyn/PI3K module and promoted by SHIP1. J Immunol (2010) 184:5809–18. doi: 10.4049/jimmunol.0901423
33. Sun J, Zhang Q, Liu X, Shang X. Downregulation of interferon-induced protein with tetratricopeptide repeats 3 relieves the inflammatory response and myocardial fibrosis of mice with myocardial infarction and improves their cardiac function. Exp Anim (2021) 70:522–31. doi: 10.1538/expanim.21-0060
34. Wang A, Kang X, Wang J, Zhang S. IFIH1/IRF1/STAT1 promotes sepsis associated inflammatory lung injury via activating macrophage M1 polarization. Int Immunopharmacol (2023) 114:109478. doi: 10.1016/j.intimp.2022.109478
35. Crites BR, Carr SN, Anderson LH, Matthews JC, Bridges PJ. Form of dietary selenium affects mRNA encoding interferon-stimulated and progesterone-induced genes in the bovine endometrium and conceptus length at maternal recognition of pregnancy. J Anim Sci (2022) 100:skac137. doi: 10.1093/jas/skac137
36. Van Goor A, Pasternak A, Walker K, Hong L, Malgarin C, MacPhee DJ, et al. Differential responses in placenta and fetal thymus at 12 days post infection elucidate mechanisms of viral level and fetal compromise following PRRSV2 infection. BMC Genomics (2020) 21:763. doi: 10.1186/s12864-020-07154-0
37. Chen P, Zhou L, Chen J, Lu Y, Cao C, Lv S, et al. The immune atlas of human deciduas with unexplained recurrent pregnancy loss. Front Immunol (2021) 12:689019. doi: 10.3389/fimmu.2021.689019
38. Wang L, Wang H, Luo J, Xie T, Mor G, Liao A. Decorin promotes decidual M1-like macrophage polarization via mitochondrial dysfunction resulting in recurrent pregnancy loss. Theranostics (2022) 12:7216–36. doi: 10.7150/thno.78467
39. Gao P, Zha Y, Wei L, Zhou X, Zhu S, Zhang H, et al. G-CSF: a vehicle for communication between trophoblasts and macrophages which may cause problems in recurrent spontaneous abortion. Placenta (2022) 121:164–72. doi: 10.1016/j.placenta.2022.03.125
40. Lindau R, Vondra S, Spreckels J, Solders M, Svensson-Arvelund J, Berg G, et al. Decidual stromal cells support tolerance at the human foetal-maternal interface by inducing regulatory M2 macrophages and regulatory T-cells. J Reprod Immunol (2021) 146:103330. doi: 10.1016/j.jri.2021.103330
41. Meng YH, Zhou WJ, Jin LP, Liu LB, Chang KK, Mei J, et al. RANKL-mediated harmonious dialogue between fetus and mother guarantees smooth gestation by inducing decidual M2 macrophage polarization. Cell Death Dis (2017) 8:e3105. doi: 10.1038/cddis.2017.505
42. Wu S, Liu H, Zhou M, Shang Y, Luo L, Chen J, et al. The miR-410-5p /ITGA6 axis participates in the pathogenesis of recurrent abortion by regulating the biological function of trophoblast. J Reprod Immunol (2022) 152:103647. doi: 10.1016/j.jri.2022.103647
43. Chen X, Song QL, Li ZH, Ji R, Wang JY, Ge C, et al. Deletion of ACLY disrupts histone acetylation and IL-10 secretion in trophoblasts, which inhibits M2 polarization of macrophages: a possible role in recurrent spontaneous abortion. Oxid Med Cell Longev (2022) 2022:5216786. doi: 10.1155/2022/5216786
44. Tan W, Chen L, Guo L, Ou X, Xie D, Quan S. Relationship between macrophages in mouse uteri and angiogenesis in endometrium during the peri-implantation period. Theriogenology (2014) 82:1021–27. doi: 10.1016/j.theriogenology.2014.07.025
45. Rajakumar A, Kane MA, Yu J, Jones JW, Qu H, Badell M, et al. Alternatively activated macrophages are the primary retinoic acid-producing cells in human decidua. Reprod Sci (2020) 27:334–41. doi: 10.1007/s43032-019-00030-7
46. Sheng YR, Hu WT, Shen HH, Wei CY, Liu YK, Ma XQ, et al. An imbalance of the IL-33/ST2-AXL-efferocytosis axis induces pregnancy loss through metabolic reprogramming of decidual macrophages. Cell Mol Life Sci (2022) 79:173. doi: 10.1007/s00018-022-04197-2
47. Sheng YR, Hu WT, Wei CY, Tang LL, Liu YK, Liu YY, et al. IL-33/ST2 axis affects the polarization and efferocytosis of decidual macrophages in early pregnancy. Am J Reprod Immunol (2018) 79:e12836. doi: 10.1111/aji.12836
48. Hao F, Tang LC, Sun JX, Li WX, Zhao Y, Xu XH, et al. Decreased nitric oxide content mediated by asymmetrical dimethylarginine and protein l-arginine methyltransferase 3 in macrophages induces trophoblast apoptosis: a potential cause of recurrent miscarriage. Hum Reprod (2021) 36:3049–61. doi: 10.1093/humrep/deab225
49. Zhang S, Ding J, Wang J, Yin T, Zhang Y, Yang J. CXCL5 downregulation in villous tissue is correlated with recurrent spontaneous abortion. Front Immunol (2021) 12:717483. doi: 10.3389/fimmu.2021.717483
50. Cui L, Jin X, Xu F, Wang S, Liu L, Li X, et al. Circadian rhythm-associated rev-erbα modulates polarization of decidual macrophage via the PI3K/Akt signaling pathway. Am J Reprod Immunol (2021) 86:e13436. doi: 10.1111/aji.13436
51. Dai JC, Yang JY, Chang RQ, Liang Y, Hu XY, Li H, et al. GAS6-mediated dialogue between decidual stromal cells and macrophages is essential for early pregnancy maintenance by inducing M2-like polarization and cell proliferation of decidual macrophages. Mol Hum Reprod (2022) 28:gaac006. doi: 10.1093/molehr/gaac006
52. Ding J, Wang J, Cai X, Yin T, Zhang Y, Yang C, et al. Granulocyte colony-stimulating factor in reproductive-related disease: function, regulation and therapeutic effect. BioMed Pharmacother (2022) 150:112903. doi: 10.1016/j.biopha.2022.112903
53. Tang L, Wu M, Lu S, Zhang H, Shen Y, Shen C, et al. Fgf9 negatively regulates bone mass by inhibiting osteogenesis and promoting osteoclastogenesis Via MAPK and PI3K/AKT signaling. J Bone Miner Res (2021) 36:779–91. doi: 10.1002/jbmr.4230
54. Ma Y, Dong C, Chen X, Zhu R, Wang J. Silencing of miR-20b-5p exerts inhibitory effect on diabetic retinopathy via inactivation of THBS1 gene induced VEGF/Akt/PI3K pathway. Diabetes Metab Syndr Obes (2021) 14:1183–93. doi: 10.2147/DMSO.S299143
55. Huang T, Wang L, Liu D, Li P, Xiong H, Zhuang L, et al. FGF7/FGFR2 signal promotes invasion and migration in human gastric cancer through upregulation of thrombospondin-1. Int J Oncol (2017) 50:1501–12. doi: 10.3892/ijo.2017.3927
56. Wu S, Xu R, Zhu X, He H, Zhang J, Zeng Q, et al. The long noncoding RNA LINC01140/miR-140-5p/FGF9 axis modulates bladder cancer cell aggressiveness and macrophage M2 polarization. Aging (Albany NY) (2020) 12:25845–64. doi: 10.18632/aging.202147
57. Hegab AE, Ozaki M, Kagawa S, Hamamoto J, Yasuda H, Naoki K, et al. Tumor associated macrophages support the growth of FGF9-induced lung adenocarcinoma by multiple mechanisms. Lung Cancer (2018) 119:25–35. doi: 10.1016/j.lungcan.2018.02.015
58. Zhang S, Chu C, Wu Z, Liu F, Xie J, Yang Y, et al. IFIH1 contributes to M1 macrophage polarization in ARDS. Front Immunol (2020) 11:580838. doi: 10.3389/fimmu.2020.580838
59. Liu Z, Sun J, Gong T, Tang H, Shen Y, Liu C. The prognostic and immunological value of guanylate-binding proteins in lower-grade glioma: potential markers or not? Front Genet (2021) 12:651348. doi: 10.3389/fgene.2021.651348
60. Aktas A, Berberoglu Z, Fidan Y, Yazıcı AC, Koc G, Aral Y, et al. Higher levels of circulating CXCL-9 and CXCL-11 in euthyroid women with autoimmune thyroiditis and recurrent spontaneous abortions. Gynecol Endocrinol (2014) 30:157–60. doi: 10.3109/09513590.2013.871514
61. Othman R, Omar MH, Shan LP, Shafiee MN, Jamal R, Mokhtar NM. Microarray profiling of secretory-phase endometrium from patients with recurrent miscarriage. Reprod Biol (2012) 12:183–99. doi: 10.1016/s1642-431x(12)60085-0
62. Langmia IM, Apalasamy YD, Omar SZ, Mohamed Z. Interleukin 1 receptor type 2 gene polymorphism is associated with reduced risk of preterm birth. J Matern Fetal Neonatal Med (2016) 29:3347–50. doi: 10.3109/14767058.2015.1125466
63. Kreines FM, Nasioudis D, Minis E, Irani M, Witkin SS, Spandorfer S. IL-1β predicts IVF outcome: a prospective study. J Assist Reprod Genet (2018) 35:2031–35. doi: 10.1007/s10815-018-1296-0
64. Regal JF, Burwick RM, Fleming SD. The complement system and preeclampsia. Curr Hypertens Rep (2017) 19:87. doi: 10.1007/s11906-017-0784-4
65. Xue Y, Yang N, Ma L, Gu X, Jia K. Predictive value of the complement factors b and h for women with gestational diabetes mellitus who are at risk of preeclampsia. Pregnancy Hypertens (2022) 30:210–14. doi: 10.1016/j.preghy.2022.10.010
Keywords: Unexplained recurrent spontaneous abortion, maternal-fetal interface, macrophage, decidual stromal cells, placenta, intercellular communication
Citation: Zhao X, Jiang Y, Luo S, Zhao Y and Zhao H (2023) Intercellular communication involving macrophages at the maternal-fetal interface may be a pivotal mechanism of URSA: a novel discovery from transcriptomic data. Front. Endocrinol. 14:973930. doi: 10.3389/fendo.2023.973930
Received: 20 June 2022; Accepted: 04 May 2023;
Published: 17 May 2023.
Edited by:
Eytan R. Barnea, BioIncept, LLC, United StatesCopyright © 2023 Zhao, Jiang, Luo, Zhao and Zhao. This is an open-access article distributed under the terms of the Creative Commons Attribution License (CC BY). The use, distribution or reproduction in other forums is permitted, provided the original author(s) and the copyright owner(s) are credited and that the original publication in this journal is cited, in accordance with accepted academic practice. No use, distribution or reproduction is permitted which does not comply with these terms.
*Correspondence: Hongli Zhao, ejZobEAxNjMuY29t
†These authors have contributed equally to this work