- 1Department of Diagnostic and Therapeutic Ultrasonography, Tianjin Medical University Cancer Institute and Hospital, National Clinical Research Center for Cancer, Key Laboratory of Cancer Prevention and Therapy, Tianjin’s Clinical Research Center for Cancer, Tianjin, China
- 2Department of Thyroid and Neck Cancer, Tianjin Medical University Cancer Institute and Hospital, National Clinical Research Center of Cancer, Key Laboratory of Cancer Prevention and Therapy, Tianjin’s Clinical Research Center for Cancer, Tianjin, China
- 3Department of Ultrasonography, Cangzhou Hospital of Integrated Traditional Chinese and Western Medicine of Hebei Province, Cangzhou, Hebei, China
- 4Department of Breast and Thyroid Surgery, Tianjin Union Medical Center, Tianjin, China
Objective: Central lymph node metastasis (CLNM) is a predictor of poor prognosis for papillary thyroid carcinoma (PTC) patients. The options for surgeon operation or follow-up depend on the state of CLNM while accurate prediction is a challenge for radiologists. The present study aimed to develop and validate an effective preoperative nomogram combining deep learning, clinical characteristics and ultrasound features for predicting CLNM.
Materials and methods: In this study, 3359 PTC patients who had undergone total thyroidectomy or thyroid lobectomy from two medical centers were enrolled. The patients were divided into three datasets for training, internal validation and external validation. We constructed an integrated nomogram combining deep learning, clinical characteristics and ultrasound features using multivariable logistic regression to predict CLNM in PTC patients.
Results: Multivariate analysis indicated that the AI model-predicted value, multiple, position, microcalcification, abutment/perimeter ratio and US-reported LN status were independent risk factors predicting CLNM. The area under the curve (AUC) for the nomogram to predict CLNM was 0.812 (95% CI, 0.794-0.830) in the training cohort, 0.809 (95% CI, 0.780-0.837) in the internal validation cohort and 0.829(95%CI, 0.785-0.872) in the external validation cohort. Based on the analysis of the decision curve, our integrated nomogram was superior to other models in terms of clinical predictive ability.
Conclusion: Our proposed thyroid cancer lymph node metastasis nomogram shows favorable predictive value to assist surgeons in making appropriate surgical decisions in PTC treatment.
Introduction
The global prevalence of thyroid cancer has sharply increased in the past few decades, and it is also increasing in China. Papillary thyroid carcinoma (PTC) is the most common type of thyroid cancer, which accounts for approximately 80% of all thyroid carcinomas (1–3). PTC is usually an indolent cancer, and the 10-year survival rate of PTC can reach 93% if standardized treatment is accepted (4). However, PTC easily metastasizes to cervical lymph nodes, and the prevalence of central lymph node metastasis (CLNM) can reach as high as 40% to 60% (5, 6). CLNM status is an important risk factor for high recurrence rates and low patient survival (7, 8). A key and controversial problem in thyroid cancer management is if prophylactic central lymph node dissection (CLND) is necessary. CLND is recommended for patients who are suspected of CLNM in preoperative assessment. Some researchers demonstrated that prophylactic CNLD can more accurately stage tumors and reduce recurrence rates in patients with intermediate and high-risk thyroid cancer, while others argue that patients may gain no benefit or some temporary morbidity (such as hypocalcemia and spinal accessory nerve dysfunction), and endoscopic lymphadenopathy (9, 10). Therefore, most studies do not recommend the routine use of prophylactic CLND in PTC (11, 12).
According to the American Thyroid Association guidelines, preoperative neck ultrasound (US) is recommended to evaluate cervical lymph nodes, but the diagnosis rate is not accurate; although it has high diagnostic value for accessing lateral lymph node metastasis(LLNM), it has relatively low sensitivity in the diagnosis of CLNM (13–15).Thus, an effective and non-invasive way to predict CLNM before surgery is urgently needed to provide optimal surgical treatments.
Thyroid cancer nomograms are widely used as a prognostic tool to understand the nature of thyroid cancer lesions, assess the unknown risk of disease and predict the possible outcome of treatment. Several established models based on the clinical and ultrasound characteristics to predict CLNM, but their performance is not satisfactory and not adaptable to actual clinical work (16–19). Currently, the use of artificial intelligence (AI) in medicine has gained interest, particularly in analyzing and diagnosing medical images (20, 21). AI models provide another opinion to assist radiologists in interpreting the images by improving the accuracy and consistency of disease diagnosis and by reducing the time to output results. Several studies have investigated deep learning to diagnose thyroid malignancy and have achieved better performance than human readers (22–25). Therefore, the prospect of deep learning in predicting lymph node metastasis is worth exploring. To our knowledge, deep learning has not yet been integrated with clinical and ultrasound factors to construct a combined nomogram to predict CLNM in PTC patients.
Recently, we developed a thyroid cancer lymph node metastasis AI system-based deep learning model to predict the status of cervical lymph nodes. The purpose of the present study was to (1) evaluate the value of this system to predict CLNM and to (2) combine it with additional clinical and ultrasound characteristics to establish a more robust and generalizable model for the prediction of CLNM in PTC patients. The flowchart of our model development is shown in Figure 1.
Materials and methods
Patients
In this study, a total of 3359 PTC patients who had undergone first-time thyroidectomy to treat thyroid carcinoma at two centers (center#1: Tianjin Medical University Cancer Institute and Hospital; center#2: Binzhou Medical University Hospital) were enrolled from March 2011 to June 2018. Data from center#1 were randomly divided into two groups as follows: 70% for a training cohort (n=2114) and 30% for an internal validation cohort(n=906), respectively. Data from center#2 (n = 339) were used for an external validation cohort. This study was approved by the Ethics Committee of Tianjin Medical University Cancer Institute and Hospital(No. bc2020190), and the requirements for informed consent were waived.
Total thyroidectomy or thyroid lobectomy with therapeutic or prophylactic lymph node dissection was performed for patients. Ipsilateral central neck lymph node dissection (CLND) was routinely performed, total thyroidectomy with bilateral CLND was performed for patients with bilateral PTC or patients with clinical evidence of contralateral CLNM. At the center#1, the inclusion criteria were as follows: (1) the diagnosis of the primary site was pathologically proven PTC by postoperative pathology; (2) cervical lymph node dissection was performed and pathologically examined; (3) all patients had undergone preoperative cervical ultrasonography to assess the status of the primary site and central cervical lymph nodes; and (4) BRAFV600E analysis was necessary. The exclusion criteria were as follows: (1) the patient had undergone preoperative radiofrequency ablation, radiotherapy or chemotherapy; (2) other malignant tumors were present; and (3) the preoperative ultrasound image was absent or insufficient. All pathology specimens were reviewed retrospectively by two or more experienced pathologists. At the center#2, we have the same inclusion criteria, but the BRAFV600E analysis is not within the scope of the record.
Ultrasonography and image analysis
All patients had undergone ultrasound examination before surgery to assess the characteristics of the nodules and status of the central lymph node (CLN). All ultrasound images were reviewed and interpreted by two experienced radiologists (Dr. Wei X and Dr. Zhang S with 15 and 30 years of experience in thyroid cancer US diagnosis, respectively).
All ultrasound examinations used a Phillips EPIQ 5, IU 22, HD11 (Philips Healthcare, Eindhoven, The Netherlands) equipped with a high-frequency linear array probe (5–12 MHz) to perform thyroid cross-section, longitudinal section and cervical lymph node scan. We reviewed the size, number, location and sonographic features of the thyroid lesions in the longitudinal and transverse axes. The size of the tumor was the largest diameter of the tumor and was divided into the following groups: ≤0.5 cm, 0.5~1.0 cm, 1.0~2.0 cm and ≥2.0 cm. If there was more than one nodule that was suspected as malignant, we defined it as multiple. In multiple cases, the size of the tumor was classified according to the diameter of the largest tumor. The position of the lesion was evaluated from the following four aspects: upper, middle, lower and isthmus. We further divided several typical ultrasound features of nodules according to the American College of Radiology Thyroid Imaging Reporting and Data System (ACR TI-RADS) (26), including the composition (mixed cystic and solid or solid), echogenicity (isoechoic or hyperechoic, hypoechoic or very hypoechoic), shape (taller-than-wide<1 or taller-than-wide≥1), margin (clear or unclear) and microcalcifications (yes or no). In addition, Hashimoto’s thyroiditis and the abutment of the thyroid capsule were evaluated on the basis of ultrasound images. Hashimoto’s thyroiditis manifests as uneven echogenicity of the thyroid parenchyma on ultrasonography, with a few or multiple lamellar hypoechoic areas showing grid-like changes. The measurement of abutment or the perimeter in a thyroid lesion was calculated by the average ratio (1/2) on the transverse + longitudinal section of a nodule. Based on our previous study (27), we selected the 1/4 (25%) perimeter of the thyroid lesion as the cutoff value.
The ultrasound features of the involved lymph nodes were based on various criteria. Lymph nodes showing one or more suspicious US features (internal microcalcifications, cystic changes, hyperechogenicity, round shape, loss of hilar echogenicity or the presence of peripheral flow) on ultrasound images were regarded as US-reported metastatic CLN according to ACR TI-RADS (26).
BRAFV600E mutation analysis
Fine needle aspiration biopsies were repeated at least three times per aspiration using a 22-gauge needle for BRAFV600E mutation assessment in cytology. Immediately after aspiration, the needle and syringe were washed with 1 ml of normal saline, and samples with sufficient numbers of tumor cells were used for real-time PCR analysis (28).
Thyroid cancer lymph node metastasis AI system
The fully automated deep learning model used in this research was developed by the author, ZHZ, and a cloud-based artificial intelligence diagnosis platform, named the thyroid cancer lymph node metastasis AI system, was established based on this model. This model uses the Mask R-CNN framework as a computer vision framework for nodules segmentation and has the following capabilities: uses a convolutional neural network as a backbone; automatically learns and trains according to the range of artificially labeled nodules; recognizes the relevant features of the nodule; and uses the relevant features as quantifiable parameters (29). The nodule image was then enlarged to 1.5 times the pixel size, and the SE-ResNeXt-50 model was used as the classification model (30). We used mirrored, rotated, folded and normalized data augmentation during the training process and applied random dropout to prevent the model from overfitting. ResNeXt improves the accuracy without increasing the complexity of the parameters while also reducing the number of hyperparameters. After predicting each image of the patient’s nodule by the SE-ResNeXt-50 neural network, we obtained the corresponding labels [benign nodules (BN), malignant nodules without cervical lymph node metastasis (MN-LN(-)), and malignant nodules with cervical lymph node metastasis (MN-LN(+))] of the patient. A logistic regression model was then established based on the relationships among cervical lymph node status, sex, age and model prediction results. Finally, we obtained the final AI model-predicted value of the patient’s probability of cervical lymph node metastasis. The cloud-based AI diagnosis platform can be accessed on the following website: http://thyai.zzinf.com/.
Our proposed model was implemented using Python (version 3.7.6; https://www.python.org/), DL toolkit Pytorch (version 1.5.1; https://pytorch.org/) and OpenCV (version 4.4.0; https://opencv.org). All experiments were conducted on a workstation equipped with a NVDIA 2080Ti GPU.
Development of a thyroid cancer lymph node metastasis nomogram
We used data in the training cohort to construct the nomogram, which included the AI-predicted value, clinical characteristics and ultrasound features. Pearson’s chi-squared test and t-test were applied for univariate analysis. Statistically significant factors in univariate analysis were incorporated into multivariate logistic regression analysis to construct the predictive model. Statistical significance was decided by a criterion of two-sided P<0.05. A nomogram was then developed based on the multivariate analysis in the training cohort.
Model evaluation and comparison
To fully evaluate our thyroid cancer lymph node metastasis nomogram, we compared the model with the following other methods: (1) the thyroid cancer lymph node metastasis AI system; (2) a clinical model based on the age, sex and remaining ultrasound features of the primary site in the multiple logistic regression analysis; and (3) the US-reported CLN status based on the involved lymph nodes.
Statistical analysis
The Mann-Whitney U test and chi-square test were separately used to compare the differences in continuous variables and categorical variables. The model predictions were assessed by sensitivity, specificity, positive predictive value, negative predictive value, the area under the curve (AUC) of the receiver operating characteristic (ROC) curve and 95% confidence interval (CI) as well as calibration curves in both the training and validation cohorts. Delong test was used to compare different AUC. Calibration plot analysis was performed by bootstrapping with 1,000 replications. Decision curve analysis was used to evaluate clinical usefulness and net benefits. All analyses were performed using R statistical software (version 3.3.3; www.R-project.org). P<0.05 was considered statistically significant.
Results
Clinical characteristics and ultrasound features of the patients
The clinical and ultrasound characteristics of the patient are summarized in Table 1.
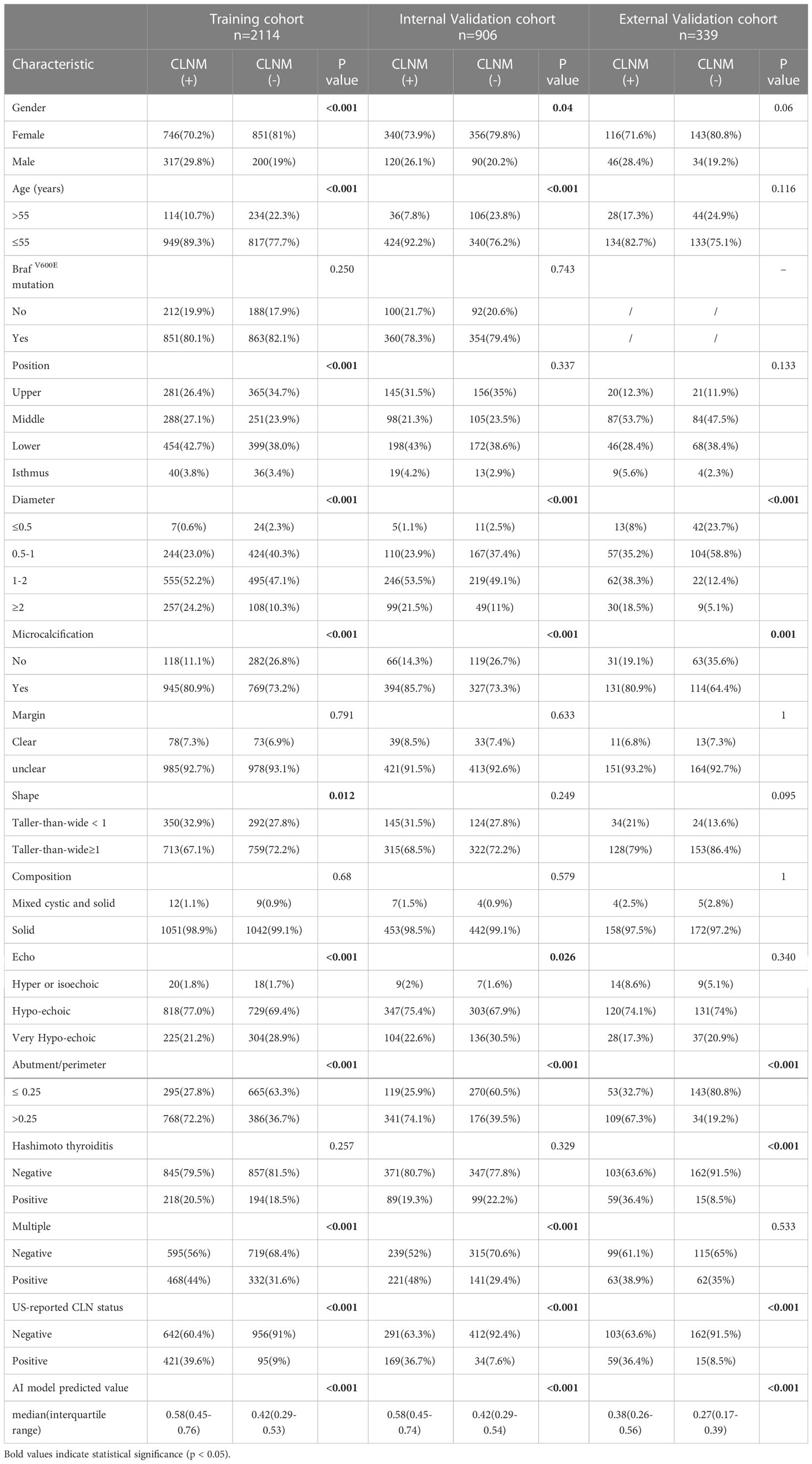
Table 1 Clinicopathological and sonographic characteristics of patients in PTC by central lymph node status.
Univariate and multivariate analyses of CLNM risk factors
Table 1 shows the association between CLNM and several risk factors in PTC patients. According to univariate analysis, gender (P<0.001), age (P<0.001), tumor size (P<0.001), tumor position (P<0.001), multiple (P<0.001), taller-than-wide (P=0.012), echo (P<0.001), microcalcification (P<0.001), abutment/perimeter ratio>0.25 (P<0.001), US-reported CLN status (P<0.001) and AI model-predicted value (P<0.001) were closely related to CLNM. Multivariate regression analysis showed that AI model-predicted value (P<0.001), tumor position (P<0.001), multifocality (P<0.001), microcalcification (P=0.001), abutment/perimeter ratio>0.25 (P<0.001) and US-reported CLN status (P<0.001) were significantly associated with a high risk of CLNM (Table 2).
Construction and validation of the thyroid cancer lymph node metastasis nomogram
Multivariable analysis demonstrated that the AI model-predicted value, multifocality, tumor position, microcalcification, abutment/perimeter ratio and US-reported CLN status remained important predictors of CLNM metastasis in PTC patients. Thus, a thyroid cancer lymph node metastasis nomogram incorporating these six predictors was constructed (Figure 2). The nomogram showed that the AI model-predicted value was the largest contributor to the scores followed by US-reported CLN status and abutment/perimeter ratio>0.25. Microcalcification, tumor position and multifocality showed a modest impact on the model. The AUC for the nomogram to predict CLNM was 0.812 (95% CI, 0.794-0.830) in the training cohort, 0.809 (95% CI, 0.780-0.837) in the internal validation cohort, and 0.829 (95%CI, 0.785-0.872) in the external validation cohort (Figure 3A–C). The calibration curve of this nomogram presented good agreement between the bias-corrected prediction and ideal reference line with an additional 1000 bootstraps in the training and two validation cohorts (Figure 4A–C).
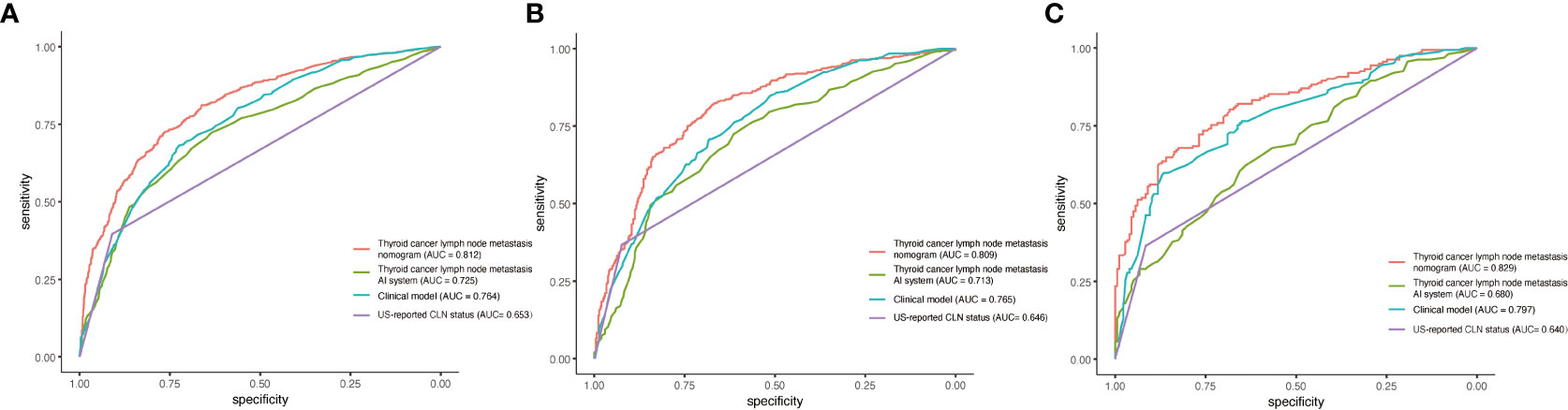
Figure 3 Performance of the thyroid cancer lymph node metastasis nomogram, thyroid cancer lymph node metastasis AI system, clinical model and US-reported CLN status in PTC patients. (A) ROC curve for predicting CLNM in the training cohort. (B) ROC curve for predicting CLNM in the internal validation cohort. (C) ROC curve for predicting CLNM in the external validation cohort.
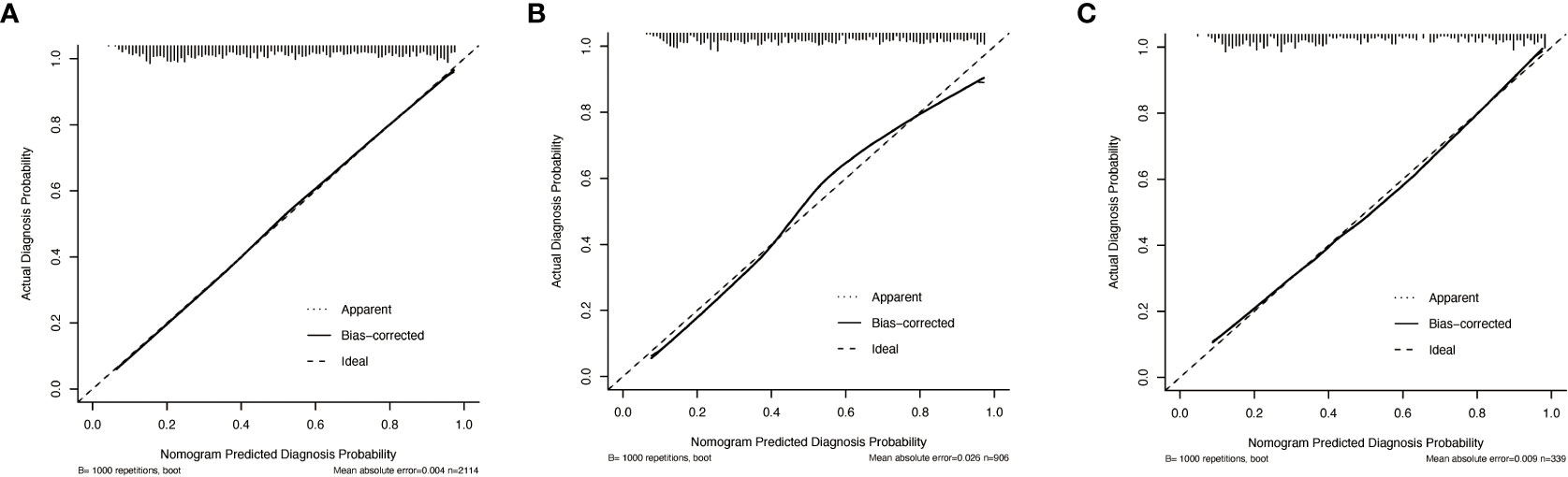
Figure 4 Calibration curves of the thyroid cancer lymph node metastasis nomogram, thyroid cancer lymph node metastasis AI system, clinical model and US-reported CLN status in PTC patients. (A) Calibration curves for predicting CLNM in the training cohort. (B) Calibration curves for predicting CLNM in the internal validation cohort. (C) Calibration curves for predicting CLNM in the external validation cohort.
Performance of the thyroid cancer lymph node metastasis nomogram
We also compared the thyroid cancer lymph node metastasis nomogram with the thyroid cancer lymph node metastasis AI system, clinical model and US-reported CLN status. In the training cohort, the nomogram yielded remarkable performance (sensitivity = 72.4%, specificity = 76.5% and AUC = 0.812; 95% CI: 0.794-0.830) and was superior to the thyroid cancer lymph node metastasis AI system (sensitivity = 67.3%, specificity = 68.9% and AUC = 0.725; 95% CI: 0.703-0.746), clinical model (sensitivity =68.9%, specificity = 72.1% and AUC = 0.764; 95% CI: 0.744-0.784) and US-reported CLN status (sensitivity =39.6%, specificity = 91.0% and AUC = 0.653; 95% CI: 0.636-0.670). In the internal validation cohort, the performance of the nomogram also achieved reasonable performance (sensitivity = 73.9%, specificity = 76.7% and AUC = 0.809; 95% CI: 0.780-0.837) and was better than that of the thyroid cancer lymph node metastasis AI system (sensitivity =50.9%, specificity = 83.2% and AUC = 0.713; 95% CI: 0.679-0.746), clinical model (sensitivity =78.1%, specificity = 60.5%, and AUC = 0.765; 95% CI: 0.734-0.795) and US-reported CLN status (sensitivity =36.7%, specificity = 92.4%, and AUC = 0.646; 95% CI: 0.620-0.671). In the external validation cohort, the thyroid cancer lymph node metastasis nomogram still shows the most outstanding performance, compared with several other models. (Table 3)
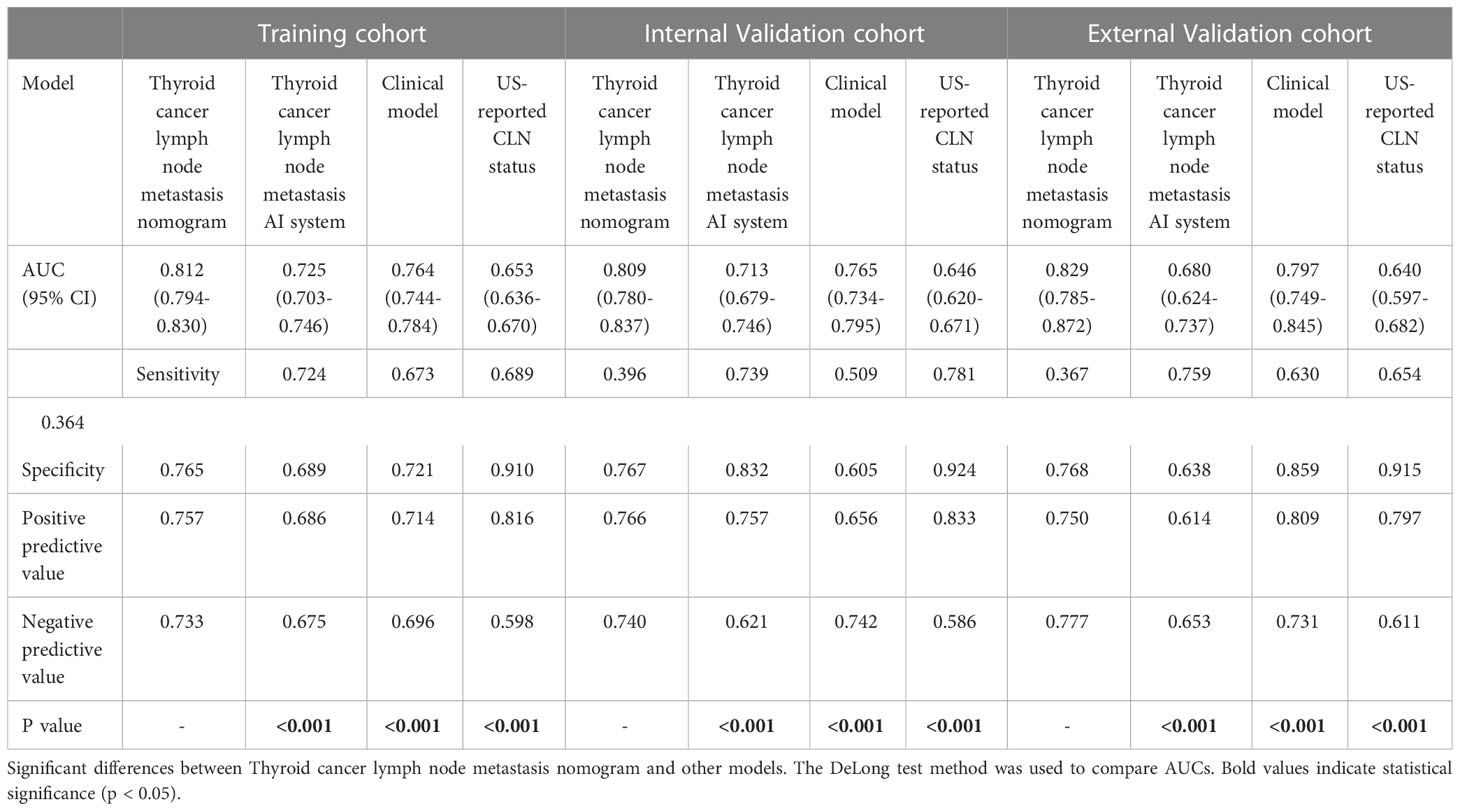
Table 3 Identification performance of thyroid cancer lymph node metastasis nomogram, thyroid cancer lymph node metastasis AI system, clinical model and US-reported CLN status in the training cohort, internal validation cohort and external validation cohort.
We also performed decision curve analysis (DCA) to compare the clinical usability and benefits of predicting the risk of CLNM of this model with those of the traditional ultrasound methods. The DCA curves of the new nomogram showed greater net benefits across a range of CLNMs risks in the three cohorts than the other models (Figure 5A–C).
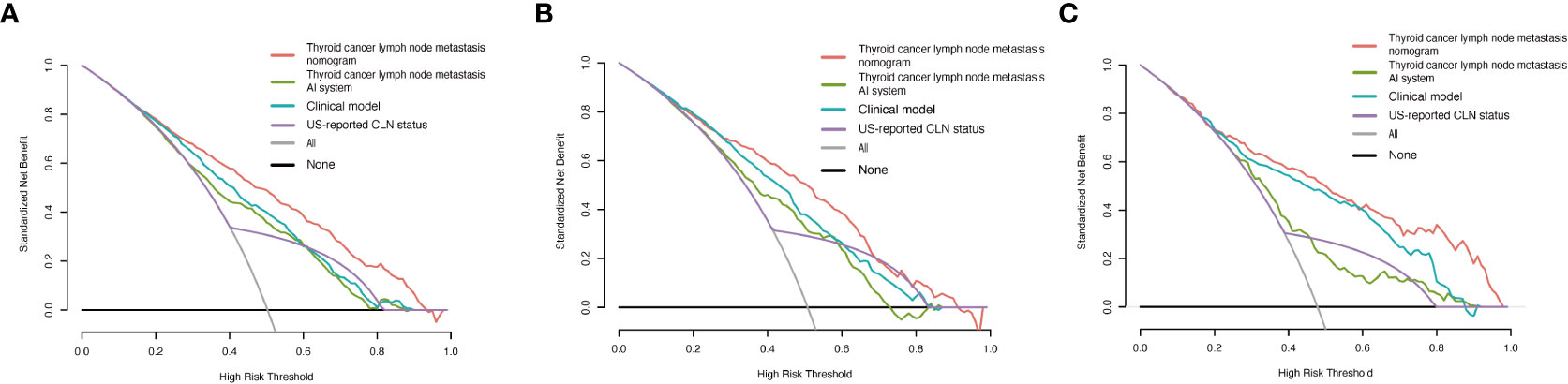
Figure 5 Decision curve analysis of the thyroid cancer lymph node metastasis nomogram, thyroid cancer lymph node metastasis AI system, clinical model and US-reported CLN status in PTC patients. (A) Decision curve analysis for predicting CLNM in the training cohort. (B) Decision curve analysis for predicting CLNM in the internal validation cohort. (C) Decision curve analysis for predicting CLNM in the external validation cohort.
Discussion
Lymph node metastasis is common among PTC patients. Thyroidectomy combined with therapeutic lymph node dissection has become a preferred initial surgical strategy for PTC patients with clinically positive lymph nodes, but disagreement persists regarding whether patients with negative lymph node metastasis on ultrasound should undergo preventive central neck dissection. Total thyroidectomy plus prophylactic CLND and total thyroidectomy alone did not differ significantly in local recurrence rates or postoperative complication rates between PTC patients with clinically node-negative. Therefore, prophylactic CLND may not be required if total thyroidectomy is planned (31, 32).
Ultrasonography is one of the most commonly used non-invasive tools applied to the examination of thyroid nodules, but it still has some limitations. For example, ultrasonography relies greatly on the radiologists’ work experience, and less experienced radiologists may show less accuracy in the identification of CLNM. Therefore, it is necessary to develop a reliable and noninvasive preoperative tool to predict CLNM in PTC.
In the present study, we performed a systematic and comprehensive analysis of the clinical and ultrasound characteristics of 3359 patients from two medical centers with PTC and incorporated our established thyroid cancer lymph node metastasis AI system. We then developed and validated a thyroid cancer lymph node metastasis nomogram to predict CLNM. The AUC value of our model was exceeded 80% in both the training and two validation cohorts. Additionally, the calibration plot showed that the predicted and observed metastasis risks of CLNM were in good agreement. Thus, our model has good practical effects in clinical applications.
Nomograms are widely used for the prediction of cancer prognosis, mainly for their ability to simplify statistical prediction models to estimate the probability of occurrence of an event (e.g., death or recurrence) and to make predictions based on the actual situation of individual patients (33). In general, one of the most conventional methods for predicting lymph node metastasis in PTC is to establish a clinical model that integrates the statistical analysis information of ultrasound and clinicopathological factors. For instance, Wang et al. established a nomogram to predict CLNM in PTMC patients and considered that age < 55 years, male sex, tumor size 0.5-1.0 cm, multifocal lesions, extrathyroidal extension and lateral lymph node metastasis are independent risk factors for CLNM (34). Lu et al. screened six variables for demographic and clinicopathological characteristics as potential risk factors and further constructed a model for lymph node involvement based on the Surveillance, Epidemiology, and End Results (SEER) Program (35). Although a large amount of data was included in the above studies, the performance of these models was not satisfactory and lacked detailed analysis of several clinical features, such as the absence of BRAFV600E mutation testing.
Deep learning consists of a neural network including many layers and features extracted from the original input image. Currently, deep learning is widely used in medical imaging to achieve computer-aided diagnosis, providing assisted diagnostic suggestions in clinical settings and obtaining more accurate results faster than radiologists. In our previous research, we performed a series of studies on artificial intelligence diagnosis of the thyroid in the area of ultrasound. We applied a large dataset of over 300,000 images to build a DCNN model, which had ameliorative accuracy, sensitivity and specificity in the classification of thyroid nodules. The area under the curve exceeded 90% for both the internal test set and the external test set (36). In addition, we constructed a deep CNN model, named the Brief Efficient Thyroid Network (BETNET), for the localization and classification of thyroid nodules, which precisely and intuitively shows the nodular characteristic area with a higher weight within the neural network (22). The abovementioned studies demonstrate the feasibility of applying a deep convolutional neural network in patients with PTC. Thus, we aimed to establish a deep learning platform for the prediction of lymph node status in patients with PTC before surgery.
There have been a few studies applying deep learning models to predict CLNM from ultrasound images. However, these previous studies are based on traditional machine learning-based radiomics methods that extract intensity, boundary, texture and wavelet features from ultrasound images and establish the relationship between these high-throughput features and lymph node status (37). Jiang et al. established a radiomics nomogram based on the SWE radiomics signature and achieved an area under the curve of nearly 85% (37). Although this model achieved better results, the sample size of the patients was only 237, suggesting that the diagnostic value of the shear-wave elastography images in thyroid ultrasound is debatable (38). Additionally, due to the imbalance of medical development in different regions, this model based on SWE radiomics it is not universal to each hospital.
In our preliminary work, we successfully established a cloud-based artificial intelligence diagnosis platform called the thyroid cancer lymph node metastasis AI system based on deep learning to accurately localize thyroid nodules on ultrasound and automatically distinguish the nature of nodules and lymph node status, which included age and sex as independent risk factors affecting cervical lymph node metastasis. Due to the different scanning angles and positions, the object area may pan, and its shape may change, causing differences between images of the same category. Unlike previous deep learning models that predict classification results from a single image, our system simultaneously inputs multiple images of different sections of a patient’s lesion and precisely visualizes and locates the nodes to determine the status of the lymph nodes in a comprehensive manner, which is highly interpretable and can be better implemented for clinical applications. In this study, we further evaluated the diagnostic performance of this system in assessing the risk of CLNM.
We then performed detailed statistical analysis of the other clinical and ultrasound characteristics of the patients. Ultrasound parameters are descriptive and distinguishable, and they can be used as a reference for ACR classification. Certain independent risk factors in the clinical features also assist in the determination of lymph node metastasis. The results of the present suggested that multifocality, tumor position, microcalcification, abutment/perimeter>0.25, AI-predicted value and US-reported CLN status were significantly associated with CLNM. These independent risk factors were incorporated to form a new combined model, and a nomogram called the thyroid cancer lymph node metastasis nomogram was developed at the same time. In addition, the external validation further proves the universality of our model. Although the diagnostic performance of the thyroid cancer lymph node metastasis AI system is slightly lower than that of clinical model, AI-predicted value as the largest contributor to scores in the nomogram.
According to ACR TI-RADS guidelines, calcifications within thyroid cancer are classified into four types as follows: microcalcifications, macrocalcifications, marginal calcifications and noncalcifications. Many studies have reported that the formation of microcalcifications is caused by the rapid proliferation of cancer cells and is significantly associated with the incidence of CLNM (39). In our previous studies, we evaluated cervical lymph node metastases based on the tumor abutment/perimeter ratio of the primary site, providing a relatively high reference value to assess the risk of papillary thyroid microcarcinoma metastasis (27). As expected, the 1/4 (25%) perimeter of the thyroid lesion was closely related to CLNM in PTC patients. Moreover, in the present study of Chinese patients, we identified a cohort of more than 3000 patients with classic PTC to explore the relationship between BRAFV600E mutation and CLNM. To our knowledge, this is the largest cohort of samples included in relevant research, and we conclude that BRAFV600E has limited value as an indicator of the risk of CLNM in PTC.
The present study had several limitations. First, regardless of training, internal validation or external validation cohort, our model performed well. These results need to be validated with a larger prospective cohort to test the application value of our model in clinical practice. Second, the immunohistochemical staining patterns and results of fine-needle aspiration may affect the prediction of lymph node status. Thus, we should incorporate these factors into a future study. Finally, our model was only applied to PTC and not to other thyroid cancer subtypes. Future research will focus on other thyroid cancer subtypes, including medullary thyroid carcinoma and follicular thyroid carcinoma.
Conclusion
In the present study, we developed a thyroid cancer lymph node metastasis nomogram combining deep learning, clinical characteristics and ultrasound features. This nomogram will serve as a noninvasive tool to predict CLNM in patients with PTC that will assist inexperienced radiologists in diagnosing the status of lymph nodes and provide effective guidance to surgeons in preoperative diagnosis.
Data availability statement
The raw data supporting the conclusions of this article will be made available by the authors, without undue reservation.
Ethics statement
This study was approved by the Ethics Committee of Tianjin Medical University Cancer Institute and Hospital (No. bc2020190), and the requirements for informed consent were waived.
Author contributions
LC, SZ, MG, and XW designed this study. YZ, JZ, XQW and XC conducted the experiment and interpreted the data. HZ, XC, QG and LH analyzed the data. All authors contributed to the article and approved the submitted version.
Funding
This study has received funding by National Natural Science Foundation of China (#81771852), Tianjin Major Science and Technology Project of Artificial Intelligence (#18ZXZNSY00300), Tianjin Health Research Project (#ZD20018, #QN20018), Tianjin Research Innovation Project for Postgraduate Students(#2020YJSS178) and the Science and Technology Development Fund of Tianjin Education Commission for Higher Education(#2021KJ194).
Acknowledgments
We thank Guanghe Cui for his help in providing external validation cohort from Binzhou Medical University Hospital.
Conflict of interest
The authors declare that the research was conducted in the absence of any commercial or financial relationships that could be construed as a potential conflict of interest.
Publisher’s note
All claims expressed in this article are solely those of the authors and do not necessarily represent those of their affiliated organizations, or those of the publisher, the editors and the reviewers. Any product that may be evaluated in this article, or claim that may be made by its manufacturer, is not guaranteed or endorsed by the publisher.
Supplementary material
The Supplementary Material for this article can be found online at: https://www.frontiersin.org/articles/10.3389/fendo.2023.964074/full#supplementary-material
References
1. Byrd JK, Yawn RJ, Wilhoit CST, Sora ND, Meyers L, Fernandes J, et al. Well differentiated thyroid carcinoma: current treatment. Curr Treat options Oncol (2012) 13(1):47–57. doi: 10.1007/s11864-011-0173-1
2. Lim H, Devesa SS, Sosa JA, Check D, Kitahara CM. Trends in thyroid cancer incidence and mortality in the united states, 1974-2013. JAMA (2017) 317(13):1338–48. doi: 10.1001/jama.2017.2719
3. Morris LGT, Sikora AG, Tosteson TD, Davies L. The increasing incidence of thyroid cancer: The influence of access to care. Thyroid: Off J Am Thyroid Assoc (2013) 23(7):885–91. doi: 10.1089/thy.2013.0045
4. Yu QA, Ma DK, Liu KP, Wang P, Xie CM, Wu YH, et al. Clinicopathologic risk factors for right paraesophageal lymph node metastasis in patients with papillary thyroid carcinoma. J endocrinol Invest (2018) 41(11):1333–8. doi: 10.1007/s40618-018-0874-4
5. Pereira JA, Jimeno J, Miquel J, Iglesias M, Munné A, Sancho JJ, et al. Nodal yield, morbidity, and recurrence after central neck dissection for papillary thyroid carcinoma. Surgery (2005) 138(6):1095–100, discussion 1100-1. doi: 10.1016/j.surg.2005.09.013
6. Roh JL, Kim JM, Park CI. Central lymph node metastasis of unilateral papillary thyroid carcinoma: Patterns and factors predictive of nodal metastasis, morbidity, and recurrence. Ann Surg Oncol (2011) 18(8):2245–50. doi: 10.1245/s10434-011-1600-z
7. Suh YJ, Kwon H, Kim SJ, Choi JY, Lee KE, Park YJ, et al. Factors affecting the locoregional recurrence of conventional papillary thyroid carcinoma after surgery: A retrospective analysis of 3381 patients. Ann Surg Oncol (2015) 22(11):3543–9. doi: 10.1245/s10434-015-4448-9
8. Grogan RH, Kaplan SP, Cao H, Weiss RE, Degroot LJ, Simon CA, et al. A study of recurrence and death from papillary thyroid cancer with 27 years of median follow-up. Surgery (2013) 154(6):1436–46; discussion 1446-7. doi: 10.1016/j.surg.2013.07.008
9. Medas F, Canu GL, Cappellacci F, Anedda G, Conzo G, Erdas E, et al. Prophylactic central lymph node dissection improves disease-free survival in patients with intermediate and high risk differentiated thyroid carcinoma: A retrospective analysis on 399 patients. Cancers (Basel) (2020) 12(6):1658. doi: 10.3390/cancers12061658
10. Polistena A, Monacelli M, Lucchini R, Triola R, Conti C, Avenia S, et al. Surgical morbidity of cervical lymphadenectomy for thyroid cancer: A retrospective cohort study over 25 years. Int J Surg (2015) 21:128–34. doi: 10.1016/j.ijsu.2015.07.698
11. Kim SK, Woo JW, Lee JH, Park I, Choe JH, Kim JH, et al. Prophylactic central neck dissection might not be necessary in papillary thyroid carcinoma: Analysis of 11,569 cases from a single institution. J Am Coll Surg (2016) 222(5):853–64. doi: 10.1016/j.jamcollsurg.2016.02.001
12. Sippel RS, Robbins SE, Poehls JL, Pitt SC, Chen H, Leverson G, et al. A randomized controlled clinical trial: No clear benefit to prophylactic central neck dissection in patients with clinically node negative papillary thyroid cancer. Ann Surg (2020) 272(3):496–503. doi: 10.1097/sla.0000000000004345
13. Kim E, Park JS, Son K-R, Kim J-H, Jeon SJ, Na DG. Preoperative diagnosis of cervical metastatic lymph nodes in papillary thyroid carcinoma: Comparison of ultrasound, computed tomography, and combined ultrasound with computed tomography. Thyroid (2008) 18(4):411–8. doi: 10.1089/thy.2007.0269
14. Roh JL, Park JY, Kim JM, Song CJ. Use of preoperative ultrasonography as guidance for neck dissection in patients with papillary thyroid carcinoma. J Surg Oncol (2009) 99(1):28–31. doi: 10.1002/jso.21164
15. Jeong HS, Baek CH, Son YI, Choi JY, Kim HJ, Ko YH, et al. Integrated 18F-FDG PET/CT for the initial evaluation of cervical node level of patients with papillary thyroid carcinoma: Comparison with ultrasound and contrast-enhanced CT. Clin Endocrinol (Oxf) (2006) 65(3):402–7. doi: 10.1111/j.1365-2265.2006.02612.x
16. Wang Y, Guan Q, Xiang J. Nomogram for predicting central lymph node metastasis in papillary thyroid microcarcinoma: A retrospective cohort study of 8668 patients. Int J Surg (2018) 55:98–102. doi: 10.1016/j.ijsu.2018.05.023
17. Feng JW, Hong LZ, Wang F, Wu WX, Hu J, Liu SY, et al. A nomogram based on clinical and ultrasound characteristics to predict central lymph node metastasis of papillary thyroid carcinoma. Front Endocrinol (Lausanne) (2021) 12:666315. doi: 10.3389/fendo.2021.666315
18. Zou Y, Shi Y, Liu J, Cui G, Yang Z, Liu M, et al. A comparative analysis of six machine learning models based on ultrasound to distinguish the possibility of central cervical lymph node metastasis in patients with papillary thyroid carcinoma. Front Oncol (2021) 11:656127. doi: 10.3389/fonc.2021.656127
19. Wu Y, Rao K, Liu J, Han C, Gong L, Chong Y, et al. Machine learning algorithms for the prediction of central lymph node metastasis in patients with papillary thyroid cancer. Front Endocrinol (Lausanne) (2020) 11:577537. doi: 10.3389/fendo.2020.577537
20. Zhou W, Yang Y, Yu C, Liu J, Duan X, Weng Z, et al. Ensembled deep learning model outperforms human experts in diagnosing biliary atresia from sonographic gallbladder images. Nat Commun (2021) 12(1):1259. doi: 10.1038/s41467-021-21466-z
21. Penzkofer T, Padhani AR, Turkbey B, Haider MA, Huisman H, Walz J, et al. ESUR/ESUI position paper: Developing artificial intelligence for precision diagnosis of prostate cancer using magnetic resonance imaging. Eur Radiol (2021) 31(12):9567–9578. doi: 10.1007/s00330-021-08021-6
22. Zhu J, Zhang S, Yu R, Liu Z, Gao H, Yue B, et al. An efficient deep convolutional neural network model for visual localization and automatic diagnosis of thyroid nodules on ultrasound images. Quant Imaging Med Surg (2021) 11(4):1368–80. doi: 10.21037/qims-20-538
23. Wei X, Gao M, Yu R, Liu Z, Gu Q, Liu X, et al. Ensemble deep learning model for multicenter classification of thyroid nodules on ultrasound images. Med Sci monit (2020) 26:e926096. doi: 10.12659/MSM.926096
24. Chen Y, Li D, Zhang X, Jin J, Shen Y. Computer aided diagnosis of thyroid nodules based on the devised small-datasets multi-view ensemble learning. Med Image Anal (2021) 67:101819. doi: 10.1016/j.media.2020.101819
25. Liu T, Guo Q, Lian C, Ren X, Liang S, Yu J, et al. Automated detection and classification of thyroid nodules in ultrasound images using clinical-knowledge-guided convolutional neural networks. Med Image Anal (2019) 58:101555. doi: 10.1016/j.media.2019.101555
26. Tessler FN, Middleton WD, Grant EG, Hoang JK, Berland LL, Teefey SA, et al. Reporting and data system (TI-RADS): White paper of the ACR TI-RADS committee. J Am Coll Radiol (2017) 14(5):587–95. doi: 10.1016/j.jacr.2017.01.046
27. Wei X, Wang M, Wang X, Zheng X, Li Y, Pan Y, et al. Prediction of cervical lymph node metastases in papillary thyroid microcarcinoma by sonographic features of the primary site. Cancer Biol Med (2019) 16(3):587–94. doi: 10.20892/j.issn.2095-3941.2018.0310
28. Zarkesh M, Zadeh-Vakili A, Akbarzadeh M, Nozhat Z, Fanaei SA, Hedayati M, et al. BRAF V600E mutation and microRNAs are helpful in distinguishing papillary thyroid malignant lesions: Tissues and fine needle aspiration cytology cases. Life Sci (2019) 223:166–73. doi: 10.1016/j.lfs.2019.03.034
29. He K, Gkioxari G, Dollar P, Girshick R. Mask R-CNN. IEEE Trans Pattern Anal Mach Intell (2020) 42(2):386–97. doi: 10.1109/tpami.2018.2844175
30. Hu J, Shen L, Sun G. (2018). Squeeze-and-excitation networks, in: Proceedings of the IEEE conference on computer vision and pattern recognition. doi: 10.48550/arXiv.1709.01507
31. Alsubaie KM, Alsubaie HM, Alzahrani FR, Alessa MA, Abdulmonem SK, Merdad MA, et al. Prophylactic central neck dissection for clinically node-negative papillary thyroid carcinoma. Laryngoscope (2022) 132(6):1320–8. doi: 10.1002/lary.29912
32. Ahn JH, Kwak JH, Yoon SG, Yi JW, Yu HW, Kwon H, et al. A prospective randomized controlled trial to assess the efficacy and safety of prophylactic central compartment lymph node dissection in papillary thyroid carcinoma. Surgery (2022) 171(1):182–9. doi: 10.1016/j.surg.2021.03.071
33. Iasonos A, Schrag D, Raj GV, Panageas KS. How to build and interpret a nomogram for cancer prognosis. J Clin Oncol (2008) 26(8):1364–70. doi: 10.1200/jco.2007.12.9791
34. Yang Z, Heng Y, Lin J, Lu C, Yu D, Tao L, et al. Nomogram for predicting central lymph node metastasis in papillary thyroid cancer: A retrospective cohort study of two clinical centers. Cancer Res Treat (2020) 52(4):1010–8. doi: 10.4143/crt.2020.254
35. Lu S, Zhao R, Ni Y, Ding J, Qiu F, Peng Y, et al. Development and validation of a nomogram for preoperative prediction of cervical lymph node involvement in thyroid microcarcinoma. Aging (Albany NY) (2020) 12(6):4896–906. doi: 10.18632/aging.102915
36. Li X, Zhang S, Zhang Q, Wei X, Pan Y, Zhao J, et al. Diagnosis of thyroid cancer using deep convolutional neural network models applied to sonographic images: A retrospective, multicohort, diagnostic study. Lancet Oncol (2019) 20(2):193–201. doi: 10.1016/s1470-2045(18)30762-9
37. Jiang M, Li C, Tang S, Lv W, Yi A, Wang B, et al. Nomogram based on shear-wave elastography radiomics can improve preoperative cervical lymph node staging for papillary thyroid carcinoma. Thyroid (2020) 30(6):885–97. doi: 10.1089/thy.2019.0780
38. Han DY, Sohn YM, Seo M, Yun SJ, Park WS, Jeon SH, et al. Shear-wave elastography in thyroid ultrasound: Can be a predictor of extrathyroidal extension and cervical lymph node metastasis in papillary thyroid carcinoma? Med (Baltimore) (2020) 99(52):e23654. doi: 10.1097/md.0000000000023654
Keywords: deep learning, papillary thyroid carcinoma, central lymph node metastasis, nomogram, ultrasound
Citation: Chang L, Zhang Y, Zhu J, Hu L, Wang X, Zhang H, Gu Q, Chen X, Zhang S, Gao M and Wei X (2023) An integrated nomogram combining deep learning, clinical characteristics and ultrasound features for predicting central lymph node metastasis in papillary thyroid cancer: A multicenter study. Front. Endocrinol. 14:964074. doi: 10.3389/fendo.2023.964074
Received: 08 June 2022; Accepted: 10 February 2023;
Published: 21 February 2023.
Edited by:
Terry Francis Davies, Icahn School of Medicine at Mount Sinai, United StatesReviewed by:
Erivelto Martinho Volpi, Centro de Referência no Ensino do Diagnóstico por Imagem, BrazilZeyu Wu, Guangdong Provincial People’s Hospital, China
Copyright © 2023 Chang, Zhang, Zhu, Hu, Wang, Zhang, Gu, Chen, Zhang, Gao and Wei. This is an open-access article distributed under the terms of the Creative Commons Attribution License (CC BY). The use, distribution or reproduction in other forums is permitted, provided the original author(s) and the copyright owner(s) are credited and that the original publication in this journal is cited, in accordance with accepted academic practice. No use, distribution or reproduction is permitted which does not comply with these terms.
*Correspondence: Xi Wei, d2VpeGlAdG11LmVkdS5jbg==
†These authors have contributed equally to this work and share first authorship